GABA, not BOLD, reveals dissociable learning-dependent plasticity mechanisms in the human brain
Figures
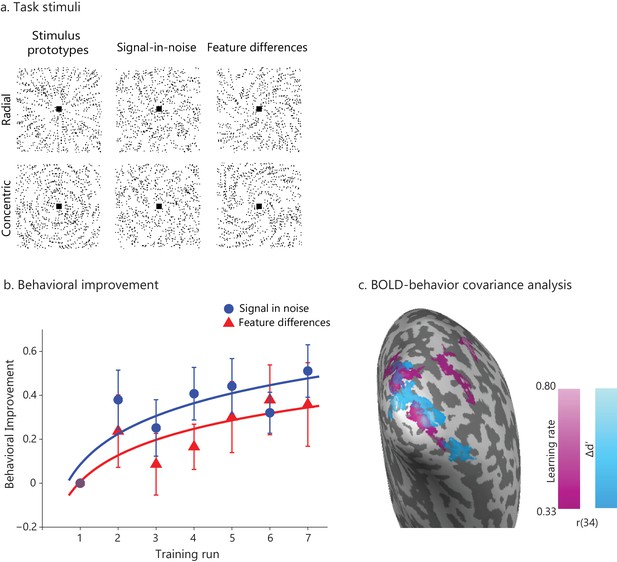
Learning-dependent changes in behavior and brain activation.
(a) Stimuli: Example stimuli comprising radial and concentric Glass patterns. Stimuli are shown for the Signal in noise task (25% signal, spiral angle 0° for radial and 90° for concentric) and the Feature-differences task version (100% signal, spiral angle 38° for radial and 52° for concentric). Prototype stimuli (100% signal, spiral angle 0° for radial and 90° for concentric) are shown for illustration purposes only. (b) Behavioral improvement during training: mean d’ per training run normalized to d’ in the first run. Data were fitted with a logarithmic function; error bars indicate standard error of the mean across participants. The trend of higher performance in the SN than the FD task was not statistically significant. No significant improvement was observed for a no-training control group who did not receive training in between test sessions (Figure 1—figure supplement 1). (c) Whole-brain covariance analyses (cluster threshold corrected, p<0.05) with either learning rate (magenta) or Δd’ (blue) on fMRI data (first two runs vs. last two runs) that were pooled across the two tasks showed positive significant clusters in the posterior occipito-temporal cortex. Activations are shown on the cortical surface of the right hemisphere (sulci are shown in dark grey, gyri in light grey). The color bar indicates Pearson’s r correlation values. Figure 1—figure supplement 3 illustrates the relationship between BOLD change extracted from this region and measures of behavioral improvement (learning rate, Δd’) per task. Significant activations were observed in bilateral occipito-temporal cortex and fronto-parietal regions (Figure 1—source data 1). Further, GLM analysis of the fMRI data across training runs showed significant changes in occipito-temporal BOLD for both tasks (Figure 1—figure supplement 2). For all figures, data are included for the same training duration across participants (i.e. seven runs), as several participants (n = 9) were missing data from the eighth run. Including data from participants that were trained for an additional eighth run (Figure 1—figure supplement 4a) showed similar results as the analysis including seven training runs from all participants; that is, the whole brain covariance analysis of BOLD change with behavioural improvement showed similar activation maps (Figure 1—figure supplement 4b,c).
-
Figure 1—source data 1
Tables for whole brain GLM covariance analysis of BOLD with behavioral improvement.
- https://doi.org/10.7554/eLife.35854.008
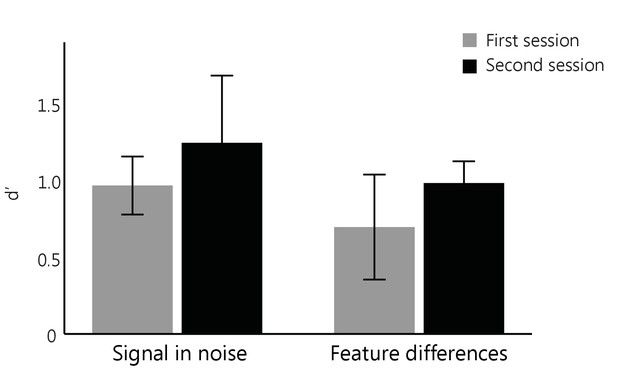
No training control group.
