Genome-wide quantification of the effects of DNA methylation on human gene regulation
Figures
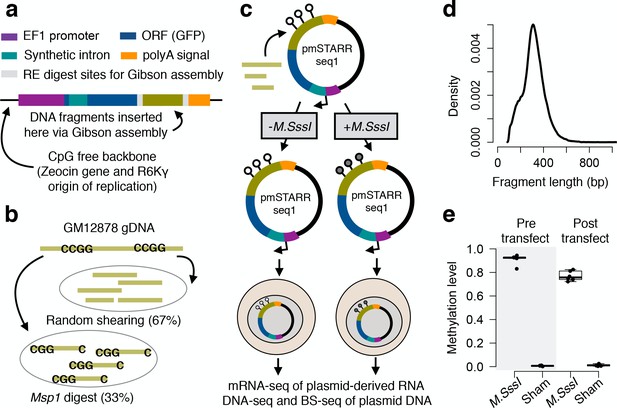
mSTARR-seq experimental design.
(A) The CpG-free pmSTARRseq1 vector is designed so that functional regulatory elements self-transcribe to produce a processed mRNA transcript, including a transcribed region (dark blue) that spans a synthetic intron (teal) and the sequence of the regulatory element itself (green). (B) GM12878 DNA was fragmented through random shearing or Msp1 digest, which recognizes the sequence CCGG. The resulting fragments were mixed in a 2:1 ratio, and (C) cloned into pmSTARRseq1 in high-throughput. We subjected the resulting library to either experimental methylation (M.SssI treatment) or a sham treatment, and transfected each pool into the K562 cell line (n = 6 replicates per condition). After a 48 hr incubation period, plasmid DNA and plasmid-derived mRNA were extracted and the variable insert regions were sequenced. (D) Inserts were of mean size 321 bp ± 107 bp s.d. (E) Bisulfite sequencing of pre- and post-transfection plasmid DNA confirmed that M.SssI treatment successfully methylates CpG sites introduced into pmSTARRseq1 and that methylation status is not substantially perturbed by transfection (y-axis: mean CpG methylation level per experimental replicate, based on CpG sites in the region used for Gibson assembly, and therefore present on every plasmid). Whiskers on boxplots represent the values for the third and first quartiles, plus or minus 1.5 × the interquartile range, respectively. Evidence for lack of confounding by a transfection-induced type I interferon response is shown in Figure 1—figure supplement 1. An analysis of fragment diversity levels in mSTARR-seq DNA-seq and RNA-seq libraries is shown in Figure 1—figure supplement 2. A comparison of fragment diversity between this experiment and other high-throughput reporter assays, as well as the effect of repeated library transformation on diversity is shown in Figure 1—figure supplement 3. mSTARR-seq coverage by genomic compartment and ENCODE chromatin state annotations is shown in Figure 1—figure supplement 4.
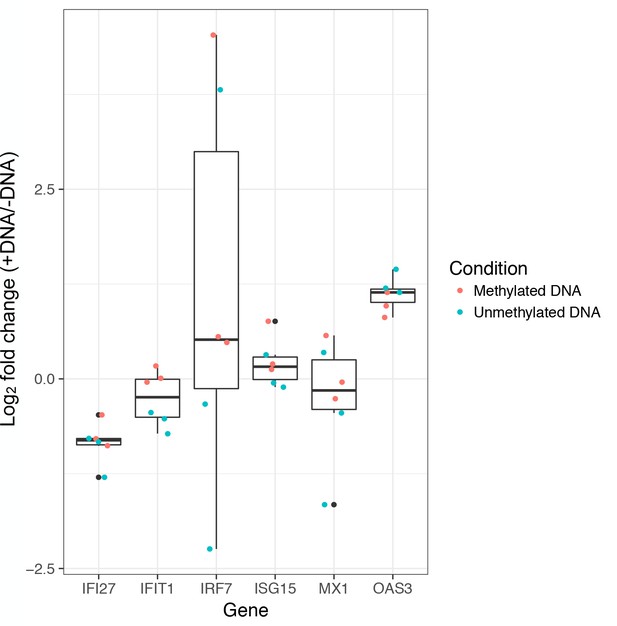
Plasmid transfection does not induce a strong type I interferon (IFN-I) response.
