Decoding m6Am by simultaneous transcription-start mapping and methylation quantification
Figures
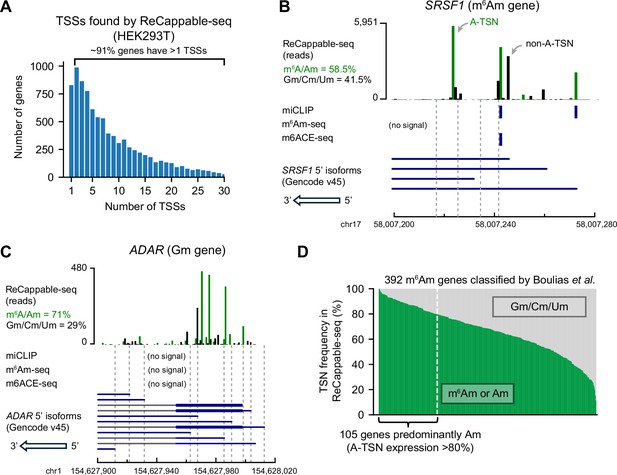
Many m6Am genes are mistakenly annotated.
(A) Genes tend to have multiple TSSs. Shown is a histogram displaying the number of TSSs per protein-coding gene in HEK293T cells. TSSs (87,624 TSSs from 9199 genes) were mapped using ReCappable-seq. These TSSs have expression levels ≥1 TPM (transcription-start nucleotide per million). (B, C) Examples of genes that were mistakenly classified by previous studies (Boulias et al., 2019). SRSF1 (B) was previously designated as m6Am because of the miCLIP signals overlapping with A-TSSs. However, based on ReCappable-seq, ~41.5% of the reads are mapped to non-A-TSSs in SRSF1. Notably, one of the most expressed m6Am A-TSS (chr17:58,007,228) was mistakenly considered as internal m6A because this position was not previously annotated as a TSS (Linder et al., 2015). ADAR (C) was previously classified as Gm. There is no m6Am signal based on miCLIP (Boulias et al., 2019), m6Am-seq (Sun et al., 2021), or m6ACE-seq (Koh et al., 2019) mapped to ADAR. However, ~71% of the transcripts from ADAR are A-initiated. (D) Previously classified m6Am genes express considerable levels of non-A-initiated transcripts. Each column represents a gene previously classified as m6Am gene by miCLIP (Boulias et al., 2019). For each gene, the percentage of transcript isoforms starting with m6Am/Am (in green) or Gm/Cm/Um (in gray) are shown. The percentage was calculated by weighting each transcript isoform by its expression level. The TSN frequencies were obtained using ReCappable-seq in HEK293T cells.
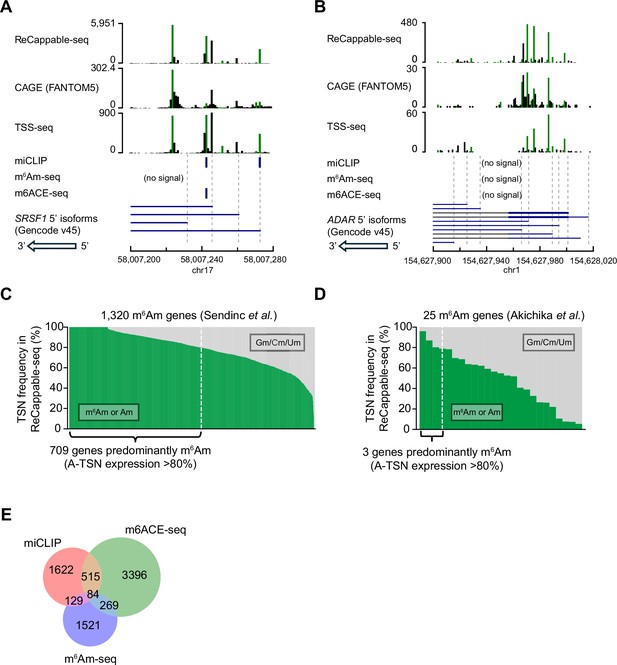
Corresponding to Figure 1.
(A, B) Corresponding to Figure 1B and C, the heterogeneity of TSS usage in SRSF1 and ADAR can also be observed in TSS mapping data generated by CAGE and TSS-seq. In these plots, HEK293T CAGE data were downloaded from FANTOM5 Noguchi et al., 2017; HEK293T TSS-seq data were generated in our previous study (Despic and Jaffrey, 2023). (C, D) Similar to Figure 1B, shown are the m6Am genes classified by (C) (Sendinc et al., 2019) and (D) (Akichika et al., 2019). For each gene, the percentage of transcripts starting with m6Am/Am (in green) or Gm/Cm/Um (in gray) are shown. The transcription-start nucleotide frequencies are obtained by ReCappable-seq data of HEK293T cells. For (D), the 25 m6Am genes are collected from Table S3 ‘List of genes whose TEs are up- or down-regulated upon CAPAM KO’ from Akichika et al., 2019. (E) Venn diagram showing the inconsistency between m6Am maps generated by the existing m6Am mapping methods. In this analysis, only m6Am sites mapped to the primary chromosome are used. Source of data: miCLIP, Boulias et al., 2019; m6Am-seq, Sun et al., 2021; m6ACE-seq, (Koh et al., 2019).
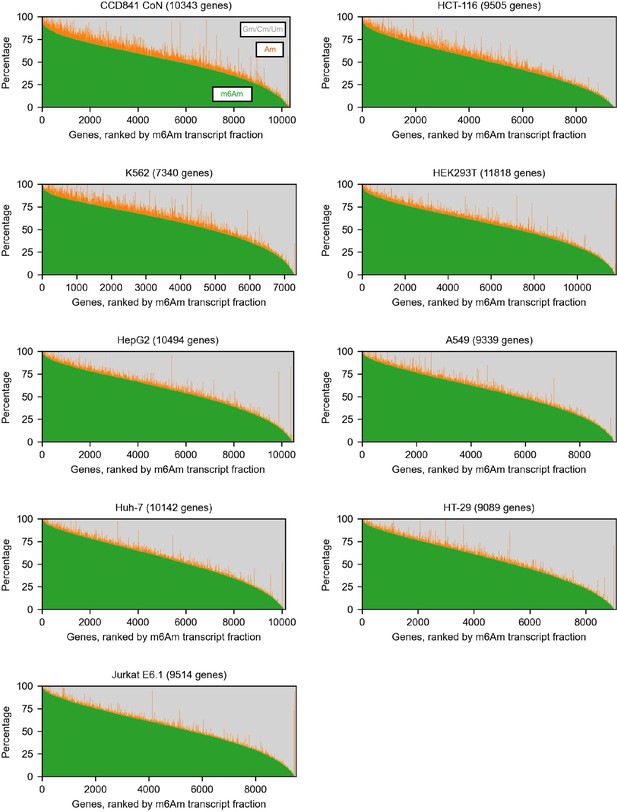
Corresponding to Figure 1.
Similar to Figure 1D, the fractions of transcripts starting with m6Am, Am, and other bases in different cell lines. This estimation is based on CROWN-seq. Only genes with at least 50 mapped reads were shown.
