Introducing µGUIDE for quantitative imaging via generalized uncertainty-driven inference using deep learning
Figures
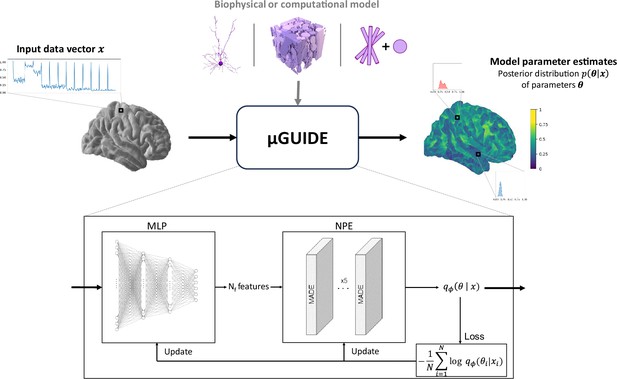
µGUIDE framework.
µGUIDE takes as input an observed data vector and relies on the definition of a biophysical or computational model (Ascoli et al., 2007; Callaghan et al., 2020; Jelescu et al., 2020). It outputs a posterior distribution of the model parameters. Based on a Simulation-Based Inference (SBI) framework, it combines a Multi-Layer Perceptron (MLP) with three layers and a Neural Posterior Estimator (NPE). The MLP learns a low-dimensional representation of , based on a small number of features (), that can be either defined a priori or determined empirically during training. The MLP is trained simultaneously with the NPE, leading to the extraction of the optimal features that minimize the bias and uncertainty of .
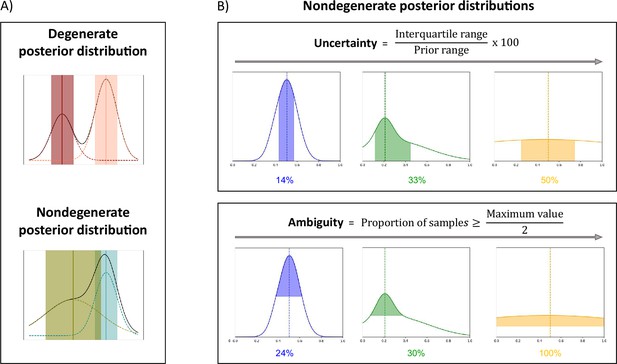
µGUIDE summarizes information contained in the estimated posterior distributions.
(A) Examples of degenerate and non-degenerate posterior distributions. Two Gaussian distributions are fitted to the obtained posterior distribution, where the means and standard deviations are represented by the vertical lines and shaded areas. A voxel is considered as degenerate if the derivative of the fitted Gaussian distributions changes signs more than once (i.e. multiple local maxima), and if the two Gaussian distributions are not overlapping (the distance between the two Gaussian means is inferior to the sum of their standard deviations). (B) Presentation of the measures introduced to quantify a posterior distribution on exemplar non-degenerate posterior distributions. Maximum A Posteriori (MAP) is the most likely parameter estimate (dashed vertical lines). Uncertainty measures the dispersion of the 50% most probable samples using the interquartile range, with respect to the prior range. Ambiguity measures the Full Width at Half Maximum (FWHM), in percentage with respect to the prior range.
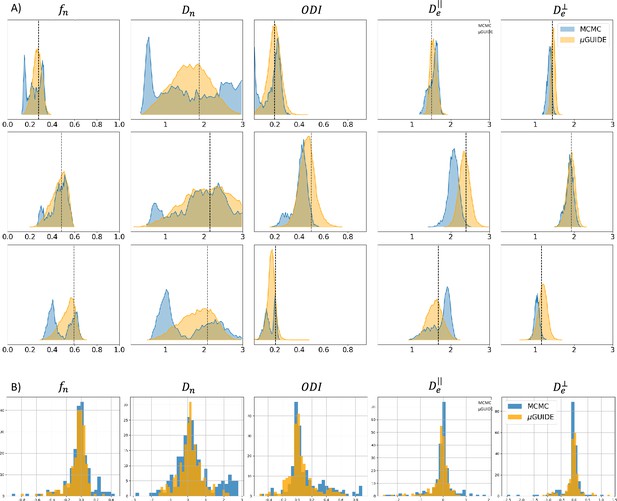
Comparison between µGUIDE and Markov-Chain-Monte-Carlo (MCMC).
