Passive and active DNA methylation and the interplay with genetic variation in gene regulation
Figures
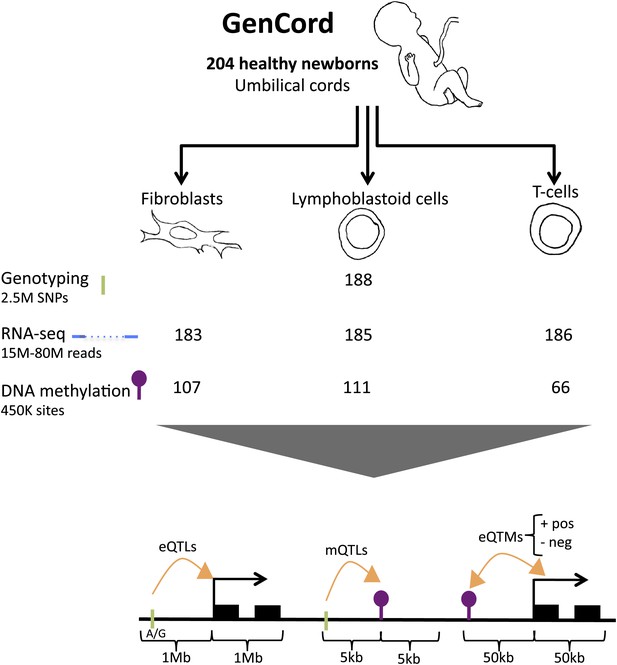
GenCord project scheme.
We collected umbilical cord and cord blood samples from 204 newborn babies, from which we derived three cell-types: fibroblasts, lymphoblastoid cells and T-cells. Genotyping, RNA-sequencing and DNA methylation levels were assayed. The number of samples without genetic and technical outliers is indicated for each assay and each cell-type. We then correlated and utilized different properties of all datasets in order to assess: expression Quantitative Trait Loci (eQTLs), methylation QTLs (mQTLs), positive (pos) and negative (neg) expression Quantitative Trait Methylation (eQTMs). Green ticks represent Single Nucleotide Polymorphisms (SNPs), purple lollipops represent methylation sites, black boxes represent exons and orange arrows depict associations between two data-types. Shown are the maximum distances between each pair of variables tested. See Figure 1—figure supplement 1–7 for data processing and quality checks.
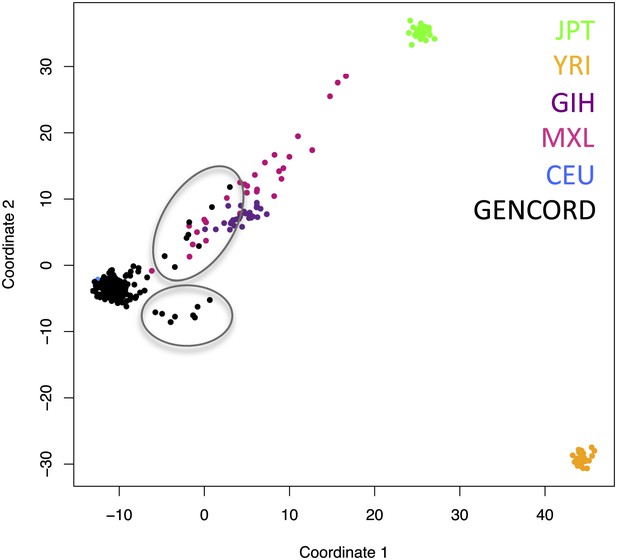
Genetic outliers removed from analyses involving genetic variation.
Multidimensional scaling (MDS) plot showing the genetic clustering of our 204 GenCord individuals (in black) and 30 individuals from each of the following HapMap populations: Western and Northern European in Utah (CEU, in blue), Japanese in Tokyo (JPT, in green), Yoruba in Ibadan, Nigeria (YRI, in orange), Gujarati Indians in Houston (GIH, in purple), Mexican ancestry in Los Angeles (MXL, in pink). The 16 GenCord individuals inside gray circles were considered genotypic outliers and were not included in analyses involving genetic variation.
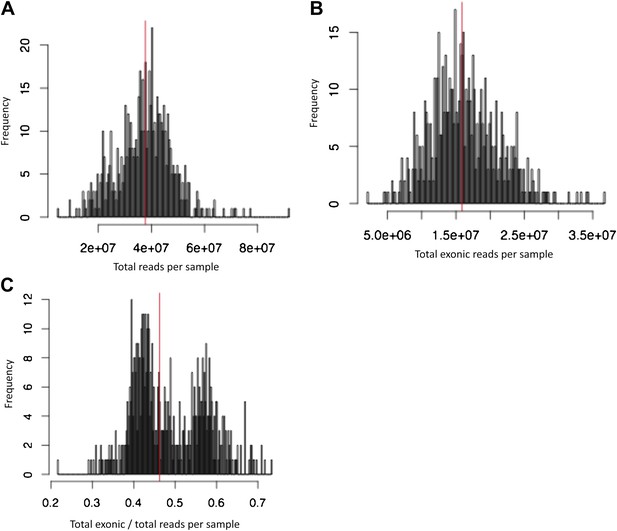
Number of reads per sample, before removing technical outliers.