We tested a no-training control group (n = 8) who did not receive training in between two test sessions on consecutive days. We found no significant improvement in participant performance (as measured by d’) for neither task (main effect of Session: F(1,6)= 1.13, p=0.33; Task x Session interaction: F(1,6)=0.0003, p=0.99).
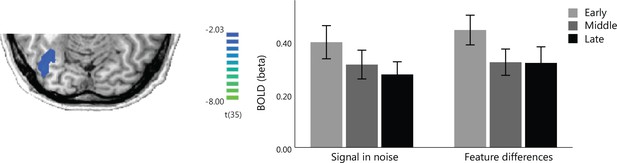
BOLD changes during training.
GLM analysis of the fMRI data across all training runs within the MRS mask showed significant BOLD changes in occipito-temporal cortex during training across tasks. Bar-plots show BOLD signal (percent signal change) in occipito-temporal cortex across runs; mean data are plotted during training: early (first two training runs), middle (middle three training runs), late (last two training runs) for the two tasks; error bars indicate standard error of the mean across participants.
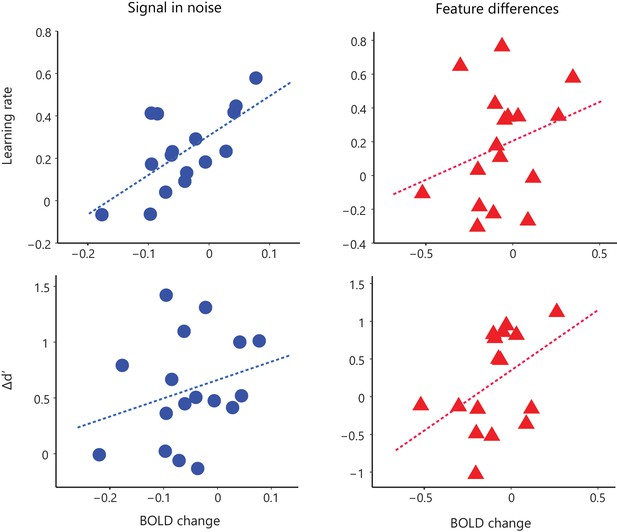
Relating BOLD change to behavioral improvement.
We extracted BOLD from voxel clusters in occipito-temporal region that showed significant correlations of behavioral change with BOLD change (i.e. covariance analysis, Figure 1c) and plotted BOLD change (late vs. early training runs) against behavioral improvement (learning rate, Δd’) across participants for each task (SN, FD). These plots show similar learning dependent changes in behavioral performance and fMRI activation during training in both learning tasks. Note, that these plots are for illustration purposes only; no further statistics were conducted on these signals as the fMRI activations were derived from a whole-brain covariance analysis of BOLD with behavioral improvement (Figure 1c).
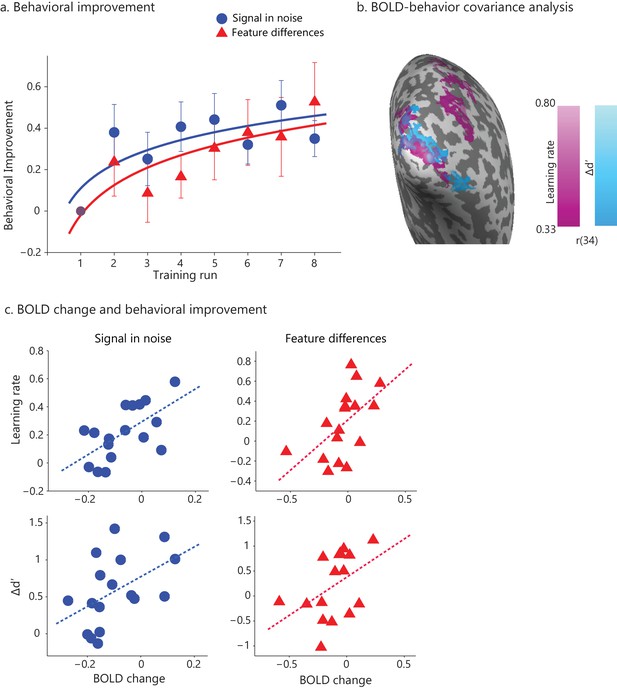
Behavior and brain imaging analyses including data from the eighth training run.