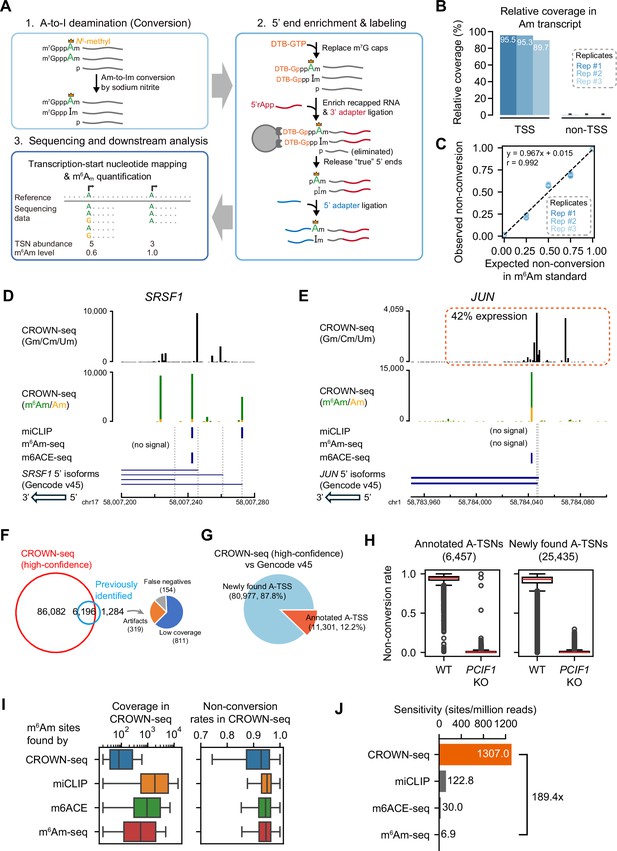
CROWN-seq correctly maps and quantifies m6Am.
(A) Schematic of CROWN-seq. RNAs are firstly treated with sodium nitrite, which causes Am at the transcription-start position to be converted to Im. To isolate the TSN, m7G caps are replaced with 3’-desthiobiotin (DTB) caps. These DTB caps are enriched on streptavidin beads, while uncapped background RNA fragments are uncapped and washed away. After washing, an enriched pool of transcript 5’ ends is released from the beads by cleaving the triphosphate bridge, leaving 5’ monophosphate ends that are ligated to an adapter. After adapter ligation, cDNA was synthesized and amplified for Illumina sequencing. During sequencing, the converted sequences were aligned to a reference genome. The TSNs can be determined as the first base immediately after the 5’ adapter sequence. To quantify m6Am stoichiometry, we count the number of A (m6Am) and G (Am) bases at the TSN position. (B) CROWN-seq enriches reads that contain the TSN. The relative coverage of reads mapped to the TSS and non-TSS regions across the m7G-ppp-Am-initiated RNA standard was calculated. The average relative coverage of reads that map to the TSS and to non-TSS positions are shown for three technical replicates. The 95% CI of the relative coverages is shown using error bars. (C) CROWN-seq exhibits high quantitative accuracy for measuring m6Am stoichiometry. RNA standards were prepared with 0%, 25%, 50%, 75%, and 100% m6Am stoichiometry. To make m6Am standards in different m6Am levels, we generated both Am transcripts and m6Am transcripts by in vitro transcription with cap analogs m7G-ppp-Am and m7G-ppp-m6Am. Five transcripts were made in the Am and m6Am form and mixed to achieve the indicated m6Am stoichiometry. These transcripts have identical 5’ ends and different barcodes. Linear least-squares regression was performed to calculate the correlation between expected non-conversion rates and the observed average non-conversion rates for each standard. All TSNs shown in this plot have high sequencing coverage, ranging from 656 to 21,545 reads. (D) CROWN-seq results for SRSF1. CROWN-seq shows that 54.0% of SRSF1 transcripts initiate with A. Among the A-initiated transcripts, 93.4% were resistant to conversion (A’s, shown in green), and therefore m6Am. As a result, SRSF1 has 50.4% m6Am transcripts, 3.6% Am transcripts, and 46.0% non-A-initiated transcripts. Notably, a previous miCLIP study identified an internal m6A site (Linder et al., 2015) which we found was m6Am at the TSN based on CROWN-seq. (E) CROWN-seq results for JUN. CROWN-seq shows that ~58% of JUN transcripts initiate with A. Unlike SRSF1 which A-TSNs are highly methylated, JUN A-TSNs are only ~75% methylated. As a result, JUN has 43.5% m6Am transcripts, 14.5% Am transcripts, and 42% non-A-initiated transcripts. (F) CROWN-seq identifies most m6Am sites identified in previous studies. 7480 m6Am sites in HEK293T cells found either by miCLIP (Boulias et al., 2019), m6Am-seq (Sun et al., 2021), or m6ACE-seq (Koh et al., 2019) were analyzed. The high-confidence sites in CROWN-seq were defined as A-TSN with ≥20 unique mapped reads. The results shown are from HEK293T cells, which is the same cell line used in all previous studies. Among the 1,284 sites uniquely found in other studies, 811 sites are also mapped by CROWN-seq but at lower coverage (1–19 reads); 319 sites are mapped very far (>100 nt) away from any TSS annotation and thus can be considered as false positives; the remaining 154 sites are mapped very closely to known TSSs and may be false negative results in CROWN-seq. (G) Many A-TSNs identified in CROWN-seq in HEK293T cells are not annotated. In this analysis, A-TSSs in (F) were intersected with the TSS annotation in Gencode v45. Only 12.2% of A-TSSs found by CROWN-seq are previously annotated. (H) CROWN-seq exhibits high accuracy in TSN discovery. In this analysis, we compared the non-conversion of A-TSNs between wild-type and PCIF1 knockout cells. For the 6,457 A-TSNs annotated by Gencode v45, most of them have high non-conversion rates in wild-type cells and very low non-conversion rates in PCIF1 knockout cells, indicating correct TSN mapping. Similar to the annotated TSNs, 25,435 newly found A-TSNs were also found to have differential m6Am between wild-type and PCIF1 knockout. Thus, these newly found A-TSNs were also mostly true positives. In this analysis, only A-TSNs mapped by at least 20 reads in both wild-type and PCIF1 knockout HEK293T cells were used. (I) The previously identified m6Am sites are biasedly in higher expression and higher m6Am stoichiometry. Shown are the sequencing coverage (left) and non-conversion rates (right) of different sets of m6Am sites in HEK293T CROWN-seq data. In total, 98,147 sites found by CROWN-seq, 2129 sites found by miCLIP (Boulias et al., 2019), 3693 sites found by m6ACE-seq (Koh et al., 2019), and 1610 sites found by m6Am-seq (Sun et al., 2021) are shown. (J) CROWN-seq has much higher sensitivity in m6Am discovery than all existing m6Am mapping methods. In this analysis, sensitivity is defined as m6Am/A-TSN found per million mapped reads. For CROWN-seq, sensitivity was defined as the slope of linear regression result between sequencing depth and A-TSN number among different samples in this study (see Figure 2—figure supplement 1G). For other methods, sensitivity was defined as the number of reported m6Am sites over the number of reads in all libraries required for m6Am identification.
