Primate amygdala neurons evaluate the progress of self-defined economic choice sequences
Figures
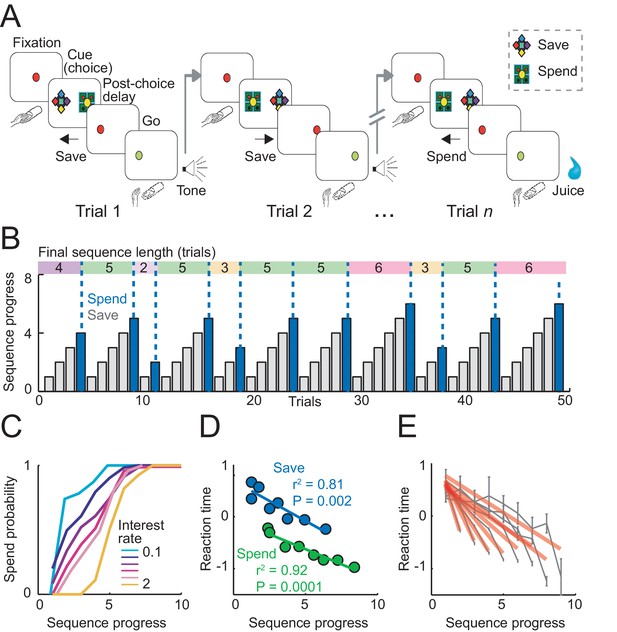
Sequential choice task and behavior.
(A) Monkeys made sequential choices to save (accumulate) or spend (consume) reward. Consecutive save choices increased reward amount according to the current interest rate; spend choice resulted in reward delivery. Different save cues indicated interest rates. Choice sequences lasted up to nine consecutive trials (~12 s per trial) and were self-determined, which allowed the animals to plan their behavior several steps in advance. (B) Example behavioral data. Bars show trial-by-trial choice record (gray: save, blue: spend). Sequence progress corresponds to the cumulative trial record within each sequence. Colored boxes delineate saving sequences; numbers indicate the sequence length. (C) Increases in spend choice probability as a function of sequence progress, shown for different interest rate conditions. (D) Reaction times decreased with sequence progress on both spend and save trials (linear regression; mean reaction times for equally populated sequence progress bins; error bars smaller than symbols). Reaction times in this figure were measured as the latencies with which the animals released a touch key at the end of a trial to initiate reinforcer delivery (liquid reward on spend trials, auditory cue on save trials). (E) Reaction times adapted to sequence length. Gray data show reaction times as a function of sequence progress separately for different sequence lengths (N = 19,612 trials). Red lines show fits from linear regression.
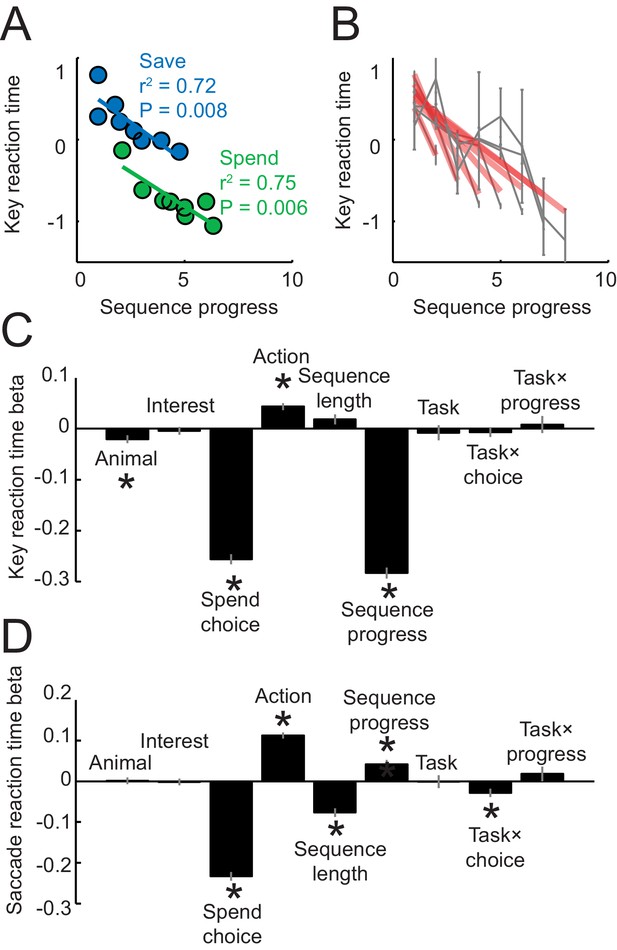
Reaction times analyses.