(A) Posterior distributions obtained using either µGUIDE or MCMC on three exemplar simulations with Model 2 (SM − ). Names of the model parameters are indicated in the titles of the panels. (B) Bias between the ground truth values used for simulating the diffusion signals, and the Maximum A Posteriori extracted from the posterior distributions using either µGUIDE or MCMC. Sharper and less biased posterior distributions are obtained using µGUIDE.
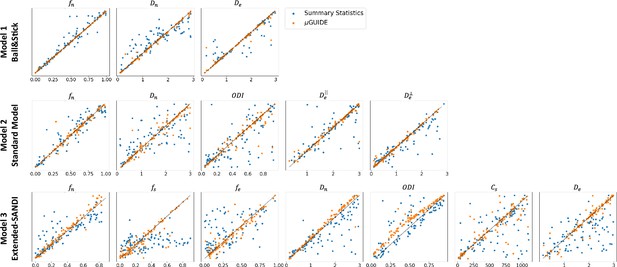
Fitting accuracy comparison between µGUIDE’s Multi-Layer Perceptron (MLP)-extracted features and manually defined summary statistics.
Maximum A Posterioris (MAPs) extracted from the posterior distributions versus ground truth parameters used for generating the signal for the three models. Orange points correspond to the MAPs obtained using MLP-extracted features (µGUIDE) and the blue ones to the MAPs with the manually defined summary statistics. Only the non-degenerate posterior distributions were kept. The summary statistics used in those three models are the direction-averaged signal for the Ball&Stick model, the LEMONADE system of equations (Novikov et al., 2018) for the Standard Model (SM), and the summary statistics defined in Jallais et al., 2022 for the extended-SANDI model. Results are shown on 100 exemplar noise-free simulations with random parameter combinations. The optimal features extracted by the MLP allow to reduce the bias and variance of the obtained microstructure posterior distributions.
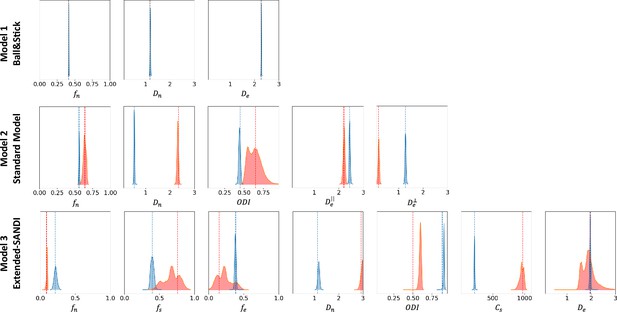
Exemplar posterior distributions of the microstructure parameters for the Ball&Stick, Standard Model (SM), and extended-SANDI models, obtained using µGUIDE on exemplar noise-free simulations.
As the complexity of the model increases, degeneracies appear (red posterior distributions). µGUIDE allows to highlight those degeneracies present in the model definition.
