Tumor Evolution: Finding the mutations that drive resistance
Despite being a major cause of death, cancer is still far from being fully understood. Most cancer treatments target mutations that happen during the very early stages of the disease, as these genetic variants will be present in the majority of tumor cells (Bailey et al., 2018). However, not all cells inside a tumor are genetically identical, and this heterogeneity is one of the biggest problems in cancer therapy (Gatenby and Brown, 2018). As tumors evolve and become more heterogeneous, some cancer cells acquire new mutations that make them resistant to certain treatments, and drugs targeting these sites could prevent cancers from reoccurring (Figure 1A).
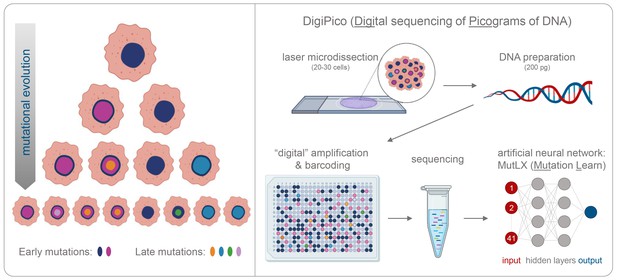
Detecting rare mutations in tumor cells.
(A) Cancer usually begins with a mutation (dark blue shape in top cell) in a single tumor cell that it passes on to its daughter cells following division. A daughter cell can then gain a new mutation (shown in pink) that it passes on to its progeny. These cells also divide and acquire new mutations (shown in different colors). Over time this leads to a population of cells that are genetically distinct from each other: the initial mutation is present in all the cells, whereas mutations that occurred later are present in a smaller number of cells (bottom row). (B) Now, KaramiNejadRanjba et al. have created a sequencing approach called DigiPico that can identify mutations that occur later during tumor evolution. First, cell material is extracted from a small group of 20–30 cells using laser microdissection and diluted down to single molecules of DNA which are plated into 384 individual wells (top panel). The DNA molecule in each well is amplified to create individual libraries, which are then combined and sequenced (bottom panel). After sequencing, an artificial neural network called MutLX is applied to the data to determine which of the genetic variants put into the algorithm (shown in dark red) are mutations that appear later during tumor evolution (shown in dark blue) and which are artefacts generated by the amplification process. Figure created using BioRender (BioRender.com).
Whole-genome sequencing is a widely used strategy for identifying genetic variants which are present in the majority of tumor cells. However, mutations that arise later during tumor evolution are almost impossible to detect using this approach, as they only appear in a handful of cells (Figure 1A; Hrdlickova et al., 2017; Turajlic et al., 2019). Although whole-genome sequencing of single cells can uncover these rarer mutations, this technique requires a high number of individual cells, which are often difficult to collect and expensive to sequence. In addition, amplifying the small quantities of DNA extracted from tumor cells often introduces errors that can be mistaken for cancer mutations. Now, in eLife, Ahmed Ahmed from the University of Oxford and co-workers from the United Kingdom and Germany – including Eli M Carrami as first author – report how they developed a new whole-genome sequencing technique that can overcome these limitations and identify mutations that occur later in tumor evolution (Carrami et al., 2020).
In the first stage of this approach, termed DigiPico, genomic material was extracted from a small group of tumor cells and diluted down to 384 single molecules of DNA (Figure 1B). Each DNA molecule was then individually amplified to create a library of repeated fragments which were sequenced into reads. Carrami et al. hypothesized that if a mutation is randomly generated during the amplification process, it will only appear in a small proportion of the repeated fragments. However, if a mutation is truly related to the cancer sample, it will be present from the start and will therefore be detected in all the reads that result from amplifying the single DNA molecule. Moreover, true mutations that arise during tumor evolution will be distributed regularly across the different libraries, while artefacts that arise during amplification will appear more randomly.
The sequencing data were analyzed using common processing and mapping algorithms and compared to whole-genome sequencing data from the tumor material and blood of the same patient. This identified genomic variants that were unique to the DigiPico data, and true positive mutations that were also found in the majority of tumor cells and the blood of patients. KaramNejadRanjbar et al. then employed a neural network called MutLX, which uses a form of artificial intelligence, to determine whether the unique variants detected by DigiPico were artefacts or cancer related mutations (LeCun et al., 2015). Excluding artefacts dramatically decreased the number of candidates for mutations that occurred late in the evolution of the tumor.
