Science Forum: RIPOSTE: a framework for improving the design and analysis of laboratory-based research
Figures
Box 1 Figure 1
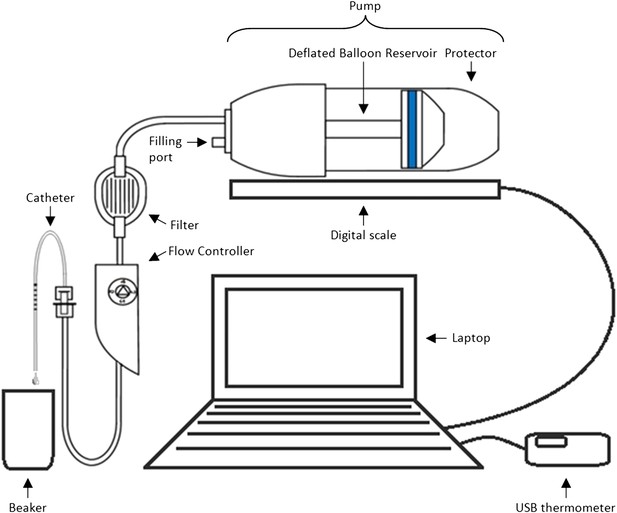
Equipment set-up for elastomer pump experiment.
https://doi.org/10.7554/eLife.05519.005
Box 2 Figure 1
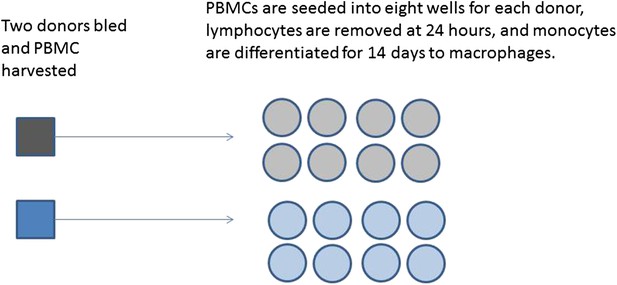
Production of differentiated macrophages from donor samples.
https://doi.org/10.7554/eLife.05519.007
Box 2 Figure 2
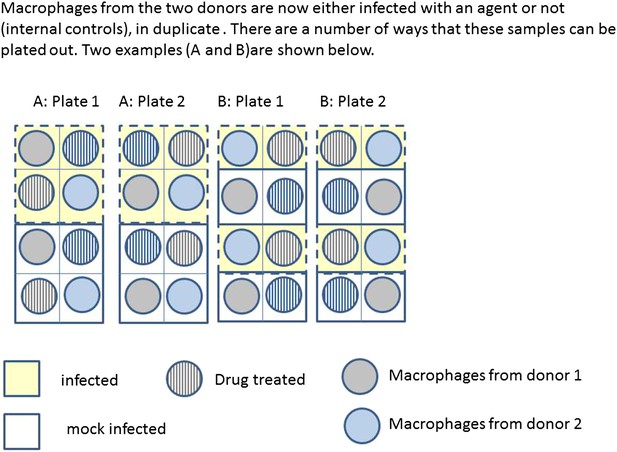
Infection and treatment of donor macrophages.
https://doi.org/10.7554/eLife.05519.008Tables
Box 1 Table 1
Two potential study designs in which either (a) four pumps and four catheters of the same type are tested simultaneously or (b) pump and catheter types are balanced during each 48 hr period of data collection, assuming only four pump-catheter units can be used concurrently and each is tested for 48 hr, three times in succession
Arrangement | Duration | Bench 1 | Bench 2 | Bench 3 | Bench 4 |
---|---|---|---|---|---|
Suboptimal design with potential for confounding | |||||
1 | 48 hrs × 3 | P C | P C | P C | P C |
2 | 48 hrs × 3 | P c | P c | P c | P c |
3 | 48 hrs × 3 | p C | p C | p C | p C |
4 | 48 hrs × 3 | p c | p c | p c | p c |
Optimal, balanced design | |||||
1 | 48 hrs × 3 | P C | P c | p C | p c |
2 | 48 hrs × 3 | P c | P C | p c | p C |
3 | 48 hrs × 3 | p c | p C | P c | P C |
4 | 48 hrs × 3 | p C | p c | P C | P c |
-
There are four benches of equipment being tested, each with one of each type of pump and one of each type of catheter (P = existing pump; p = new pump, C = existing catheter; c = new catheter).