We included data from participants that were trained for an additional eighth run in the analyses for both the behavioral and brain imaging data. All analyses showed similar results, as the analyses including seven training runs from all participants (Figure 1b,c) (a) Behavioral improvement during training: mean d’ per training run normalized to d’ in the first run. Data were fitted with a logarithmic function; error bars indicate standard error of the mean across participants. No significant differences between the two tasks were observed for learning rate (t(34)=0.09, p=0.929) nor Δd’ (t(34)=0.14, p=0.886). (b) Whole-brain covariance analyses with either learning rate (purple) or Δd’ (blue) on fMRI data that were pooled across the two tasks showed positive significant clusters in the posterior occipito-temporal cortex. Activations are shown on the cortical surface of the right hemisphere (sulci are shown in dark grey, gyri in light grey). The color bar indicates Pearson’s r correlation values. (c) We then extracted BOLD from the occipito-temporal region revealed by the covariate analysis and plotted BOLD change against behavioral improvement (learning rate, Δd’) across participants for each task (SN, FD). Note, that these plots are for illustration purposes only; no further statistics were conducted on these signals as the fMRI activations were derived from a whole-brain covariance analysis of BOLD with behavioral improvement (Figure 1—figure supplement 4b).
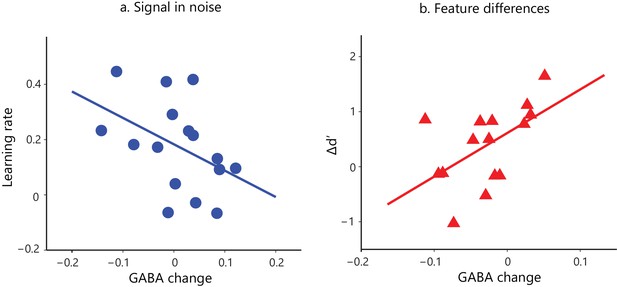
Task-dependent correlations of GABA change and behavioral improvement.
We measured MRS GABA in the posterior occipito-temporal cortex (Figure 2—figure supplement 1). We pre-processed and fit the data with ProFit (Schulte and Boesiger, 2006). We excluded three participants (FD task) due to fat contamination in the spectra (Figure 2—figure supplement 2). We did not observe significant differences in mean GABA concentration in occipito-temporal cortex before vs. after training (Figure 2—figure supplement 3). Here, we show skipped Pearson’s correlations indicating (a) a significant negative correlation of GABA change in occipito-temporal cortex with learning rate for the Signal-in-noise task (r = −0.43, CI=[−0.74,–0.07]) and (b) a significant positive correlation with Δd’ for the Feature-differences task (r = 0.54, CI=[0.05,0.85]). Correlations of GABA change with Δd’ for the Signal-in-noise task or learning rate for the Feature-differences task were not significant (Figure 2—figure supplement 4a). Negative learning rate or negative Δd’ represents decreased sensitivity during training. As participants were trained only for a single training session and without trial-by-trial feedback, these measures may be noisier and result in negative values. Correlations of GABA change with behavioral improvement remained significant when we removed data from participants with negative learning rate or Δd’. Further, including data from participants that were trained for an additional eighth run showed similar results: the correlations of GABA change with behavioral improvement (learning rate computed with eight training runs; Δd’ (last vs. first training run) remained significantly different (Fisher’s z = 2.4, p=0.02) between tasks (Figure 2—figure supplement 4b).
-
Figure 2—source data 1
GABA change, learning rate and Δd' per participant.