qPCR-based assessment (following (Muerdter et al., 2018)) of mRNA induction of six IFN-I response genes after transient transfection. These genes were chosen because they were initially used to identify a type I interferon response in STARR-seq data by Muerdter et al. (2018), and because each is a key player in cGAS/STING signaling, the primary avenue through which mammalian cells sense cytoplasmic DNA. Results are shown for experiments conducted using total RNA derived from K562 cells transfected with methylated or unmethylated pmSTARRseq1 plasmid pools (containing human DNA inserts). The y-axis represents the fold change in mRNA expression levels (on a log2 scale) between cells transfected with plasmid DNA (+DNA) relative to the mean levels observed for the same gene in untransfected cells (-DNA). Whiskers on boxplots represent the values for the third and first quartiles, plus or minus 1.5 × the interquartile range, respectively. Log2-fold changes did not significantly differ from 0 (one-sided Wilcoxon-signed rank test, p > 0.05) except for OAS3 (p = 0.047), and none of the genes was significantly affected after multiple hypothesis test correction. This result agrees with ENCODE RNA-seq data for K562s indicating inactivity of the cGAS/STING pathway.
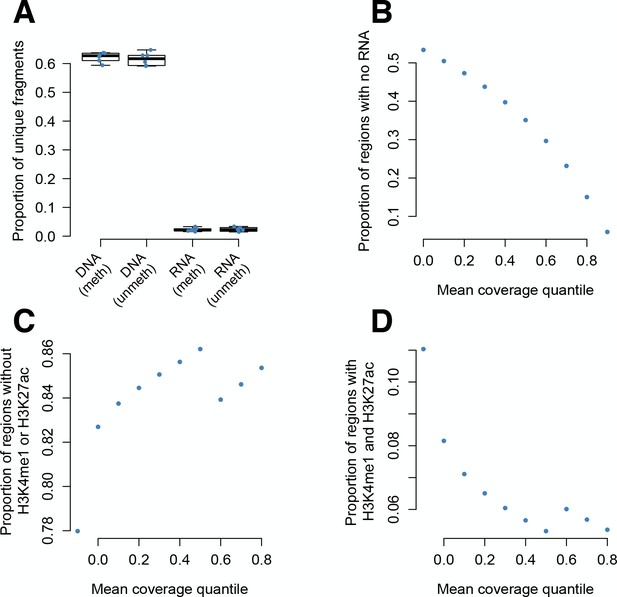
Diversity in plasmid DNA-seq libraries versus mRNA-seq libraries.
(A) As previously observed in STARR-seq experiments (Arnold et al., 2013), plasmid DNA libraries extracted from transfected K562 cells are more diverse than mRNA-derived libraries (y-axis: proportion of all sequenced fragments that had a unique start and end coordinate within a given replicate, for methylated and unmethylated DNA-seq and mRNA-seq libraries). Whiskers on boxplots represent the values for the third and first quartiles, plus or minus 1.5 × the interquartile range, respectively. (B) Most fragments that appear in DNA-seq libraries but not RNA-seq libraries were at low abundance in the plasmid DNA pool. Y-axis: the proportion of 200 bp regions with zero counts from all mRNA-seq libraries, binned by quantile of normalized mean DNA-seq coverage across all input libraries (x-axis). (C–D) For fragments that had zero counts from all mRNA-seq libraries, the proportion of regions that overlap chromatin states (C) lacking H3K4me1 and H3K27ac (indicating inactive regions) and (D) marked by H3K4me1 and H3K27ac (indicating active regions). Proportions are binned by quantile of normalized mean DNA-seq coverage across all input libraries (x-axis). DNA fragments that were input into the experiment at high abundance, but for which no mRNAs were observed, were more likely to come from endogenously inactive regions and less likely to come from endogenously active regions. This pattern suggests that lower fragment diversity in mRNA-seq libraries relative to DNA-seq libraries is not purely technical, but also influenced by the fragment’s regulatory potential. In B-D, results are shown for the unmethylated condition for simplicity (parallel analyses for the methylated condition yielded similar results).
