Developmental stage shapes the realized energy landscape for a flight specialist
Figures
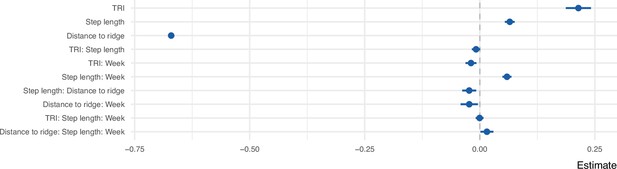
Coefficient estimates of the step selection function predicting the probability of use as a function of uplift proxies, week since emigration, and step length.
All variables were z-transformed prior to modeling. The error bars show 95% confidence intervals.
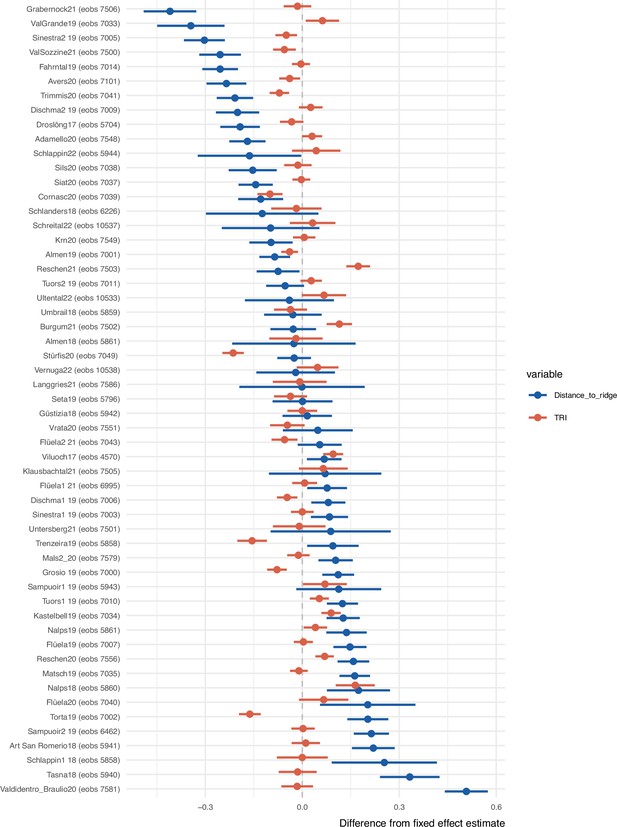
Individual-specific slopes for Topographic Ruggedness Index (TRI) and distance to ridge line.
The error bars show 95% confidence intervals. The difference between each individual’s estimate from the fixed effect estimate (Figure 1) is shown. Individuals are ordered based on their distance to ridge line estimates.
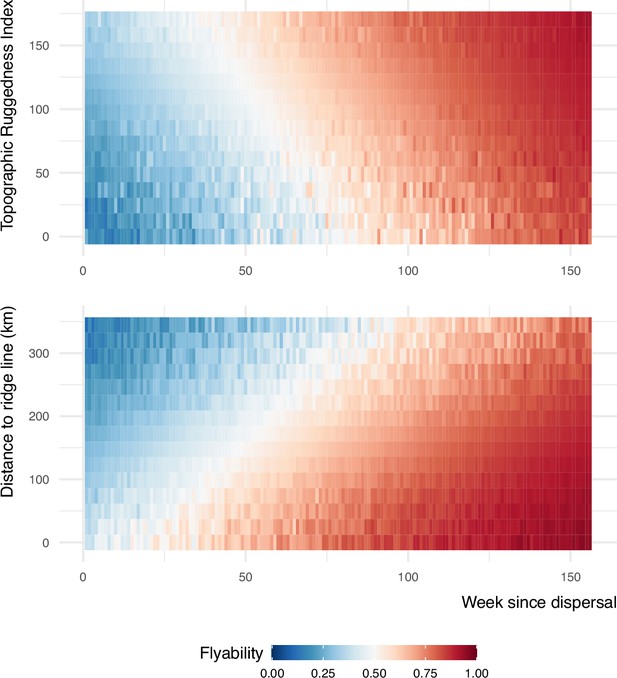
Flyability index predicted using the step-selection model for combinations of topography and week since emigration values.