(A) Number of total reads per sample. (B) Number of total reads mapping uniquely, properly paired and with MAPQ ≥ 10 to exons. (C) Proportion of reads mapping to exons. Vertical red lines indicate median. Samples with less than 5M exonic reads were considered technical outliers.
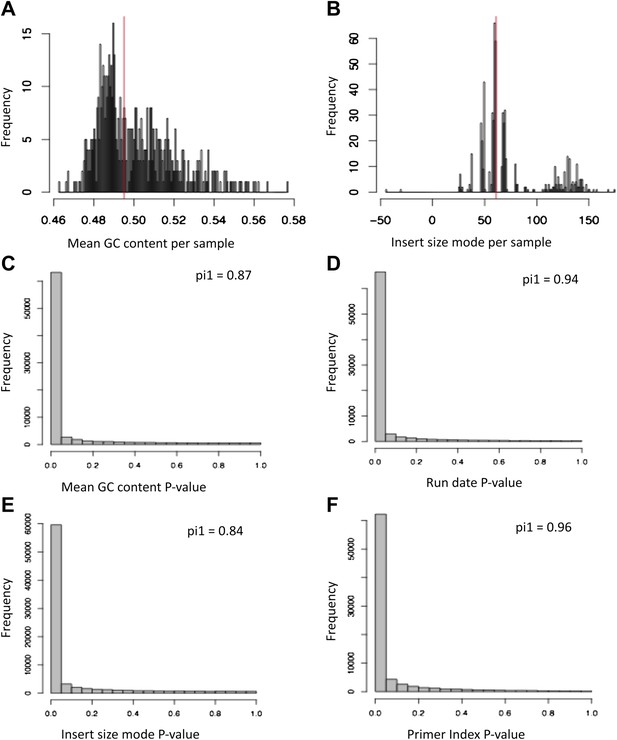
Covariates for which expression data was corrected.
(A) Histogram of mean GC content per library before removing outliers. (B) Histogram of insert size mode per library before removing outliers. The two samples in the extreme left were considered technical outliers and were removed. (C)–(F) p value distributions, with π1 indicated in each plot, of the linear regression effects of the four covariates we later corrected for on scaled exon levels.
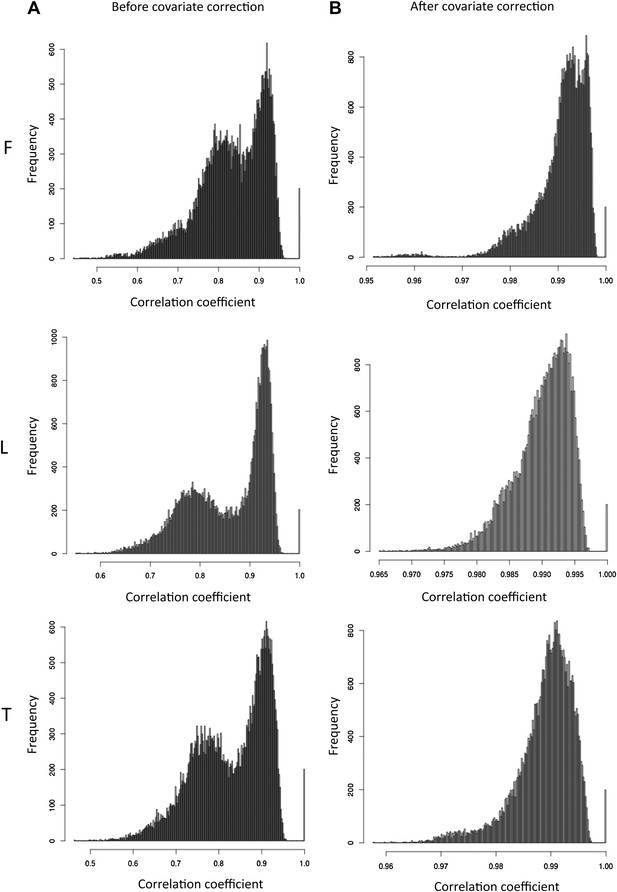
Pair wise correlations among individuals before and after covariate correction.
(A) Histograms of scaled exon counts pair wise spearman correlation coefficients between samples (all libraries scaled to 10M reads) in fibroblasts (F), LCLs (L) and T-cells (T). (B) Histogram of pair wise spearman correlation coefficients of expression levels after covariate correction between individuals in each cell-type.