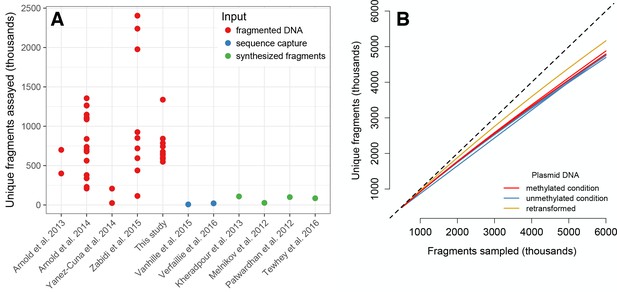
Fragment diversity in mSTARR-seq libraries is comparable to previous work, and this diversity can be easily regenerated across experiments.
(A) Y-axis depicts the number of unique plasmid-derived mRNA fragments reported in a given STARR-seq (Arnold et al., 2013; Arnold et al., 2014; Zabidi et al., 2015), MPRA (Melnikov et al., 2012; Patwardhan et al., 2012; Tewhey et al., 2016), or CAP-STARR (Vanhille et al., 2015; Verfaillie et al., 2016) experiment. Each dot represents a replicate transfection (in cases where data on individual replicates were available). Published STARR-seq, MPRA, or CAP-STARR experiments that did not report the number of unique plasmid-derived mRNA fragments per replicate are not included. (B) Retransforming a given plasmid pool results in almost no loss in diversity. We retransformed a small amount (<300 ng) of the plasmid pool described in the main text into 300 ul of electrocompetent GT115 E. coli, and sequenced the inserts from the retransformed sample. For a given number of sampled fragments (x-axis), the retransformed sample and the post-transfection DNA-seq plasmid libraries from the main experiment have a comparable number of unique fragments (defined as uniquely mapped fragments with unique start and end positions). Each solid line represents an individual replicate, and the dashed line shows the line where x = y. The retransformed plasmid DNA library appears slightly more diverse than the methylated and unmethylated condition libraries, presumably because (i) the methylated and unmethylated condition libraries were sequenced post-transfection (which likely results in some loss of diversity) and (ii) the retransformed plasmid was supercoiled DNA rather than ligation product, likely resulting in a more efficient transfection. Importantly, almost all (98.17%) of fragments observed in the retransformed library are also present in methylated and unmethylated condition libraries.
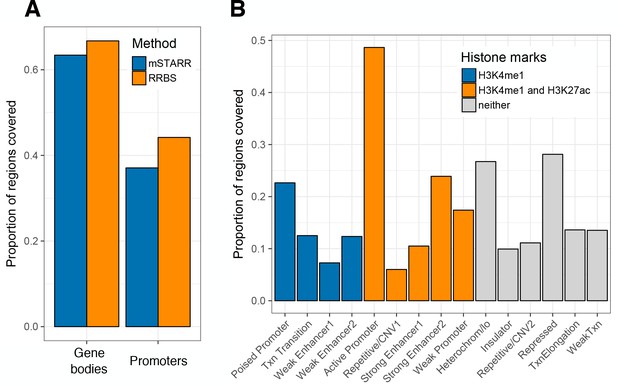
Regions covered by mSTARR-seq.
(A) The proportion of gene bodies (defined as the transcription start site (TSS) to the transcription end site) and promoters (defined as the 2 kb region upstream of the TSS) in the human genome that overlap mSTARR-seq fragments, compared to an in silico-generated reduced representation bisulfite sequencing (RRBS) library. A gene or promoter was considered to be ‘covered’ by a given data set if >1 bp overlapped a fragment from the data set. mSTARR-seq fragments provide similar coverage of genic regions relative to an RRBS library, and 57% of fragments expected from an in silico RRBS library are also found in our K562 mSTARR-seq experiments (93% of expected RRBS fragments are actually represented in the mSTARR-seq plasmid input library; those that are not represented in the final experimental analysis are therefore located in regions that are not consistently expressed in K562s: see also Figure 1—figure supplement 2). (B) The proportion of regions annotated in a given K562 ENCODE chromatin state that overlap mSTARR-seq fragments (y-axis and overlap are defined as in A).
