Whole genome comparison of a large collection of mycobacteriophages reveals a continuum of phage genetic diversity
Abstract
The bacteriophage population is large, dynamic, ancient, and genetically diverse. Limited genomic information shows that phage genomes are mosaic, and the genetic architecture of phage populations remains ill-defined. To understand the population structure of phages infecting a single host strain, we isolated, sequenced, and compared 627 phages of Mycobacterium smegmatis. Their genetic diversity is considerable, and there are 28 distinct genomic types (clusters) with related nucleotide sequences. However, amino acid sequence comparisons show pervasive genomic mosaicism, and quantification of inter-cluster and intra-cluster relatedness reveals a continuum of genetic diversity, albeit with uneven representation of different phages. Furthermore, rarefaction analysis shows that the mycobacteriophage population is not closed, and there is a constant influx of genes from other sources. Phage isolation and analysis was performed by a large consortium of academic institutions, illustrating the substantial benefits of a disseminated, structured program involving large numbers of freshman undergraduates in scientific discovery.
https://doi.org/10.7554/eLife.06416.001eLife digest
Viruses are unable to replicate independently. To generate copies of itself, a virus must instead invade a target cell and commandeer that cell's replication machinery. Different viruses are able to invade different types of cell, and a group of viruses known as bacteriophages (or phages for short) replicate within bacteria. The enormous number and diversity of phages in the world means that they play an important role in virtually every ecosystem.
Despite their importance, relatively little is known about how different phage populations are related to each other and how they evolved. Many phages contain their genetic information in the form of strands of DNA. Using genetic sequencing to find out where and how different genes are encoded in the DNA can reveal information about how different viruses are related to each other. These relationships are particularly complicated in phages, as they can exchange genes with other viruses and microbes.
Previous studies comparing the genomes—the complete DNA sequence—of reasonably small numbers of phages that infect the Mycobacterium group of bacteria have found that the phages can be sorted into ‘clusters’ based on similarities in their genes and where these are encoded in their DNA. However, the number of phages investigated so far has been too small to conclude how different clusters are related. Are the clusters separate, or do they form a ‘continuum’ with different genes and DNA sequences shared between different clusters?
Here, Pope, Bowman, Russell et al. compare the individual genomes of 627 bacteriophages that infect the bacterial species Mycobacterium smegmatis. This is by far the largest number of phage genomes analyzed from a single host species. The large number of genomes analyzed allowed a much clearer understanding of the complexity and diversity of these phages to be obtained. The isolation, sequencing and analysis of the hundreds of M. smegmatis bacteriophage genomes was performed by an integrated research and education program, called the Science Education Alliance Phage Hunters Advancing Genomics and Evolutionary Science (SEA-PHAGES) program. This enabled thousands of undergraduate students from different institutions to contribute to the phage discovery and sequencing project, and co-author the report. SEA-PHAGES therefore shows that it is possible to successfully incorporate genuine scientific research into an undergraduate course, and that doing so can benefit both the students and researchers involved.
The results show that while the genomes could be categorized into 28 clusters, the genomes are not completely unrelated. Instead, a spread of diversity is seen, as genes and groups of genes are shared between different clusters. Pope, Bowman, Russell et al. further reveal that the phage population is in a constant state of change, and continuously acquires genes from other microorganisms and viruses.
https://doi.org/10.7554/eLife.06416.002Introduction
Bacteriophages are the dark matter of the biological universe, forming a vast, ancient, dynamic, and genetically diverse population, replete with genes of unknown function (Pedulla et al., 2003). Phages are the most abundant organisms in the biosphere, and the ∼1031 tailed phage particles participate in ∼1023 infections per second on a global scale, with the entire population turning over every few days (Suttle, 2007). The population is not only vast and dynamic, but comparisons of virion structures suggest that it is also extremely old (Krupovic and Bamford, 2010). It is thus not surprising that bacteriophages are genetically highly diverse, although their comparative genomics has lagged behind that of other microbes, largely due to the lack of individual isolates for genomic analyses (Hatfull and Hendrix, 2011). To date, there are approximately 2000 completely sequenced bacteriophage genomes in the GenBank database, a small number relative to the more than 30,000 sequenced prokaryotic genomes (http://www.ncbi.nlm.nih.gov/genome/browse/), in spite of phage genomes being only 1–5% of the size of their host genomes.
Double-stranded DNA tailed phages are proposed to have evolved with common ancestry but with different phages having differential access to a large common gene pool (Hendrix et al., 1999). Phage genomes are typified by their mosaic architectures generated by gene loss and gain through horizontal genetic exchange; however, the parameters influencing access to the common gene pool are numerous and likely include host range, genome size, replication mode, and life style (temperate vs lytic). Migration to new hosts is probably common, but is affected by local host diversity and mutation rates, as well as resistance mechanisms such as receptor availability, restriction, CRISPRs, and abortive infection systems (Buckling and Brockhurst, 2012; Jacobs-Sera et al., 2012; Hoskisson et al., 2015). Constraints on gene acquisition may also be imposed by synteny—particularly among virion structural genes—and by size limits of DNA packaging (Juhala et al., 2000; Hatfull and Hendrix, 2011).
We have previously described comparative analyses of modest numbers of mycobacteriophages and shown that they can be sorted by nucleotide sequence and gene content comparisons into groups of closely related genomes referred to as ‘clusters’ (designated Cluster A, B, C, etc.); phages without any close relatives are referred to as ‘singletons’. Some of the clusters can be further divided into subclusters (e.g., Subcluster A1, A2, A3, etc.) according to nucleotide sequence relatedness (Pedulla et al., 2003; Hatfull et al., 2006, 2010; Pope et al., 2011b). The genomes are mosaic whereby individual phages are constructed as assemblages of modules, many of which are single genes (Pedulla et al., 2003). Each mycobacteriophage cluster has features particular to that cluster (e.g., regulatory systems, repeated sequences, tRNA genes, etc. [Pope et al., 2011a, 2011b, 2013, 2014a, 2014b]), but because of the pervasive mosaicism, the relationships among phages within clusters and between clusters are complex. Collections of phages have been isolated on other hosts such as Bacillus spp., Escherichia coli, Pseudomonas spp., Propionibacterium spp. and Staphylococcus spp. (Kwan et al., 2005, 2006; Kropinski et al., 2007; Marinelli et al., 2012; Hatfull et al., 2013; Grose and Casjens, 2014; Grose et al., 2014; Lee et al., 2014) and these can be similarly divided into clusters based on DNA similarity. Recent analysis of 337 phages infecting 31 bacterial species within the Enterobacteriaceae (Grose and Casjens, 2014) reveals 56 clusters of phage genomes. It is thus clear that there is substantial diversity within the phage population, even when comparing phages of a common host and which are expected to be in direct genetic contact with each other in their natural environment (Hatfull and Hendrix, 2011). Nonetheless, the numbers of genomes isolated on a particular host generally are too small to define the nature and the size of the populations at large with any substantial resolution.
Viral metagenomic studies provide valuable insights into phage diversity and population dynamics, but typically generate few complete genome sequences or any specific information relating viral genomes to specific bacterial hosts (Hambly and Suttle, 2005; Rodriguez-Brito et al., 2010; Mokili et al., 2012). A recent analysis of Synechococcus phages using metagenomic analysis coupled with viral tagging showed that there are multiple ‘populations’ of these phages (similar to the clusters described above), but suggested that these represent distinct groups of related phages rather than a continuous spectrum of diversity (Deng et al., 2014). This differs from prior predictions that the phage population as a whole likely spans a continuum of diversity—albeit with uneven representation of different groups of related phages—because of genomic mosaicism (Hendrix, 2003; Hatfull, 2010, 2012). However, as the Synechococcus phage data are derived from a single sample using a single host, it is unclear if this extends to phages of other hosts (Deng et al., 2014).
Here we describe the comparative analysis of a large number of completely sequenced mycobacteriophage genomes and demonstrate that they represent a spectrum of diversity and do not constitute discrete populations. Rarefaction analyses of their constituent genes are consistent with populations of gene families shared among mycobacteriophages being augmented by the introduction of new gene families from outside sources. The assembling of a large and highly informative collection of bacteriophages by a consortium of students and faculty at multiple institutions demonstrates that a course-based research experience (CRE) can be successfully implemented at large scale without compromising the authenticity or richness of a scientific investigation imbued with discovery and project ownership.
Results and discussion
A genome-by-genome approach to defining phage diversity
Exploring phage diversity using a genome-by-genome approach has notable advantages and some potential disadvantages. The main advantage is that complete genome sequences give information about genome length and composition, providing key insights into genome mosaicism and how genome segments are shared and exchanged. A difficulty is that there are not large extant phage collections available for most bacterial hosts, and isolation, purification, and characterization of phages can be slow and time-consuming. Because isolation typically requires plaque formation and growth in the laboratory, some naturally occurring phages may escape isolation using standard methods. Thus, although the diversity of phages isolated and propagated in the laboratory may not capture all types of phage, it represents a minimum, not a maximum, index of diversity.