-
Figure 2—source data 1
A comparison of m6Am mapping methods.
- https://cdn.elifesciences.org/articles/104139/elife-104139-fig2-data1-v1.xlsx
-
Figure 2—source data 2
The design of m6Am standards.
- https://cdn.elifesciences.org/articles/104139/elife-104139-fig2-data2-v1.xlsx
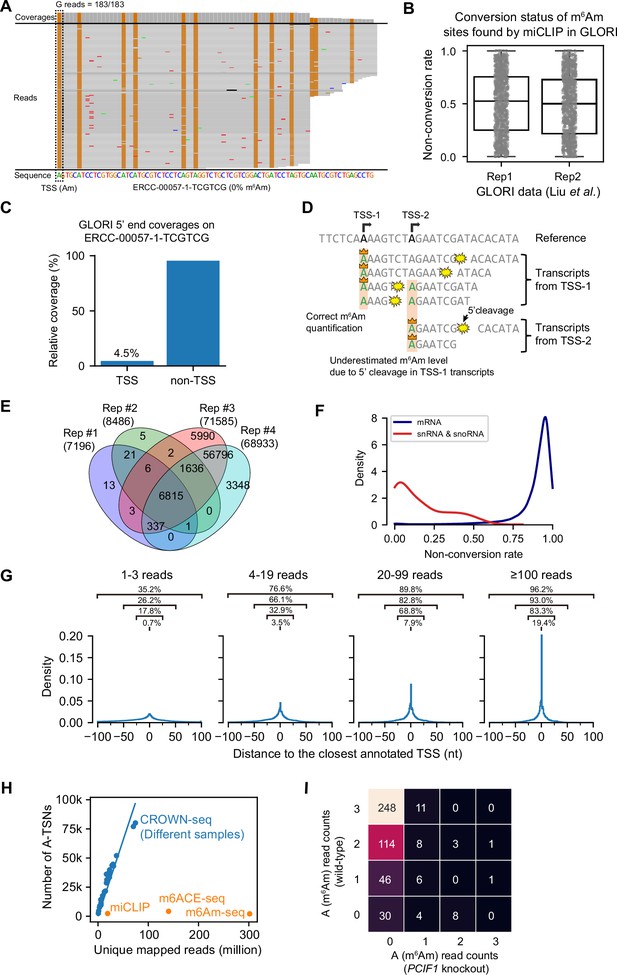
Corresponding to Figure 2.
(A) GLORI can completely convert 5’ end Am in a transcript synthesized by in vitro transcription. Shown is the IGV plot demonstrating the coverage and individual reads mapped to the TSS of ERCC-00057–1-TCGTCG transcript. The reference sequence is also shown at the bottom. In this plot, the bases that match the reference are shown in gray, while G mismatches are shown in brown. The A-to-G mutation in the TSS position indicates successful A-to-I conversion during sodium nitrite treatment. In this assay, the 5’ Am in ERCC-00057–1-TCGTCG transcript was made by using m7GpppAmG analog during in vitro transcription (see Materials and methods). The ERCC-00057–1-TCGTCG transcript was decapped and spiked into the RNA sample for GLORI experiment. (B) GLORI underestimated m6Am stoichiometry in m6Am sites found by miCLIP. In this analysis, we reanalyzed the reads in HEK293T GLORI libraries (GSE210563) generated by Liu et al., 2023. To obtain the non-conversion rate of m6Am sites, we extracted the 5’ ends mapped to miCLIP sites found by Boulias et al., 2019 (GSE122948). 1084 and 1067 m6Am sites were analyzed in each replicate, respectively. (C) Only a few reads in GLORI can mapped to the desired TSS. In this analysis, we analyzed the GLORI mapping result of ERCC-00057–1-TCGTCG transcript in (A). We calculated the relative ratio of reads whose 5’ end mapped to not failed to map to the desired TSS of the ERCC-00057–1-TCGTCG. Only ~4.5% of the reads can be correctly mapped to the TSS. (D) A schema showing the reason why m6Am stoichiometry is incorrectly estimated in the transcriptome. In transcriptome, a gene can have multiple TSSs, whose 5’ ends can overlap with each other. As a result, the A-TSN from TSS-2 can also be transcribed as an internal A base in transcripts generated by TSS-1. During the GLORI experiment, 5’ cleavage happens very frequently, which results in massive 5’ ends from internal A’s. Since the GLORI library is unable to distinguish between the ‘true’ 5’ ends with m7G cap and the ‘false’ 5’ ends with monophosphate, both ‘true’ 5’ ends and ‘false’ 5’ ends are counted for TSS-2. Because most of the internal A’s are not N6-methylated, the ‘false’ 5’ ends will dilute the m6Am signal from the ‘true’ 5’ ends and result in underestimated m6Am stoichiometry. (E) CROWN-seq shows high reproducibility in m6Am identification. In the Venn diagram, four replicates of HEK293T CROWN-seq results were compared. Among the replicates, replicates #1 and #2 are two technical replicates of the same biological sample; while replicates #3 and #4 are the two technical replicates of another biological sample. In this analysis, A-TSNs with at least 20 reads mapped were included. Since replicate #1 and #2 have lower sequencing depth (~3 million reads each) than replicate #3 and #4 (~70 million reads each), the numbers of A-TSNs reported by replicate #1 and #2 are much lower than that by replicate #3 and #4. (F) Kernel density estimate (KDE) plot for the distribution of non-conversion rates of A-TSNs belonging to mRNA and snRNA/snoRNA reported by CROWN-seq. In this analysis, all sequencing results from HEK293T replicates were merged. Only A-TSNs with at least 20 reads were analyzed. (G) The distance between CROWN-seq identified TSSs and annotated TSSs in Gencode v45. In this plot, the TSSs identified in CROWN-seq replicate #3 were analyzed. 2,054,368, 333,959, 120,378, and 63,849 TSSs with 1–3, 3–19, 19–99, and ≥100 mapped reads were shown respectively. The percentage of TSSs well overlapped with annotation, as well as TSS located within the [–25, 25], [–50, 50], and [–100, 100] regions proximal to the annotated TSSs were also indicated. (H) Corresponding to Figure 2J, shown are the number of uniquely mapped reads (X-axis) and the number of called m6Am or A-TSN in different methods. For CROWN-seq, all libraries used in this study are shown in dots. We performed linear regression (shown in line) to calculate the sensitivity of CROWN-seq (i.e. the slope of linear regression) which is shown in Figure 2J. (I) CROWN-seq exhibited high TSS mapping accuracy in low-coverage sites. In this heatmap, each block represents A-TSNs with a certain number of A reads (i.e. m6Am reads) mapped in wild-type (Y-axis) and PCIF1 knockout (X-axis) HEK293T. Only A-TSNs have 3 reads mapped in wild-type and 3–39 mapped in PCIF1 knockout are shown. For example, shown in the upper left corner, there are 248 A-TSNs that have 3 A reads in wild-type (i.e. 100% non-conversion) and 0 A reads (i.e. 0% non-conversion) in PCIF1 knockout cells.