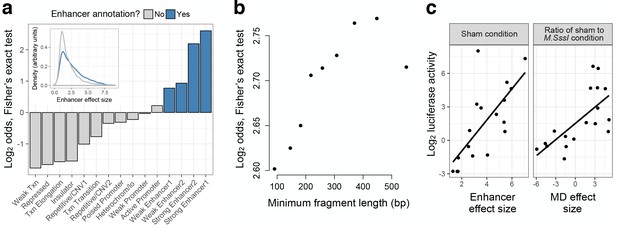
mSTARR-seq identifies regions with endogenous regulatory activity.
(A) Regions with significant regulatory activity are enriched for enhancer chromatin states in K562 cells (blue). Y-axis shows the two-sided Fisher’s Exact Test log2(odds) for enrichment/depletion of mSTARR-seq enhancers in 15 K562-annotated chromatin states (p<0.05 for all tests except ‘Weak promoter’). Inset: mSTARR-seq effect size for regions in enhancer chromatin states versus other chromatin states, for regions with significant activity only. (B) Binning regions with significant mSTARR-seq enhancer activity by fragment length reveals that larger fragments are more strongly enriched for ENCODE-annotated ‘strong enhancers’. The y-axis depicts the log2(odds) from a two-sided Fisher’s exact test for enrichment of mSTARR-seq enhancers (binned by deciles of fragment length) in either of the two ‘strong enhancer’ chromatin states (p<0.05 for all tests). (C) Agreement between conventional CpG-free luciferase reporter assays (Klug and Rehli, 2006) and enhancer activity (left) and MD-dependent activity (right) estimated from mSTARR-seq for 18 candidate regulatory elements (Supplementary file 3). mSTARR-seq activity explains 86.0% and 76.1% (p < 10−15) of variance in normalized luciferase activity, respectively (linear mixed model controlling for batch and assay fragment length). The dynamic range for mSTARR-seq enhancer detection is shown in Figure 2—figure supplement 1. The effects of sample size and sequencing effort on coverage, regions analyzed, and CpG sites analyzed are shown in Figure 2—figure supplement 2. Sensitivity versus specificity of enhancer detection as a function of fragment length is shown in Figure 2—figure supplement 3.
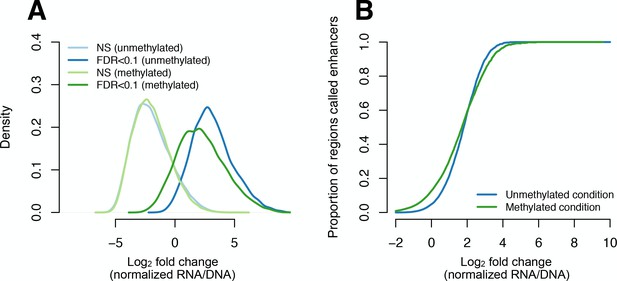
Effect size distributions for regions identified as enhancers (FDR < 0.1).
(A) Distributions of the mean log2 ratio of normalized plasmid mRNA gene expression levels to plasmid DNA input counts, for regions that exhibited significant (dark lines) versus non-significant (light lines) regulatory activity. Normalization was conducted using limma, and regulatory activity was assessed using linear models (as described in Materials and methods). Separate distributions are shown for methylated and unmethylated condition samples, respectively. (B) X-axis as in panel A, plotted against the proportion of regions that are called as significant enhancers as a function of effect size, for both methylated and unmethylated condition samples.