Authentic research in a CRE
The 2012 report from the President's Council of Advisors on Science and Technology (PCAST) focused on the poor retention of undergraduate students in science, technology, engineering and mathematics (STEM) as an impediment to meeting US economic demands (PCAST, 2012). One of the PCAST recommendations is to replace traditional introductory laboratory courses with research-based experiences that would inspire freshman students and promote STEM retention. A powerful strategy is to engage students in scientific discovery through CREs. The successful implementation of this strategy depends on (i) identifying research questions that can engage students in contributing genuine advances in scientific knowledge without requiring prior expert knowledge, and (ii) designing the project so that large numbers of students can participate in a meaningful fashion.
We have previously described the Howard Hughes Medical Institute (HHMI) Science Education Alliance Phage Hunters Advancing Genomics and Evolutionary Science (SEA-PHAGES) program, in which beginning undergraduate students isolate, purify, sequence, annotate, and compare bacteriophages, and have described its educational advantages (Jordan et al., 2014). By taking advantage of the massive diversity of the phage population so that each student can isolate a unique phage, the program encourages student ownership of their science. And because the collective discoveries by many students generate new scientific insights, the program creates a scientific community of students engaged in authentic research.
The SEA-PHAGES program has contributed to the growth of the collection of sequenced mycobacteriophages to nearly 700 individual isolates (http://phagesdb.org), of which 627 were selected for a detailed analysis (Supplementary file 1). This is by far the largest collection of sequenced phage genomes for any single host and thus promises to substantially advance our understanding of phage diversity. The phages were isolated using either direct plating or by enrichment using Mycobacterium smegmatis mc2155 as a host, and sequenced using next-generation approaches (see ‘Materials and methods’). More than 5000 students—primarily freshmen—at 74 institutions have been involved since inception of the SEA-PHAGES program in 2008, and the phages isolated represent a broad geographical distribution (Figure 1) and a variety of viral morphotypes (http://phagesdb.org). The new insights gained from comparative genomic analyses of these phages—as described below—demonstrate the effectiveness of viral discovery and genomics as a model for CRE development.
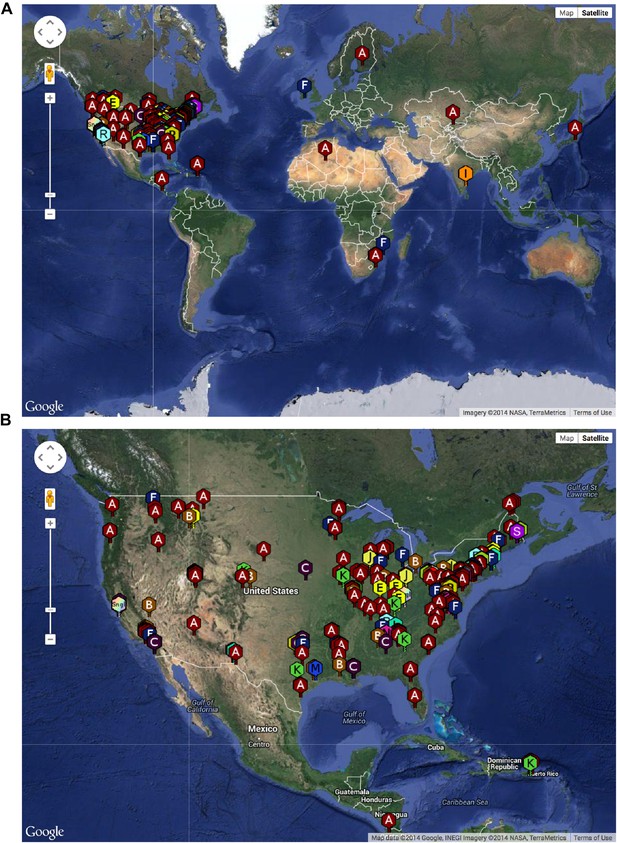
Geographical distribution of sequenced mycobacteriophages.
(A) Locations of sequenced mycobacteriophages across the globe. (B) Locations of sequenced mycobacteriophages across the United States. Colors and letter designations on the isolates refer to the cluster to which the genomes belong. Data from www.phagesdb.org.
Assembling mycobacteriophages into clusters and subclusters
Using previously reported parameters based primarily on nucleotide sequence similarity spanning >50% genome length (Hatfull et al., 2006), the 627 genomes were assembled into 20 clusters (A–T) and eight singletons (with no close relatives) (Figure 2, Supplementary file 1); 11 clusters were subdivided into 2 to 11 subclusters (Table 1). There is considerable variation in cluster size with substantial differences in the numbers of genomes in each cluster (2–232), but there is relatively little variation in either genome length or the numbers of genes per genome in any given cluster (Table 1). Cluster assignment is of practical utility and is generally robust, with clustered phages typically sharing genome architectures, as noted for the Enterobacteriacea (Grose and Casjens, 2014). For example, Cluster A phages are similar in size and transcriptional organization, and share an unusual immunity system (Brown et al., 1997; Pope et al., 2011b). Cluster M phages all contain large numbers of tRNA genes (Pope et al., 2014a), Cluster K (Pope et al., 2011a) and Cluster O (Cresawn et al., 2015) phages have different but characteristic repeated sequences, and Cluster J phages have an unusual capsid with a triangulation (T) number of 13 (Pope et al., 2013). Therefore, the organization of related mycobacteriophages into clusters provides a framework for identifying and interpreting gene trafficking within and among potentially distinct groups of genomes.
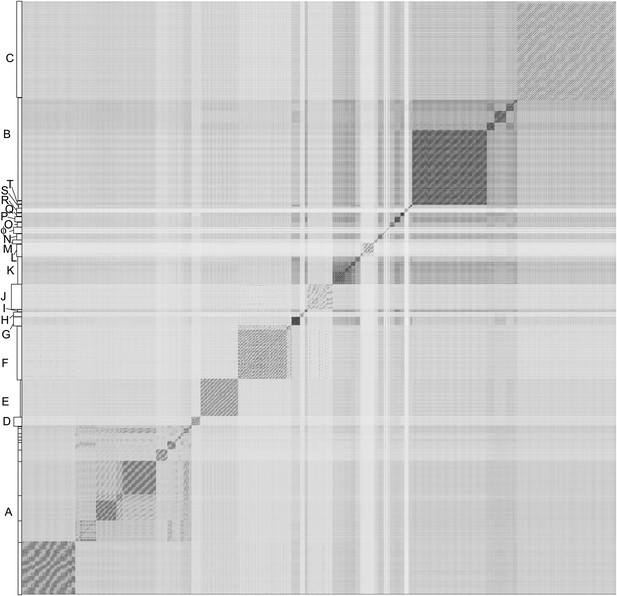
Nucleotide sequence comparison of 627 mycobacteriophages displayed as a dotplot.
Complete genome sequences of 627 mycobacteriophages were concatenated into a single file which was compared with itself using Gepard (Krumsiek et al., 2007) and displayed as a dotplot using default parameters (word length, 10). The order of the genomes is as listed in Supplementary file 1. Nucleotide similarity is a primary component in assembling phages into clusters, which typically requires evident DNA similarity spanning more than 50% of the genome lengths.
-
Figure 2—source data 1
Concatenated DNA sequences for 627 phage genomes.