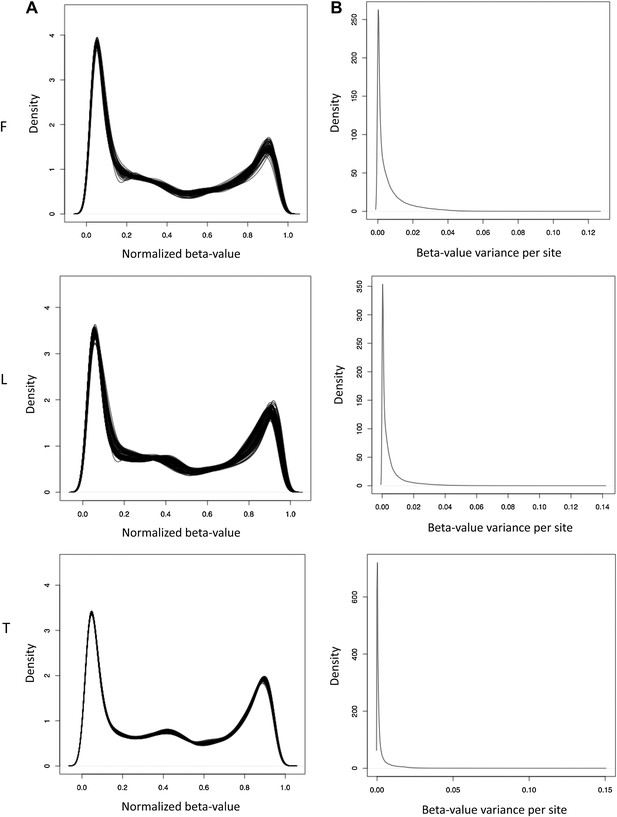
Normalized β-value and variance across individuals.
(A) Normalized beta-value distribution in fibroblasts (F), LCLs (L) and T-cells (T). (B) Distribution of normalized β-value variance per site across individuals.
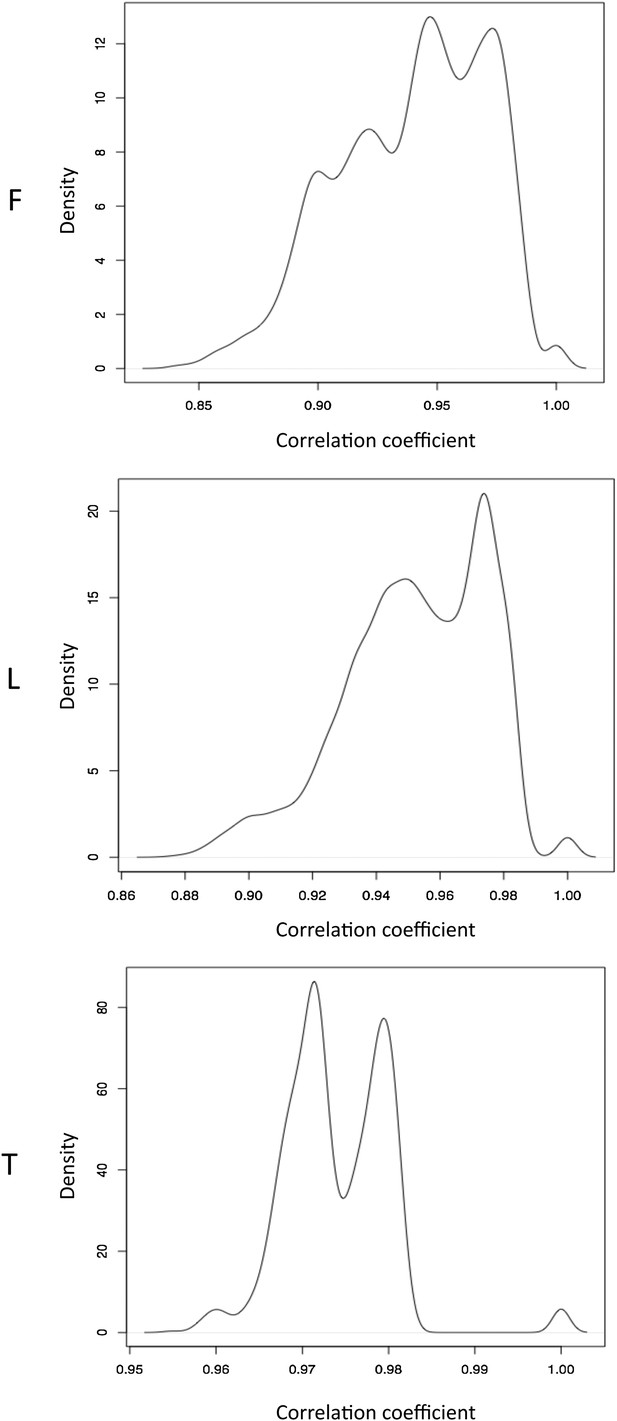
Normalized β-value pair wise correlations between individuals.
Distributions of pair wise spearman correlation coefficients between samples in fibroblasts (F), LCLs (L) and T-cells (T).