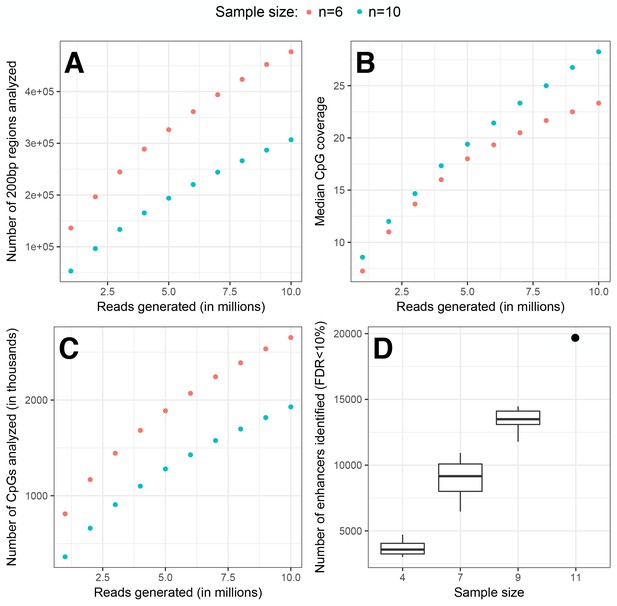
Effect of sequencing depth and sample size on the data set properties.
Using our main data set on MD regulatory activity, we randomly removed samples and reads to create new data sets with different properties. Data sets with a total of n = 6 and n = 10 samples include n = 3 and 5 replicates in each experimental condition, respectively, each with a fixed numbers of reads sampled for each mRNA-seq library (x-axis in A-C). As a function of RNA-seq reads generated (subsampled), we show: (A) the number of analyzable 200 bp regions based on the criteria reported in the main text (regions must be covered in >50% of both DNA and RNA samples in the data set); this has the effect of imposing a stricter threshold on larger data sets; (B) the median coverage in mRNA libraries for each CpG site included in analyzed regions; and (C) the total number of CpG sites included in analyzed regions. Each point shows the mean value across five random read subsets of the depth shown on the x-axis. As expected, more regions and CpGs are retained for analysis with increasing sequencing depth, but more regions are analyzed in a smaller data set (n = 6 versus 10 replicates), because of our requirement for a region to be covered in >50% of samples. However, smaller data sets suffer from reduced power. (D) The number of enhancers identified from a linear model (FDR < 10%) when we randomly remove replicates from our main data set; the dot at n = 11 is the number of identified enhancers reported in the main text.
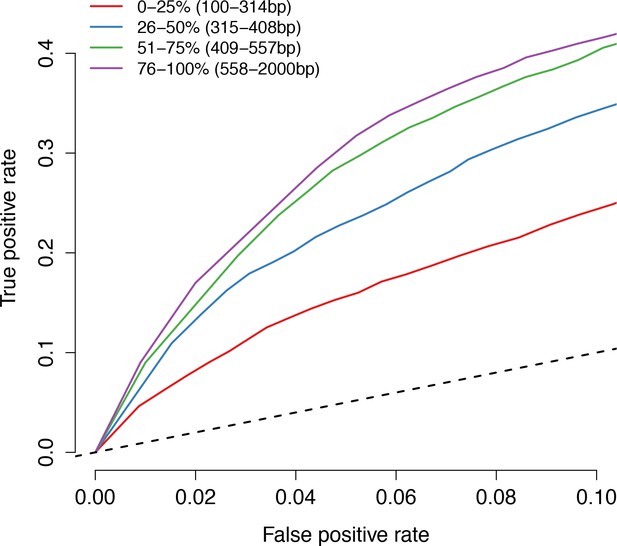
Known enhancers on larger DNA query fragments are more likely to exhibit significant regulatory activity in mSTARR-seq.
Lines show the trade-off between the false positive (x-axis) and true positive (y-axis) rates, for detecting ENCODE-annotated enhancers found on fragments of different lengths in unmethylated condition mSTARR-seq samples. Each line represents one quartile of the distribution of fragment lengths observed in our experiments: progressively better performance is obtained with progressively larger fragment sizes.