- https://doi.org/10.7554/eLife.06416.005
Diversity and genetic isolation of mycobacteriophage genome clusters
Cluster | # Subclusters | # Genomes | Average # genes* | Average length (bp) | Total phams† | Total genes | CLASP‡ | CAP§ | CCI# | CII¶ |
---|---|---|---|---|---|---|---|---|---|---|
A | 11 | 232 | 90 ± 5.3 | 51,514 | 1085 | 20,880 | 38.3 | 12.4 | 0.08 | 80.2 |
B | 5 | 109 | 100.4 ± 4.5 | 68,653 | 421 | 10,944 | 66.2 | 23.2 | 0.24 | 81.0 |
C | 2 | 45 | 231 ± 5.9 | 155,504 | 486 | 10,395 | 89.3 | 29.4 | 0.48 | 84.6 |
D | 2 | 10 | 89.3 ± 6.4 | 64,965 | 147 | 893 | 88.1 | 64.3 | 0.61 | 71.4 |
E | 1 | 35 | 141.9 ± 3.4 | 75,526 | 236 | 4967 | 87.2 | 63.8 | 0.60 | 59.3 |
F | 3 | 66 | 105.3 ± 5.3 | 57,416 | 658 | 6950 | 54.4 | 4.9 | 0.16 | 55.8 |
G | 1 | 14 | 61.5 ± 1.2 | 41,845 | 72 | 861 | 96.0 | 91.1 | 0.85 | 55.6 |
H | 2 | 5 | 98.4 ± 5.7 | 69,469 | 207 | 492 | 61.6 | 31.5 | 0.48 | 67.6 |
I | 2 | 4 | 78 ± 3.7 | 49,954 | 147 | 312 | 58.9 | 35.0 | 0.53 | 23.8 |
J | 1 | 16 | 239.8 ± 9.3 | 110,332 | 530 | 3776 | 70.8 | 40.1 | 0.45 | 58.5 |
K | 5 | 32 | 95.7 ± 4.6 | 59,720 | 411 | 3069 | 51.8 | 20.0 | 0.23 | 73.5 |
L | 3 | 13 | 127.9 ± 6.5 | 75,177 | 246 | 1663 | 78.2 | 50.8 | 0.52 | 72.4 |
M | 2 | 3 | 141 ± 8.8 | 81,636 | 201 | 423 | 73.5 | 63.0 | 0.70 | 69.2 |
N | 1 | 7 | 69.1 ± 2.2 | 42,888 | 152 | 484 | 64.1 | 45.6 | 0.45 | 40.8 |
O | 1 | 5 | 124.2 ± 3.1 | 70,651 | 151 | 621 | 90.6 | 83.3 | 0.82 | 64.2 |
P | 2 | 9 | 78.8 ± 2.1 | 47,668 | 159 | 709 | 76.1 | 42.3 | 0.50 | 34.0 |
Q | 1 | 5 | 85.2 ± 3.7 | 53,755 | 90 | 426 | 96.6 | 90.4 | 0.95 | 73.3 |
R | 1 | 4 | 101.5 ± 2.5 | 71,348 | 117 | 406 | 91.4 | 84.8 | 0.87 | 71.8 |
S | 1 | 2 | 109 ± 2.0 | 65,172 | 117 | 218 | 91.7 | 91.7 | 0.93 | 70.9 |
T | 1 | 3 | 66.7 ± 2.4 | 42,833 | 83 | 200 | 86.1 | 82.5 | 0.80 | 62.7 |
Dori | 1 | 1 | 94 | 64,613 | 94 | 94 | N/A | N/A | N/A | 35.8 |
DS6A | 1 | 1 | 97 | 60,588 | 96 | 97 | N/A | N/A | N/A | 58.3 |
Gaia | 1 | 1 | 194 | 90,460 | 193 | 194 | N/A | N/A | N/A | 58.0 |
MooMoo | 1 | 1 | 98 | 55,178 | 98 | 98 | N/A | N/A | N/A | 31.6 |
Muddy | 1 | 1 | 71 | 48,228 | 70 | 71 | N/A | N/A | N/A | 71.4 |
Patience | 1 | 1 | 109 | 70,506 | 109 | 109 | N/A | N/A | N/A | 57.8 |
Sparky | 1 | 1 | 93 | 63,334 | 93 | 93 | N/A | N/A | N/A | 48.4 |
Wildcat | 1 | 1 | 148 | 78,296 | 148 | 148 | N/A | N/A | N/A | 69.6 |
-
*
Average number of protein-coding genes per genome, with standard deviation.
-
†
Total phams is the sum of all phamilies (groups of homologous mycobacteriophage genes) in that cluster.
-
‡
The Cluster Averaged Shared Phamilies (CLASP) index is the average of the percentages of phamilies shared pairwise between genomes within a cluster.
-
§
The Cluster-Associated Phamilies (CAP) index is the percentage of the average number of phamilies per genome within a cluster whose phamilies are present in every cluster member.
-
#
The Cluster Cohesion Index (CCI) is generated by dividing the average number of genes per genome by the total number of phamilies (phams) in that cluster.
-
¶
The Cluster Isolation Index (CII) is the percentage of phams that are present only in that cluster, and not present in other mycobacteriophages.
-
N/A: Not applicable.
Gene content relationships among sequenced mycobacteriophages
Genome mosaicism is more apparent from comparison of gene product amino acid sequences than nucleotide sequence comparisons because of the accumulation of genome rearrangements over a longer period of evolution, during which indications of DNA similarity are lost. To compare mycobacteriophage gene contents we grouped related genes into protein families (‘phamilies’ or ‘phams’) using Phamerator (Cresawn et al., 2011), which we modified to use kClust (Hauser et al., 2013) so as to easily accommodate the large numbers of comparisons. The 69,633 genes assembled into 5205 phams of which 1613 (31%) are orphams (single-gene phamilies [Hatfull et al., 2010]). Approximately 25% of phams can be assigned functions in viral structure and assembly, DNA metabolism, integration, lysis, and regulation, but the vast majority are of unknown function. Representation of gene content relationships among all 627 phages as a network phylogeny reveals relationships that are in accord with the cluster and subcluster designations derived from nucleotide sequence comparisons (Figure 3). The multiple branches between clusters/subclusters reflect the phylogenetic complexities that arise from genome mosaicism, where genes within a genome have distinct evolutionary histories.
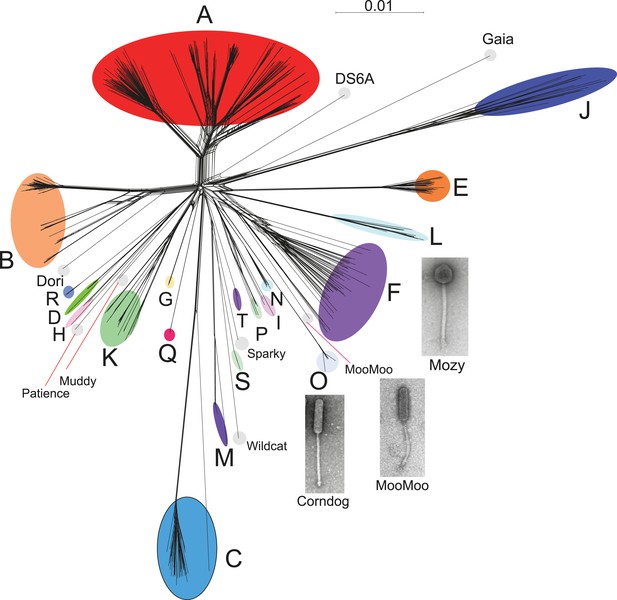
Network phylogeny of 627 mycobacteriophages based on gene content.
Genomes of 627 mycobacteriophages were compared according to shared gene content using the Phamerator (Cresawn et al., 2011) database Mykobacteriophage_627, and displayed using SplitsTree (Huson and Bryant, 2006). Colored circles indicate grouping of phages labeled according to their cluster designations generated by nucleotide sequence comparison (Figure 2); singleton genomes with no close relatives are labeled but not circled. Micrographs show morphotypes of the singleton MooMoo, the Cluster F phage Mozy, and the Cluster O phage Corndog. With the exception of DS6A, all of the phages infect Mycobacterium smegmatis mc2155.
-
Figure 3—source data 1
Nexus file containing phamily assignments for 627 phage genomes.
- https://doi.org/10.7554/eLife.06416.011
The distribution of orphams (genes without mycobacteriophage homologues) provides additional support for cluster/subcluster assignments; Figure 4). A relatively high proportion of orphams is a characteristic of both singleton genomes and single-genome subclusters (Figure 4). At least 30% of genes in all of the singleton genomes are orphams, and the single-genome subclusters have a minimum of 15% orphams; genomes in other clusters and subclusters typically have fewer than 10% orphams (Figure 4). The presence of numerous orphams ensures that the lack of cluster inclusion did not result from sequence errors or insufficient or inappropriate gene annotation. Notable exceptions are Predator (Subcluster H1) and Mendokysei (Cluster T), both of which are in very small clusters/subclusters, and KayaCho (Subcluster B4). KayaCho may warrant separation into a new subcluster (e.g., B6), but overall the orpham distribution is consistent with the cluster/subcluster designations.
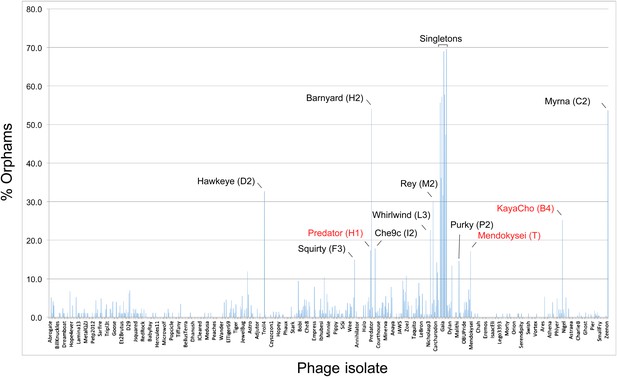
Proportions of orphams in mycobacteriophage genomes.
