Science Forum: The single-cell eQTLGen consortium
Figures
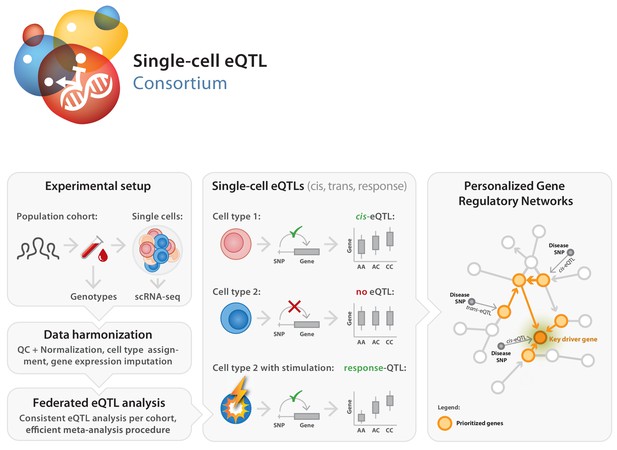
Set-up of the single-cell eQTLGen (sc-eQTLGen) consortium.
The sc-eQTLGen consortium combines an individual’s genetic information with single-cell RNA expression (scRNA-seq) data of peripheral blood mononuclear cells (PBMCs) in order to identify effects of genetic variation on downstream gene expression levels (eQTLs) and to enable reconstruction of personalized gene regulatory networks. Right panel is adapted from van der Wijst et al. (2018b).
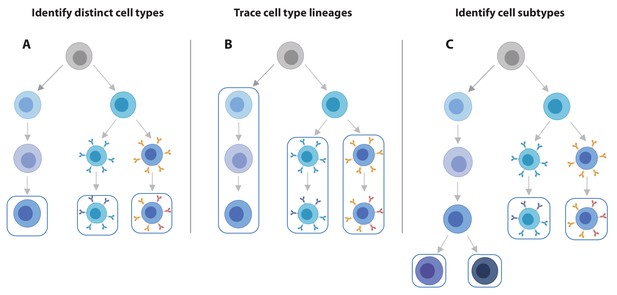
scRNA-seq data offers increased flexibility in the eQTL analysis strategy over bulk RNA-seq data.
Using scRNA-seq data for eQTL mapping offers a number of advantages over bulk RNA-seq based approaches, of which the flexibility in analysis strategy is a major one. (A) From single cell data, individual cell types can be identified and we can map eQTLs for each of these. (B) Alternatively, lineages based on either knowledge of cell developmental lineages or through pseudo-time based approaches can be constructed. By positioning cells across a trajectory dynamic changes in the allelic effects on gene expression levels as a function of trajectory position can be integrated. (C) Finally, as the discoveries of new cell subtypes are made or cell type definitions are being refined, the analysis can be revisiting by re-classifying cells and determining how the genetic effects on gene expression vary on these new annotations.
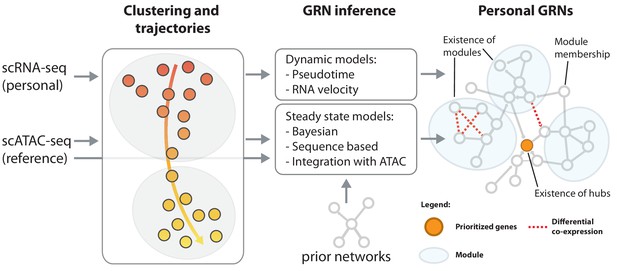
Reconstruction of personalized gene regulatory networks.
Individual and cell-type specific scRNA-seq data will be used to construct personalized gene regulatory networks. Some single cell datasets allow for the inference of trajectories, for instance in response to a stimulus. These can be used as input to dynamic models to infer causal (directed) interactions. Steady state datasets, characterized by cell type clusters can be analyzed with models that exploit co-expression, prior networks or cell type-specific reference scATAC-seq datasets in combination with sequence motifs to infer directed transcription factor-target relations. Topological comparison between personalized networks of groups of individuals can reveal coordinated differences, for instance the change of connectivity in densely connected modules, change of connectivity of hub genes or changes of module membership of individual genes.