- https://doi.org/10.7554/eLife.35854.014
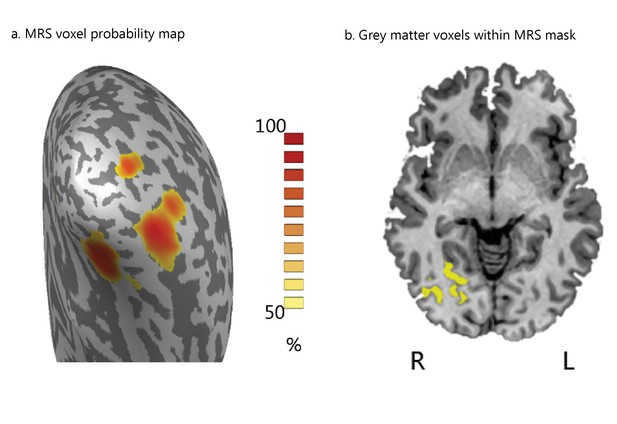
MRS voxel placement.
(a) MRS voxels were placed in the posterior occipito-temporal cortex. Anatomical landmarks (Middle Occipital Gyrus, Superior Temporal Gyrus) were used to guide consistent voxel placement across participants. The color bar indicates the probability of overlap across participants for each 1 × 1×1 mm anatomical voxel included in the MRS voxels. The figure highlights the grey matter voxels on the cortical surface that are present in at least 50% of the participants MRS masks. (b) Grey matter voxels included within the MRS mask, shown on an axial brain slice. When these clusters are mapped on the cortical surface (a) they correspond to separable regions in the lateral and medial occipito-temporal cortex–as the voxel overlap is thresholded between 50% and 100%, extending both anteriorly and posteriorly from the center of the MRS voxel. Despite this cautious voxel placement for each individual participant, there was some variability in the voxel location across participants due to the variability in the shape of the skull and brain morphology (e.g. extent of ventricles posteriorly; extent of the cerebellum superiorly). To control for potential variability of the MRS voxel placement within and between participants, across MRS blocks, we extracted the Talairach coordinates of each participant’s pre- and post-training MRS acquisition voxels. The mean difference in voxel placement within participants was minimal (x = 0.60 mm; y = 0.40 mm; z = 0.52 mm). Only for one participant (Signal-in-noise task) was the difference in the MRS voxel position before and after training larger than two standard deviations above the mean; data for this participant were excluded from further analyses. The following analyses showed that the variability in voxel placement across participants was not statistically significant: a) a multivariate ANOVA showed no significant group differences in MRS voxel positioning before (X: F(1,34)= 0.003, p=0.95; Y: F(1,34)= 0.834, p=0.37; Z: F(1,34)= 1.482, p=0.23) or after (X: F(1,34)= 0.039, p=0.85; Y: F(1,34)= 0.027, p=0.87; Z: F(1,34)= 1.899, p=0.18) training, b) all voxels were within three standard deviations of the average Euclidean distance (5.6 ± 2.5 mm) from the mean coordinates (x = 29.6 ± 3.44 mm, y = -67.3 ± 4.3 mm, z = 3.6 ± 2.3 mm) across participants, c) the average Euclidean distance from the mean MRS voxel coordinates did not differ significantly between tasks (t(34)=0.35, p=0.74).
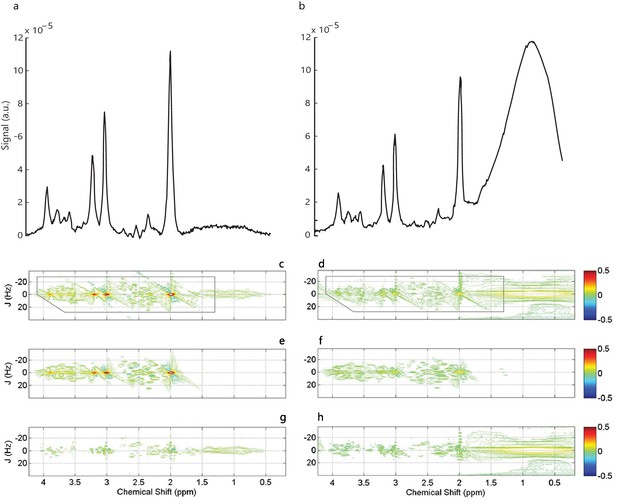
Fat contamination.
