Resting state functional connectivity in the human spinal cord
Figures
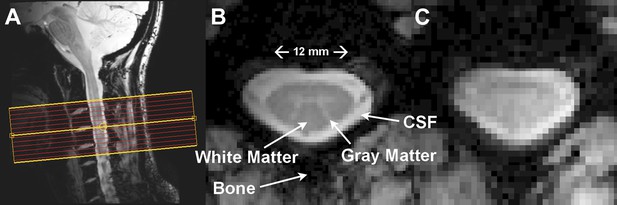
Resting state spinal cord fMRI at 7 Tesla.
(A) Mid-sagittal slice from a healthy volunteer showing the complete cervical cord and typical axial slice placement for this resting state study. In all subjects the imaging stack was centered on the C3/C4 junction, providing full coverage of C3 and C4 and partial coverage of C2 and C5. (B) T2*-weighted anatomical image at C4 acquired with 0.6 × 0.6 × 4 mm3 voxels and interpolated to 0.31 × 0.31 × 4 mm3. Excellent contrast permits visualization of the characteristic butterfly-shaped gray matter column. (C) A single T2*-weighted functional image of this axial slice (acquired with 0.91 × 0.91 × 4 mm3 voxels). Functional images are high quality with minimal geometric distortions and T2* blurring and permit adequate spatial delineation between white matter and cerebrospinal fluid.
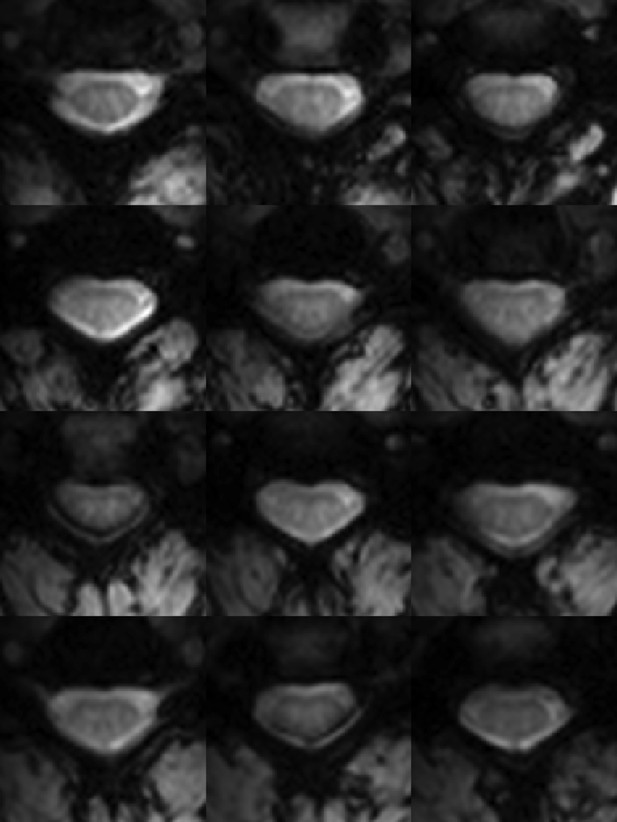
Functional weighted spinal cord images at 7 Tesla.
A single volume of twelve contiguous T2*-weighted slices centered on the C3/C4 junction (as illustrated in Figure 1A) in one subject. Each volume was acquired with 0.91 × 0.91 × 4 mm3 voxels and resampled to 0.31 × 0.31 × 4 mm3 voxels during the affine functional-to-anatomical registration. Excellent contrast between white matter and cerebrospinal fluid facilitates accurate registration between such functional volumes and high-resolution anatomical images (Figure 1B). The use of a 3D acquisition sequence with relatively short echo time and relatively few k-space lines per radiofrequency pulse provides high-quality images with minimal signal drop-out and geometric distortions, although artifacts caused by fat shift of the nerve root sleeve in the phase-encode direction still affect the dorsal edge in a few slices.