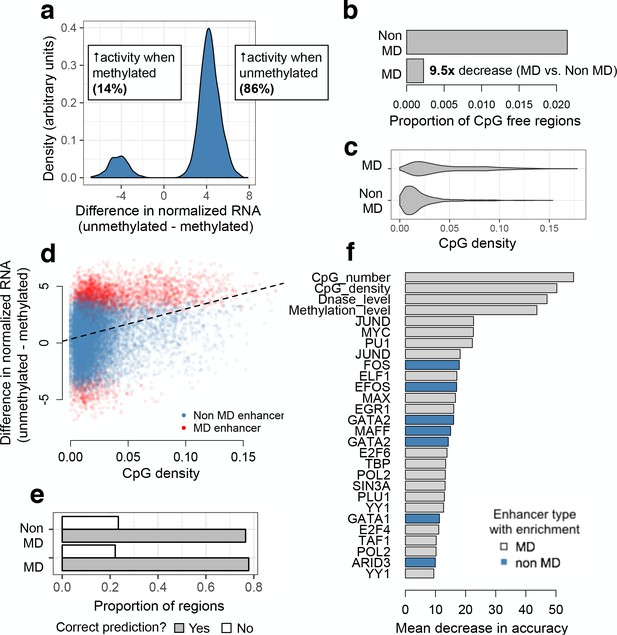
mSTARR-seq identification and prediction of MD enhancers.
(A) The distribution of differences in normalized mRNA transcript abundance between the unmethylated and methylated conditions for all significant MD enhancers. (B) CpG-free MD enhancers occur at a 9.5-fold lower rate than CpG-free windows with no MD enhancer activity. (C) Distribution of fragment CpG density for regions identified as MD versus non-MD enhancers. (D) CpG-dense mSTARR-seq enhancers tend to be repressed by DNA methylation (positive y-axis value; Spearman’s rho = 0.284, p<10−15; n = 19,703 regions with mSTARR-seq regulatory activity). (E) The proportion of non-MD and MD enhancers that were accurately classified via a random forests (RF) classifier. (F) Features that distinguish MD and non-MD enhancers in the RF classifier (10% FDR; note that the feature set [Supplementary file 6] includes some repeated ChIP-seq experiments for the same TF). X-axis: mean decrease in predictive accuracy when permuting the focal variable. Blue: positive prediction of non-MD enhancers; gray: positive prediction of MD enhancers. Mean decrease in accuracy is calculated as the mean difference in accuracy between 1000 models in which the focal variable is permuted and one where it is not, normalized by the standard deviation of these difference values; it is thus analogous to a standardized effect size. Statistical calibration and dynamic range of detection for models used to detect MD activity are shown in Figure 3—figure supplement 1. Effect of varying the threshold used to predict MD versus non-MD enhancers is shown in Figure 3—figure supplement 2. Motifs for TFs that are significant predictors of MD enhancer activity are shown in Figure 3—figure supplement 3.
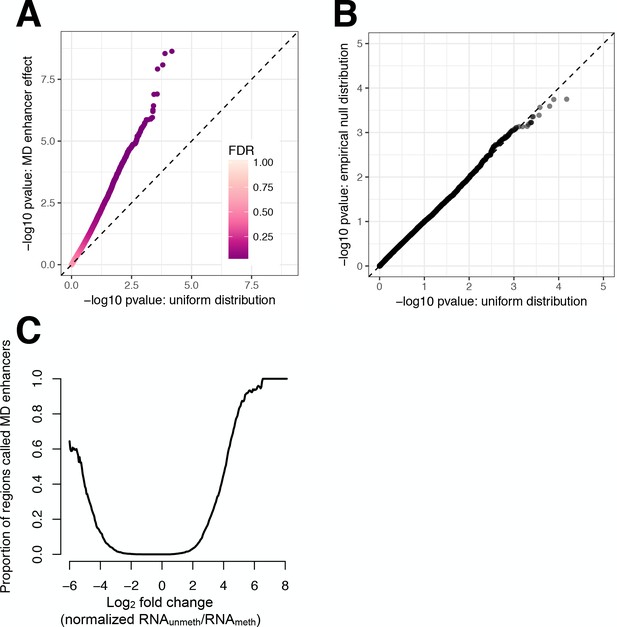
Linear models for detecting MD enhancers are well-calibrated, but primarily detect large effect size regions.