The proportions of genes that are orphams (i.e., single-gene phamilies with no homologues within the mycobacteriophage dataset) are shown for each phage. The order of the phages is as shown in Supplementary file 1. All of the singleton genomes have >30% orphams, and most of the other genomes with relatively high proportions of orphams are the single-genome subclusters (Table 2) including Hawkeye (D2), Myrna (C2), Squirty (F3), Barnyard (H2), Che9c (I2), Whirlwind (L3), Rey (M2), and Purky (P2). Three phages shown in red type are not singletons or single-genome subclusters but have relatively high proportions of orphams. Predator and Mendokysei are members of the diverse and small clusters (five or fewer genomes) H and T, respectively; KayaCho is a member of Subcluster B4 but has a sufficiently high proportion of orphams to arguably warrant formation of a new subcluster, B6.
-
Figure 4—source data 1
Pham table containing phamily designations for 627 phage genomes.
- https://doi.org/10.7554/eLife.06416.013
The diversity of different clusters is highly varied
To determine the extent to which the various clusters/subclusters represent discrete groups, we generated a heat map showing pairwise shared gene content (Figure 5) and quantified the cluster/subcluster diversity (Table 1, Figure 6). The heat map strikingly illustrates that diversity is non-uniform, with genomes in some clusters (e.g., Subclusters B1, C1) being very closely related, whereas in others they display substantial differences (e.g., Subclusters A1, F1). The variation is also evident within the large Cluster A group, with some subclusters having low diversity (e.g., A4, A5, A6), some being highly diverse (e.g., A1, A2), and some plausibly further splitting into subgroups (A3) (Figure 5).
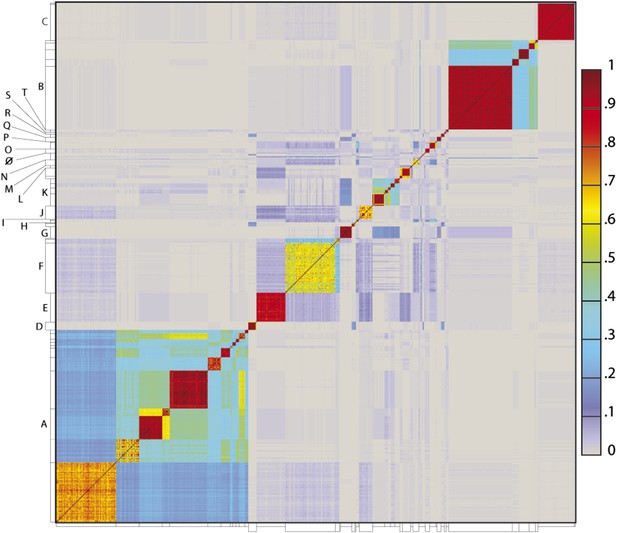
Heat map representation of shared gene content among 627 mycobacteriophages.
The percentages of pairwise shared genes was determined using a Phamerator (Cresawn et al., 2011) database (Mykobacteriophage_627) populated with 627 completely sequenced phage genomes. The 69,574 genes were assembled into 5205 phamilies (phams) of related sequences using kClust, and the average proportions of shared phams calculated. Genomes are ordered on both axes according to their cluster and subcluster designations (Supplementary file 1) determined by nucleotide sequence similarities (Figure 2). The values (proportions of pairwise shared phams averaged between each partner) are colored as indicated.
-
Figure 5—source data 1
Dataset showing percentages of pairwise shared phamilies.
- https://doi.org/10.7554/eLife.06416.017
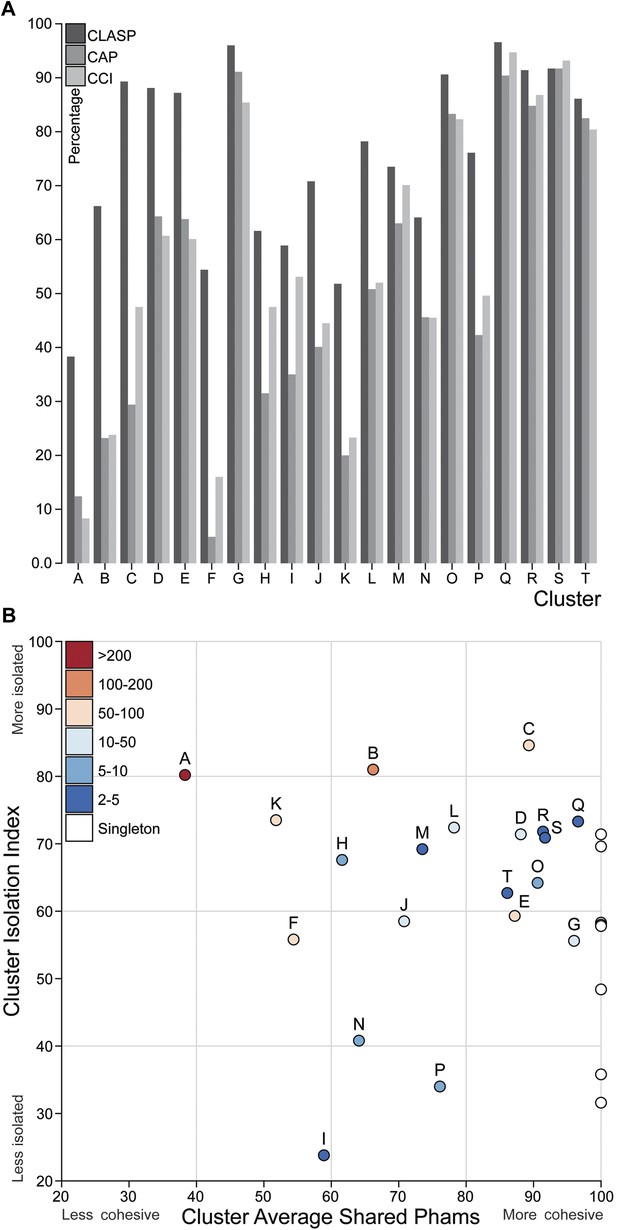
Cluster diversity and isolation.
(A) The CLuster Averaged Shared Phamilies (CLASP; blue), Cluster Associated Phamilies (CAP; red) and Cluster Cohesion Index (CCI; green) values are plotted for each mycobacteriophage cluster. (B) The Cluster Isolation Index (CII) and CLASP values (both shown as percentages) are plotted for each phage cluster. Singletons (white circles) are not individually labeled but correspond to the values shown in Table 1.
-
Figure 6—source data 1
Datasets showing numbers of CLuster Average Shard Phamilies.
- https://doi.org/10.7554/eLife.06416.019
We quantified the cluster diversity using three different measures, CLuster Average Shared Phamilies (CLASP), Cluster Associated Phamilies (CAP), and Cluster Cohesion Index (CCI) (Tables 1, 2, Figure 6A). Both CAP (the number of phams present in all genomes within a cluster divided by the average number of genes per genome) and CCI (the average number of genes per genome as a percentage of the total number of phams in that cluster) show substantial variation between clusters (Table 1, S2), and little evidence for commonly conserved ‘core genes’, as suggested for T4-related phages (Petrov et al., 2010). However, both of these parameters are somewhat influenced by cluster/subcluster size, which varies from cluster to cluster. In contrast, CLASP (the percentage of phamilies shared between two genomes, then averaged across all possible pairs within a cluster or subcluster) is relatively insensitive to cluster/subcluster size (as seen by a resampling analysis; Figure 6—figure supplement 1), but still shows substantial variation from one cluster to another (Table 1, Figure 6A).