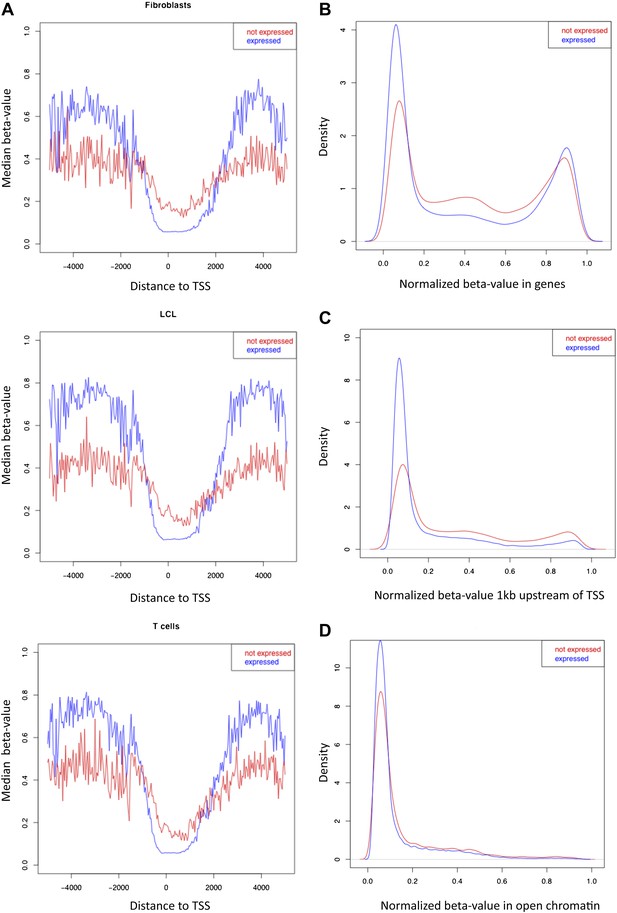
β-value distributions in distinct genomic features for expressed and non-expressed genes.
(A) Median β-value across individuals is plotted for CpG sites by their position relative to the nearest transcription start site (TSS) in each cell-type, for expressed (blue) and non-expressed (red) genes. Based on the region where expressed genes have lower methylation levels than non-expressed genes, the promoter proximal region of our analyses was defined from -1kb to +2kb relative to the TSS. (B)–(D) β-value distributions in genes (B), 1kb window upstream of TSS (C) and open-chromatin (D) in one LCL sample for expressed (blue) and non-expressed (red) genes. Other cell-types look very similar.
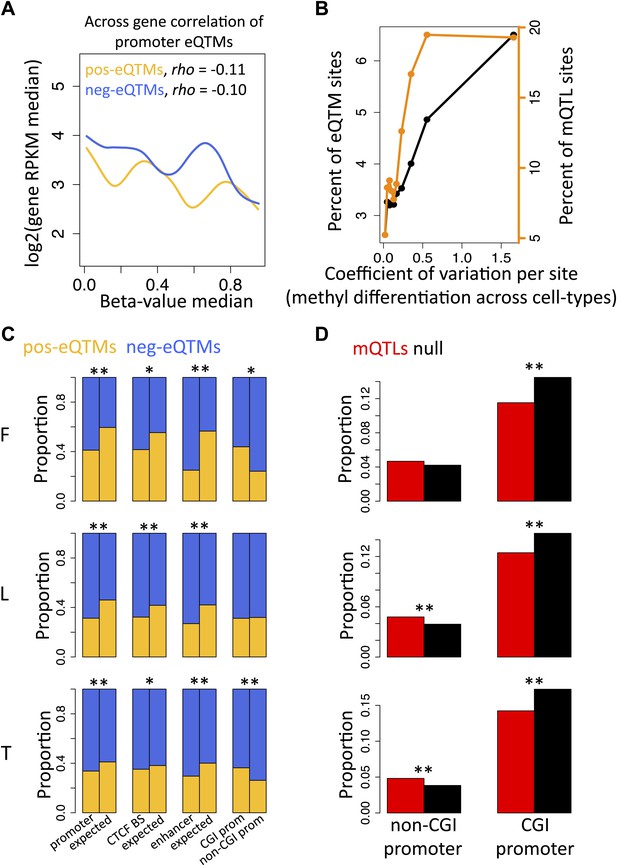
Inter-individual DNA methylation variation in cell-type differentiation and in different genomic contexts.
(A) The median methylation level of promoter eQTM sites (x-axis) correlates negatively with across gene median number of reads per kilobase per million reads (RPKM) irrespective of whether they are pos-eQTMs (yellow, N = 1149) or neg-eQTMs (blue; N = 5112). Spearman correlation coefficient rho is indicated in the plot with p=1.1 × 10−4 and p=1.7 × 10−13 for pos and neg-eQTMs, respectively. See Figure 2—figure supplements 1 and 2. (B) As the level of cell-type methylation differentiation increases (x-axis), a larger proportion of sites are associated to gene expression (eQTMs, left y-axis) and affected by genetic variation (mQTLs, right y-axis). Proportions are plotted by 10 bins each containing 10% of the data (0.1 quantiles). Level of methylation differentiation is measured for each site as the coefficient of variation of the median methylation level per cell-type. See Figure 2—figure supplement 3. (C) Proportion of eQTMs that are positive (pos-eQTMs, yellow) or negative (neg-eQTMs, blue) overlapping vs non-overlapping (expected) distinct genomic features (promoters, CTCF binding sites, enhancers), or overlapping CpG island promoters (CGI prom) vs overlapping non-CpG island promoters (non-CGI prom). For T-cells there are no CTCF or chromatin ChIP-seq data available so the data of an LCL were used instead (see Materials and methods). (D) For each non-CGI and CGI promoters (x-axis), the proportion (y-axis) of overlapping mQTLs was calculated (red bars) and was compared to the proportion of overlapping null SNPs (black bars). One star indicates p<0.05, two stars indicate p<1 × 10−6, Fisher’s exact test.