The interactions between Topographic Ruggedness Index (TRI) and distance to ridge lines with week are among the set of criteria that young eagles used for selecting where to fly during their commuting flights.
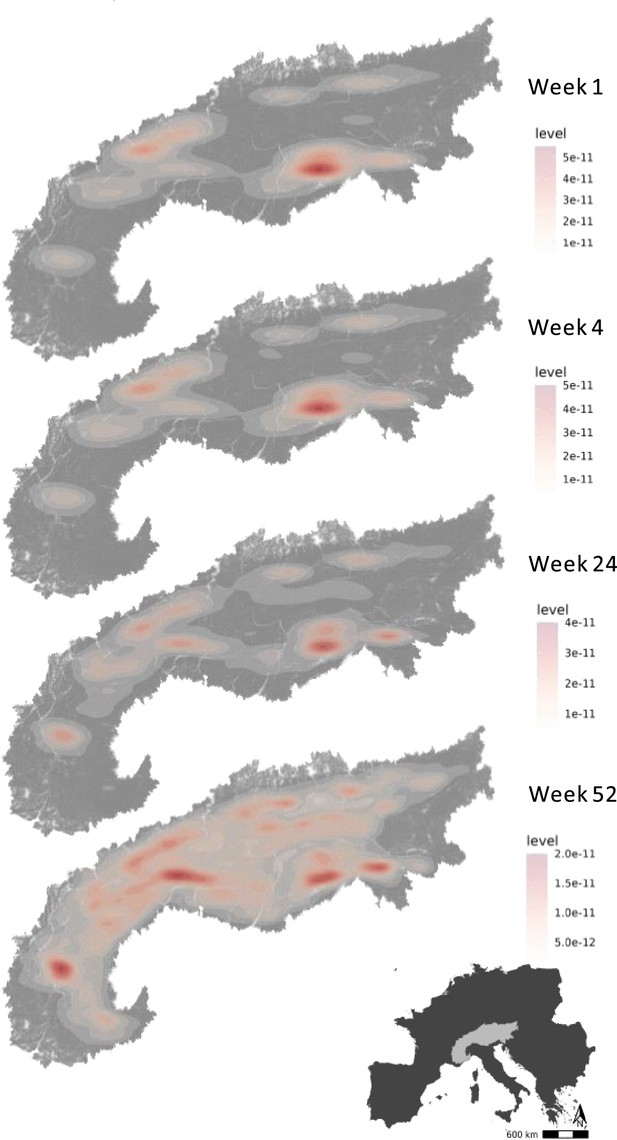
Hotspots of energy availability for golden eagles’ flight in the Alps.
Flyable areas were defined as cells within a 100 * 100 m grid with predicted flyability above 0.7 based on our step-selection model. The maps show the 2D kernel density estimation of flyable areas for golden eagles at different timestamps since dispersal: week 1, week 4 (1 month), week 24 (6 months), and week 52 (1 year). The raw prediction maps for every week since dispersal are shown in Video 1.
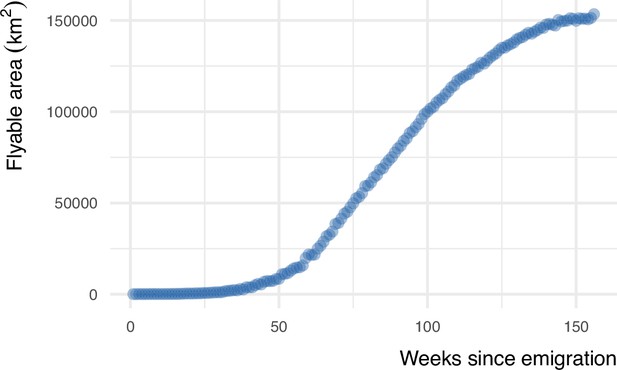
The flyable area for juvenile golden eagles in the Alpine region from the first week until 3 years after emigration.