Genometrics and Cluster Cohesion Indexes of mycobacteriophages
Cluster | Subcluster | # Genomes | Average # genes | Average length (bp) | # Phams | CLASP* | CAP† | CCI‡ |
---|---|---|---|---|---|---|---|---|
A | 232 | 90.0 | 51,514 | 1085 | 38.3 | 12.4 | 8.0 | |
A1 | 72 | 91.2 | 51,954 | 416 | 72.3 | 36.9 | 22.0 | |
A2 | 28 | 93.4 | 52,805 | 312 | 64.7 | 30.1 | 30.0 | |
A3 | 37 | 87.7 | 50,325 | 163 | 81.1 | 48.8 | 54.0 | |
A4 | 46 | 87.4 | 51,376 | 125 | 92.7 | 70.6 | 70.0 | |
A5 | 16 | 86.0 | 50,531 | 152 | 81.4 | 58.7 | 57.0 | |
A6 | 11 | 97.8 | 51,677 | 128 | 90.2 | 75.1 | 76.0 | |
A7 | 3 | 84.3 | 52,941 | 115 | 74.9 | 64.4 | 73.0 | |
A8 | 4 | 97.8 | 51,597 | 107 | 93.5 | 86.8 | 91.0 | |
A9 | 4 | 96.0 | 52,838 | 106 | 92.7 | 83.4 | 91.0 | |
A10 | 7 | 80.0 | 49,174 | 112 | 81.6 | 60.9 | 71.0 | |
A11 | 4 | 98.5 | 52,260 | 113 | 93.6 | 88.3 | 87.0 | |
B | 108 | 100.4 | 68,653 | 421 | 66.2 | 23.2 | 24.0 | |
B1 | 77 | 101.8 | 68,532 | 144 | 93.2 | 72.9 | 71.0 | |
B2 | 8 | 89.9 | 67,267 | 101 | 94.9 | 84.6 | 89.0 | |
B3 | 12 | 102.8 | 68,698 | 121 | 96.3 | 84.7 | 85.0 | |
B4 | 8 | 96.1 | 70,619 | 166 | 79.9 | 45.8 | 58.0 | |
B5 | 3 | 96.3 | 70,033 | 108 | 91.7 | 87.2 | 89.0 | |
C | 45 | 231.0 | 155,504 | 486 | 89.3 | 29.4 | 48.0 | |
C1 | 44 | 231.0 | 155,297 | 345 | 91.9 | 73.2 | 67.0 | |
C2 | 1 | 229.0 | 164,602 | 227 | N/A | N/A | N/A | |
D | 10 | 89.3 | 64,965 | 147 | 88.1 | 64.3 | 61.0 | |
D1 | 9 | 87.3 | 64,697 | 100 | 94.9 | 88.8 | 87.0 | |
D2 | 1 | 107.0 | 67,383 | 107 | N/A | N/A | N/A | |
E | 35 | 141.9 | 75,526 | 235 | 87.2 | 63.8 | 60.0 | |
F | 66 | 105.3 | 57,416 | 658 | 54.4 | 4.9 | 16.0 | |
F1 | 60 | 104.8 | 57,486 | 573 | 59.6 | 20.6 | 18.0 | |
F2 | 5 | 110.8 | 55,996 | 207 | 65.7 | 49.0 | 54.0 | |
F3 | 1 | 107.0 | 60,285 | 105 | N/A | N/A | N/A | |
G | 14 | 61.5 | 41,845 | 72 | 96.0 | 91.1 | 85.0 | |
H | 5 | 98.4 | 69,469 | 207 | 61.6 | 31.5 | 48.0 | |
H1 | 4 | 95.8 | 69,137 | 131 | 81.9 | 67.9 | 73.0 | |
H2 | 1 | 109.0 | 70,797 | 110 | N/A | N/A | N/A | |
I | 4 | 78.0 | 49,954 | 147 | 58.9 | 35.0 | 53.0 | |
I1 | 3 | 76.0 | 47,588 | 101 | 77.5 | 66.7 | 75.0 | |
I2 | 1 | 84.0 | 57,050 | 84 | N/A | N/A | N/A | |
J | 16 | 239.8 | 110,332 | 530 | 70.8 | 40.1 | 45.0 | |
K | 33 | 95.7 | 59,720 | 411 | 51.8 | 20.0 | 23.0 | |
K1 | 15 | 94.3 | 59,877 | 166 | 85.5 | 47.9 | 57.0 | |
K2 | 4 | 96.3 | 56,597 | 128 | 85.2 | 77.7 | 75.0 | |
K3 | 3 | 98.2 | 61,322 | 111 | 92.2 | 89.5 | 88.0 | |
K4 | 5 | 94.0 | 57,865 | 106 | 93.7 | 87.2 | 89.0 | |
K5 | 6 | 98.2 | 62,154 | 144 | 82.1 | 68.2 | 68.0 | |
L | 13 | 127.9 | 75,177 | 246 | 78.2 | 50.8 | 52.0 | |
L1 | 3 | 123.7 | 74,050 | 135 | 92.6 | 88.8 | 92.0 | |
L2 | 9 | 129.3 | 75,456 | 170 | 90.1 | 72.2 | 76.0 | |
L3 | 1 | 128.0 | 76,050 | 126 | N/A | N/A | N/A | |
M | 3 | 141.0 | 81,636 | 201 | 73.5 | 63.0 | 70.0 | |
M1 | 2 | 135.0 | 80,593 | 138 | 96.6 | 96.6 | 98.0 | |
M2 | 1 | 153.0 | 83,724 | 152 | N/A | N/A | N/A | |
N | 7 | 69.1 | 42,888 | 152 | 64.1 | 45.6 | 45.0 | |
O | 5 | 124.2 | 70,651 | 151 | 90.6 | 83.3 | 82.0 | |
P | 9 | 78.8 | 47,668 | 159 | 76.1 | 42.3 | 50.0 | |
P1 | 8 | 78.4 | 47,313 | 126 | 82.9 | 52.9 | 62.0 | |
P2 | 1 | 82.0 | 50,513 | 82 | N/A | N/A | N/A | |
Q | 5 | 85.2 | 53,755 | 90 | 96.6 | 90.4 | 95.0 | |
R | 4 | 101.5 | 71,348 | 117 | 91.4 | 84.8 | 87.0 | |
S | 2 | 109.0 | 65,172 | 117 | 91.7 | 91.7 | 93.0 | |
T | 3 | 66.7 | 42,833 | 83 | 86.1 | 82.5 | 80.0 |
-
*
Cluster Averaged Shared Phamilies.
-
†
Cluster Associated Phamilies.
-
‡
Cluster Cohesion Index.
The discreteness of different clusters is highly varied
The heat map of genome comparisons (Figure 5) also illustrates the degrees to which clusters and subclusters share gene content, a reflection of cluster discreteness, or how isolated discrete clusters are from each other. For example, although the Cluster A phages are highly diverse, they also appear relatively isolated and share relatively few genes with other clusters (Figure 5). In contrast, phages in Cluster E share substantial numbers of genes with other clusters, including those in Clusters F, J, L, P, and several singletons. We have quantified these relationships with the Cluster Isolation Index (CII, the percentage of phams present within a cluster that are not present in other mycobacteriophage genomes), which demonstrates the considerable variation in isolation from phages of other clusters/subclusters (Table 1, Figure 6B). For example, at one extreme, 84.6% of Cluster C gene phamilies are found only in Cluster C and not elsewhere. At the other extreme, only 23.8% of Cluster I gene phamilies are constrained to that cluster, with the remainder having relatives present in genomes in other clusters. Other clusters form a spectrum of relationships between these extremes (Table 1, Figure 6B), and clusters such as I and P—which share recognizable DNA sequence similarity (Figure 2—figure supplement 1)—share >60% of their genes with other phages (low CII values; Table 1). Thus, although some clusters could be considered as discrete groups—as reported for the Synechococcus phages (Deng et al., 2014)—this is far from being a universal or characteristic feature of groups of related phages.
Cluster isolation analyses reveal additional complexities arising from highly mosaic genomes. For example, the singleton Dori is clearly related to Cluster B phages (Figure 3) with which it shares limited DNA similarity (Figure 2—figure supplement 2) with 20–26% of its genes (Figure 4—figure supplement 1), but also has nucleotide similarity and shares genes with Cluster N and I2 phages, among others (Figure 2—figure supplement 2, Figure 4—figure supplement 1), as reflected in its low CII (Table 1, Figure 6B). Likewise, the singleton MooMoo has segments of DNA similarity and shares ∼20% of its gene content (as determined by shared phams) with Cluster F phages (Figure 3, Figure 2—figure supplement 3, Figure 4—figure supplement 1), but also has similarity to Clusters N and I, as well as a low CII (Table 1, Figure 6B). It has low DNA similarity to Cluster O (Figure 2—figure supplement 3), but has several phams in common with the Cluster O phages, and has the same unusual prolate morphology (Figure 3). Complex relationships are also seen in the singletons Gaia and Sparky (Figure 4—figure supplement 2).
Taken together, the analyses of both cluster diversity and cluster isolation show that mycobacteriophage populations contain a continuum of diversity, with non-uniform abundance of different types of phages. The prevalence of isolated phages may not necessarily reflect the proportions of different types of phages in the environment, but the availability of a large collection of isolated phages enables capture and whole genome analysis of relatively rare phages that are critical to understanding the complexities of genome relationships. We recently reported genomic analysis of the singleton mycobacteriophage Patience, which has a substantially lower GC% than its host (50.3% vs 67.4%), has a different codon usage profile, but is undergoing codon selection for growth in a high GC% environment (Pope et al., 2014b). If there is a flux of phage genomes and genes entering the mycobacterial neighborhood, then we predict that the phages of a single host do not reflect a closed system with discrete populations, but one that is open with ever-expanding diversity.