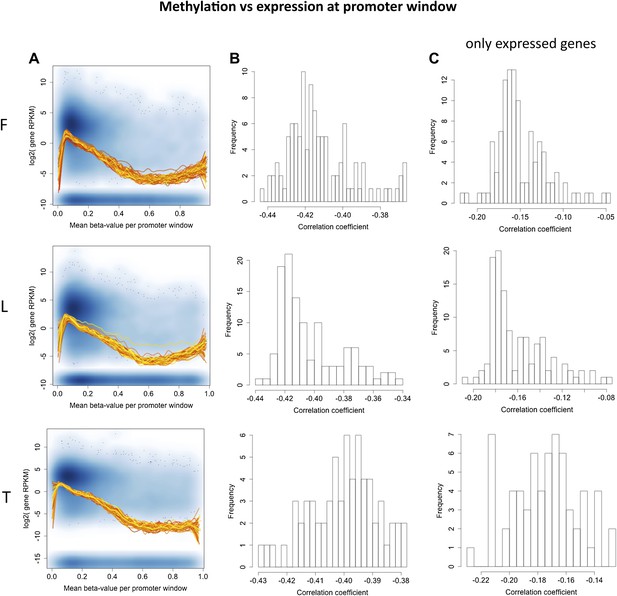
Correlation between promoter DNA methylation and across gene expression.
(A) Shown is the median methylation level across all sites falling in the promoter region of a gene (x-axis) by the gene expression level of the gene (y-axis) as the log2 of the median number of reads per kilobase per million reads (RPKM). The background scatter plot represents the data of a single individual, while the fitted lines depict the smoothened mean distribution of values for each individual. Non-expressed genes were artificially plotted at the bottom of the plot. (B) Histogram of Spearman rank correlation coefficients for each sample of correlations explained in (A). All p values<2.2×10−16. (C) Distribution of Spearman rank correlation coefficients for each sample of correlations explained in (A) taking only expressed genes, which were used in our eQTM analyses (at least 1 exonic read in >90% of individuals). In fibroblasts (F) all p values<6.2×10−8, in LCLs (L) all p values<8.2×10−18, in T-cells (T) all p-values<2.6×10−44.
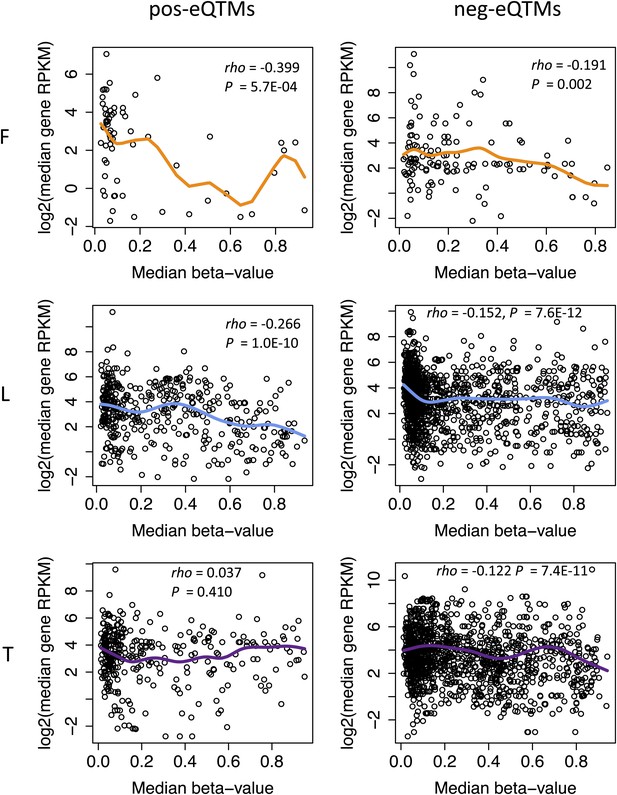
pos and neg-eQTMs correlation with across gene expression.
Shown are the correlations between the median methylation levels of eQTM sites (x-axis) and the median gene expression levels (y-axis) for positive-eQTMs (left panels) and negative-eQTMs (right panels) in fibroblasts (F), LCLs (L) and T-cells (T). The Spearman correlation coefficient rho and p value are indicated in each plot. Fitted lines depict the smoothened mean distribution of values.