Table 1
RIPOSTE discussion framework
Item | Prompt/Consideration | Details (relevance of question will depend on study type) |
---|---|---|
Research aims, objectives, specific outcomes and hypotheses | ||
Aims and objectives | Define the key aims of the study | What does the study ultimately aim to show? |
What are the primary and any secondary objectives? | ||
Outcomes, interventions and predictors of interest | Identify the variables and quantities/qualities of interest that will be measured (these may be different for each hypothesis) | What is the primary outcome/response variable? |
Are there any secondary outcomes you also wish to measure and/or assess? | ||
What are the key interventions/groups/predictors you will be testing? | ||
Research questions/Hypotheses | List the research question(s) that will be addressed and/or any hypotheses that you would like to test | The research question(s) should be defined in such a way that they |
- relate directly to the study objectives | ||
- relate to a specific outcome (or set of outcomes) and specific comparisons/predictors | ||
Each hypothesis should | ||
- be clearly testable | ||
- indicate what signifies a positive result for example, what is the minimum effect you would deem important? | ||
Study planning | ||
Logistical considerations | Ethical approval | Will ethical approval be required for the study? |
- Will statistical support be required for the ethics application? | ||
Statistical support | What level of ongoing statistical support is available for this study? | |
Data collection and management | How will the data be recorded and stored—will this require construction of a database? | |
What steps will be taken to validate the data entered against what was collected? | ||
Who will be responsible for data entry and validation? | ||
Will any additional information (‘meta data’) be recorded to indicate data quality? | ||
Materials and techniques | Laboratory equipment and methods | What specialist equipment and/or techniques will be used? |
Are there any aspects of these that may impact or limit the design of the study? | ||
Configuration and standardisation of materials and methods | Is there an accepted or validated way to measure the outcomes for this specific study or preliminary work be required to determine this? | |
What are the possible sources of variation or systematic bias between samples/batches/observers/laboratories/centres? | ||
Are any aspects susceptible to systematic variation and/or bias? What steps will be taken to minimise measurement bias and variation with consideration to: | ||
- Technical factors—such as sample collection, processing, storage and analysis? | ||
- Biological factors—which may include the effects of comorbidities, diet, medications, stress, biological rhythms etc, on the measurement variable? | ||
Possible steps to consider in addressing these sources of variation might be the use of existing standards for sample processing or analysis (e.g., BRISQ, ISO, ASTM or CLSI), equipment calibration and maintenance, user training, randomisation of interventions. | ||
Software | What software (if any) will be used during data processing/collection/storage? | |
What software will be used during data analysis—will specialist software be required? | ||
Does the software conform to any quality assurance standards, if applicable? | ||
Is the software up-to-date? | ||
What constraints/limits are there to the available resources? | What constraints are there? For example, due to cost and/or time | |
- Are there any limits in terms of the available equipment (e.g., number of plates/chips) or materials (e.g., binding agents/gels)? | ||
- What would be the maximum number of samples that could be used/processed given the available resources and time? | ||
Study design | ||
Design | Units of measurement | What are the sampling units in the study (e.g., blood samples from individuals)? |
Will the units be organised according to any structure (e.g., onto plates, chips, and/or into batches) or clustered/correlated in any way (e.g., samples from different centres), or within families, matched or paired samples/measurements? | ||
Will any repeated or replicate samples be taken? For example, any measurements over time; any biological replicates; any technical replicates. | ||
Are there any inclusion/exclusion criteria? | ||
Randomisation | Will any interventions or conditions be allocated at random to the units? | |
- If so, how? (e.g., method of random allocation and process of generating random numbers) | ||
- If not, why not? | ||
Are there any other possible confounders (e.g., batches or plates) to which the units may need to be randomly allocated? | ||
Blinding (masking) | Will blinding be used? If not, why not? | |
Who will be blinded and how? | ||
How will allocation be concealed and how will masking be maintained? | ||
Under what circumstances will the data be unblinded? | ||
Groups, treatments, and other predictors of interest | What are the primary groups or treatments of interest? | |
What is your control or comparison group? | ||
Are there multiple independent variables to assess simultaneously (for example, treatment and time)? If so, will a factorial design be used (involving testing all levels of each variable with all levels of each other)? | ||
Are there any interactions of interest (which may, for example, lead to factorial designs)? | ||
Use of analytical controls | What analytical controls will be used? For example, qualitative (positive/negative) and/or quantitative quality controls (QCs); comparative/normalisation controls | |
How will the controls be used/for what purpose? | ||
Other potential biases, confounders and sources of variability | Will you take any steps to minimise any background noise/variation? | |
Will you measure and take into account any potential confounding variables? For example, the age and sex of any participants; batch/plate/chip effects; etc. | ||
Sample size considerations | Sample size will depend on the primary objective of the study, whether the aim is to test hypotheses, estimate a quantity with specified precision or assess feasibility | |
Hypothesis testing: | ||
- Is there a single pre-specified primary hypothesis? Is a correction for multiple testing required? | ||