The mycobacteriophage population is not a closed system
Both the huge diversity of phamilies in mycobacteriophages and the high frequency of orphams suggest that genes are constantly added to phage genomes from outside sources just as genes are added to the genomes of their bacterial hosts via horizontal gene transfer. Such gene influx—for example, from host-jumping phages such as Patience (Pope et al., 2014b)—would provide genetic novelty and enable phages to adapt to their ever-changing hosts. To examine gene flux into the mycobacteriophage population, we performed a rarefaction analysis by re-sampling the gene phamilies within the phage population (Figure 7). Remarkably, the rarefaction curves of the entire collection—including the 95% confidence limits—do not fit a hyperbola as would be expected if the mycobacteriophages were limited to an isolated set of genes, and about 2.5 new gene phamilies are predicted to be identified with each newly isolated phage (Figure 7A). Similar independent analyses on the phages of Cluster A or the phages of Cluster B show that this is also observed within these clusters (Figure 7B,C). Thus both individual clusters and the collection as a whole are not genetically fixed, but are in constant flux. While a hyperbola can model sampling of gene phamilies from a finite pool, it does not accommodate the influx of new phamilies. The addition of a linear term (see ‘Materials and methods’), representing the introduction of new phamilies from outside sources, results in a non-asymptotic curve which predicts the continual identification of new phams even after large numbers of genomes have been sampled (R > 0.999; Figure 7D). This linear term acts as a surrogate for the linear range of a second hyperbolic curve, one representing the resampling of a much larger set of gene phamilies available for introduction into mycobacteriophage genomes. Unfortunately, the current dataset remains insufficient to confidently extrapolate to give an estimate of the total number of viral protein families in the biosphere, which has been previously estimated to be anywhere between a half a million and 2 billion (Rohwer, 2003; Ignacio-Espinoza et al., 2013).
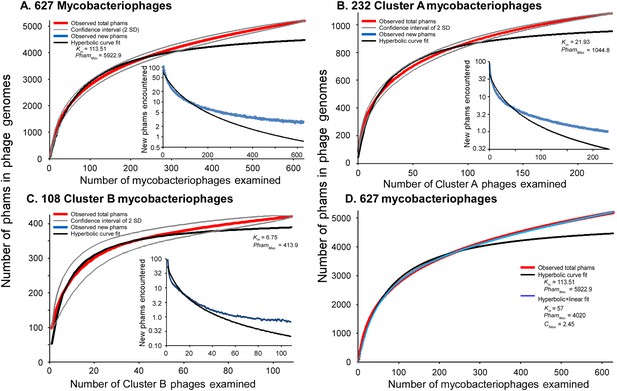
Rarefaction analysis of mycobacteriophage genomes.
(A) The numbers of phamilies are reported for between 1 and 627 phage genomes sampled at random without replacement; the mean of 10,000 iterations is shown in red; gray lines indicate a confidence interval of two standard deviations. The black line shows a hyperbolic curve fit to the data from phage counts 1 to 314. The inset shows the number of new phams encountered upon the inclusion of each phage, with the mean number for the 10,000 iterations shown in blue and the predicted value from the hyperbolic curve shown in black. (B) Rarefaction analysis of 232 Cluster A phages. The total numbers of phamilies are reported for between 1 and 232 phages sampled at random without replacement from Cluster A; the mean of 10,000 iterations is shown in red; gray lines indicate a confidence interval of two standard deviations. The black line shows a hyperbolic curve fit to the data from phage counts 1 to 117. The inset shows the number of new phams encountered upon the inclusion of each phage, with the mean number for 10,000 iterations shown in blue and the predicted value from the hyperbolic curve shown in black. (C) Rarefaction analysis of 108 Cluster B phages; the hyperbolic curve was fit to the data from phage counts 1 to 54. (D) Fits of the hyperbolic (Equation 1) and hyperbolic with linear (Equation 2) models for phamily identification within genome samples.
-
Figure 7—source data 1
Datasets for determination of rarefaction curves.
- https://doi.org/10.7554/eLife.06416.024
We note that because of the generally slow pace of the advancement of phage genomics, we have little insight into the phage populations of other hosts. We retrieved all double-stranded DNA tailed phage genomes in GenBank that we could identify (a total of 1781), corresponding to about 120 host bacterial genera, with a median number of phages per host genus of two. Using similar parameters for pham building as described above, the 181,717 predicted genes assemble into 47,479 phamilies. The relatively low representation of each phamily (3.8 genes/phamily) compared to the mycobacteriophages (13.4 genes/phamily) is a further reflection of the gross under-sampling of the phage population as a whole.
Implications for bacteriophage taxonomy
Bacteriophage taxonomic classification reflecting phylogeny presents substantial challenges because of genome mosaicism (Lawrence et al., 2002). Classification by viral morphology is well established, but may not accurately reflect the genetic relationships, as illustrated for the prolate-headed MooMoo (Figure 3). We also note that the mycobacteriophage myoviruses have a high CII and form a discrete group (Table 1) as do the Synechococcus myophages (Deng et al., 2014), perhaps reflecting a virulent lifestyle that constrains productive gene exchange; T4-related phages from diverse hosts share a core set of 15–20% of their genes, and whole genome comparisons reveal extensive mosaicism (Petrov et al., 2010). Host range mutability thus may differ in phages with different morphotypes, limiting access to the gene pool, and although grouping phages into clusters and subclusters provides analytical advantages because of the wide range in prevalence of different phages (Table 1), it is not suitable as a broadly applicable hierarchical taxonomic system. The comparative analysis of these mycobacteriophages thus supports reticulate taxonomies that more accurately reflect the phylogenetic complexities (Lawrence et al., 2002; Lima-Mendez et al., 2007).
Implications for student learning through research experiences
A research experience can be a powerful vehicle that enables a person to gain an understanding of the process of science (Hunter et al., 2007). When the research experience occurs early and at a large scale, as described here, the focus can shift from selecting a few ‘qualified’ students to exploring the potential interests of many students. Clearly, an essential ingredient is the nature of the research project, as definitions of research may vary from an inquiry-based exercise to authentic research with the potential to contribute publishable findings. To optimize the educational benefits, the research project must be intellectually and technically accessible to beginning students (i.e., few prerequisites) and scalable so that many students can simultaneously make progress in parallel, yet independently (Hatfull et al., 2006). Importantly, each student's findings should contribute to a scientific question with integration of all students' discoveries advancing a scientific question of significance, as judged by scientific peer review. This, we believe, defines an ‘authentic’ research experience. We note that in the SEA-PHAGES platform, substantial student effort is invested in arriving at high-quality genome annotations by close manual inspection followed by expert verification, a critical component of the detailed comparative analysis of phage gene content described here.
Concluding comments
Bacteriophage genomics has progressed relatively slowly compared to that of other microbes in spite of their relatively small genome sizes. Here we have demonstrated that programmatically integrating the research and education missions at large scale provides an effective solution to expanding our knowledge of viral diversity, with a multitude of insights gained as a consequence of the scale of phage discovery. The nature of different genomic types, the variations of the diversity both within clusters and shared genome content among clusters, and the expanse of the mycobacteriophage population can be viewed at an unprecedented level of resolution. Our conclusions align well with comparative analyses of phages of Enterobacteriacea (Grose and Casjens, 2014) and Bacillus spp. (Grose et al., 2014) and we predict that these are general parameters of bacteriophage diversity, at least when sampling broadly across the environment. Both the rarefaction analysis described here and preliminary analysis of phamilies of all sequenced DNA phages illustrate how little of the global phage population has been genomically sampled. With a near endless supply of diverse viruses readily accessible for isolation and analyses, integrated research/education programs will continue to play substantial roles in defining the nature of the virosphere.
Materials and methods
Phages and genomes
View detailed protocolIn addition to extant GenBank sequence information, mycobacteriophages were isolated, sequenced, and annotated in the Phage Hunters Integrating Research and Education (PHIRE) or SEA-PHAGES programs. Phage genomes were shotgun sequenced using either 454, Ion Torrent, or Illumina platforms to at least 20-fold coverage. Shotgun reads were assembled de novo with Newbler versions 2.1 to 2.9. Assemblies were checked for low coverage or discrepant areas, and targeted Sanger reads were used to resolve weak areas and identify genome ends. All genome sequences are publically available at phagesDB.org or in GenBank. Nucleotide comparisons used BLASTN or Gepard (Krumsiek et al., 2007).
Database construction
View detailed protocolTo create Phamerator database Mykobacteriophage_627, phamilies were constructed by first clustering the entire database of 69,574 genes using strict kClust parameters (70% clustering threshold and 0.25 alignment coverage of the longer sequence). This was followed by multiple sequence alignment of each preliminary cluster using Kalign (Lassmann and Sonnhammer, 2005). Consensus sequences were then extracted using HHmake and HHconsensus (Remmert et al., 2012). The resulting list of sequences was subjected to a second—and less strict—round of clustering via kClust (30% clustering threshold and 0.5 alignment coverage of the longer sequence) to obtain the final phamily assignments.