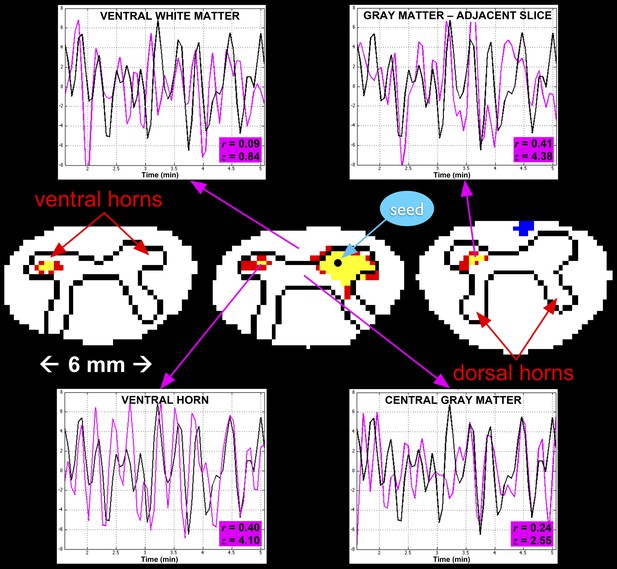
A single-subject analysis of resting state functional connectivity with corresponding time series.
For clarity, only outlines of the gray matter butterfly and surrounding white matter are shown (rostro-caudal from left to right). Red and yellow represent statistically significant positive correlation with the seed time series (using a two-sided 99.9% confidence interval where red is 3.29 < z ≤ 3.89 and yellow is z > 3.89), and blue represents negative correlation (z < −3.29). The seed voxel is selected in the right ventral horn in C5, and exhibits functional connectivity with the contralateral ventral horn in the same slice as well as adjacent slices. Such connectivity between ventral horns is observed across all subjects. In each of the four plots, a 3.5-min segment of the seed time course is shown in black and the time course of the corresponding region of interest is shown in magenta. The highest correlations are observed in the contralateral ventral horn on the same slice (z = 4.10) and on the adjacent slices (z = 4.38). Correlations with central gray matter (z = 2.55) and adjacent white matter (z = 0.84) are relatively low, which, given the small size of the spinal cord, suggest that such correlations are genuine and not dominated by widespread physiological noise.
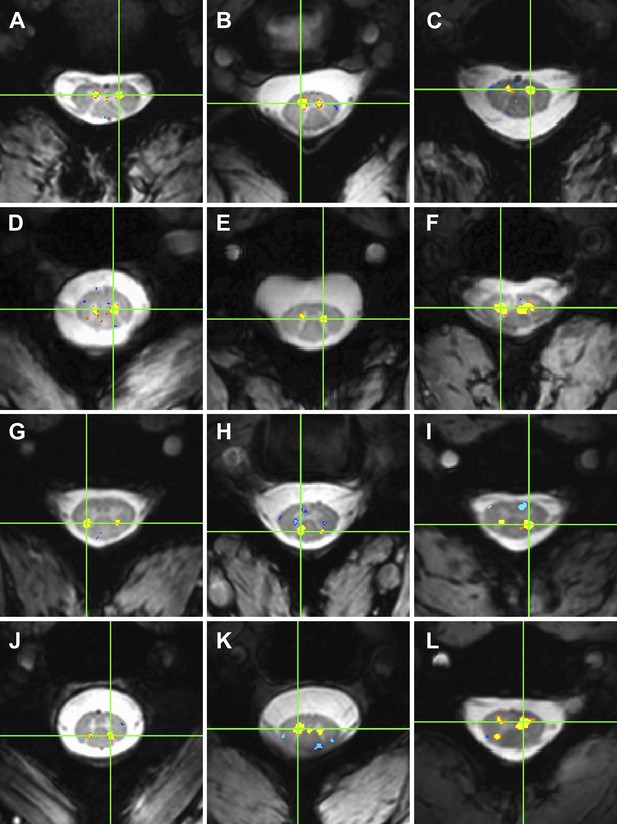
Examples of within-slice resting state functional connectivity across subjects.
These analyses were performed using AFNI's ‘InstaCorr’ with p < 0.001 and no minimum cluster size. In each panel, a seed voxel is marked with a green crosshair and resultant correlations are overlaid on the anatomical image. (A)–(F) Connectivity between ventral horns for subjects 1, 3, 8, 10, 11, and 13, respectively. (G)–(J) Connectivity between dorsal horns for subjects 5, 16, 18, and 22, respectively. (K and L) Less common correlations within gray matter. In (K) (subject 20), focal connectivity between ventral horns and with central gray matter. In (L) (subject 7), connectivity between ventral horns but also with the contralateral dorsal horn. At the single-subject level, there is some evidence for functional connectivity between ventral and dorsal horns, but such correlations are less common across slices and not statistically significant at the group level.
