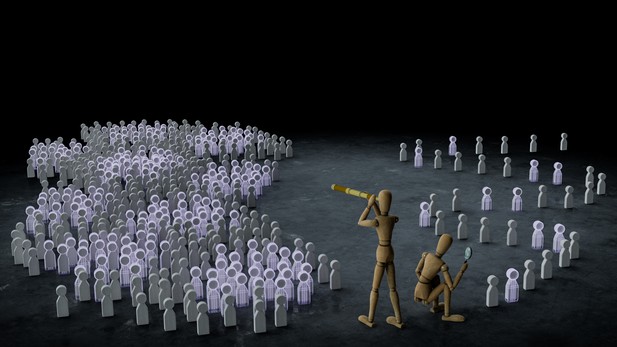
Image credit: Angus Paton (CC BY 4.0)
Scientists use statistical tools to evaluate observations or measurements from carefully designed experiments. In psychology and neuroscience, these experiments involve studying a randomly selected group of people, looking for patterns in their behaviour or brain activity, to infer things about the population at large.
The usual method for evaluating the results of these experiments is to carry out null hypothesis statistical testing (NHST) on the population mean – that is, the average effect in the population that the study participants were selected from. The test asks whether the observed results in the group studied differ from what might be expected if the average effect in the population was zero. However, in psychology and neuroscience studies, people’s brain activity and performance on cognitive tasks can differ a lot. This means important effects in individuals can be lost in the overall population average.
Ince et al. propose that this shortcoming of NHST can be overcome by shifting the statistical analysis away from the population mean, and instead focusing on effects in individual participants. This led them to create a new statistical approach named Bayesian prevalence. The method looks at effects within each individual in the study and asks how likely it would be to see the same result if the experiment was repeated with a new person chosen from the wider population at random.
Using this approach, it is possible to quantify how typical or uncommon an observed effect is in the population, and the uncertainty around this estimate. This differs from NHST which only provides a binary ‘yes or no’ answer to the question, ‘does this experiment provide sufficient evidence that the average effect in the population is not zero?’ Another benefit of Bayesian prevalence is that it can be applied to studies with small numbers of participants which cannot be analysed using other statistical methods.
Ince et al. show that the Bayesian prevalence can be applied to a range of psychology and neuroimaging experiments, from brain imaging to electrophysiology studies. Using this alternative statistical method could help address issues of replication in these fields where NHST results are sometimes not the same when studies are repeated.