Reaction times in panels A–C were measured as the latencies with which the animals released a touch key at the end of a trial to initiate reinforcer delivery (liquid reward on spend trials, auditory cue on save trials); reaction times in panel D were measured as the latency from choice cue onset to fixation of the chosen cue. (A) Key release reaction times decreased with sequence progress on instructed spend and save trials (linear regression). Compared to Figure 1D, the apparent restricted range of sequence progress along the x-axis only resulted from the data binning process for producing the plot but did not reflect genuine differences in sequence length ranges (sequence length ranges did not differ between tasks, p=0.949, t-test), and the full range of sequence lengths in the imperative tasks is shown in panel (B). (B) Key reaction times as a function of sequence progress separately for different sequence lengths. Red lines show fits from linear regression. (C) Multiple linear regression of key reaction times on different variables. ‘Task’ indicates a dummy variable for free choice vs. imperative task. Asterisks indicate significance (p<0.05 as criterion; all significant effects p<0.008). (D) Multiple linear regression of saccade reaction times on different variables. Although saccadic reaction times showed a positive relationship to the sequence (compared to the negative relationship for key reaction times), we are cautious in interpreting this effect, as touch key reactions seemed overall more directly related to the animals’ motivation: release of touch key was the final behavioral response on each trial before reinforcer delivery and, in further analyses, touch key release latencies showed a stronger relationship to upcoming reward magnitude on spend trials (R2 = 0.116, p<0.0001) compared to saccade reaction times (R2 = 0.017, p<0.001).
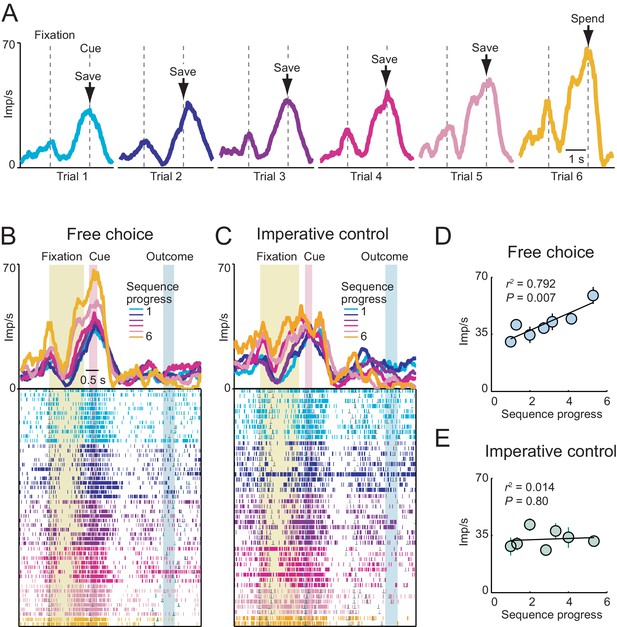
Gradually increasing activity related to sequence progress in a single amygdala neuron.
(A) Neuronal response in the fixation and cue periods, plotted for each step of a six-trial choice sequence. Color indicates sequence progress. (B) Peri-event time histogram for the same neuron, aligned to fixation onset, sorted by sequence progress. Raster display: ticks indicate impulses, rows indicate trials; grey dots indicate event markers (labelled above graph). Activity in cue phase and preceding fixation phase reflected sequence progress, before the animal indicated its current-trial choice. Visual stimulation during fixation was constant over consecutive trials. Randomized cue positions precluded preplanning of action sequences. (C) The progress-related activity in the cue phase disappeared during performance of the imperative control task. (D, E) Linear regression (Equation 2) of trial-by-trial cue-phase activity on sequence progress in free choice and imperative task (error bars: s.e.m).