In healthy tissue, fat contamination is caused by extracranial lipids when the MRS voxel is placed near the skull, compromising metabolite quantification. We used two empirical criteria to estimate contamination, following previous studies (Schmitz et al., 2017). First, we identified the resonance frequency of the largest peak in each spectrum. This is expected for NAA at 2ppm (a). If the largest peak was observed at a resonance frequency different from 2ppm (b), this indicated that the spectrum was contaminated. Second, we confirmed this contamination by visually inspecting the output of the MRS processing software (Profit, Schulte and Boesiger, 2006) that included two-dimensional spectra (c,d), their fits (e,f) and residual plots (g,h) for each measurement. The data are plotted as contours where closed loops at fixed heights indicate equal signal intensity (Stagg, 2014). The J-resolved dimension (Hz) (y-axis) is plotted against the chemical shift dimension (ppm; x axis). The logarithmic color-scale represents the range of chemical compound concentrations. Only the real part of the phased spectrum is shown, resulting in both positive and negative values (Schulte and Boesiger, 2006). The largest peak of the spectrum is NAA at 2ppm and is indicated by contours colored with the highest values of the color scale (red). Fat contamination results in a noisy spectrum (d) with large residuals (h), indicating a poor fit.
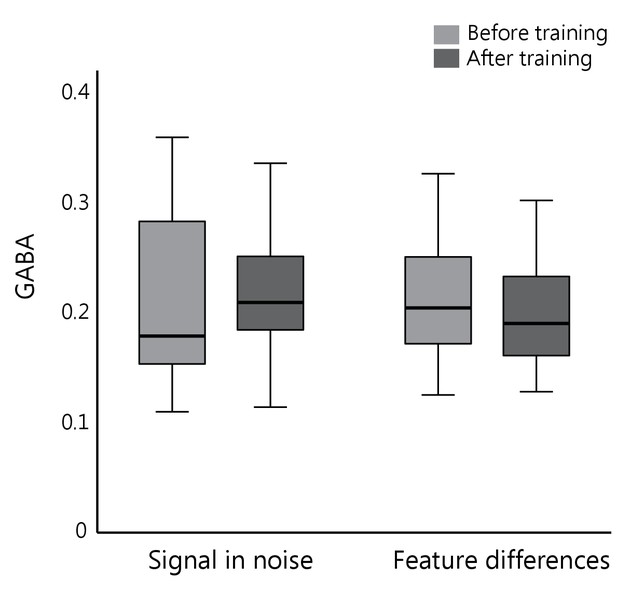
GABA concentration before vs. after training.
No significant differences were observed in GABA concentration in occipito-temporal cortex across participants before vs. after training (main effect of MRS block: F(1,34)= 0.06, p=0.81; Task x MRS block interaction: F(1,34)= 0.21, p=0.65). Boxplots denote median and interquartile ranges; indicating variability in GABA concentration across participants.
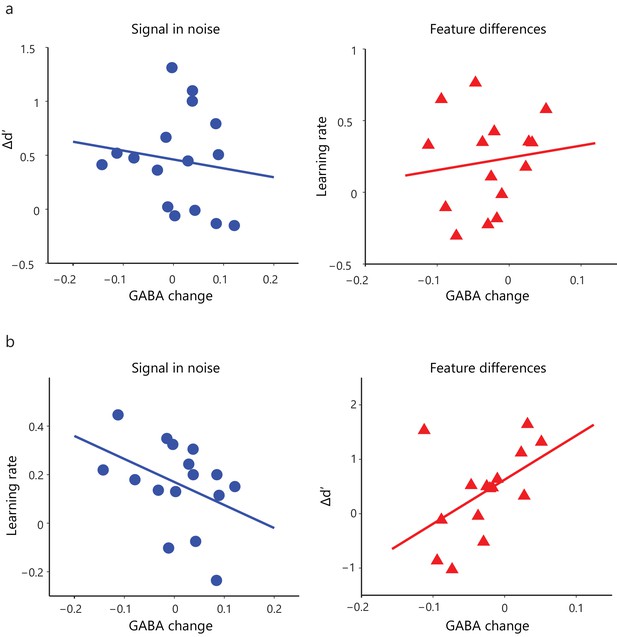
Correlations of GABA change and behavioral improvement.