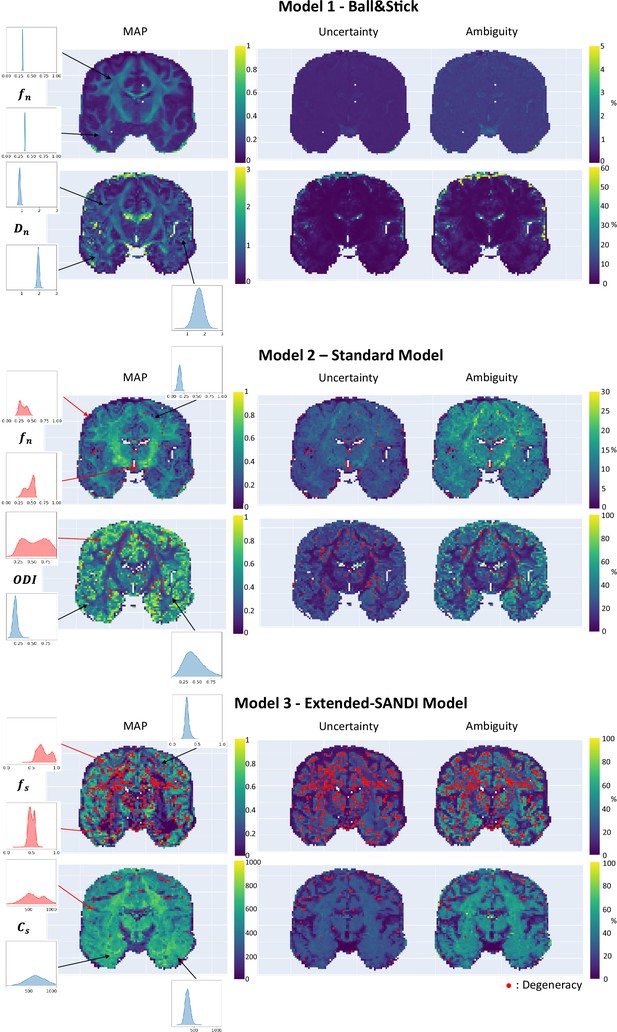
Parametric maps of the Ball&Stick (top), SM (middle) and extended-SANDI model (bottom), obtained using µGUIDE.
Maximum A Posteriori (MAP), uncertainty and ambiguity measure maps are reported, overlayed with voxels considered degenerate (red dots).
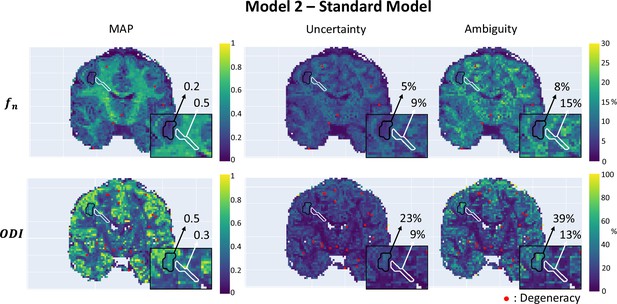
Parametric maps of a participant with epilepsy obtained using µGUIDE with the Standard Model (SM), superimposed with the grey matter (black) and white matter (white) lesions segmentation.
Mean values of the Maximum A Posterior (MAP), uncertainty, and ambiguity measures are reported in the two regions of interest. Lower MAP values are obtained in the lesions for the axonal signal fraction and the orientation dispersion index compared to healthy tissue. Higher uncertainty and ambiguity ODI values are reported, suggesting less stable estimations.
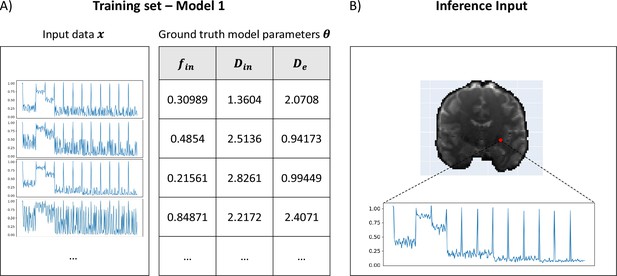
Example training set and input signals for µGUIDE.
(A) Examples of input synthetic data vectors and corresponding ground truth model parameters used in the training set of Model 1 (Ball&Stick). (B) Example of input measured signals from a voxel in a healthy participant, used for inference.
