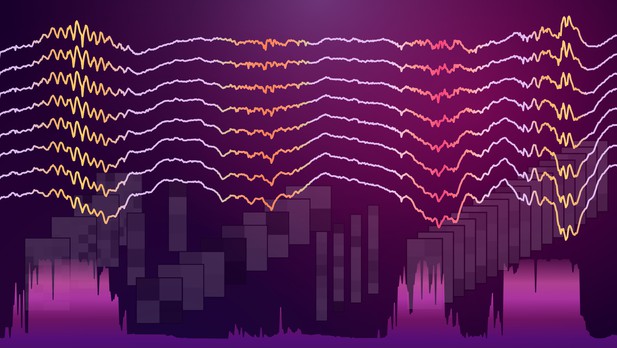
A short epoch of sharp-wave ripples detected by the convolutional neural network. Image credit: Andrea Navas-Olive (CC BY 4.0)
Artificial intelligence is finding greater use in society through its ability to process data in new ways. One particularly useful approach known as convolutional neural networks is typically used for image analysis, such as face recognition. This type of artificial intelligence could help neuroscientists analyze data produced by new technologies that record brain activity with higher resolution.
Advanced processing could potentially identify events in the brain in real-time. For example, signals called sharp-wave ripples are produced by the hippocampus, a brain region involved in forming memories. Detecting and interacting with these events as they are happening would permit a better understanding of how memory works. However, these signals can vary in form, so it is necessary to detect several distinguishing features to recognize them.
To achieve this, Navas-Olive, Amaducci et al. trained convolutional neural networks using signals from electrodes placed in a region of the mouse hippocampus that had already been analyzed, and ‘telling’ the neural networks whether they got their identifications right or wrong. Once the networks learned to identify sharp-wave ripples from this data, they could then apply this knowledge to analyze other recordings. These included datasets from another part of the mouse hippocampus, the rat brain, and ultra-dense probes that simultaneously assess different brain regions. The convolutional networks were able to recognize sharp-wave ripple events across these diverse circumstances by identifying unique characteristics in the shapes of the waves.
These results will benefit neuroscientists by providing new tools to explore brain signals. For instance, this could allow them to analyze the activity of the hippocampus in real-time and potentially discover new aspects of the processes behind forming memories.