(a) For the Signal in Noise task, the correlation of GABA change with Δd’ was not significant (r = −0.14, CI=[−0.49, 0.29]) and was not significantly different from the correlation of GABA change with learning rate (Steiger’s z = 1.25, p=0.21). For the Feature differences task, the correlation of GABA change with learning rate was not significant (r = 0.13, CI=[−0.38, 0.62]) but was significantly different from the correlation of GABA change with Δd’ (Steiger’s z = 2.27, p=0.02). (b) Correlations between GABA change and behavioral improvement (learning rate for SN; Δd’ for FD) remained significantly different between tasks (Fisher’s z = 2.4, p=0.02) when we included data from participants that were trained for an additional eighth run.
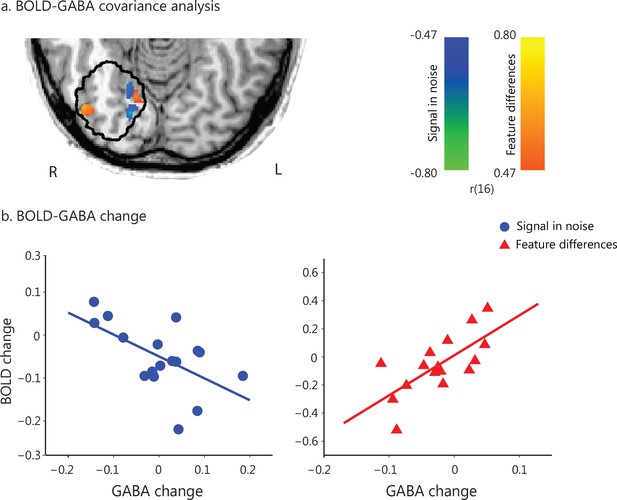
Task-dependent correlations of GABA change with BOLD change.
(a) GLM covariance analysis of GABA change with BOLD change within a masked region defined by the MRS voxel probability map (i.e. gray matter voxels within each participant’s MRS voxels with minimum 50% probability, as outlined in black). We used fMRI data (i.e. first two vs. last two fMRI runs) that were collected closer to the time when GABA was collected (before vs. after training). Activations are shown in radiological co-ordinates. GABA change correlated negatively with BOLD change for the Signal-in-Noise task (green to blue color bar), while positively for the Feature-differences task (orange to yellow color bar). The color bars indicate Pearson’s r. (b) Correlation of change in GABA and BOLD extracted from an independently defined region of interest; that is BOLD was extracted from the voxel clusters in posterior occipito-temporal cortex that resulted from the covariance analysis of fMRI with behavioral improvement (Figure 1c). This analysis showed opposite and significantly different correlations (SN: r = −0.58 CI=[−0.82,–0.22], FD: r = 0.70 CI=[0.37, 0.90], Fisher’s z = 4.19, p<0.0001) and corroborated the results shown in Figure 3a. Including data from participants that were trained for an additional eighth run showed similar results, as the analyses including seven training runs from all participants (Figure 3—figure supplement 1). In particular, a) the whole brain covariance analysis of BOLD change with GABA change showed similar activation maps (Figure 3—figure supplement 1a), b) the correlations between GABA change and BOLD change (extracted from the voxels activated in the independent covariance analysis with behavioral improvement, Figure 1—figure supplement 4b) remained significantly different between tasks (Fisher’s z = 3.26, p=0.001, Figure 3—figure supplement 1b). Finally, moderation analysis showed a significant interaction between GABA change, task and BOLD change (Figure 3—figure supplement 2).
-
Figure 3—source data 1
GABA change and BOLD change (from the voxel clusters in posterior occipito-temporal cortex, Figure 1c) per participant.