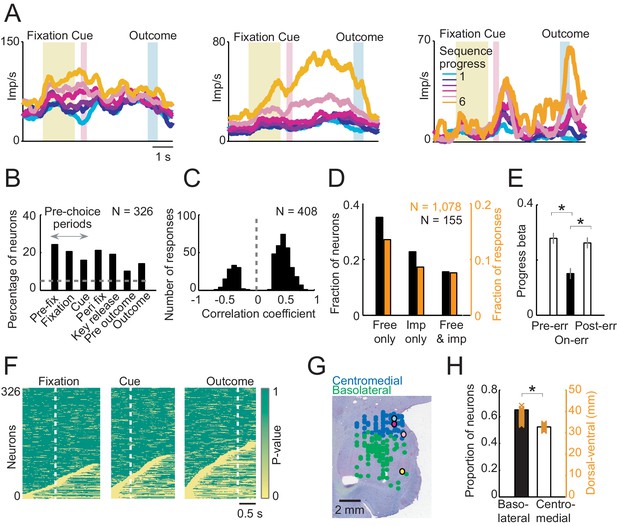
Sequence progress activity in the population of amygdala neurons.
(A) Three single neurons with progress-related activity in fixation and cue phases (left), delay period from choice to outcome (middle) and outcome phase (right). (B) Percentages of neurons with progress-related activity (linear regression, Equation 2) for all task periods. (C) Distribution of regression slopes for all responses with significant sequence progress encoding (i.e. significant regression slopes aggregated across all task periods and neurons, Equation 2). (D) Comparison with imperative task. Fraction of neurons (black) and responses (orange) significant for sequence progress (Equation 2) in the free choice task only (‘Free only’), imperative control task only (‘Imp only’) and both tasks (‘Both’), based on 155 neurons (1078 responses) tested in both tasks. The fraction of neurons and responses significant in the free choice task only was significantly higher compared to both other categories (p<0.001, z-test for dependent samples). (E) Relationship to performance errors. Bars show standardized regression coefficients (± s.e.m) from a population analysis regressing normalized activity on sequence progress for trials preceding errors (Pre-err), error trials (On-err) and trials following errors (Post-err). The strength of progress coding was reduced on error trials compared to immediately preceding trials and subsequently reappeared following error trials (p<0.001; dependent-samples t-tests). (F) Statistical p-values for linear regression of activity on sequence progress in all neurons, obtained from sliding window analysis (Equation 2; window size: 200 ms, step size: 25 ms) aligned to fixation, cue and outcome events. Data in each row are from a single neuron, sorted from bottom to top within each panel according to coding latency. For clarity, p-values>0.05 were set to 1. (G) Histological reconstruction of recording sites for progress-sensitive neurons, shown separately for centromedial and basolateral amygdala regions. Yellow, pink, magenta, and cyan symbols: example neurons in Figures 2 and 3A. Collapsing across anterior-posterior dimension resulted in symbol overlap. (H) Proportion of neurons (N = 194) with sequence progress activity in basolateral and centromedial amygdala (p=0.005, χ2-test). Orange symbols: Recording depths of sequence progress neurons (dorsal-ventral axis, reference bregma).
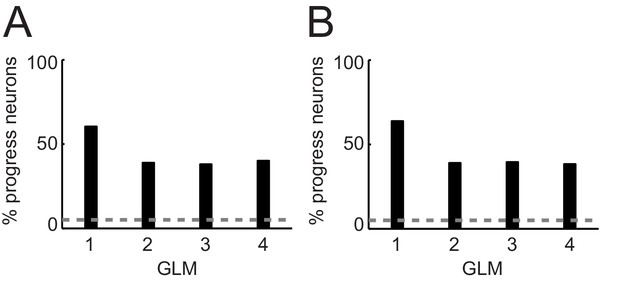
Percentages of progress-sensitive neurons obtained from different regression models.
