A neural mechanism of speed-accuracy tradeoff in macaque area LIP
Figures
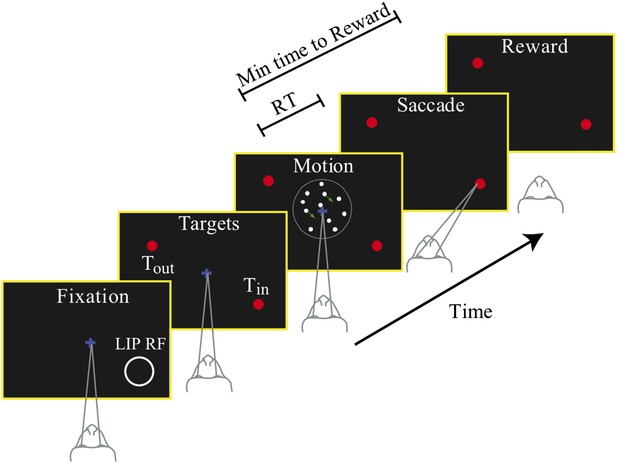
Choice-reaction time task.
Monkeys were required to identify the net direction of motion in a dynamic random dot display. Several levels of difficulty were randomly interleaved. The monkeys indicated their choice by making a saccadic eye movement to the appropriate choice target. They could do so at any time after onset of the random dot motion stimulus. Reaction time (RT) was measured from the time of motion onset until initiation of the saccade. The monkeys learned to perform the task in two different speed-accuracy regimes. For neural recordings, one choice target was placed in the response field of a single LIP neuron, as illustrated, and the other target was placed in the opposite hemifield. The circle showing the RF is for illustration purposes and was not displayed to the monkey. The target in the neuron's RF is referred to as Tin; the other target is referred to as Tout.
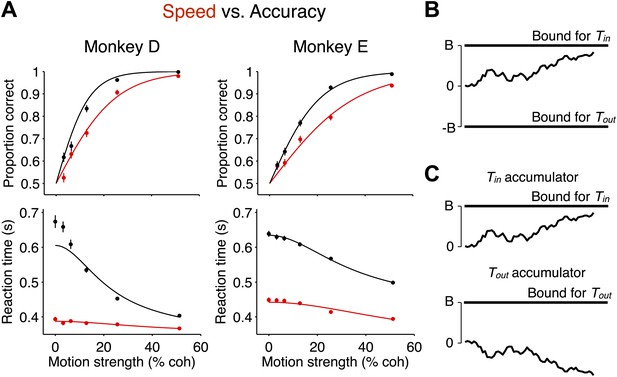
Decision accuracy and reaction time in two speed-accuracy regimes are explained by bounded evidence accumulation.
(A) Data from two monkeys. Black symbols and lines show the high accuracy regime. Red symbols and lines show the high speed regime. The top graph shows the proportion of correct choices plotted as a function of motion strength. Decision accuracy improved with stronger motion in both regimes. The bottom graph shows mean RT (±SEM) plotted as a function of motion strength. RTs decreased for stronger motion in both regimes. In the regime where the monkeys responded more slowly, their performance improved relative to the other regime. N = 3534 and 3307 trials for the high speed and high accuracy regimes, respectively, for monkey D and 4838 and 4733 trials for the same regimes for monkey E. (B and C) Bounded evidence-accumulation models. Noisy evidence is accumulated until it reaches a terminating threshold or bound, which establishes the time and sign of the decision. (B) Traditionally, this is represented as a single accumulation—or drift-diffusion process—between an upper and lower bound. (C) The model can be described equivalently as a race between two anticorrelated drift-diffusion processes, such that negative evidence for one process is positive evidence for the other. The decision is determined by the first accumulation to reach the bound. The traces in (A) show fits to the data using the bounded accumulation model.
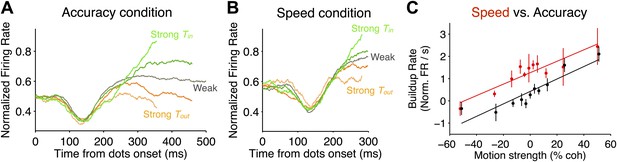
Comparison of neural responses accompanying decision formation in the two speed-accuracy regimes.