- https://doi.org/10.7554/eLife.35854.018
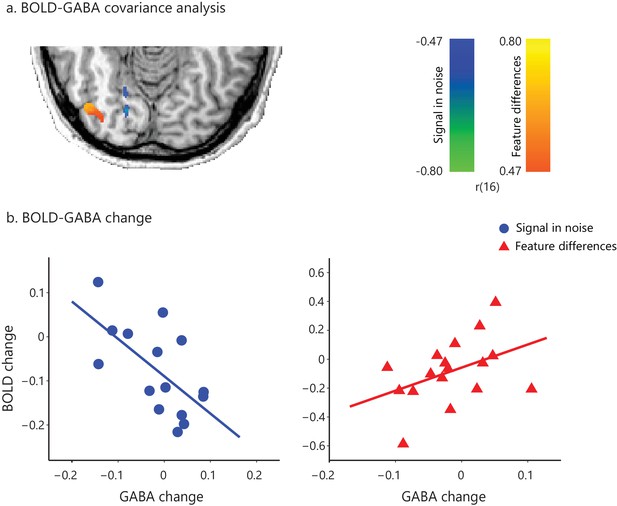
Correlations of GABA change and BOLD change for Signal-in-Noise vs Feature differences task including eighth training run.
Including data from participants that were trained for an additional eighth run resulted in similar results as data from seven runs across all participants: (a) the whole brain covariance analysis of BOLD change with GABA change showed similar activation maps with the analysis using seven training runs (Figure 3a), (b) the correlations between GABA change and BOLD change (extracted from the voxels activated in the independent covariance analysis with behavioral improvement, Figure 1—figure supplement 4b) remained significantly different between tasks (Fisher’s z = 3.26, p=0.001).
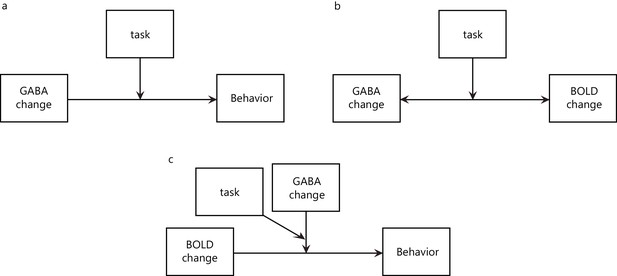
Task-dependent GABAergic plasticity moderates the relationship of functional brain plasticity and behavioral improvement.
We conducted moderation analyses to test whether task-dependent training moderates (a) the influence of GABA change on behavioral improvement and (b) the relationship between GABA and BOLD change. Model (a) was significant (F(3,27)=3.06, p=0.04), with a significant interaction between Task and GABA (F(1,27)=8.56, p=0.01; R-square change = 0.24), indicating that task-dependent training moderates the influence of GABA change on behavioral improvement. Model (b) was significant when the outcome variable was either GABA (F(3,29)= 6.28, p=0.002; Task x BOLD interaction: F(1,29)=16.27, p=0.0004; R-square change = 0.34) or BOLD (F(3,29)=8.55, p=0.0003; Task x GABA interaction: F(1,29)=24.58, p=0.00003; R-square change = 0.45), indicating that task-dependent plasticity moderates the relationship between change in GABA and BOLD. Finally, to test whether GABA, as moderated by task-dependent training, moderates the influence of BOLD on behavioral improvement, we tested a moderated moderation model (c). This analysis allowed us to test whether the influence that an independent variable (i.e. BOLD) has on the outcome (i.e. behavior) is moderated by one or more moderator variables (i.e. GABA, task). We found that this model was significant (F(7,28)= 3.77, p=0.01) and the interaction between Task, GABA and BOLD was significant (F(1,28)= 7.17, p=0.01; R-square change = 0.13). This result suggests that task-dependent GABAergic inhibition moderates the influence of functional brain plasticity on behavioral improvement. We further tested a model with Task and BOLD as moderators of the influence of GABA on behavior. This model was significant (F(5,30)= 3.26, p=0.02); however the interactions between GABA, Task and BOLD were not significant; that is, i) GABA x Task: F(1,30)=0.04, p=0.85; ii) GABA x BOLD: F(1,30)=0.02, p=0.89, suggesting that BOLD does not significantly moderate the relationship between GABA change and behavioral improvement.