Network phylogeny constructions were made using the NeighborNet function with default parameters in SplitsTree (Huson, 1998; Huson and Bryant, 2006).
Cluster diversity and isolation indices
View detailed protocolFour parameters were used to evaluate cluster diversity. The first is the CLASP index that calculates the percentage of phamilies shared between two genomes, then averages across all possible pairs within a cluster or subcluster. Because the pairwise similarities are averaged, CLASP is relatively insensitive to either the overall size of the cluster, or the heterogeneity of its diversity (such as in Cluster C in which of the 45 genomes in total, 44 are in Cluster C1, and only one is in Cluster C2). CLASP robustness with respect to cluster size was demonstrated through a resampling analysis. For each cluster with more than 30 members, a random subset (of 5, 10, 20, or 30 genomes) was selected and CLASP was calculated. For each sample size, 20 iterations were performed with replacement. As expected, there is substantial deviation among the iterations, especially at smaller sizes. However, there is little change in the average CLASP values with different sample sizes (Figure 4—figure supplement 1), showing that cluster size is not a primary driver of diversity. The resampling analyses also suggest that while a greater number of genomes helps refine the CLASP value, there is still predictive power when only 10 genomes are compared. On average, the maximum and minimum iteration values at a sample size of 10 genomes were within 8% of the whole-cluster CLASP value. This implies that, for example, increasing Cluster D from 10 to 50 or 100 genomes may raise or lower its current CLASP value of 88.1, but that value is likely to remain between ∼80 and ∼96.
The second measure used is the CAP, which is calculated as the number of phamilies present in all genomes within a cluster divided by the average number of phamilies per genome. These cluster-conserved genes could correspond to core genes that define a particular phage group such as cluster or subcluster. However, for those clusters with sufficient diversity to detect such core genes, these values are low. For example, among the 66 Cluster F genomes, only five phamilies are present in all genomes. None are virion structural genes, one is a glycosyltransferase whose role is unknown, one is a putative regulator, and the others are small proteins of unknown function. For the Cluster A genomes, 11 phamilies are conserved, seven of which are virion structural proteins, three are involved in DNA metabolism (DNA Pol, Helicase, Rec-Like protein), and one is of unknown function.
The third parameter is the Cluster Phamily Variation (CPV) index, which is the proportion of phams that are not present in all members of the cluster. CAP and CPV are inversely related but imperfectly as CPV varies with cluster size even among similarly diverse clusters; a plot of CAP values against CPV values is shown in Figure 6—figure supplement 2.
The CCI is calculated as the average number of genes per genome as a percentage of the total number of phams in that cluster. Thus if all genomes in a cluster are identical (and if phamilies occur only once in a genome), CCI would be 100; the CCI for two sets of five randomly chosen genomes is ∼2. CCI values correlate with cluster size, but similarly sized clusters as such G, J, and L, or E and K have substantially different CCI values (Table 1).
The CII is the percentage of phams present within a cluster that are not present in other mycobacteriophage genomes.
Rarefaction analysis
View detailed protocolRarefaction analysis was performed by randomly selecting subsets (without replacement) of between 1 and 627 (all), 232 (Cluster A) or 108 (Cluster B) mycobacteriophages and determining the numbers of phamilies represented. This was repeated 10,000 times to generate a mean number of phamilies observed given a number of phage genomes selected. The means of the accumulated numbers of phams and the numbers of new phages identified are plotted as the function of the number of genomes selected at random. The observed numbers were fit to a hyperbolic function for 50% of the sample (i.e., 1 to 314, 116 or 54 genomes for all, Cluster A or Cluster B phages, respectively); Hanes-Woolf regression was used to estimate PhamMax and Km of the hyperbola:
where NGenomes is the number of genomes sampled, NPhams is the number of total phams seen within those genomes, PhamMax is the total number of phams among all mycobacteriophage genomes, and Km is the number of genomes required to sample one half of PhamMax.
The lack of fit of the observed data to the hyperbola—with the observed data reflecting infinite size—suggests that the overall population is dynamic. The lack of hyperbolic fit of the data does not result from outliers such as phages with highly deviant GC%, because removing these does not improve the fit. The fit is also not substantially improved by analysis of the two largest clusters, Cluster A and Cluster B (Figure 7), suggesting that the dynamic nature of the gene pool is not an artifact of examining independent phage clusters with separate gene pools.
To model this behavior, we modified Equation 1 to include the introduction of novel phams via recombination with outside, non-mycobacteriophage genomes:
where CPhage is the number of outside phams seen in each phage. The value of CPhage was estimated from Figure 7B and new values for PhamMax and KPham were estimated by Hanes-Woolf regression following data normalization.
Data availability
-
Mycobacteriophage databasePublicly available at the Mycobacteriophage Database (http://phagesdb.org).
-
Mykobacteriophage_627Accessed via the program Phamerator, publicly available at http://phagesdb.org/Phamerator/faq/.
References
-
Transcriptional silencing by the mycobacteriophage L5 repressorThe EMBO Journal 16:5914–5921.https://doi.org/10.1093/emboj/16.19.5914
-
Bacteria-virus coevolutionAdvances in Experimental Medicine and Biology 751:347–370.https://doi.org/10.1007/978-1-4614-3567-9_16
-
The viriosphere, diversity, and genetic exchange within phage communitiesCurrent Opinion in Microbiology 8:444–450.https://doi.org/10.1016/j.mib.2005.06.005
-
Mycobacteriophages: genes and genomesAnnual Review of Microbiology 64:331–356.https://doi.org/10.1146/annurev.micro.112408.134233
-
The secret lives of mycobacteriophagesAdvances in Virus Research 82:179–288.https://doi.org/10.1016/B978-0-12-394621-8.00015-7
-
Bacteriophages and their genomesCurrent Opinions in Virology 1:298–303.https://doi.org/10.1016/j.coviro.2011.06.009
-
Comparative genomic analysis of 60 mycobacteriophage genomes: genome clustering, gene acquisition, and gene sizeJournal of Molecular Biology 397:119–143.https://doi.org/10.1016/j.jmb.2010.01.011
-
Complete genome sequences of 63 mycobacteriophagesGenome Announcements 1:.https://doi.org/10.1128/genomeA.00847-13
-
Bacteriophage genomicsCurrent Opinion in Microbiology 6:506–511.https://doi.org/10.1016/j.mib.2003.09.004
-
Evolutionary relationships among diverse bacteriophages and prophages: all the world's a phageProceedings of the National Academy of Sciences of USA 96:2192–2197.https://doi.org/10.1073/pnas.96.5.2192
-
Application of phylogenetic networks in evolutionary studiesMolecular Biology and Evolution 23:254–267.https://doi.org/10.1093/molbev/msj030
-
The global virome: not as big as we thought?Current Opinion in Virology 3:566–571.https://doi.org/10.1016/j.coviro.2013.07.004
-
Genomic sequences of bacteriophages HK97 and HK022: pervasive genetic mosaicism in the lambdoid bacteriophagesJournal of Molecular Biology 299:27–51.https://doi.org/10.1006/jmbi.2000.3729
-
Salmonella phages and prophages–genomics and practical aspectsMethods in Molecular Biology 394:133–175.https://doi.org/10.1007/978-1-59745-512-1_9
-
Order to the viral universeJournal of Virology 84:12476–12479.https://doi.org/10.1128/JVI.01489-10
-
Comparative genomic analysis of 18 Pseudomonas aeruginosa bacteriophagesJournal of Bacteriology 188:1184–1187.https://doi.org/10.1128/JB.188.3.1184-1187.2006
-
The complete genomes and proteomes of 27 Staphylococcus aureus bacteriophagesProceedings of the National Academy of Sciences of USA 102:5174–5179.https://doi.org/10.1073/pnas.0501140102
-
Imbroglios of viral taxonomy: genetic exchange and failings of phenetic approachesJournal of Bacteriology 184:4891–4905.https://doi.org/10.1128/JB.184.17.4891-4905.2002
-
Metagenomics and future perspectives in virus discoveryCurrent Opinion in Virology 2:63–77.https://doi.org/10.1016/j.coviro.2011.12.004
-
Viral and microbial community dynamics in four aquatic environmentsThe ISME Journal 4:739–751.https://doi.org/10.1038/ismej.2010.1
-
Marine viruses–major players in the global ecosystemNature Reviews Microbiology 5:801–812.https://doi.org/10.1038/nrmicro1750
Article and author information
Author details
Funding
Howard Hughes Medical Institute (HHMI) (54308198)
- Graham F Hatfull
National Institutes of Health (NIH) (GM51975)
- Graham F Hatfull
Howard Hughes Medical Institute (HHMI) (52007054)
- Graham F Hatfull
Brigham Young University
- Sandra Burnett
Cabrini College
- David Dunbar
National Institutes of Health—INBRE (GM103408)
- R Luke Daniels
National Science Foundation (NSF)
- John Dennehy
Queens College
- John Dennehy
Lehigh University
- Vassie Ware
Merrimack College
- Janine LeBlanc-Straceski
National Institutes of Health (NIH) (GM094712)
- Nicanor Austriaco
National Institutes of Health—INBRE (GM103430)
- Kathleen Cornely
Davis Foundational Grant
- Kathleen Cornely
Providence College
- Kathleen Cornely
St. Joseph's University
- Christina King Smith
University of Houston, Downtown
- Rachna Sadana
University of Maine, Honors College
- Keith Hutchinson
National Institutes of Health (NIH) (GM1003423)
- Keith Hutchinson
Howard Hughes Medical Institute (HHMI)
- Michael Rubin
- Kirk Anders
University of Puerto Rico
- Michael Rubin
University of Wisconsin, River Falls
- Karen Klyczek
Western Kentucky University
- Claire Rinehart
Gatton Academy of Science and Mathematics
- Rodney King
Georgia College
- Indiren Pillay
Del Mar College
- John Hatherill
Miami University
- Iddo Friedberg
National Science Foundation (NSF) (DUE-1205059)
- John Hatherill
National Science Foundation (NSF) (ABI-1146960)
- Iddo Friedberg
Howard Hughes Medical Institute (HHMI) (52007572)
- SK Ireland
Doris Duke Charitable Foundation
- Michelle Larsen
Gonzaga University
- Kirk Anders
National Science Foundation (NSF) (DUE-1245778)
- Kirk Anders
David Asai, Kevin Bradley, and Lucia Barker (formerly) are (or were) employees of Howard Hughes Medical Institute who also provided support for the SEA-PHAGES and PHIRE programs. DA, KB, and LB contributed to the design of the programs and the systems for data collection.