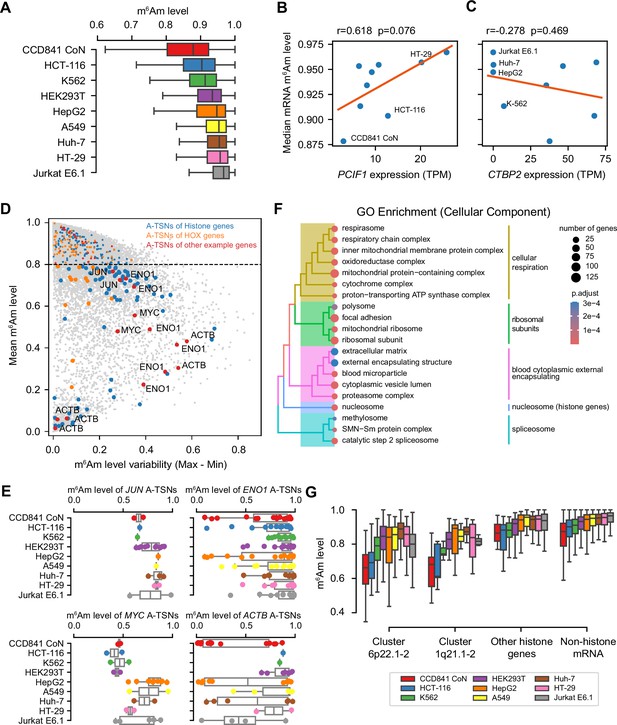
CROWN-seq reveals m6Am landscape in mRNA.
(A) Boxplot showing the overall mRNA m6Am levels (i.e., m6Am stoichiometry) among different cell lines. Only m6Am sites with ≥50 reads mapped in at least one cell line were analyzed. (B) mRNA m6Am stoichiometry is positively correlated with PCIF1 expression. In this plot, PCIF1 expression was estimated by the number of reads mapped to PCIF1 TSSs. The read counts were normalized into transcription-start nucleotide per million (TPM) for gene expression comparison. Three cell lines (CCD841 CoN, HCT-116, and HT-29) whose PCIF1 expression was estimated by western blots and RT-qPCR by Wang et al., 2023b are highlighted. Pearson’s r and p-value in this analysis were obtained by linear regression. (C) Overall mRNA m6Am stoichiometry is not correlated with CTBP2 expression. Similar to (B), CTBP2 expression was estimated by CROWN-seq. Four cell lines with very low CTBP2 expression are highlighted. (D) Some A-TSNs have relatively low and more variable m6Am stoichiometry among cell lines. In this plot, the variability of the m6Am stoichiometry of a site, which is defined as the maximum m6Am subtracted by the minimum m6Am stoichiometry among all cell lines is shown on the X-axis; the average m6Am level of a site among all cell lines is shown on the Y-axis. Several example genes are indicated in different colors. (E) Boxplots and dotplots showing the m6Am levels of different A-TSNs in JUN, ENO1, MYC, and ACTB. These genes contain A-TSNs with relatively low m6Am stoichiometry. In this plot, the exact m6Am levels of individual A-TSNs are shown in dots, while the median and IQR of the m6Am levels are shown in boxplots. Only m6Am sites with ≥50 reads mapped were analyzed. (F) Gene Ontology enrichment (Cellular Components) of genes containing lowly methylated m6Am sites. (G) A-TSNs in histone genes tend to have relatively low m6Am stoichiometry. In this plot, histone genes are categorized by their genomic localizations. Histone gene cluster 6p22.1–2 and 1q21.1–2 are the two major histone gene clusters. For histone gene cluster 6p22.1–2, 55–173 A-TSNs are shown in different cell lines; for histone gene cluster 1q21.1–2, 9–14 A-TSNs are shown; for other histone genes, 24–109 A-TSNs are shown.
-
Figure 3—source data 1
mRNA m6Am stoichiometries in different cell lines.
- https://cdn.elifesciences.org/articles/104139/elife-104139-fig3-data1-v1.xlsx
-
Figure 3—source data 2
Gene Ontology enrichment of genes with relatively low m6Am sites.
- https://cdn.elifesciences.org/articles/104139/elife-104139-fig3-data2-v1.xlsx
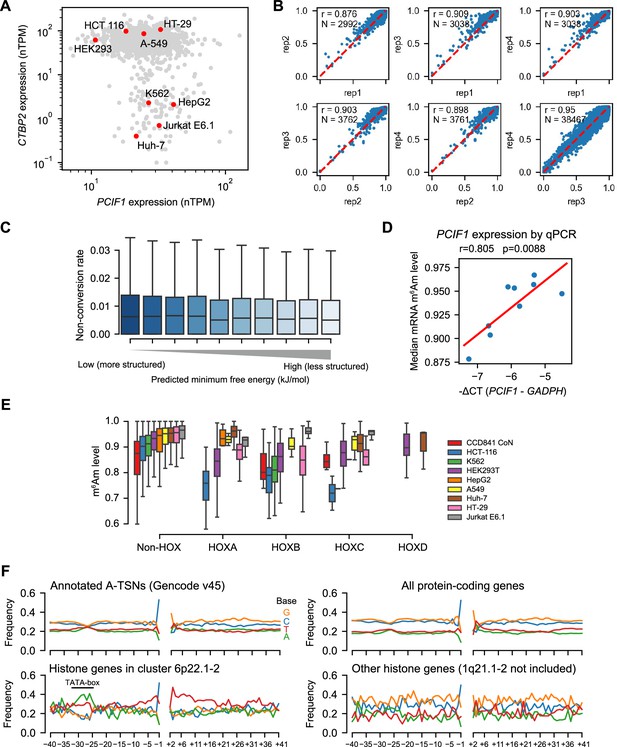
Corresponding to Figure 3.
(A) Several cell lines exhibit low CTBP2 expression. Shown are normalized RNA expression results from the Human Protein Atlas (https://www.proteinatlas.org) (Uhlén et al., 2015). (B) The 50 reads threshold yields highly correlated non-conversion rates among replicates. Shown are the pairwise comparisons of non-conversion rates of A-TSNs quantified in different HEK293T replicates. In this analysis, A-TSNs were required to have at least 50 reads mapped. As a result, the A-TSNs in different replicates have median read counts of 105–149. High correlations (Pearson’s r) in non-conversion rates were found between replicates. (C) RNA secondary structure is unlikely to affect m6Am quantification accuracy in CROWN-seq. Shown are the non-conversion rates of A-TSNs grouped by the degrees of RNA secondary structure in PCIF1 knockout HEK293T cells, which have no m6Am in mRNA. To obtain the degree of RNA secondary structure of each 5’ end, we calculated the minimum free energy of A-TSN plus the first 30 nt downstream sequence by RNAfold (Lorenz et al., 2011). 18,235 A-TSNs were binned into 10 bins based on the quantile of minimum free energy. For each bin (from left to right), the medium minimum free energies are −11.4,–9.2, −7.9,–6.9, −6.0,–5.3, −4.5,–3.6, –2.6, and –0.9 kJ/mol. (D) Comparing median mRNA m6Am stoichiometry and PCIF1 expression estimated by RT-qPCR. In this assay, RT-qPCR on PCIF1 and GADPH transcripts was performed among all nine different cell lines. The relative expression levels of PCIF1 were normalized by the expression of GAPDH. Linear regression was performed to obtain Pearson’s r and P-value. (E) Boxplots showing the m6Am levels of HOX genes among different cell lines. (F) Histone genes in cluster 6p22.1–2 exhibited distinct sequence context in the core promoter. Shown are the flanking sequences of the 146,285 A-TSN that annotated in Gencode v45 (upper left), 104,733 A-TSNs mapped to protein-coding genes in CROWN-seq (upper right), 228 histone genes in cluster 6p22.1–2 (lower left), and 119 histone genes not in neither cluster 6p22.1–2 nor 1q21.1–2 (lower left). Since a few (14) A-TSNs were found in cluster 1q21.1–2, A-TSNs in cluster 1q21.1–2 are not shown.