(A) General linear models (GLM) that include non-adaptive sequence progress. 1: Single linear regression of neuronal activity on sequence progress (Equation 2); 2: stepwise model that includes the regressors save-spend choice, cue position, left-right action, spend value, save value, sequence value, sequence length, sequence progress (Equation 7); 3: same GLM as GLM2 but excluding sequence value (subset of regressors from Equation 7); 4: same GLM as GLM2 but excluding sequence length (subset of regressors from Equation 7). (B) GLMs that include adaptive sequence progress. 1: Single linear regression of neuronal activity on adaptive sequence progress (Equation 3); 2: stepwise model that includes the regressors save-spend choice, cue position, left-right action, spend value, save value, sequence value, sequence length, adaptive sequence progress (Equation 7 but with adaptive sequence progress); 3: same GLM as GLM2 but excluding sequence value (subset of regressors from Equation 7); 4: same GLM as GLM two but excluding sequence length (subset of regressors from Equation 7).
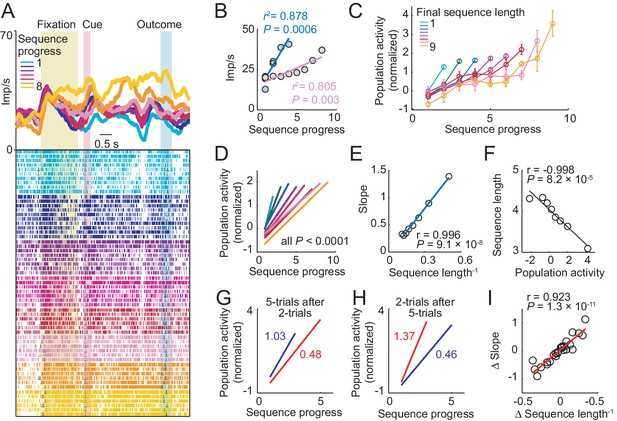
Amygdala progress activity adapts to internally planned sequence length.
(A, B) Adaptation of progress activity in a single neuron. (A) The neuron showed progress-related activity in the delay period between cue and outcome events. (B) Linear regression (Equation 2) of the neuron’s trial-by-trial delay-period activity on sequence progress for short (< five trials) and long (> four trials) sequences. Activity rose more steeply during short compared to long sequences (raw slopes: 7.33 vs. 2.14). Note that sequence lengths were internally planned rather than instructed, suggesting prospective adaptation to an internally planned parameter. (C) Adaptation to sequence length in population activity. Normalized activity for all neuronal responses significant for sequence progress (N = 408 responses from 194 neurons. Equation 2), plotted separately for different sequence lengths (mean ± s.e.m). (D) Linear regression fits to data in (C). (E) Slopes from linear fits in (D) plotted against the inverse sequence length (linear regression). (F) Prediction of final sequence length from population activity. Linear regression of sequence length on the population activity of progress-related neuron responses, measured on the second trial of each sequence. (G–I) Adaptation for consecutive choice sequences. (G) Linear fits of population activity to sequence progress for five-trial sequences and immediately preceding two-trial sequences. Colored numbers indicate slopes. (H) Linear fits for two-trial sequences and immediately preceding five-trial sequences. (I) Linear regression of slope differences (Δ Slope), obtained from neuronal activity during consecutive sequences, on corresponding differences in inverse lengths of consecutive sequences (Δ Sequence length−1).
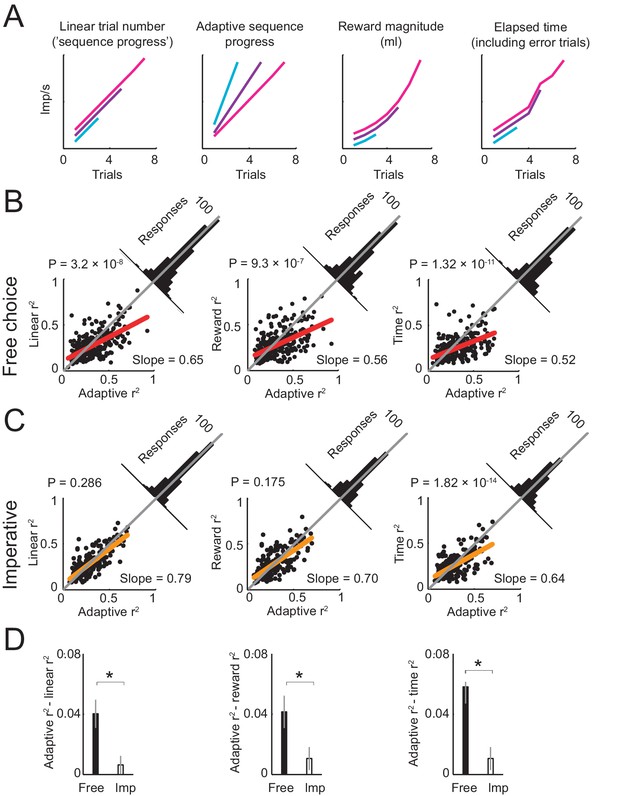
Comparing different models of sequence progress.