Flyable area was defined as the total number of cells within a 100 * 100 m grid with predicted flyability larger than 0.7 based on the step-selection model. The positive trend shows that juvenile golden eagles can fly over a larger portion of the Alpine region as they age.
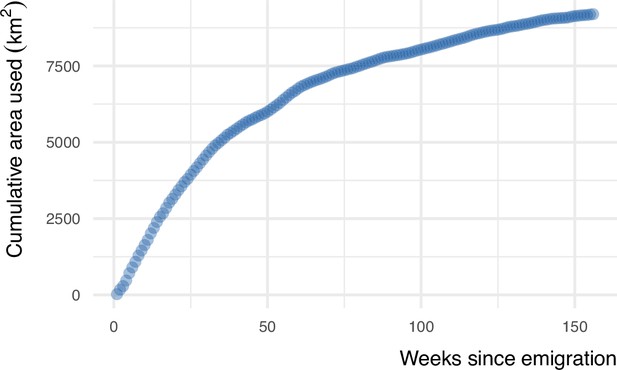
Cumulative area used by juvenile golden eagles during each week after emigration.
Used areas were calculated by extracting the commuting flights for each week, converting these to line objects, overlapping the lines with a raster of 100 * 100 km cell size, counting the number of overlapping cells and calculating the area that they covered. The predicted flyable area for juvenile golden eagles in the Alpine region for the same period of time is shown in Figure 4.
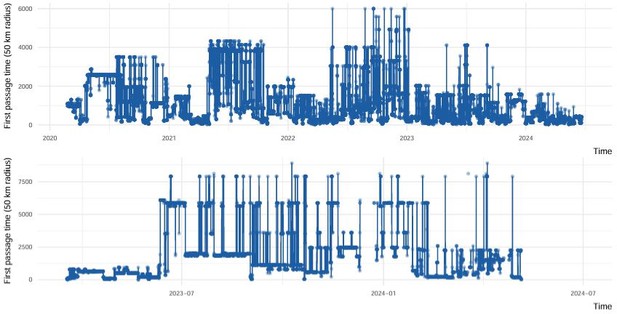
First passage times using a 50 km radius for two randomly selected individuals.

Golden eagle tracking points were either retained (used) or discarded (not used) for further data analysis based on the EmbC algorithm.
The point were clustered based on ground speed and height above ground.
Videos
The realized energy landscape of the aging golden eagles.
We used a step-selection approach to determine how juvenile golden eagles responded to topographic conditions during their commuting flights. Topography is a predictor of uplift potential and can be used as a proxy for energy availability for soaring birds. We used a step-selection model to predict flyability across the Alpine region from 1 week to 3 years after emigration. The fundamental energy landscape, defined as the total amount of energy available in the landscape, is constant, but the realized energy landscape, here estimated as flyability, changes. This is because the birds’ ability to perceive and exploit the energy within the landscape improves as they age, making the landscape cheaper to traverse. Flyability quantifies the suitability of a location for efficient flight, with higher values indicating areas where the bird is more likely to benefit from favorable uplifts. It represents the realized energy landscape that a bird can exploit based on the given topographic conditions and its own cognitive and locomotor abilities.
Additional files
-
Supplementary file 1
Details of bio-logging data included in the study.
The country and year of tagging for each individual, and the tracking duration (in terms of weeks since dispersal) that each individual contributed to the analysis, are reported. All individuals carried Bird Solar Tags manufactured by e-obs GmbH, Germany (either 45 gr or 25 gr devices). Individual local identifiers match those included in Movebank studies ‘LifeTrack Golden Eagle Alps’ and ‘LifeTrack Golden Eagle Alps Public’.
- https://cdn.elifesciences.org/articles/98818/elife-98818-supp1-v1.xlsx
-
MDAR checklist
- https://cdn.elifesciences.org/articles/98818/elife-98818-mdarchecklist1-v1.docx