- What signifies a positive result (e.g., the minimum effect size, margin of agreement)? | ||
- What existing data are available to base the sample size calculation on? (e.g., SD of outcome) | ||
- What power and overall level of significance will be used? Will one or two tailed tests be used? | ||
Feasibility, pilot and proof of concept: | ||
- Understanding sources of variation (e.g., standard deviation of the outcome) | ||
▪ The sample size needs to be large enough to give an accurate estimates of any components of variation | ||
- Estimating with precision (e.g., proportion of samples that pass QC) | ||
▪ What is the acceptable precision (e.g., width of confidence interval) required? | ||
- Preliminary proof of effect (e.g., superiority of a new cell extraction technique) | ||
▪ What probability needs to be set to observe the correct ordering of your outcomes? | ||
▪ What level of significance would provide enough evidence to progress to fully powered study? | ||
Planned analysis | ||
Data assessment and preparation | QC criteria | What pre-specified criteria will be used to assess data from quantitative analytical QCs? |
What pre-specified criteria will be used to assure the reproducibility of results? | ||
- Will any thresholds be set to screen or benchmark data quality (e.g., setting a maximum coefficient of variation that would be deemed acceptable)? | ||
Data verification | Have you allowed time for data validation and correction to be completed prior to analysis? | |
Data normalisation/correction | Will the data be normalised or transformed in any way? If so, how? | |
Outliers | What methods and criteria will be used to identify any outlying data? | |
Statistical methods | Describe the different analyses to be performed | Which models or tests will be used (e.g., t-tests; ANOVA; mixed effects models etc)? |
- Do these methods appropriately handle any repeated or correlated measurements? | ||
What assumptions do the statistical methods rely upon? How will these be assessed? Do the data require any transformation? | ||
Which comparisons will be made? For example, will all pairs of treatments be compared, or will each treatment just be compared to a control? | ||
What covariates will be adjusted for? | ||
If applicable, what model terms will be fitted, for example, which main effects and interactions, which fixed and/or random effects? | ||
Will sensitivity analyses be performed to assess the validity of the findings? | ||
Missing data | What might be the reasons for missing data? | |
How will missing data be handled, for example, will missing data points be excluded or imputed? | ||
Multiple testing | Will a correction for multiple testing be required? If so, how many tests will be accounted for? | |
Which adjustment for multiplicity will be used, for example, Tukey, Bonferroni, false-discovery rate | ||
Interim analysis | Will interim analyses be performed (before the full number of samples dictated by the sample size calculation has been collected)? If so, for what purpose (e.g., to update the required sample size)? | |
Have any necessary adjustments to the sample size been made to account for the interim analysis? | ||
Replication and/or validation | Is there an intention to replicate the results (e.g., in an independent set of samples)? | |
In there an intention to validate the results (e.g., using a different technique or method of analysis)? | ||
Reporting results | ||
Guidelines/standards | Identify relevant reporting standards | What are the most appropriate reporting guidelines or standards that apply to the study design (e.g., BRISQ, MIFlowCyt and see www.equator-network.org). Identifying reporting standards at the planning stage helps to ensure that the information required to be reported is collected during the study and/or produced during the analysis of the data. |
-
This framework is intended to support discussion within the research team as a whole, including the statistician.
Table 2
Commonly encountered examples of analytical controls
Control type | Purpose |
---|---|
QCs | Qualitative QCs typically indicate whether specific aspects of the experimental and/or analytical procedure work in the intended ways, and are often included in the same analytical run used to collect study data. For example, a negative control may be a sample or unit that is known to be negative for the outcome and, hence, should assign a negative measurement in the assay. In contrast, a positive control would be expected to assign a positive result. |
Quantitative QCs are used to monitor the performance of a quantitative measurement system and ensure that it is performing within acceptable limits. Typically quantitative QC samples are run at two or more concentrations across the range of the assay and interpreted using graphical and statistical techniques, such as Levy-Jennings plots and Westgard rules. QC materials are generally not used for calibration in the same process in which they are used as controls. | |
In instances where any QC checks fail, certain aspects of the experimental procedure may have to be altered in order to remedy the problem or one or more units associated with the violation may have to be reprocessed until satisfactory checks are achieved. | |
Comparative/normalisation controls | These can be alternative physical or biochemical parameters measured alongside the analyte of interest usually within the same sample, for the purposes of normalisation and/or correction. For example, in RT-PCR housekeeping genes are usually amplified as well as targets of interest, with the final output expressed as a ratio between the target and the housekeeping gene. |
Download links
A two-part list of links to download the article, or parts of the article, in various formats.
Downloads (link to download the article as PDF)
Open citations (links to open the citations from this article in various online reference manager services)
Cite this article (links to download the citations from this article in formats compatible with various reference manager tools)
Science Forum: RIPOSTE: a framework for improving the design and analysis of laboratory-based research
eLife 4:e05519.
https://doi.org/10.7554/eLife.05519