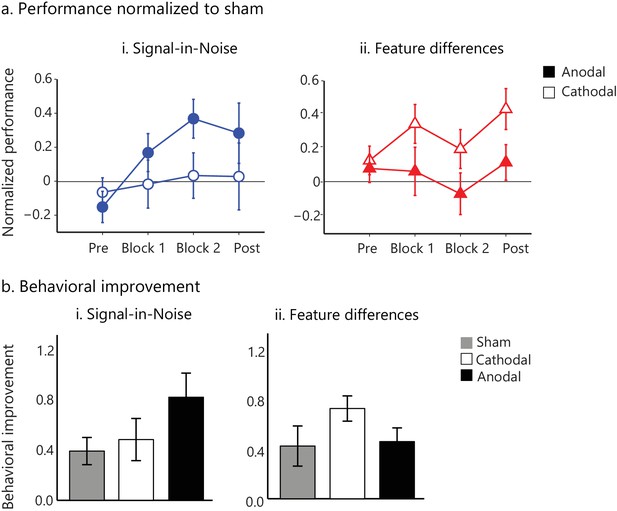
tDCs intervention facilitates visual learning.
(a) Task performance (d') for the active stimulation groups (anodal, cathodal tDCS on posterior occipito-temporal cortex (Figure 4—figure supplement 1)) normalized to the sham group for the pre- and post- training blocks (no feedback, no stimulation) and the two training blocks (Block 1, Block 2; 500 trials per block). Performance (d') was significantly enhanced for anodal (but not cathodal) stimulation in the Signal-in-Noise task, while for cathodal (but not anodal) stimulation in the Feature differences task. (b). Behavioral improvement (d’ post- minus pre-training) was enhanced for the anodal stimulation group in the Signal-in-Noise task and the cathodal stimulation group for the Feature differences task. Error bars indicate standard error of the mean across participants.
-
Figure 4—source data 1
Performance (d') normalized to the sham group for each participant of the anodal and cathodal groups for the two tasks.
- https://doi.org/10.7554/eLife.35854.021
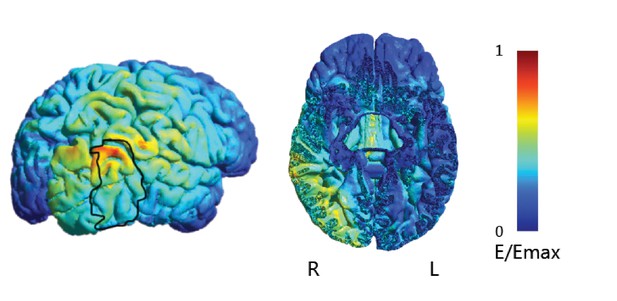
tDCs electric field simulation.
Electrical field density simulation (shown on the cortical surface and a representative axial slice) showed that the current density was largely unilaterally localized, the peak of the electric field density was observed under the anode electrode around the posterior occipito-temporal cortex) and the stimulation reached the occipito-temporal region where the MRS voxel was placed. Heatmap indicates electric field strength from 0 (blue) to maximum (red). The black outline indicates mean activation across participants (n = 33) for an independent functional localizer scan (i.e. activation for intact vs. scrambled images of objects) that has been extensively used to identify regions in the posterior occipito-temporal cortex that are involved in shape processing (Kourtzi and Kanwisher, 2001). The figure illustrates substantial overlap between the tDCs electric field and regions in the posterior occipito-temporal cortex involved in shape processing. The exact mechanism by which tDCs alters cortical excitability remains debated: while some studies suggest that the electric field reaching the cortex in standard tDCs protocols is too weak to alter neuronal firing rate (Vöröslakos et al., 2018), other studies have shown that LFP power and coherence (Krause et al., 2017) are modulated with tDCs intensities between 1 and 2mA. Further, in vivo studies in humans have shown that lower current intensity of 1-2mA, similar to that used in our study, can induce voltage gradients that may still affect cells with an appropriate orientation relative to the applied field or already depolarized cells (Opitz et al., 2016; Widge, 2018).
Additional files
-
Transparent reporting form
- https://doi.org/10.7554/eLife.35854.022