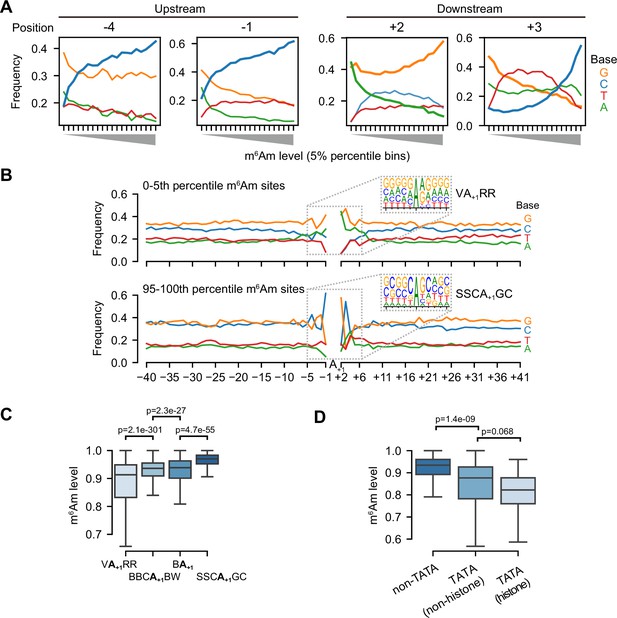
m6Am stoichiometry is related to core promoter sequence.
(A) m6Am stoichiometry is related to base composition in both upstream and downstream of A-TSNs. In these plots, 58,723 A-TSNs are grouped into twenty 5-percentile bins (X-axis). For each bin, the frequency of A, T, C, and G bases at each position relative to the A-TSN are plotted on the Y-axis. Among different positions in the promoter region, C’s in −4,–1, and +3, as well as G’s in +2 are positively correlated with high m6Am; while A’s in +2 are negatively correlated with high m6Am. Results for other promoter positions can be found in Figure 4—figure supplement 1A. (B) Motif analysis of A-TSNs with the lowest 5% m6Am stoichiometry (upper) and the A-TSNs with the highest 5% m6Am stoichiometry (lower). The core promoter region (–40 to +41) was screened for enriched motifs. The lowest 5% A-TSNs exhibited a VA+1RR TSS (V=A/C/G, R=A/G) motif, while the highest 5% A-TSNs exhibited a SSCA+1GC (S=C/G) motif. The sequence contexts for all A-TSNs are shown in Figure 3—figure supplement 1F. (C) A-TSNs expressed from different core promoters exhibit different m6Am stoichiometry. Core promoters containing the VA+1RR motif produce transcripts with relatively low m6Am stoichiometry. Transcripts using the SSCA+1GC motif exhibited relatively high m6Am stoichiometry. In comparison, the m6Am stoichiometry in conventional A-TSNs from either BBCA+1BW or BA+1 is also shown and exhibits intermediate m6Am stoichiometry. In this analysis, 14,788, 7981, 34,578, and 1376 A-TSNs were used for each of the four motifs. p-values, Student’s t-test, two-sided. (D) TATA-box containing core promoters exhibit relatively low m6Am stoichiometry. For this analysis, the TATA-box is defined as TATAWAWR (Haberle and Stark, 2018). Because many TATA-boxes found in our A-TSN dataset are outside the classic –31 to –24 region, we extended the region for the TATA-box search to –36 to –19. Since histone genes preferentially contain TATA box, we separately plotted TATA-box-containing histone genes (N=155) and TATA-box-containing non-histone genes (N=28). 58,540 A-TSNs without TATA-box are also shown. p-values, Student’s t-test, two-sided.
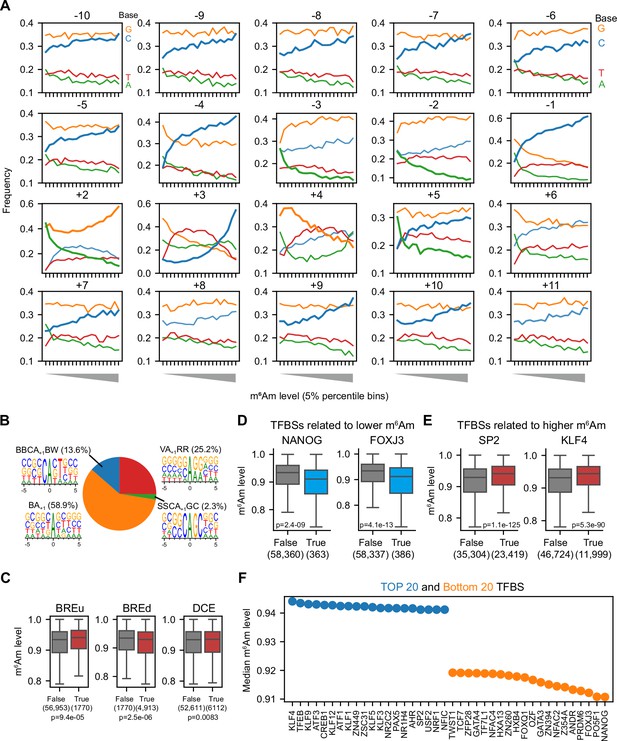
Corresponding to Figure 4.