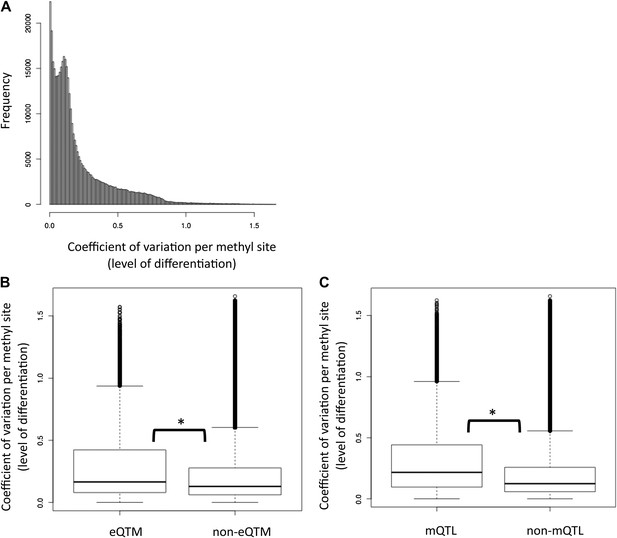
Tissue-specific methylation is enriched with eQTMs and mQTLs.
(A) Distribution of the level of differentiation metric which we defined as the coefficient of variation of the median methylation level per cell-type for each site (i.e., standard deviation of the medians divided by the mean of medians). (B) eQTM sites have higher levels of differentiation than non-eQTM sites (C) mQTL sites have higher levels of differentiation than non-mQTL sites. Stars between box plots indicate that the difference is significant with p<2.2×10−16, Wilcoxon test. eQTM and mQTL median methylation levels cover a wide range of β-values, so removing the 113 sites that were below the minimum or above the maximum median (representing effectively unmethylated or fully methylated sites) in all three cell-types did not alter the results at all.
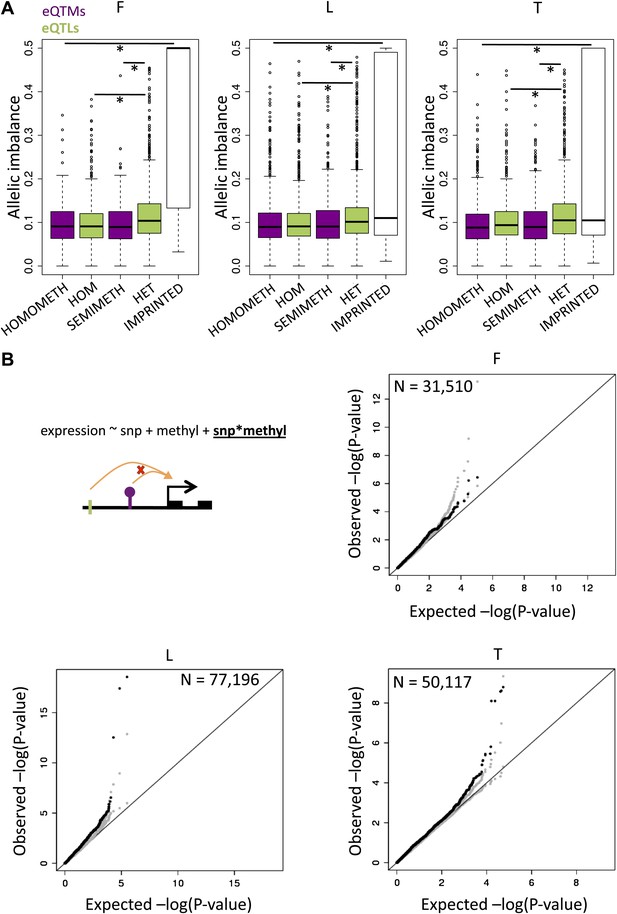
DNA methylation associated to gene expression is not significantly allelic and can interact with genetic variation.
(A) In green are depicted the distributions of allelic imbalance (i.e., absolute distance from the expected 0.5 ratio) of assayable heterozygote sites in eQTL genes of individuals that are homozygous (HOM) or heterozygous (HET) for the eQTL. The difference between distributions is significant in all cell-types with p<2.2 × 10−16, p=2.7 × 10−15 and p=3.0 × 10−8 in fibroblasts (F), LCLs (L) and T-cells (T), respectively (Wilcoxon test). This strongly indicates that allele specific expression is significantly driven by genetic variation. In purple are shown the distributions of allelic imbalance of assayable heterozygote sites in eQTM genes (excluding methylation sites affected by genetic variation) of individuals that are homomethylated (HOMOMETH, i.e., fully methylated, β-value > 0.7, or unmethylated β-value < 0.3) or semimethylated (SEMIMETH, i.e., β-value >0.3 and <0.7) for the eQTM site. The difference between distributions is not significant in any cell-type, with p=0.79, p=0.42 and p=0.49 in F, L, T, respectively. The difference between distributions of HET eQTLs and SEMIMETH eQTMs is significant in all cell-types with p=7.5 × 10−3, p=3.4 × 10−7, p=1.1 × 10−13, in F, L, T, respectively. The difference between distributions of IMPRINTED genes and HOMOMETH eQTMs is significant in all cell-types with p=6.9 × 10−34, p=9.7 × 10−4, p=8.5 × 10−5 in F, L, and T, respectively. This shows that allele specific expression is not significantly driven by DNA methylation that is not affected by genetic variation. (B) Using linear regression we tested the interaction term as shown in the illustrated formula for the effects of SNPs (green tick) and methylation sites (purple lollipop) on gene expression (black squared arrow and boxes). Qqplots illustrate the enrichment of low synergistic interaction observed p values (black), together with the 5th and 95th confidence limits based on expression permutations (gray) with respect to the expected uniform distribution.