(A) Schematic illustration of four models of gradual activity. Left to right: (i) linear non-adaptive sequence progress (cumulative record of correct trials within sequence; Equation 2); (ii) adaptive sequence progress (cumulative trial record normalized by the final sequence length; Equation 3); (iii) reward magnitude (saved juice amount in current sequence in ml, shown for a typical interest rate; Equation 4); (iv) elapsed sequence time (cumulative trial record including error trials; Equation 5). The curves are vertically separated for visibility. Values generated by the four models were used as regressors for neuronal activity. (B) Model comparison in free choice task. The adaptive progress model provided a significantly better fit compared to all alternatives. Each scatter plot compares the R2 (explained variance) obtained by fitting the adaptive progress model to the R2 obtained by fitting the non-adaptive progress (left), reward magnitude (middle), and elapsed time (right) models. Red lines: linear fits of R2 values for model pairs; deviation from unity line (gray) towards the horizontal axis indicates better fit of adaptive model. Angled histograms show distributions of R2 differences; p-values indicate deviation from normal distribution (Kolmogorov-Smirnov test). Each plot is based on all neuronal responses with significant fits for both compared models. (C) Model comparison for the imperative task. The adaptation model did not provide better fits compared to non-adaptive and reward magnitude models. Each plot is based on all neuronal responses with significant fits for both compared models. (D) Comparison of differences in model fit (R2) between free choice and imperative tasks. All R2-differences in free choice task but none in the imperative task were significantly greater than zero (p<0.05, two-tailed t-test). All differences in free choice task were significantly greater than those in imperative task (p<0.05, paired t-test). Each plot is based on all neuronal responses with significant fits for both free choice and imperative task.
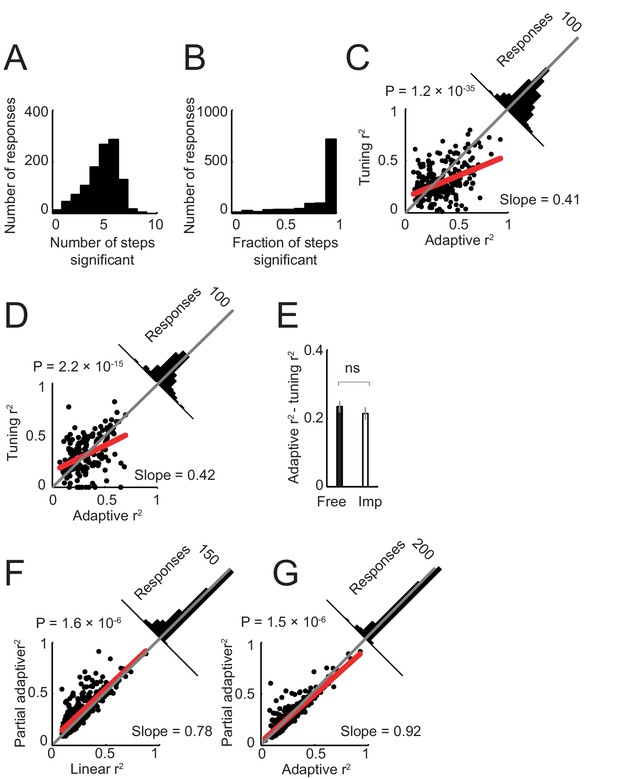
Model of neuronal activity based on tuning for specific sequence steps.
