Theory for the optimal detection of time-varying signals in cellular sensing systems
Figures
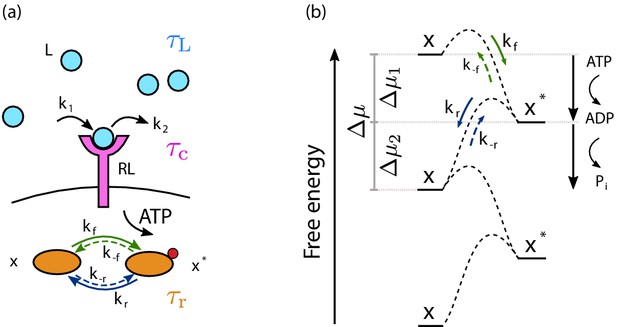
The cell signaling network.
(a) The time-varying ligand concentration is modeled as a memoryless (Markovian) signal with mean , variance , and correlation time . A free ligand molecule L (light blue circle) can bind at rate k1 to a free receptor R (magenta protein) on the cell membrane (black line), forming the complex RL, and unbind at rate k2 from RL. The correlation time of the receptor state is . The complex RL catalyzes the phosphorylation reaction, driven by adenosine triphosphate (ATP) conversion, of a downstream readout from the unphosphorylated (inactive) state x to the phosphorylated (active) state , with rate . The phosphorylated readout then spontaneously decays to the x state with rate . Microscopic reverse reactions of each signaling pathway are represented by dashed arrows. The relaxation time of the push–pull network is . (b) Free-energy landscape of a readout molecule across the activation/deactivation reactions. Fuel turnover, provided by ATP conversion, drives the activation (phosphorylation) reaction characterized by the forward rate and its microscopic reverse rate (green arrows). Associated with this activation reaction is a free-energy drop . The deactivation pathway corresponds to the spontaneous release of the inorganic phosphate; it is characterized by the rate and its microscopic reverse (blue arrows) and corresponds to a free-energy drop .
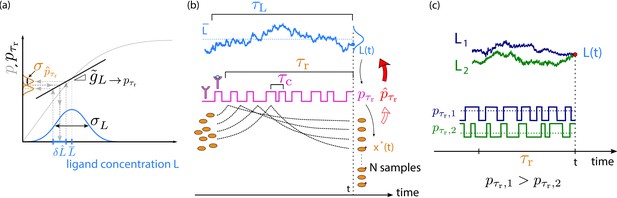
The precision of estimating a time-varying ligand concentration L.
(a) The cell estimates the current ligand concentration by estimating the average receptor occupancy over the past integration time and by inverting the dynamic input–output relation (black solid line). The latter describes the mapping between the current concentration of the time-varying signal and the average receptor occupancy over the past , see also (b); it depends on the timescale of the input signal and hence differs from the conventional static input–output relation , which describes the mapping between the average receptor occupancy and a static ligand concentration that does not vary in time (gray solid line). The squared error in the estimate of the concentration depends on the variance in the estimate of the average receptor occupancy and the dynamic gain , which is the slope of . Only in the limit , does reduce to (the linearized form of) and does the dynamic gain become the static gain , which is the slope of at the average ligand concentration . The input distribution, shown in blue, has width . (b) The average receptor occupancy over the past integration time is estimated via the downstream network, which is modeled as a device that discretely samples the ligand-binding state of the receptor via its readout molecules x (Govern and Ten Wolde, 2014a); the fraction of modified readout molecules provides an estimate of . The sensing error has two contributions (Equation 6): sampling error and dynamical error. The sampling error arises from the error in the estimate of that is due to the stochasticity of the sampling process; it depends on the number of samples, their independence, and their accuracy. (c) The dynamical error arises because the current ligand concentration is estimated via the average receptor occupancy over the past integration time : the latter depends on the ligand concentration in the past , which will, in general, deviate from the current concentration. Two different input trajectories (L1 in blue, L2 in green) ending at time t at the same value (red dot) lead to different estimates of due to their different average receptor occupancy () in the past .
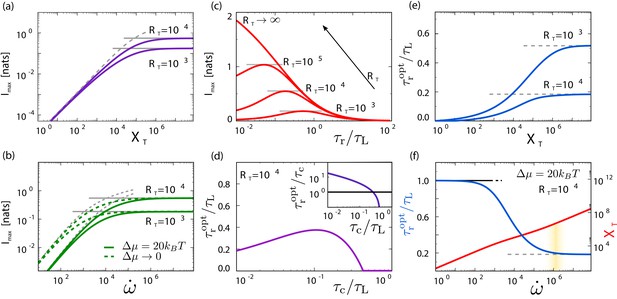
Receptors , readout molecules , and fundamentally limit sensing, and there exists an optimal integration time that depends on which of the resources is limiting.