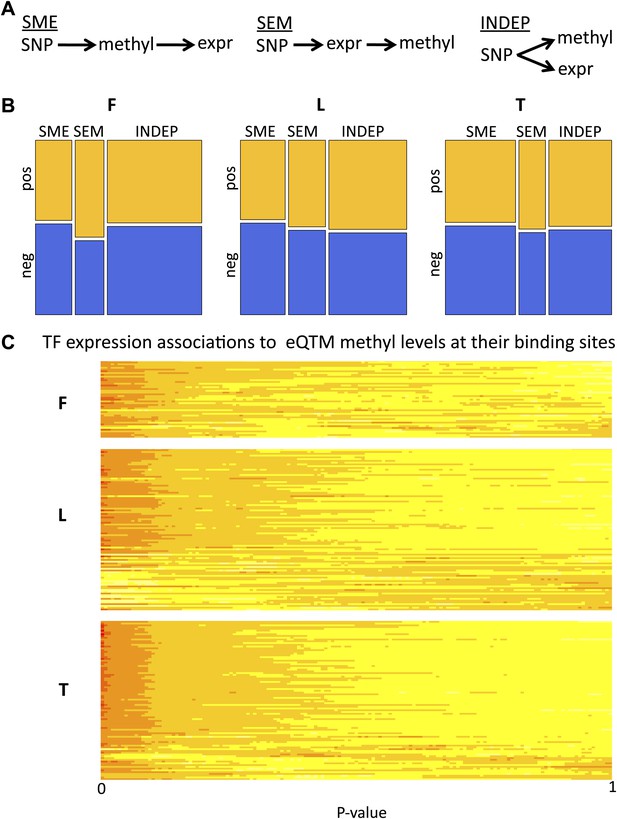
Passive and active roles of DNA methylation in gene regulation.
(A) Illustration of the three possible causative models tested of mechanistic relationships between genetic variation (SNP), DNA methylation (methyl) and gene expression (expr). Arrows indicate the causal direction of effects. The name of each model is underlined. (B) Mosaicplots illustrate the relative likelihoods of each model (x-axis), partitioned by the relative likelihoods of those involving pos-eQTMs (yellow) and neg-eQTMs (blue; y-axis), in fibroblasts (F), LCLs (L) and T-cells (T). The three types of models (INDEP, SME and SEM) are present in the three cell-types, suggesting that DNA methylation can have both active and passive roles in gene regulation. See Figure 4 –figure supplement 1 and Figure 4–Source data 1. (C) Heatmap of p value relative frequency distributions of spearman correlations between transcription factors (TF) and DNA methylation levels of eQTMs at their binding sites, sorted by π1. The enrichment of significant associations can be appreciated by the accumulation of reddish colors, reflecting higher relative frequencies, at low p values, and yellowish colors, reflecting lower relative frequencies, at higher p values. These results highlight one of the possible mechanisms of a passive role of DNA methylation regarding gene expression. See Figure 4—figure supplements 2 and 3.
-
Figure 4—source data 1
High confidence calls for INDEP, SME and SEM models in each cell-type.
- https://doi.org/10.7554/eLife.00523.019
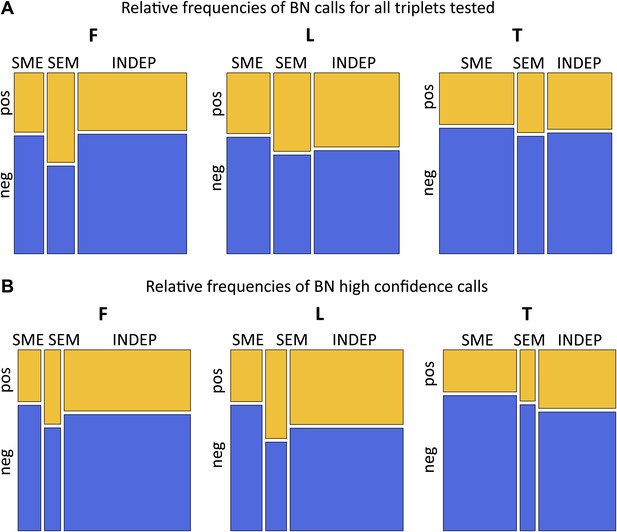
Passive and active roles of DNA methylation on gene regulation.
(A) For all exon-SNP-methyl triplets tested the best model was inferred using Bayesian networks (BN) and relative likelihood. Mosaicplots illustrate the relative frequencies of each model (x-axis), partitioned by the relative frequencies of pos-eQTMs (yellow) and neg-eQTMs (blue; y-axis), in fibroblasts (F), LCLs (L) and T-cells (T). (B) Same as in (A) only including high confidence calls (see ‘Materials and methods’ and Figure 4—source data 1).