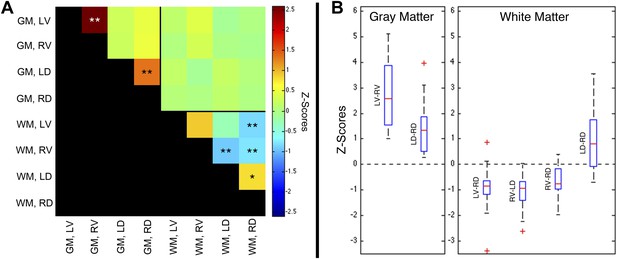
Group-level functional connectivity between sub-regions of spinal gray matter (GM) and surrounding white matter (WM) within slices.
(A) In GM, strong positive correlations are observed between left (LV) and right (RV) ventral horns, as well as left (LD) and right (RD) dorsal horns (*p<0.05; **p<0.01; Bonferroni corrected). Weaker positive and negative correlations are observed within WM. No statistically significant correlations are observed between spinal GM and WM (upper right quadrant). (B) Box-and-whisker plots showing the median and upper and lower quartiles of the six statistically significant results identified in (A). Whiskers extend out to 1.5 times the distance between the upper and lower quartiles, and an outlier (beyond the whiskers) is denoted by a plus. Wilcoxon signed rank tests identify the distributions of z-scores (across all slices and subjects) that are significantly different from zero (p < 0.05). Functional connectivity between WM sub-regions is more variable and exhibits both positive and negative median correlations. In comparison, median GM correlations between LV-RV and LD-RD are positive across all 22 subjects. Additional analysis permutations (described in Figure 5—figure supplements 1, 3, and 5 with example GM power spectra shown in Figure 5—figure supplements 2, 4, and 6) reveal that the three negative WM correlations are influenced by WM regression (step #12) and thus are open to more than one interpretation. However, supplementary analyses reveal that the positive GM correlations between ventral horns and between dorsal horns persist across all preprocessing permutations. These additional analyses further support the conclusion that positive correlations between GM horns are not artifactual—possibly created by preprocessing choices or frequency bandwidth selection—and most likely represent genuine functional connectivity.
-
Figure 5—source data 1
Matlab file containing the raw data used to generate Figure 5.
The dimensions of this matrix are 8 × 8 × 22 where the 8 × 8 represents all possible comparisons between the eight sub-regions (Figure 5A) and the upper-triangular entries are non-zero. For each of the 28 unique comparisons, the 22 entries in the third dimension correspond to the median measurement of functional connectivity across all 12 slices for each of the 22 subjects.
- https://doi.org/10.7554/eLife.02812.008
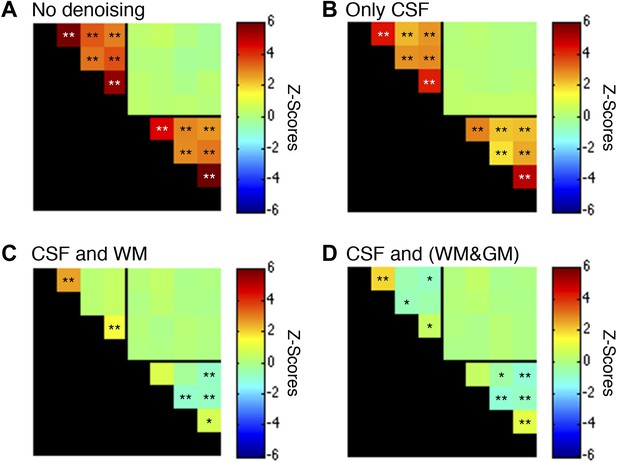
Functional connectivity matrices resulting from preprocessing pipeline permutations.