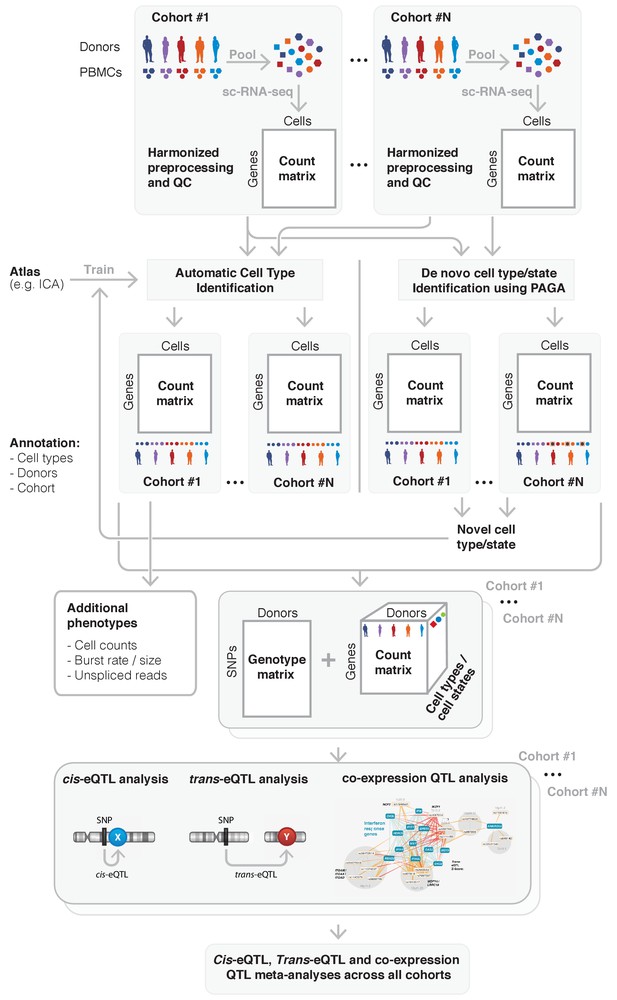
Overview of the sc-eQTLGen proposed federated approach.
sc-eQTLGen aims to identify the downstream consequences and upstream interactors of gene expression regulation. To increase the resolution and power of this analysis, datasets of multiple cohorts need to be combined while taking privacy issues into account. This will be done using a federated approach in which we will first harmonize all preprocessing and quality control (QC) steps across cohorts. Subsequently, shared gene expression matrices will be normalized and cell types will be classified based on a trained reference dataset (e.g. Immune Cell Atlas (ICA)). Any cells that cannot be classified using this trained classifier, representing new cell types or previously unknown cell states, can then be manually annotated based on marker genes, and then be used to further train the classifier. Each cohort will then separately perform a cis- and trans-eQTL and co-expression QTL analysis using their genotype and expression matrix, while using appropriate statistical models to account for effects such as gender, population structure and family-relatedness that can alter the genotype-expression relationship in a cohort-specific manner. The summary statistics will be shared and analyzed in one centralized place. Finally, these results will be used for reconstruction of personalized and context-specific gene regulatory networks. Bottom panel is reproduced from Võsa (2018).
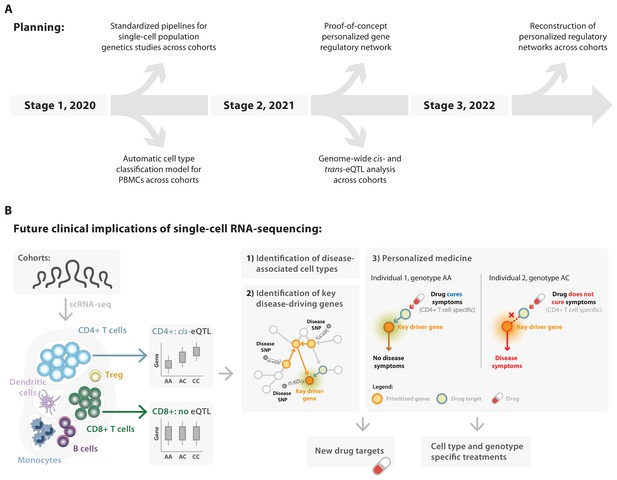
Deliverables of the single-cell eQTLGen consortium in relation to their future clinical implications.
(A) In the coming three years the sc-eQTLGen consortium aims to deliver the following: i) standardized pipelines and guidelines for single-cell population genetics studies (2020); ii) cell type classification models for PBMCs (2020); iii) summary statistics of cis- and trans-eQTLs, co-expression QTLs, cell count QTLs and variance QTLs (2021); iv) reconstruction of personalized, cell type-specific gene regulatory networks (2022). (B) These efforts of the consortium will lead to the (1) identification of disease-associated cell types and (2) key disease-driving genes, which together will aid (3) the implementation of personalized medicine and the development of new therapeutics that take all this information into account (cell type- and genotype-specific treatments). Panel B2 is adapted from van der Wijst et al. (2018b).