(A) Corresponding to Figure 4A, the base preference in –10 to +11 core promoter region of the 58,723 A-TSNs in HEK293T cell. To examine the correlation between m6Am stoichiometry and base compositions, we equally binned the A-TSNs by m6Am stoichiometry into twenty 5-percentile bins. For each bin, the frequency of A, T, C, and G bases are shown. (B) A pie plot showing the fractions of A-TSNs using different TSS motifs. In this plot, 58,723 A-TSNs in HEK293T cells are shown, where 1376 are from the SSCA+1 GC motif, 7981 are from BBCA+1BW motif, 14,788 are from VA+1RR motif, and 34,578 are from BA+1 motif. (C) Examining the relationship between core promoter elements and m6Am stoichiometry. In this analysis, core promoter elements (BREu, BREd, and DCE) were defined according to the description by Haberle and Stark, 2018 (indicated as ‘True’). BREu-containing promoters were defined as promoters that contain SSRCGCC (S=C/G, R=A/G) at –38 to –32; BREd-containing promoters were promoters that contain RTDKKKK (D=A/G/T, K=G/T) at –23 to –17; DCE containing promoters were defined as promoters which contain CTTC at +6 to+11, or CTGT at +16 to+21, or AGC at +30 to+34. Among these elements, BREu and BREd are related to TFIIB binding, while DCE is related to TAF1 binding (Haberle and Stark, 2018). (D, E), Examples of m6Am stoichiometry for A-TSNs containing specific transcription factor-binding sites. In this analysis, m6Am stoichiometry of the 58,723 A-TSNs in HEK293T was used; transcription factor-binding sites within the –50 to +51 region were identified by FIMO (Grant et al., 2011) based on the HOCOMOCO v11 core motifs database (Kulakovskiy et al., 2018). Transcripts containing NANOG and FOXJ3-binding sites (indicated as ‘True’) exhibit relatively lower m6Am stoichiometry, while SP2 and KLF4-binding sites are associated with higher relative m6Am stoichiometry. The overall effect size is relatively small, which suggests that transcription factors may not have large effects on m6Am stoichiometry. p-values, Student’s t-test, two-sided. (F) Some transcription factor binding sites (TFBS) are related to higher or lower m6Am stoichiometry. In this figure, the top 20 and bottom 20 m6Am-related TFBS are shown. The m6Am stoichiometry and the –50 to +51 sequence of the 58,723 A-TSNs in HEK293T cells were analyzed. FIMO (Grant et al., 2011) was used to scan for core motifs in the HOCOMOCO v11 database. Only TFBS occurred in the flanking sequence of at least 200 A-TSNs are shown.
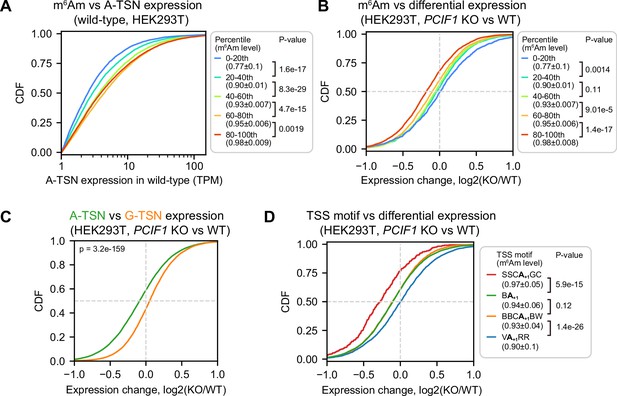
PCIF1 knockout leads to m6Am and TSS motif-related A-TSN expression changes.
(A) m6Am stoichiometry is positively related to A-TSN expression in wild-type HEK293T cells. In this cumulative distribution plot, the expression of each A-TSN was quantified by ReCappable-seq, for all A-TSNs in each indicated m6Am stoichiometry bin. A-TSNs (n=58,723) were grouped into five bins based on m6Am stoichiometry quantified by CROWN-seq. In total, 5125, 6962, 7991, 8368, and 8009 A-TSNs are shown in each bin (from low m6Am to high m6Am). These A-TSNs have an average TPM ≥1 in two ReCappable-seq replicates and coverage ≥50 in CROWN-seq. p-values, Student’s t-test for TPM (log-transformed), two-sided. (B) The expression level of high m6Am stoichiometry A-TSNs is reduced in PCIF1 knockout. Shown is a cumulative distribution plot of A-TSN expression change in HEK293T cells upon PCIF1 knockout. The differential expression of A-TSN was calculated by DESeq2 (Love et al., 2014). Similar to (A), the A-TSNs were binned based on the m6Am stoichiometry. In total, 3269, 2272, 3218, 3813, and 3369 A-TSNs are shown in each bin (from low m6Am to high m6Am). A-TSNs with a baseMean (i.e. the average of the normalized count among replicates) ≥100 were used in the differential expression test (two replicates were used for both wild-type and PCIF1 knockout) and coverage ≥50 reads in CROWN-seq. p-values, Student’s t-test, two-sided. (C) Shown are cumulative distribution plots of expression changes of A-TSNs and G-TSNs after PCIF1 depletion. 14,516 A-TSNs and 9667 G-TSNs with expression levels quantified by ReCappable-seq are shown. These A-TSNs and G-TSNs have baseMean ≥ 100 during the differential expression test. p-values, Student’s t-test, two-sided. (D) Similar to (B), A-TSNs that use different TSS motifs exhibit different changes in expression upon PCIF1 knockout. In total, 352 A-TSNs using SSCA+1GC, 7928 A-TSNs using BA+1, 2958 A-TSNs using BBCA+1BW, and 2760 A-TSNs using VA+1RR are shown. These A-TSNs have baseMean ≥ 100 during differential expression test (two replicates for both wild-type and PCIF1 knockout) and coverage ≥50 reads in CROWN-seq. p-values, Student’s t-test, two-sided.
-
Figure 5—source data 1
Comparing A-transcription-start nucleotide expression between wild-type and PCIF1 knockout.
- https://cdn.elifesciences.org/articles/104139/elife-104139-fig5-data1-v1.xlsx
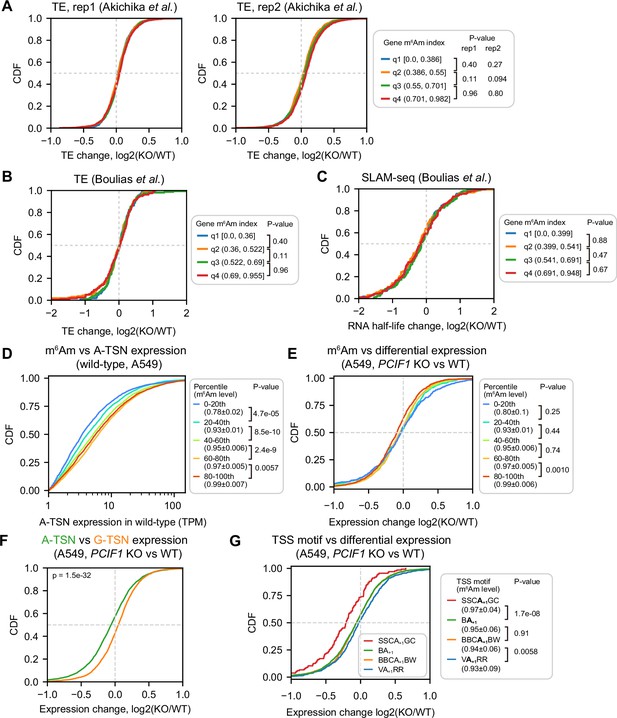
Corresponding to Figure 5.