(a, b) , , and are fundamental resources, with no trade-offs between them. Plotted is the maximum mutual information , obtained by minimizing Equation 6 over p and , for different combinations of (a) and in the irreversible limit and (b) and for two different values of . The sensing precision is bounded by the limiting resource, (solid gray lines, Equation 8 with ), (dashed gray line, Equation 8 with , panel a), or (dashed gray lines, Equation 8 with or , panel b). (c) as a function of for different values of in the Berg–Purcell limit ( and ). There exists an optimal integration time that maximizes the sensing precision; decreases with . (d) In this limit, depends non-monotonically on the receptor–ligand correlation time : it first increases with to sustain time-averaging, but then drops when becomes of order unity and time-averaging is no longer effective (see inset). (e) as a function of for different values of . When , time averaging is not possible and the optimal system is an instantaneous responder, ; when , the system reaches the Berg–Purcell regime in which is limited by rather than (see panel a). (f) and as a function of . When the power is limiting, the sampling error dominates and equals to maximize , minimizing the sampling error; then decreases to trade part of the decrease in the sampling error for a reduction in the dynamical error such that both decrease; when the sampling interval becomes comparable to , in the region marked by the yellow bar, the sampling error is no longer limited by , such that now limits both sources of error; the two sources can therefore no longer be decreased simultaneously by increasing ; the system has entered the Berg–Purcell regime, where is determined by rather than (see panel b). Parameter values unless specified: .
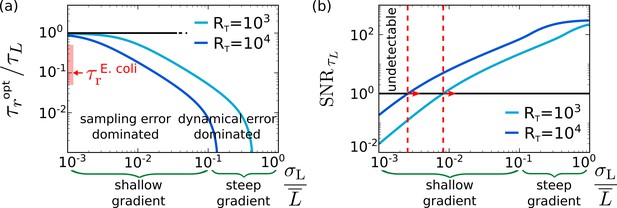
The optimal integration time for the chemotaxis system of E. coli.
(a) The optimal integration time , obtained by numerically optimizing Equation 8 with , as a function of the relative strength of the input noise, , for two different copy numbers of the receptor–CheA complexes; for an exponential gradient with length scale x0, the relative noise strength , where is the run length of E. coli. It is seen that increases as decreases because the relative importance of the sampling error compared to the dynamical error increases. The figure also shows that decreases as is increased because that allows for more instantaneous measurements (see also Figure 3). The red bar indicates the range of the estimated integration time of E. coli, , based on its attractant and repellent response, respectively (Sourjik and Berg, 2002), divided by the input timescale based on its typical run time of about a second (Berg and Brown, 1972; Taute et al., 2015). The panel indicates that E. coli has been optimized to detect shallow concentration gradients. (b) The signal-to-noise ratio , with given by Equation 6, as a function of . To be able to detect the gradient, the must exceed unity. The panel shows that the shallowest gradient that E. coli can detect (marked with dashed red line) has, for , a length scale of (corresponding to ), which is consistent with experiments based on ramp responses (Shimizu et al., 2010). Other parameter: receptor–ligand-binding correlation time (Vaknin and Berg, 2007; Danielson et al., 1994).
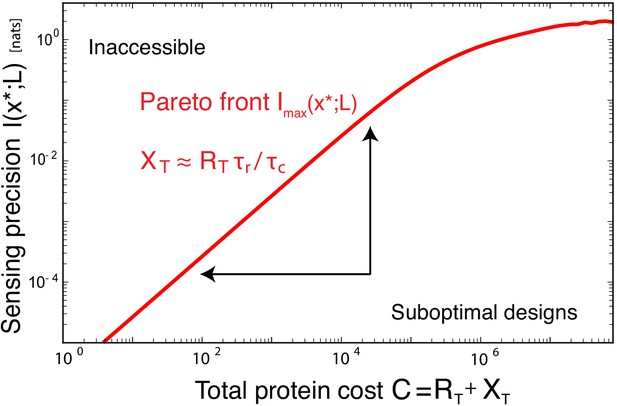
The benefit of a sensing system depends on the sensing precision it can achieve and the cost of making it.
The Pareto front characterizes the trade-off between the maximal sensing precision, quantified by the maximal mutual information , and the cost of making the sensing system, , where is the relative cost of making a readout versus a receptor protein, here taken to be . System designs below the Pareto front are suboptimal and can be improved by reducing the cost, that is, the number of proteins, and / or increasing the sensing precision. The optimal systems at the Pareto front obey, to a good approximation, the allocation principle Equation 12. The Pareto front, formed by the maximal value of as a function of C, is obtained by minimizing Equation 6 over subject to the constraint ; the quality parameter is corresponding to ; ; .