Acknowledgements
Students, faculty, and their contributions to authorship are listed in the Supplementary file 2. We thank Aileen Beard, Gerald Henkel-Johnson, and Larry McGahey at the College of St. Scholastica, the Core Facility for Imaging, Cellular and Molecular Biology at Queens College, Jennifer Kelly and Towanda Kirksey-Stanton at Jacksonville State Univeristy, Susan Crump at Merrimack College and Dr Gregory Hendricks at the University of Massachusetts Medical School Electron Microscopy Imaging Facility, Melissa Cox at North Carolina State University and Valerie Lapham at the NCSU Center for Electron Microscopy, Dr Karen M Snetselaar at Saint Joseph's University, and Rick Ellingworth at the University of Wisconsin-River Falls for excellent technical assistance. We also thank Drs Winston Anderson and Broderick Eribo for their roles as consultants at Howard University, and Dr R Edelmann and the Miami University Center for Advanced Microscopy and Imaging for their support and assistance with electron microscopy. We also thank John Morrell, Alicia Brighton, Joshua Fisher, Michael Shelfo, Brigham Wright, Jessica Engle, Brian Early, Kyle Smith, Kyler Haskell, Tambi Issac, Bryce Lunt, David Payne II, Lissenya Argueta, Bryan Merrill, Adam Gardner, Hailey Meadows, Adam Hansen, and Marshall Sheide for contributions to phage isolation.
This work was supported in part by the Howard Hughes Medical Institute SEA-PHAGES program, by the Howard Hughes Medical Institute through its Professorship grant to GFH, and by NIH grant GM51975 to GFH. Additional support was provided by the Department of Microbiology and Molecular Biology and the BYU College of Life Sciences; Cabrini College; NIH Grant No. P20 GM103408 to the College of Idaho; the National Science Foundation grant 0703449, the CUNY LSAMP program, the Office of the Provost, the Division of General Education, the Division of Mathematics and Natural Sciences, the Queens College UM/RE program and the Biology Department at Queens College; the Department of Biological Sciences at Lehigh University; the Center for Biotechnology and Biomedical Sciences of the Department of Biology in the School of Science and Engineering at Merrimack College; the NCSU Biotechnology Program and Department of Microbiology; a Davis Foundation grant, the Providence College Undergraduate Research Committee, RI-INBRE and NIGMS grant R15-GM094712 to Providence College; the Department of Biology, Saint Joseph's University; the Natural Science Department at the University of Houston-Downtown; the University of Maine Honors College, the University of Maine Department of Molecular and Biomedical Sciences, Maine-INBRE and NIH-INBRE Grant 8P20GM1003423-12; an Institutional Development Award (IDeA) from the National Institute of General Medical Sciences of the National Institutes of Health (P20GM0103423) to the University of Maine at Machias; the Howard Hughes Medical Institute, RISE and BRIC Programs, the Department of Biology, and the Offices of the Academic Dean and Chancellor at the University of Puerto Rico at Cayey; the University of Wisconsin River Falls Biology Department; the Gatton Academy of Science and Mathematics in Kentucky and the Western Kentucky University Bioinformatics and Information Science Center; Georgia College STEM Initiative and a Georgia College Faculty Research Grant; NSF Grant REVISION DUE-1205059 and the Department of Natural Sciences at Del Mar College; Miami University Department of Microbiology and the College of Arts and Science Dean's office and National Science Foundation ABI award 1146960; the HHMI Scicomp Project (52007572) to Xavier University of Louisiana; the Doris Duke Foundation; the Gonzaga University Biology Department, NSF-TUES grant DUE-1245778 and HHMI Undergraduate Science Education grant to Gonzaga University; the School of Biological Sciences and the School of Molecular Biosciences at Washington State University; and the Benjamin Harris Memorial Fund through the Pittsburgh Foundation.
Copyright
© 2015, Pope et al.
This article is distributed under the terms of the Creative Commons Attribution License, which permits unrestricted use and redistribution provided that the original author and source are credited.
Metrics
-
- 20,821
- views
-
- 2,492
- downloads
-
- 274
- citations
Views, downloads and citations are aggregated across all versions of this paper published by eLife.
Download links
Downloads (link to download the article as PDF)
Open citations (links to open the citations from this article in various online reference manager services)
Cite this article (links to download the citations from this article in formats compatible with various reference manager tools)
Further reading
-
- Genetics and Genomics
Protein synthesis plays a major role in homeostasis and when dysregulated leads to various pathologies including cancer. To this end, imbalanced expression of eukaryotic translation initiation factors (eIFs) is not only a consequence but also a driver of neoplastic growth. eIF3 is the largest, multi-subunit translation initiation complex with a modular assembly, where aberrant expression of one subunit generates only partially functional subcomplexes. To comprehensively study the effects of eIF3 remodeling, we contrasted the impact of eIF3d, eIF3e or eIF3h depletion on the translatome of HeLa cells using Ribo-seq. Depletion of eIF3d or eIF3e, but not eIF3h reduced the levels of multiple components of the MAPK signaling pathways. Surprisingly, however, depletion of all three eIF3 subunits increased MAPK/ERK pathway activity. Depletion of eIF3e and partially eIF3d also increased translation of TOP mRNAs that encode mainly ribosomal proteins and other components of the translational machinery. Moreover, alterations in eIF3 subunit stoichiometry were often associated with changes in translation of mRNAs containing short uORFs, as in the case of the proto-oncogene MDM2 and the transcription factor ATF4. Collectively, perturbations in eIF3 subunit stoichiometry exert specific effect on the translatome comprising signaling and stress-related transcripts with complex 5’ UTRs that are implicated in homeostatic adaptation to stress and cancer.
-
- Cell Biology
- Genetics and Genomics
Calcineurin is a highly conserved calcium/calmodulin-dependent serine/threonine protein phosphatase with diverse functions. Inhibition of calcineurin is known to enhance the lifespan of Caenorhabditis elegans through multiple signaling pathways. Aiming to study the role of calcineurin in regulating innate immunity, we discover that calcineurin is required for the rhythmic defecation motor program (DMP) in C. elegans. Calcineurin inhibition leads to defects in the DMP, resulting in intestinal bloating, rapid colonization of the gut by bacteria, and increased susceptibility to bacterial infection. We demonstrate that intestinal bloating caused by calcineurin inhibition mimics the effects of calorie restriction, resulting in enhanced lifespan. The TFEB ortholog, HLH-30, is required for lifespan extension mediated by calcineurin inhibition. Finally, we show that the nuclear hormone receptor, NHR-8, is upregulated by calcineurin inhibition and is necessary for the increased lifespan. Our studies uncover a role for calcineurin in the C. elegans DMP and provide a new mechanism for calcineurin inhibition-mediated longevity extension.