(A) PCIF1 knockout did not alter translation efficiency in HEK293T cells. Shown are ribosome profiling results by Akichika et al., 2019. In this analysis, 3325 genes were equally binned into quantiles by gene m6Am index. p-values, Student’s t-test, two-sided. (B) PCIF1 knockout did not alter translation efficiency in HEK293T cells. Shown are ribosome profiling results by Boulias et al., 2019. In this analysis, 637 genes were equally binned into quantiles by gene m6Am index. p-values, Student’s t-test, two-sided. (C) PCIF1 knockout did not alter RNA stability in HEK293T cells. Shown are RNA half-life results estimated by SLAM-seq by Boulias et al., 2019. In this analysis, 666 genes were equally binned into quantiles by gene m6Am index. p-values, Student’s t-test, two-sided. (D) m6Am stoichiometry is positively related to A-TSN expression in wild-type A549 cells. In this cumulative distribution plot, A-TSNs with expression quantified by ReCappable-seq in different m6Am stoichiometry bins are shown. To group different A-TSNs, we first binned 58,723 A-TSNs into five bins based on m6Am stoichiometry quantified by CROWN-seq. Then the A-TSNs detected in ReCappable-seq were annotated by the predefined bins. In total, 5125, 6962, 7991, 8368, and 8009 A-TSNs are shown in each bin (from low m6Am to high m6Am). These A-TSNs have an average TPM ≥1 in two ReCappable-seq biological replicates and coverage ≥50 in CROWN-seq. p-values, Student’s t-test for TPM (log-transformed), two-sided. (E) A-TSNs in high m6Am stoichiometry are more susceptible to PCIF1 knockout. Shown are the cumulative distributions of A-TSN expression change in A549 cells upon PCIF1 knockout. The differential expression of A-TSN was calculated by DESeq2 (Love et al., 2014). Similar to (D), the A-TSNs were binned based on the m6Am stoichiometry. In total, 481, 738, 973, 1212, and 1087 A-TSNs are shown in each bin (from low m6Am to high m6Am). These A-TSNs have baseMean (i.e. the average of the normalized count among replicates) ≥100 during the differential expression test (2 biological replicates for both wild-type and PCIF1 knockout) and coverage ≥50 in CROWN-seq. p-values, Student’s t-test, two-sided. (F) Shown are cumulative distributions of expression changes of A-TSNs and G-TSNs. 4975 A-TSNs and 3510 G-TSNs with expression levels quantified by ReCappable-seq are shown. These TSNs have an average baseMean ≥ 100 of two replicates. p-values, Student’s t-test, two-sided. (G) Similar to (F), A-TSNs using different TSS motifs exhibited different changes in expression upon PCIF1 knockout. In total, 135 A-TSNs using SSCA+1GC, 2.502 A-TSNs using BA+1, 1131 A-TSNs using BBCA+1BW, and 723 A-TSNs using VA+1RR are shown. These A-TSNs have baseMean ≥ 100 during the differential expression test (two biological replicates for both wild-type and PCIF1 knockout) and coverage ≥50 in CROWN-seq. p-values, Student’s t-test, two-sided.
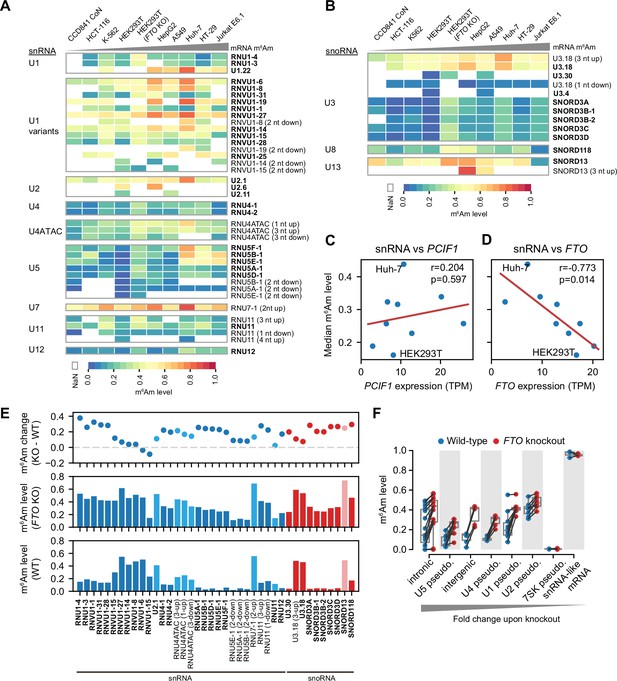
CROWN-seq reveals m6Am landscape in snRNA and snoRNA.
(A) Heatmaps showing m6Am stoichiometry in different snRNA gene families and isoforms. Cell lines in the column are ranked by the overall mRNA m6Am stoichiometry. The name of each snRNA isoform is shown on the right. A-TSNs already annotated in Gencode v45 are highlighted in bold. For newly found A-TSNs, the relative distance between the new A-TSN and the nearest annotated A-TSN is shown in brackets. Note that Gencode v45 contains snRNA annotation from different databases. For example, RNU1-4 and U1.22 are both U1 snRNA, however, RNU1-4 is from the HGNC database and U1.22 is from the RFAM database. (B) Similar to (A), Heatmaps show the m6Am stoichiometry in different snoRNA isoforms. (C, D), snRNA methylation levels are not well correlated with PCIF1 expression, but negatively correlated with FTO expression. The RNA expression levels of PCIF1 and FTO were estimated by reading counts in CROWN-seq, which were converted into TPM to normalize the sequencing depth. Linear regressions were performed to obtain Pearson’s r and p-value of the correlations. (E) FTO depletion leads to increased m6Am level (i.e. m6Am stoichiometry) in many kinds of snRNA and snoRNA. In this plot, the difference in m6Am levels between wild-type and FTO knockout cells is shown in the first row. The exact m6Am levels in FTO knockout and wild-type cells are shown in the second and third rows. Different kinds of snRNA and snoRNA are shown in different colors. (F) FTO depletion leads to increased m6Am stoichiometry in snRNA and snoRNA pseudogenes. In this plot, shown are the annotated pseudogenes of U1, U2, U4, U5, and 7SK, as well as the newly identified snRNA/snoRNA pseudogenes in intronic and intergenic regions. Several mRNAs exhibited 5’ ends resembling snRNA pseudogenes. However, these snRNA-like mRNA 5’ ends showed high and stable m6Am stoichiometry in both wild-type and FTO knockout cells.
-
Figure 6—source data 1
The changes of stoichiometry of m6A sites close to the 5’ end of mRNA upon FTO knockout.
- https://cdn.elifesciences.org/articles/104139/elife-104139-fig6-data1-v1.xlsx
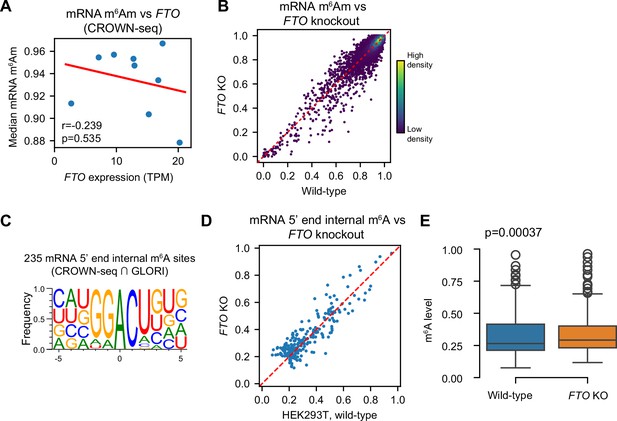
Corresponding to Figure 6.