(A) QQ-plot comparing the distribution of -log10 p-values from a linear model for detecting MD enhancers (as described in Materials and methods) versus a uniform distribution expected under the null. Points are colored by their false discovery rate, assigned using qvalue. The plot highlights that many regions that do not pass our genome-wide significance threshold (FDR > 0.1), nevertheless, have low p-values and deviate from a uniform distribution, suggesting that there are many small effect size MD enhancers that we are unable to detect. (B) QQ-plot comparing the distribution of -log10 p-values for a uniform distribution versus the empirical null for the linear model used to identify MD enhancers (as described in Materials and methods). The empirical null was obtained by permuting the condition label for each sample (unmethylated/methylated) ten times, and extracting the p-values associated with the interaction effect used to detect MD enhancers. The empirical null and expected uniform distribution are very similar, with the empirical null slightly under-enriched for low p-values (KS test: D = 0.015915, p=0.003606). (C) Proportion of regions identified as MD enhancers, as a function of the mean log2 ratio of normalized plasmid mRNA from the unmethylated versus methylated conditions. Normalization was conducted using limma (as described in Materials and methods).
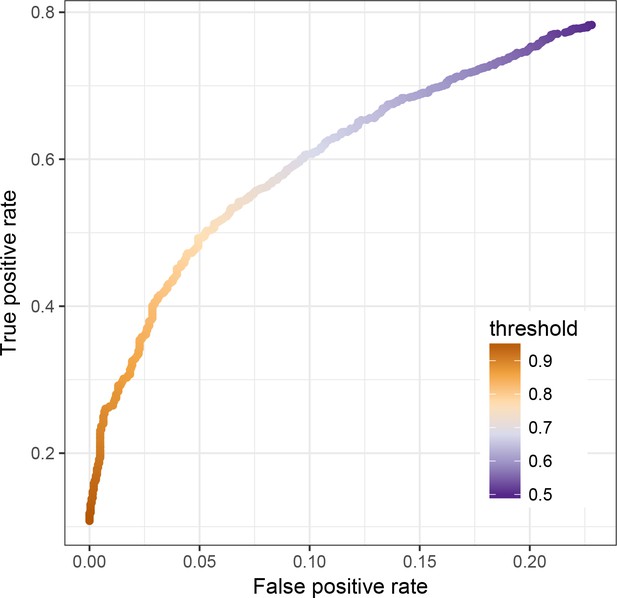
Impact of the threshold used to assign enhancers to the MD or non-MD class in random forests analyses.
In the main text, we report the proportion of regions that was correctly assigned by our random forests analysis as MD enhancers or non-MD enhancers. Each iteration of the random forest (n = 1000 iterations total) assigns a given region to a given outcome class, and we considered a given region to be assigned to a given outcome overall if the majority (>50%) of trees ‘voted’ for this class. Here, we show the false positive (x-axis) and true positive (y-axis) rates for the RF analysis if a threshold above 50% is used for assignment.
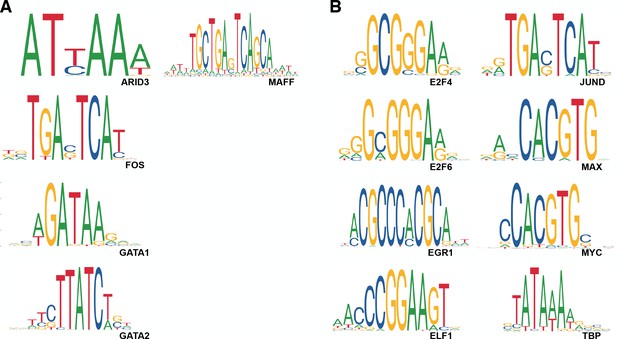
Motifs for transcription factors identified as predictors of MD regulatory activity in random forest analyses.