Previous neurophysiological studies involving behavioral sequences showed that single neurons in the amygdala (Sugase-Miyamoto and Richmond, 2005) and frontal cortex (Mushiake et al., 2006) can be tuned to specific steps of a sequence. Accordingly, we examined whether such step-tuning provided a good model for amygdala activity in our sequential choice task. We fitted a general linear model that included indicator (dummy) variables for specific sequence steps (i.e. separate regressors for separate steps; Equation 6), to neuronal activity in all task periods. (A). Distribution of neuronal responses with significant effects for different numbers of sequence steps. The majority of responses showed significant effects for several sequence steps (rather than specificity for individual steps). (B) Distribution of responses with significant effects for different fractions of steps (one indicates significant effects for all step regressors). The majority of responses were significant for all step regressors. (C) Model comparison in free choice task. The adaptive progress model provided a significantly better fit compared to the step-tuning model. (D) Model comparison in imperative task. (E) Comparison of differences in model fit (R2) between free choice and imperative tasks. (F) Model comparison in free choice task. A partial adaptive sequence progress model (Equation 8) provided a better fit compared to the non-adaptive progress model (R2 values were significantly higher, p<0.0001, rank-sum test). (G) Model comparison in free choice task. A partial adaptive sequence progress model and the full adaptive sequence progress model fit the data equally well (R2 values were not significantly different, p=0.423, rank-sum test).
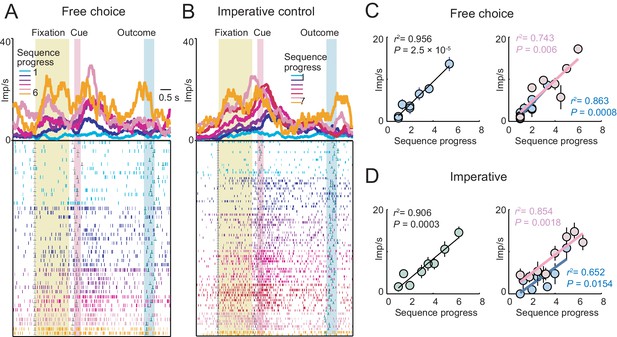
A single amygdala neuron with gradually increasing activity in both free choice and imperative task.
(A, B) Peri-event time histograms for activity in free choice (A) and imperative (B) task, aligned to fixation onset, sorted by sequence progress. (C, D) Linear regression (Equation 2) of fixation-period activity on sequence progress in free choice (C) and imperative (D) task, shown across all trials (left panels) and separately for short and long sequences (right panels). The neuron showed significant progress-related activity in both tasks without apparent adaptation to sequence length.
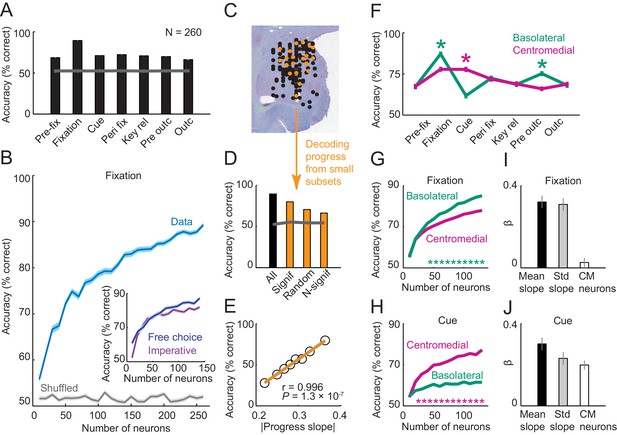
Population decoding of sequence progress.