(A) FTO expression correlates weakly to mRNA m6Am stoichiometry. Shown are FTO expression measured by CROWN-seq (X-axis) and median mRNA m6Am levels among nine different cell lines. (B) FTO depletion causes small changes in mRNA m6Am stoichiometry. In this scatter plot, only mRNA A-TSNs which have at least 50 reads in both wild-type and FTO knockout cells were shown. m6Am levels were estimated by CROWN-seq. (C) The internal m6A sites identified by both CROWN-seq and GLORI (Liu et al., 2023) showed a classic DRACU motif. (D, E) FTO knockout showed a subtle effect in changing the stoichiometry of internal m6A around 5’ ends. Shown are (D) a scatter plot comparing m6A stoichiometry between wild-type and FTO knockout cells, and (E) a boxplot showing the overall m6A stoichiometry difference. In (E), Student’s t-test (two-sided) was performed to calculate the significance of the difference in m6A stoichiometry.
Tables
Reagent type (species) or resource | Designation | Source or reference | Identifiers | Additional information |
---|---|---|---|---|
Gene (Homo sapiens) | PCIF1 | Enesmbl | ENSG00000100982 | |
Gene (H. sapiens) | FTO | Enesmbl | ENSG00000140718 | |
Cell line (H. sapiens) | HEK293T | ATCC | CRL-3216 | |
Cell line (H. sapiens) | HEK293T, PCIF1 knockout | Boulias et al., 2019 | ||
Cell line (H. sapiens) | HEK293T, FTO knockout | Mauer et al., 2019 | ||
Cell line (H. sapiens) | A549 | ATCC | CCL-185 | |
Cell line (H. sapiens) | A549, PCIF1 knockout | This study | ||
Cell line (H. sapiens) | HepG2 | ATCC | HB-8065 | |
Cell line (H. sapiens) | Huh-7 | ThermoFisher | huh 7 Cells | |
Cell line (H. sapiens) | Jurkat E6.1 | ATCC | TIB-152 | |
Cell line (H. sapiens) | K-562 | ATCC | CCL-243 | |
Cell line (H. sapiens) | CCD841 CoN | ATCC | CRL-1790 | |
Cell line (H. sapiens) | HCT-116 | ATCC | CCL-247 | |
Cell line (H. sapiens) | HT-29 | ATCC | HTB-38 | |
Sequence-based reagent | ReCappable-seq 5' adapter (11 N) | IDT | RNA adapter | rCrCrUrArCrArCrGrArCrGrCrUrCrUrUrCrCr GrArUrCrUrNrNrNrNrNrNrNrNrNrNrNrArUrArU |
Sequence-based reagent | ReCappable-seq 3' adapter | IDT | DNA adapter | /5rApp/WWAGATCGGAAGAGCACACGTC/3ddC/ |
Sequence-based reagent | CROWN-seq 5' adapter (8 N) | IDT | RNA adapter | rCrCrUrArCrArCrGrArCrGrCrUrCrUrUr CrCrGrArUrCrUrNrNrNrNrNrNrNrNrArUrArU |
Sequence-based reagent | CROWN-seq 5' adapter (11 N) | IDT | RNA adapter | rCrCrUrArCrArCrGrArCrGrCrUrCrUrUrCr CrGrArUrCrUrNrNrNrNrNrNrNrNrNrNrNrArUrArU |
Sequence-based reagent | CROWN-seq 3' adapter | IDT | RNA adapter | /5’rApp/AGATCGGAAGAGCACACGTCTGAACTCCAGTCACAAAAAAAAAAAAAAACCCCCCCCCCAAAAAAAAAAAAAAA/3AmMO/ |
Sequence-based reagent | ReCappable-seq/ CROWN-seq RT primer | IDT | RT primer | GACGTGTGCTCTTCCGATCT |
Sequence-based reagent | GLORI 5' adapter (11 N) | IDT | RNA adapter | rCrCrUrArCrArCrGrArCrGrCrUrCrUrUrCrCr GrArUrCrUrNrNrNrNrNrNrNrNrNrNrNrArUrArU |
Sequence-based reagent | GLORI 3' adapter | IDT | DNA adapter | /5rApp/AGATCGGAAGAGCACACGTC/3AmMO/ |
Sequence-based reagent | GLORI RT primer | IDT | RT primer | GACGTGTGCTCTTCCGATCT |
Sequence-based reagent | PCIF1_qPCR_F | IDT | qPCR primer | GGAGAATCGTCCCTACTACTT |
Sequence-based reagent | PCIF1_qPCR_R | IDT | qPCR primer | GCTTTCTGGGCTTGTTCT |
Sequence-based reagent | GAPDH_qPCR_F | IDT | qPCR primer | GTGGACCTGACCTGCCGTCT |
Sequence-based reagent | GAPDH_qPCR_R | IDT | qPCR primer | GGAGGAGTGGGTGTCGCTGT |
Software, algorithm | HISAT2 | Kim et al., 2019 | RRID:SCR_015530 | v2.2.1 |
Software, algorithm | UMI-tools | Smith et al., 2017 | RRID:SCR_017048 | v1.1.1 |
Software, algorithm | BEDtools | Quinlan and Hall, 2010 | RRID:SCR_006646 | v2.27.1 |
Software, algorithm | SAMtools | Li et al., 2009 | RRID:SCR_002105 | v1.16.1 |
Software, algorithm | Python3 | Python | RRID:SCR_008394 | v3.8.7 |
Software, algorithm | R | R | RRID:SCR_001905 | v4.2.2 |
Software, algorithm | numpy | PyPI | RRID:SCR_008633 | v1.23.5 |
Software, algorithm | pandas | PyPI | RRID:SCR_018214 | v1.5.2 |
Software, algorithm | scipy | PyPI | RRID:SCR_008058 | v1.93 |
Software, algorithm | pysam | Li et al., 2009 | RRID:SCR_021017 | v0.19.1 |
Software, algorithm | DESeq2 | Love et al., 2014 | RRID:SCR_015687 | v1.38.1 |
Software, algorithm | RUVSeq | Risso et al., 2014 | RRID:SCR_006263 | v1.38.0 |
Software, algorithm | GLORI analysis pipeline | This paper | v1.0; https://github.com/jhfoxliu/GLORI_pipeline | |
Software, algorithm | ReCappble-seq analysis pipeline | This paper | v1.0; https://github.com/jhfoxliu/ReCappable-seq | |
Software, algorithm | CROWN-seq analysis pipeline | This paper | v1.0; https://github.com/jhfoxliu/CROWN-seq |
Additional files
-
Supplementary file 1
The overview of CROWN-seq libraries.
- https://cdn.elifesciences.org/articles/104139/elife-104139-supp1-v1.xlsx
-
MDAR checklist
- https://cdn.elifesciences.org/articles/104139/elife-104139-mdarchecklist1-v1.docx