(A and B) Population firing rates plotted as function of time from stimulus onset and sorted by stimulus strength. Lighter green colors correspond to stronger Tin motion; lighter orange colors correspond to stronger Tout motion; darker colors correspond to intermediate stimulus strengths. Averages depict combined data from both monkeys. In both regimes, the responses exhibit characteristic ramping profiles that depend on stimulus strength approximately 200 ms after stimulus onset. Prior to averaging, responses for each neuron were normalized based on the peri-saccadic response (0–50 ms prior to saccade initiation) measured during a block of visually-guided delayed saccade trials. N = 35 neurons in each speed-accuracy regime. Error bars show SEM. For each condition, traces end at the point beyond which fewer than 50% of the trials would contribute to the averages, owing to the different RTs for each trial. (C) Buildup rate plotted as a function of motion coherence for the high accuracy regime (black) and the high speed regime (red). Error bars show SEM for the estimated buildup rates for each data point. Line fits capture the relationship between buildup rate and motion strength. Overall, the buildup rates were shifted to larger values in the high speed regime, but the change in buildup rate per unit motion strength was similar in the two regimes.
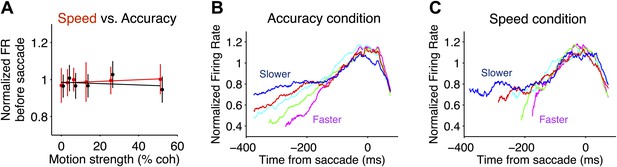
Comparison of neural responses accompanying decision termination in the two speed-accuracy regimes.
(A) Pre-saccadic responses plotted as a function of motion coherence for Tin choices. Firing rates were measured in a 50 ms window, centered 75 ms before the saccade (accuracy and speed regime in black and red, respectively). The flat regression is consistent with the idea that the responses reach a common value before Tin choices, for all motion strengths. Normalization procedure is identical to Figure 3. (B and C) Tin responses plotted as a function of time from the saccade and sorted by reaction-time quintile (colors). Averages depict combined data from both monkeys. In both speed-accuracy regimes, responses are stereotyped before the saccade. N = 35 neurons in each speed-accuracy regime. Error bars show SEM.
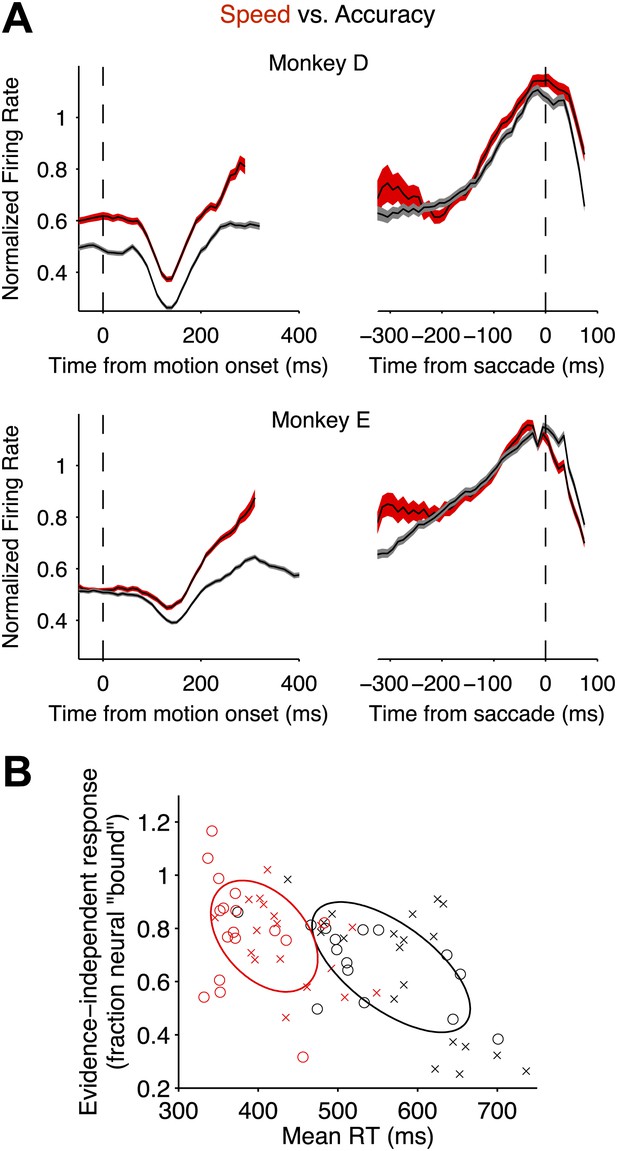
Speed-accuracy tradeoff alters LIP responses from the beginning of the decision process.