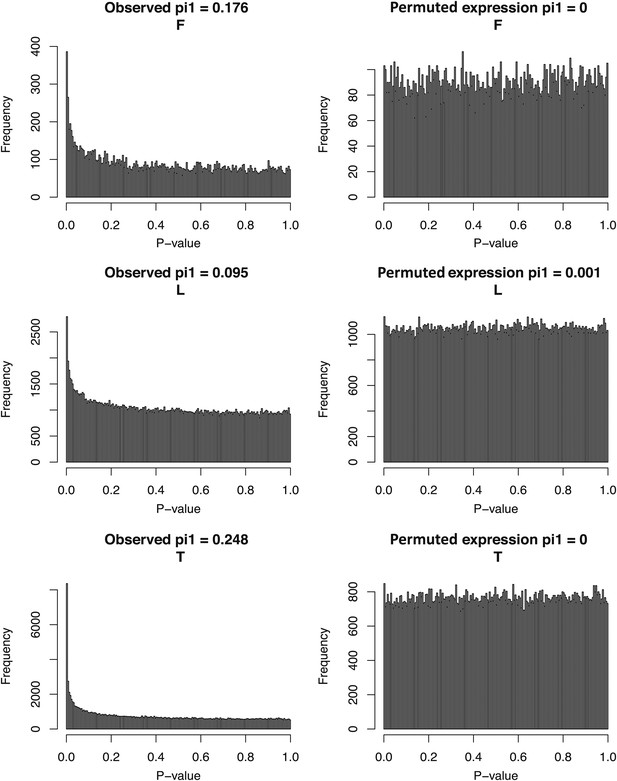
Associations between TF abundance and DNA methylation at their target binding sites.
Observed (left panels) and expected (right panels) p value distributions of spearman correlations between transcription factor expression levels and DNA methylation levels of eQTMs at their binding sites (see ‘Materials and methods’) in fibroblasts (F), LCLs (L) and T-cells (T). π1 statistic, reflecting the fraction of true positives, is indicated on top of each p value distribution.
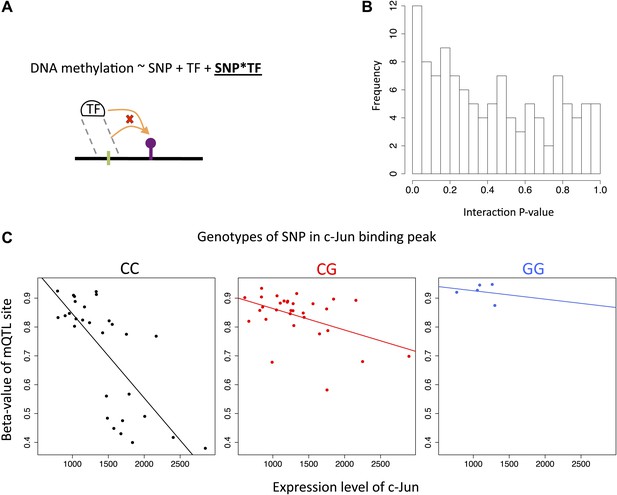
Interactions between SNPs and transcription factor levels on DNA methylation.
(A) We tested whether genetic variants that could potentially alter TF binding would interact with TF abundance (transcription level) on their effect on DNA methylation levels. To do so we took the top mQTL SNPs that fell in TF peaks and whose associated methylation site correlated significantly with the TF expression level of that peak (10% FDR for both types of associations), and required that the SNP and the TF abundance were not correlated and that the SNP had at least four minor allele homozygotes (N = 114). (B) We find an enrichment of low p values for interactions between genetic variants and TF abundance on DNA methylation levels, with π1 estimating 15% of true positives. (C) Plotted is the top interaction with p=1×10−4 involving the TF c-Jun in T-cells. The SNP falls in the binding peak of c-Jun. The methylation site and the SNP are 172 bp away from each other and both fall in an intron of gene SLC9A9.
Tables
Summary of main association analyses in GenCord.
https://doi.org/10.7554/eLife.00523.011Test | Samples | Window size | Unit | FDR (%) | Nominal p value | Fibroblasts | LCLs | T-cells | |
eQTLs | Genotypes and expression | 183 (F); 185 (L); 186 (T) | 1 Mb | Genes | 10 | 2.2 × 10−5 (F); 3.2 × 10−5 (L); 1.8 × 10−5 (T) | 2433 | 3372 | 2115 |
mQTLs | Genotypes and methylation | 107 (F); 111 (L); 66 (T) | 5 kb | Methylation sites | 10 | 4.4 × 10−4 (F); 7.9 × 10−4 (L); 1.3 × 10−3 (T) | 14,189 | 22,411 | 32,318 |
eQTMs | Methylation and expression | 110 (F); 118 (L); 66 (T) | 50 kb | Genes | 10 | 7.6 × 10−5 (F); 7 × 10−4 (L); 6.9 × 10−4 (T) | 596 | 3680 | 3838 |
-
Table 1—source data 1
Significant eQTL, mQTL and eQTM associations found in each cell-type.
- https://doi.org/10.7554/eLife.00523.012