Experimentally validating some of the detected mutants revealed that together, DigiPico and MutlX, are able to eliminate false positives and identify rare mutations. Using the new technique, KaramNejadRanjbar et al. were able to discover a hyper-mutation event called kataegis in a recurring ovarian tumor (Chan and Gordenin, 2015), which conventional sequencing approaches had not been able to detect.
One of the main advantages of this method is that it can identify rare mutations that appear late during tumor evolution from just a small sample of cells. Furthermore, the precision and robustness of the technique also makes it easier to characterize current mutational processes, even in cancers which have a high number of genomic re-arrangements, such as ovarian tumor cells. These findings demonstrate how DigiPico and MutLX can be used to study the evolution of tumors, during cancer development, progression and recurrence.
References
-
Clusters of multiple mutations: incidence and molecular mechanismsAnnual Review of Genetics 49:243–267.https://doi.org/10.1146/annurev-genet-112414-054714
-
The evolution and ecology of resistance in cancer therapyCold Spring Harbor Perspectives in Medicine 8:a033415.https://doi.org/10.1101/cshperspect.a033415
-
RNA-Seq methods for transcriptome analysisWiley Interdisciplinary Reviews: RNA 8:e1364.https://doi.org/10.1002/wrna.1364
-
Resolving genetic heterogeneity in cancerNature Reviews Genetics 20:404–416.https://doi.org/10.1038/s41576-019-0114-6
Article and author information
Author details
Publication history
Copyright
© 2020, Bley
This article is distributed under the terms of the Creative Commons Attribution License, which permits unrestricted use and redistribution provided that the original author and source are credited.
Metrics
-
- 1,615
- views
-
- 129
- downloads
-
- 2
- citations
Views, downloads and citations are aggregated across all versions of this paper published by eLife.
Download links
Downloads (link to download the article as PDF)
Open citations (links to open the citations from this article in various online reference manager services)
Cite this article (links to download the citations from this article in formats compatible with various reference manager tools)
Further reading
-
- Cancer Biology
- Chromosomes and Gene Expression
MYC family oncoproteins regulate the expression of a large number of genes and broadly stimulate elongation by RNA polymerase II (RNAPII). While the factors that control the chromatin association of MYC proteins are well understood, much less is known about how interacting proteins mediate MYC’s effects on transcription. Here, we show that TFIIIC, an architectural protein complex that controls the three-dimensional chromatin organisation at its target sites, binds directly to the amino-terminal transcriptional regulatory domain of MYCN. Surprisingly, TFIIIC has no discernible role in MYCN-dependent gene expression and transcription elongation. Instead, MYCN and TFIIIC preferentially bind to promoters with paused RNAPII and globally limit the accumulation of non-phosphorylated RNAPII at promoters. Consistent with its ubiquitous role in transcription, MYCN broadly participates in hubs of active promoters. Depletion of TFIIIC further increases MYCN localisation to these hubs. This increase correlates with a failure of the nuclear exosome and BRCA1, both of which are involved in nascent RNA degradation, to localise to active promoters. Our data suggest that MYCN and TFIIIC exert an censoring function in early transcription that limits promoter accumulation of inactive RNAPII and facilitates promoter-proximal degradation of nascent RNA.
-
- Cancer Biology
Hepatocellular carcinoma (HCC), the most common type of liver tumor, is a leading cause of cancer-related deaths, and the incidence of liver cancer is still increasing worldwide. Curative hepatectomy or liver transplantation is only indicated for a small population of patients with early-stage HCC. However, most patients with HCC are not candidates for radical resection due to disease progression, leading to the choice of the conventional tyrosine kinase inhibitor drug sorafenib as first-line treatment. In the past few years, immunotherapy, mainly immune checkpoint inhibitors (ICIs), has revolutionized the clinical strategy for HCC. Combination therapy with ICIs has proven more effective than sorafenib, and clinical trials have been conducted to apply these therapies to patients. Despite significant progress in immunotherapy, the molecular mechanisms behind it remain unclear, and immune resistance is often challenging to overcome. Several studies have pointed out that the complex intercellular communication network in the immune microenvironment of HCC regulates tumor escape and drug resistance to immune response. This underscores the urgent need to analyze the immune microenvironment of HCC. This review describes the immunosuppressive cell populations in the immune microenvironment of HCC, as well as the related clinical trials, aiming to provide insights for the next generation of precision immunotherapy.