As before, functional data were band-pass filtered between 0.01 and 0.08 Hz (*p<0.05; **p<0.01; Bonferroni corrected). For clarity the labels are not shown for each column/row but are the same as in Figure 5. (A) Preprocessing was performed as described in the Methods except CSF and WM regressors (steps #11 and #12) were not applied. Each GM sub-region is highly correlated with all other GM sub-regions and similarly each WM sub-region is highly correlated with all other WM sub-regions. Interestingly, there are no significant group-level correlations between GM and WM sub-regions, suggesting that ‘global’ GM fluctuations tend to be constrained to GM and ‘global’ WM fluctuations tend to be constrained to WM. (B) Preprocessing was performed as described in ‘Materials and methods’ except a WM regressor (step #12) was not applied. The application of only CSF regressors reduced correlations within both GM and WM, but all inter-region correlations remained and were statistically significant. (C) Preprocessing was performed exactly as described in ‘Materials and methods’ (i.e., this panel is the same as Figure 5A except for a larger dynamic range for z-scores). Regression of the principal eigenvector of all time series in the WM mask significantly altered correlations within both GM and WM. This unintuitive result may be explained if WM masks contain signal contributions from adjacent GM voxels, which is certainly possible given the small size of the GM butterfly and unavoidable partial volume effects, and sub-millimeter functional-to-anatomical warping inaccuracies caused by magnetic field inhomogeneities. (D) To investigate the possibility that WM masks contain fractions of GM voxels, and the impact of different regression masks on correlations between sub-regions, preprocessing was performed as described in ‘Materials and methods’ except step #12 extracted the principal eigenvector of all time series within a combined WM and GM mask. Given the small size of each GM mask relative to its neighboring WM mask, the principal eigenvector from the combined mask (WM&GM) should be similar to only using WM. Indeed, regression with these modified eigenvectors produces similar group-level correlations between WM sub-regions and introduce weak negative correlations between GM sub-regions. In GM, positive correlations between ventral horns and between dorsal horns still persist after this more invasive regressor, providing further evidence that these strong temporally correlated fluctuations are unlikely to be caused by physiological motion or spatially-correlated noise. To further investigate the spatial extent of global fluctuations within GM, we repeated the preprocessing as described in ‘Materials and methods’ but eroded the WM mask slightly before extracting the principal eigenvector. The results of this tertiary analysis (not shown) were slightly different but statistically similar to Figure 5A, demonstrating that voxels on and near the GM-WM boundary in fact characterize physiological fluctuations from both tissue types due to unavoidable partial volume effects and sub-millimeter warping inaccuracies. Overall, these supplementary analyses suggest that the preprocessing performed in the main manuscript (regression of the principal eigenvector from WM masks without erosion) is an appropriate strategy for suppressing extraneous fluctuations within both WM and GM without introducing substantial negative correlations between GM sub-regions. Finally, power spectra for WM and GM sub-regions in (C) are presented in Figure 5—figure supplement 2.
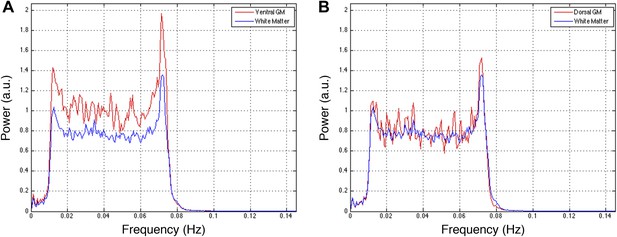
Power spectra across gray and white matter sub-regions for data filtered between 0.01 and 0.08 Hz.
Power spectra for (A) ventral and (B) dorsal GM sub-regions in Figure 5—figure supplement 1C that exhibit significant positive correlations (z > 1.65; one-tailed), and all WM sub-regions. For each frequency the plotted power represents median power across slices and subjects. Within a range from 0.015 Hz to 0.075 Hz, ventral GM exhibits 32% more power than WM whereas dorsal GM exhibits only 5% more power. This difference in power between GM sub-regions may be explained through the preprocessing pipeline permutations presented in Figure 5—figure supplement 1. Figure 5—figure supplement 1A,B show comparable z-scores between ventral and dorsal GM horns, and this difference does not appear until after WM regression (Figure 5—figure supplement 1C) or WM&GM regression (Figure 5—figure supplement 1D). Therefore, regression with this final eigenvector suppresses a larger contribution of signal fluctuations (and thus power) from dorsal GM than ventral GM, which may be attributed to the small size of the dorsal horns and partial volume averaging effects (because the WM mask is not eroded before PCA), as well as to unavoidable sub-millimeter registration inaccuracies. The large peak at ∼0.75 Hz is likely due to physiological noise, so it could be argued that this noise power significantly affects the measurements of functional connectivity. To investigate this further and discount the possibility that our results are driven by physiological noise around 0.75 Hz, the analyses in Figure 5—figure supplement 1 are repeated after data are filtered with a narrower band-pass filter between 0.01 Hz and 0.07 Hz. These results are presented in Figure 5—figure supplement 3.