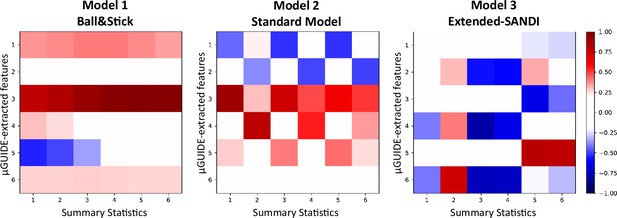
Correlation matrices between features extracted by the Multi-Layer Perceptron (MLP) in µGUIDE and manually defined summary features for the three models.
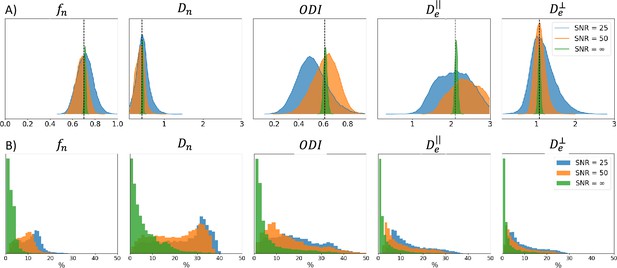
SNR uncertainty comparison between signals with different noise levels: no noise, , and using Model 2.
(A) Posterior distributions obtained on one example parameter combination (vertical black dashed line) with the three noise levels. (B) Histogram of the uncertainty obtained for 1000 signals with different noise levels (in %). Similar ground truths are used for each noise level.
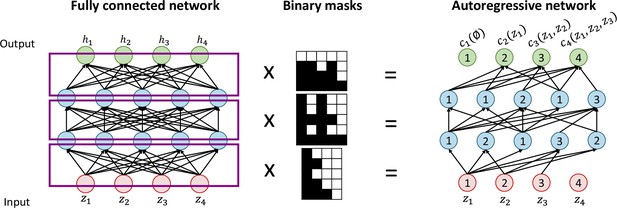
Schematic of Masked Autoencoder for Distribution Estimation (MADE) autoregressive network construction.
Tables
Number of degenerate cases per parameter on 10,000 noise-free simulations.
Training and estimations of the posterior distributions were performed on CPU. Time for training each model and time for estimating posterior distributions of 10,000 noise-free simulations, define if they are degenerate or not, and extract the Maximum A Posteriori (MAP), uncertainty, and ambiguity are also reported.
Model (SNR = ∞) | Training time (CPU) | Fitting time (on 10,000 simulations) | Number of degeneracies (on 10,000 simulations) | |||||||
---|---|---|---|---|---|---|---|---|---|---|
Model 1: Ball&Stick | 11 min | 96 s | 0 | 0 | 0 | - | - | - | - | - |
Model 2: Standard Model | 2h02 | 135 s | 4 | 34 | 23 | 3 | 8 | - | - | - |
Model 3: extended-SANDI model | 2h02 | 1412 s | 205 | 4 | 260 | 57 | - | 1395 | 2571 | 1011 |
Number of degenerate cases per parameter on 10,000 noisy simulations (Rician noise with SNR = 50).
Training and estimations of the posterior distributions were performed using a GPU. Time for training each model and time for estimating posterior distributions of 10,000 noisy simulations, define if they are degenerate or not, and extract the Maximum A Posteriori (MAP), uncertainty, and ambiguity are also reported.
Model (SNR = 50) | Training time (CPU) | Fitting time (on 10,000 simulations) | Number of degeneracies (on 10,000 simulations) | |||||||
---|---|---|---|---|---|---|---|---|---|---|
Model 1: Ball&Stick | 26 min | 79 s | 0 | 0 | 0 | - | - | - | - | - |
Model 2: Standard Model | 42 min | 82 s | 75 | 71 | 117 | 109 | 29 | - | - | - |
Model 3: Extended-SANDI model | 50 min | 238 s | 47 | 24 | 784 | 6 | - | 828 | 1047 | 56 |