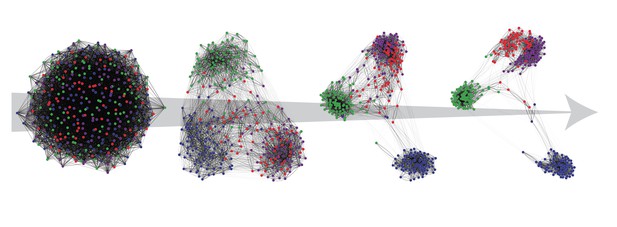
Increasingly separate groups of cells emerge as the algorithm Self-Assembling Manifold (SAM) repeats its steps to classify cell types and find informative differences in gene expression. Image credit: Alexander J. Tarashansky (CC BY 4.0)
New technologies have enabled scientists to closely examine the activity of individual cells. One increasingly popular technique to do this is called single-cell RNA-sequencing and it relies on the fact that although all cells in an organism carry the same DNA, different cell types use different genes. This technique is powerful but can struggle to identify meaningful distinctions between cell types, especially when the differences are subtle.
In single-cell RNA-sequencing, the messenger RNA (mRNA) copied from each gene is collected and counted, and usually the more a gene is copied the more active it is. Differences in gene activity (also called gene expression) between two cells often imply that they are different types of cells. However, since only an infinitesimal amount of mRNAs can be collected from a single cell, the counting is often inaccurate. In addition, the transient changes in gene expression can make cells of the same type have different gene expressions. These factors make it challenging to determine what genes are informative for distinguishing between cell types.
To address this problem, Tarashansky et al. have developed a computational approach called Self-Assembling Manifold (or SAM for short) to identify differences in gene expression that can lead to a better classification of cell types. First, SAM groups the cells randomly and looks for genes with different expression patterns between the groups. By looking at differences between groups instead of differences between individual cells, SAM is ‘averaging out’ individual differences within groups. SAM then uses the resulting information to re-classify the cells and start the process over again, taking the new groups and finding differences between them. SAM repeats these steps until the classification stops changing and becomes stable. SAM does not require any existing knowledge about cell types or gene expression, meaning it is unbiased and widely applicable. To test the usefulness of the algorithm, Tarashansky et al. used SAM to identify new cell types in the medically important parasitic worm Schistosoma mansoni, which infects hundreds of millions of people worldwide every year.
SAM can tell cell types apart better than existing approaches, and it can find meaningful differences in systems with a lot of meaningless variability as demonstrated by evaluating SAM’s performance on 55 other datasets. The potential applications of this approach are many, including the creation of detailed cell atlases recording the different types of cells throughout entire organisms.