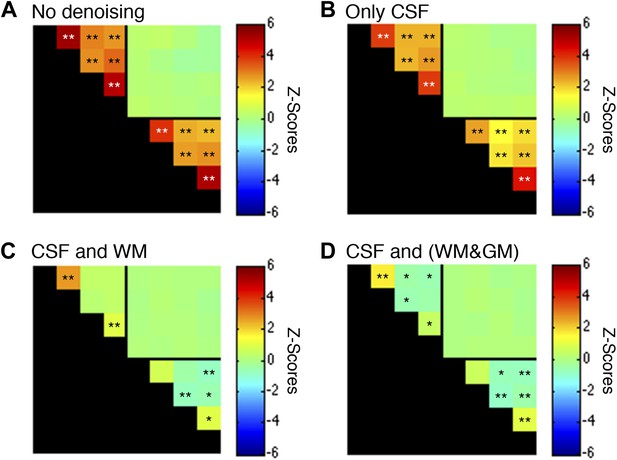
Functional connectivity matrices resulting from preprocessing pipeline permutations after band-pass filtering between 0.01 and 0.07 Hz.
Functional connectivity matrices resulting from preprocessing pipeline permutations after band-pass filtering between 0.01 and 0.07 Hz to suppress power from likely physiological noise at ∼0.75 Hz (*p<0.05; **p<0.01; Bonferroni corrected). For clarity the labels are not shown for each column/row but are the same as in Figure 5. (A) Preprocessing was performed as described in the Methods except CSF and WM regressors (steps #11 and #12) were not applied, and step #13 used a different frequency bandwidth. (B) Preprocessing was performed as described in ‘Materials and methods’ except a WM regressor (step #12) was not applied and step #13 used a different frequency bandwidth. (C) Preprocessing was performed as described in ‘Materials and methods’ except step #13 used a different frequency bandwidth. (D) Preprocessing was performed as described in ‘Materials and methods’ except step #12 extracted the principal eigenvector of all time series within a combined WM and GM mask and step #13 used a different frequency bandwidth. Only a couple of minor changes are apparent between these matrices and those presented in Figure 5—figure supplement 1, suggesting that a slight decrease in the upper filter range from 0.08 Hz to 0.07 Hz to further suppress physiological noise does not appear to have a significant impact on the group-level connectivity analyses. Power spectra for WM and GM sub-regions in (C) are presented in Figure 5—figure supplement 4.
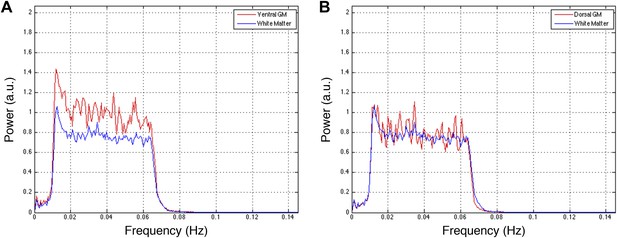
Power spectra across gray and white matter sub-regions for data filtered between 0.01 and 0.07 Hz.
Power spectra for (A) ventral and (B) dorsal GM sub-regions in Figure 5—figure supplement 3C that exhibit significant positive correlations in the original analysis (Figure 5A; z > 1.65; one-tailed), and all WM sub-regions. For each frequency the plotted power represents median power across slices and subjects. Within a range from 0.015 Hz to 0.065 Hz, ventral GM similarly exhibits 31% more power than WM whereas dorsal GM exhibits 5% more power. The narrower bandwidth completely suppresses the noise peak at ∼0.75 Hz but the results from functional connectivity analyses are almost unchanged (Figure 5—figure supplement 1 vs Figure 5—figure supplement 3), confirming that this peak does not significantly affect the overall results. Our original decision to filter resting state spinal cord data between 0.01 Hz and 0.08 Hz (step #13) was motivated by the approach most commonly used for resting state analyses in the brain (filtering between 0.01 Hz and 0.08–0.1 Hz), although it is not yet clear if this frequency range is optimal for resting state spinal cord analyses. To investigate the possibility that frequencies above 0.08 Hz may contribute to inherent functional connectivity, the analyses in Figure 5—figure supplement 1 are once again repeated after data are filtered with a wider band-pass filter between 0.01 Hz and 0.13 Hz. These results are presented in Figure 5—figure supplement 5.