(A) Decoding accuracy (% correct classification) of a nearest-neighbor classifier. Leave-one-out cross-validated decoding was significantly above decoding from shuffled data (gray line; all p<0.0001; Wilcoxon test). Decoding was based on normalized single-trial activity of all neurons that met criteria of decoding analysis (N = 260) without pre-selection for task-relatedness. (B) Performance increased with the number of neurons, shown here for the fixation period. Data for each neuron number show mean (± s.e.m) over 100 iterations of randomly selected neurons. Inset shows decoding for a subset of neurons recorded in both free choice and imperative task. (C–E) Decoding sequence progress from small neuronal subsets (N = 20) helped to identify the factors that influenced performance. (C) Illustration of subset analysis: Decoding was performed for the fixation period based on sets of 20 randomly selected neurons (illustrated in orange) for defined subpopulations, including (i) only neurons significant for sequence progress (‘Signif’; Equation 2); (ii) randomly chosen neurons irrespective of significance (‘Random’); (iii) only non-significant neurons (‘N-signif’). For each subset category, we performed 3000 iterations. (D) Performance from defined neuronal subsets (N = 20, orange bars) and from all neurons (N = 260, black bar). In all cases, decoding was significantly above chance. (E) Performance depended on individual neurons’ progress sensitivities. Linear regression (Equation 2) of performance from randomly selected subsets (‘Random’) on subsets’ mean progress slopes. (F) Comparison of sequence progress decoding for basolateral (N = 137) and centromedial (N = 123) amygdala neurons (mean ± s.e.m; *p<0.0001, Wilcoxon test). (G, H) Increases in decoding performance with number of basolateral and centromedial neurons in fixation (G) and cue (H) periods (*p<0.0001, Wilcoxon test). (I, J) Subset analysis for basolateral and centromedial neurons. Bars show regression coefficients obtained from regressing decoding performance on subsets’ mean rectified progress slopes, standard deviation (‘Std’) of progress slopes, and fraction of centromedial (‘CM’) neurons in the subset.
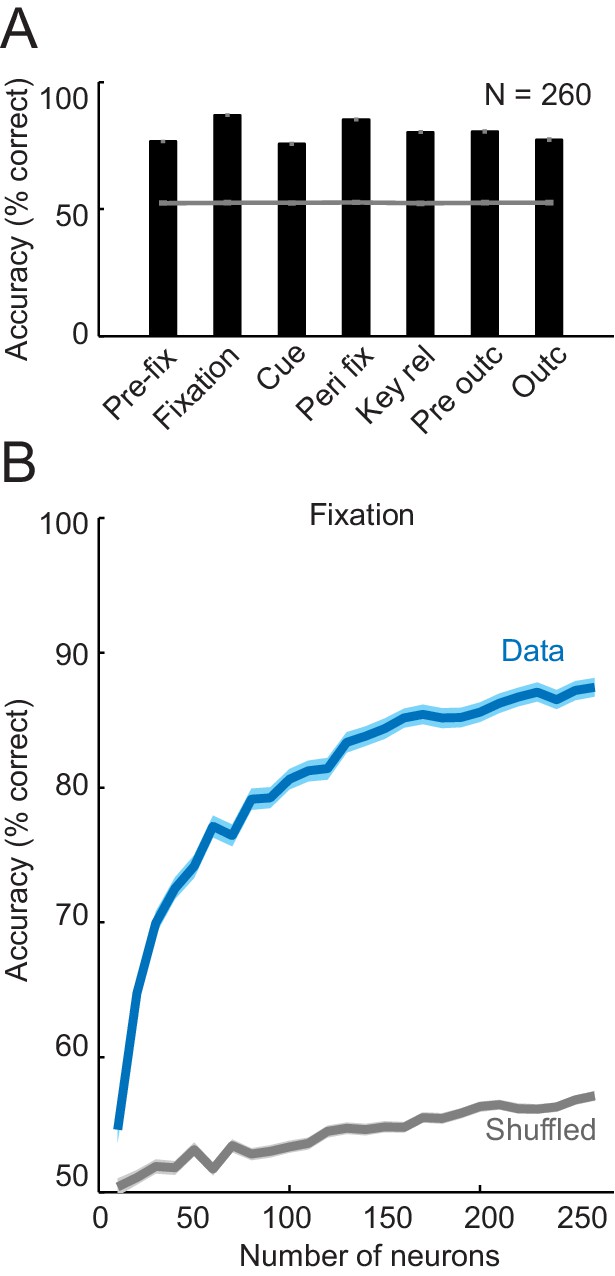
Population decoding of sequence progress using support vector machine classifier.
(A) Decoding accuracy (% correct classification). Leave-one-out cross-validated decoding was significantly above decoding from shuffled data (gray line; all p<0.0001; Wilcoxon test). Decoding was based on normalized single-trial activity of all neurons that met criteria of decoding analysis (N = 260) without pre-selection for task-relatedness. (B) Performance increased with the number of neurons, shown here for the fixation period. Data for each neuron number show mean (± s.e.m) over 100 iterations of randomly selected neurons.