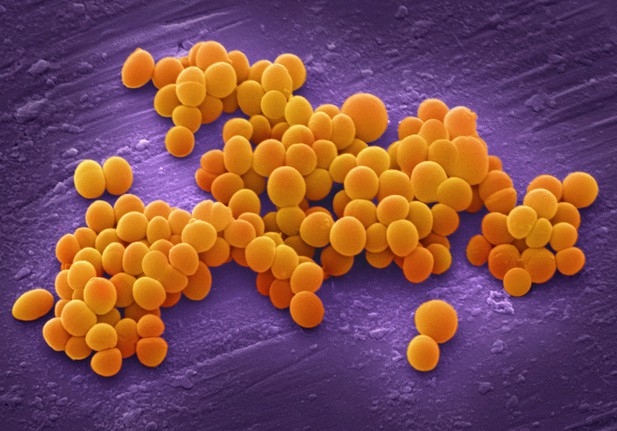
Clusters of Staphylococcus aureus bacteria that are resistant to the antibiotic drug methicillin. Image credit: Annie Cavanagh (CC BY-NC 4.0)
The rise in antibiotic resistance is threatening our ability to treat bacterial infections. Bacteria often evolve resistance by acquiring new genetic mutations during the treatment period. Understanding how resistance emerges and spreads through a bacterial population is crucial to prevent antibiotic drugs from failing.
Mathematical models are a useful tool for exploring how bacteria will respond to antibiotics and assessing the risk of resistance. Usually, these models only consider instances where bacteria acquire one genetic mutation that makes them virtually impervious to treatment. But, in nature, this is not the only possibility. Although some mutations do give bacteria a high level of resistance, numerous others only provide small amounts of protection against the drug. If these mutations accumulate in the same bacterial cell, their effects can combine to make the strain highly resistant to treatment. But it was unclear how the emergence of multiple mutations affects the risk of treatment failure and the diversity of the bacterial population.
To answer this question, Igler et al. devised a mathematical model in which each bacterium is able to mutate multiple times during the treatment period. The model revealed that if one mutation provides a high level of resistance on its own, the risk of bacteria surviving treatment is very high. But, if it takes more than two mutations to achieve a high level of resistance, the risk drops to almost nothing.
Igler et al. also found that the chance of bacteria evolving high enough resistance is affected by the type of antibiotics used and how fast the drug decays. With low-level resistance mutations, adapting treatment to maintain an acceptable number of sensitive bacteria as competitors for (a small number of) resistant bacteria was more effective at delaying treatment failure than trying to kill all the bacteria at once.
These findings suggest that adjusting the treatment strategy used for bacterial infections according to the proportion of low- and high-level resistance mutations could slow down the evolution of resistance. To apply these models in the real world, it will be important to measure the level of resistance conferred by single mutations. The type of models used here could also predict the response of other diseases that resist treatment, such as cancer.