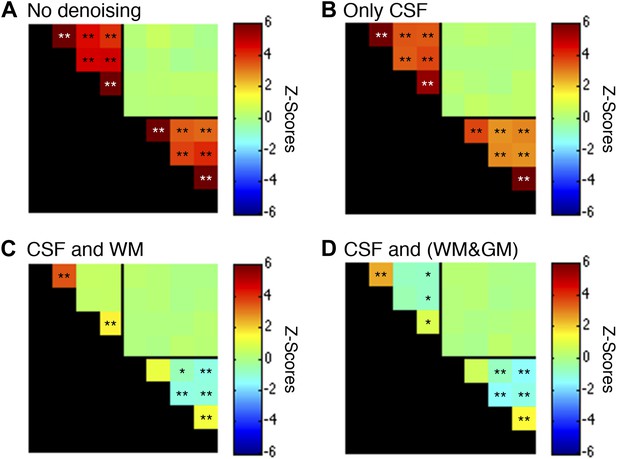
Functional connectivity matrices resulting from preprocessing pipeline permutations after band-pass filtering between 0.01 and 0.13 Hz.
Functional connectivity matrices resulting from preprocessing pipeline permutations after band-pass filtering between 0.01 and 0.13 Hz (*p<0.05; **p<0.01; Bonferroni corrected). For clarity the labels are not shown for each column/row but are the same as in Figure 5. (A) Preprocessing was performed as described in the Methods except CSF and WM regressors (steps #11 and #12) were not applied, and step #13 used a different frequency bandwidth. (B) Preprocessing was performed as described in ‘Materials and methods’ except a WM regressor (step #12) was not applied and step #13 used a different frequency bandwidth. (C) Preprocessing was performed as described in ‘Materials and methods’ except step #13 used a different frequency bandwidth. (D) Preprocessing was performed as described in ‘Materials and methods’ except step #12 extracted the principal eigenvector of all time series within a combined WM and GM mask and step #13 used a different frequency bandwidth. The inclusion of frequencies between 0.08 Hz and 0.13 Hz primarily strengthens GM correlations between ventral horns (relative to the results obtained using only frequencies between 0.01 Hz and 0.08 Hz) but also increases the statistical significance of WM correlations between LD and RD. Power spectra for WM and GM sub-regions in (C) are presented in Figure 5—figure supplement 6.
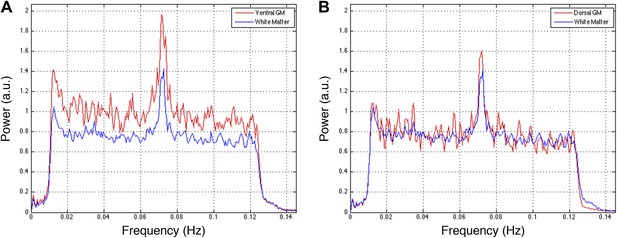
Power spectra across gray and white matter sub-regions for data filtered between 0.01 and 0.13 Hz.
Power spectra for (A) ventral and (B) dorsal GM sub-regions in Figure 5—figure supplement 5C that exhibit significant positive correlations in the original analysis (Figure 5A; z > 1.65; one-tailed), and all WM sub-regions. For each frequency the plotted power represents median power across slices and subjects. Within the range of higher frequencies between 0.075 Hz and 0.125 Hz, ventral GM exhibits 28% more power than WM whereas dorsal GM and WM exhibit comparable total power (< 0.3% difference). These data show that the original filter bandwidth between 0.01 Hz and 0.08 Hz was a sound choice, but also suggest that frequencies slightly above 0.08 Hz may contain additional power related to GM connectivity. Future work will acquire resting state spinal cord data with a faster sampling rate to better understand the relative frequency-dependent contributions from BOLD signal fluctuations and physiological noise.