(A) Population firing rates plotted for each monkey. Red and black traces correspond to the speed and accuracy regimes, respectively. On the left, responses are plotted as a function of time from motion onset averaged across both directions of motion and all motion strengths (same normalization as in Figures 3 and 4). Trials contribute data to the average only up to 100 ms before the saccade. Averages are shown up to the time when half of the 50% coherent motion trials contribute data. On the right, responses are plotted as a function of time from the saccade. Responses are averaged across all trials that ended in a Tin choice. Trials contribute data to the average only from 200 ms after motion onset onwards. Curve thickness (shading) shows SEM. N = 17 and 15 neurons for the high speed and high accuracy regimes, respectively, for monkey D. N = 18 and 20 neurons for the high speed and high accuracy regimes, respectively, for monkey E. (B) The relationship between the magnitude of the evidence-independent neural response and mean RT across experiments. The response statistic captures the evidence-independent component of the firing rate early in the decision process, expressed as a fraction of the average response from the neuron 75 ms before all Tin choices (‘Materials and methods’). Larger values imply that less evidence-dependent signal is required to reach the bound. A value of 1 indicates that on average the evidence-independent signal alone would reach the ‘neural bound’ by 300 ms. Neurons from monkey D are shown as circles; neurons from monkey E are shown as crosses. Red symbols correspond to neurons recorded in the high speed regime; black symbols correspond to the high accuracy regime. Ellipses are drawn to the 50% confidence region based on the covariance matrix calculated for each speed-accuracy regime. The correlations in each regime were significant (p<0.05).
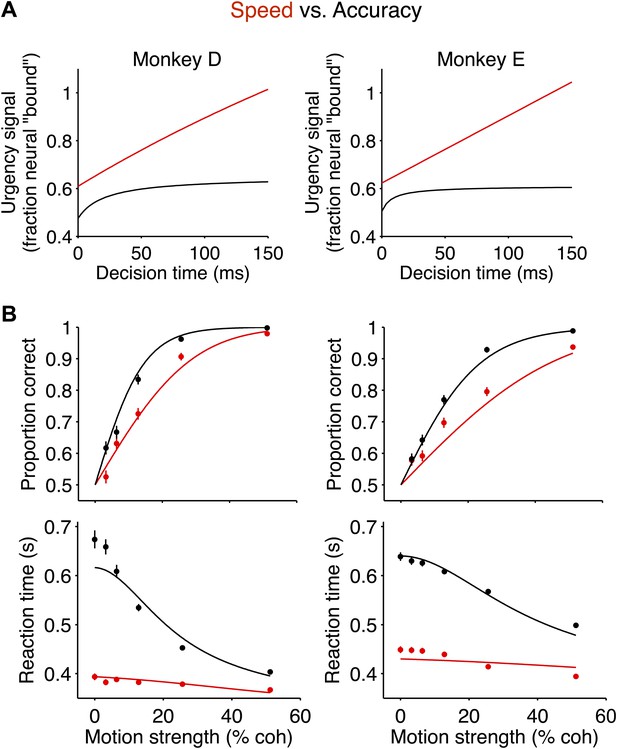
The neurally-derived urgency signal explains the magnitude of the behavioral change between speed-accuracy regimes.
(A) Hyperbolic ‘urgency’ functions fit to the neural responses from 200 ms after motion onset (decision time = 0) to the point in time beyond which fewer than 50% of the trials contribute to the averages for the highest motion strength in each regime (Figure 5A, Equation 6 in ‘Materials and methods’). Left column is for monkey D; right column is for monkey E. (B) Neurally-constrained fits to behavior. Points show behavioral data from Figure 2. The curves are neurally-constrained model fits to the data. All model parameters are shared (i.e., constrained to be equal) between speed-accuracy regimes except for the difference in excursion, which is measured directly from the neural responses.
Tables
Bayes Information Criterion (BIC) comparison of fits to behavioral data based on neurally-constrained models
Model | monkey D | monkey E |
---|---|---|
Baseline only | 664 | 340 |
Time-dependent only | 124 | 174 |
Full urgency signal | 71 | 170 |
-
Because the neurally-derived signals are incorporated in the model via a single scaling factor, all of the fits use the same number of free parameters. Lower BIC values indicate preferred models.