TFs enriched in the (A) non-MD class and (B) MD class are shown. All motifs were downloaded from JASPAR (Khan et al., 2018).
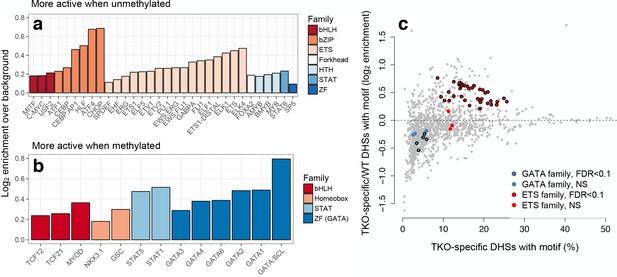
mSTARR-seq identifies MD transcription factor-DNA binding.
(A) Transcription factor motifs enriched in MD enhancers that are more active when unmethylated, colored by TF family. (B) TF motifs enriched in MD enhancers that are more active when methylated. (C) DNase hypersensitive sites (DHS) specific to murine stem cells that lack DNA methylation (DNMT triple knock-outs: TKO) are strongly enriched for ETS family binding sites relative to wild-type cells with intact DNA methylation. In contrast, DHSs specific to wild-type cells are enriched for GATA family binding sites relative to triple knock-outs. DHS data are from22. X-axis: % knockout-specific DHSs that contain a given TF-binding motif (n = 1251 motifs). Y-axis: Ratio of knockout versus wild-type specific DHSs containing a given TF-binding site motif. Colored dots circled in black show significant enrichment for an ETS or GATA family TF (10% FDR, hypergeometric test). Evidence for CpG site and TFBS-dependent convergence to endogenous K562 methylation levels for some tested sites is shown in Figure 4—figure supplement 1.
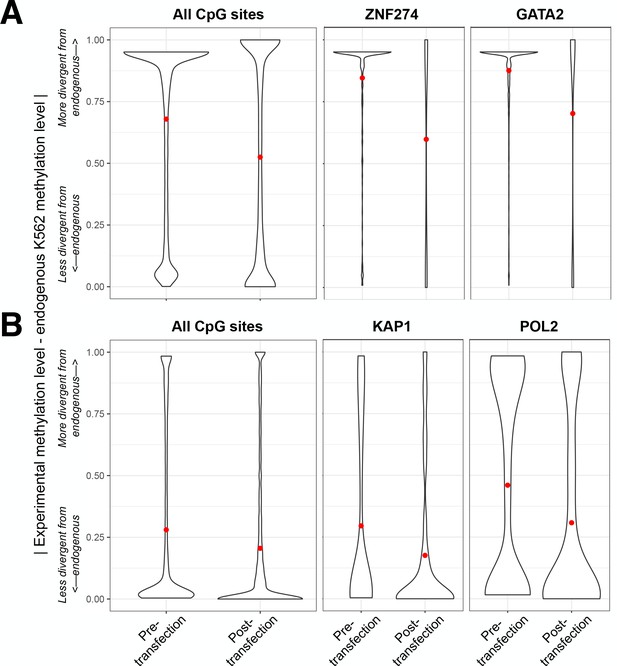
Methylation levels of human insert fragments in mSTARR-seq experiments converge toward endogenous methylation patterns post-transfection.
The absolute difference between the mSTARR-seq experimental methylation levels and endogenous methylation levels from whole genome bisulfite sequencing of K562 cells (y-axis) is shown for all measured CpG sites, as well as CpG sites in specific TFBS, pre- and post-transfection. Red points on the y-axis represent mean values, and comparisons are shown for methylated condition (A) and unmethylated condition (B) replicates seperately. TFs plotted are those associated with the strongest evidence for demethylation (A) or induction of methylation (B) post-transfection. The pre-transfection experimental methylation level for all CpG sites was estimated from CpG sites introduced during Gibson assembly (as these sites are almost never the targets of post-transfection methylation changes); post-transfection experimental methylation levels were estimated from locus-specific bisulfite sequencing data.
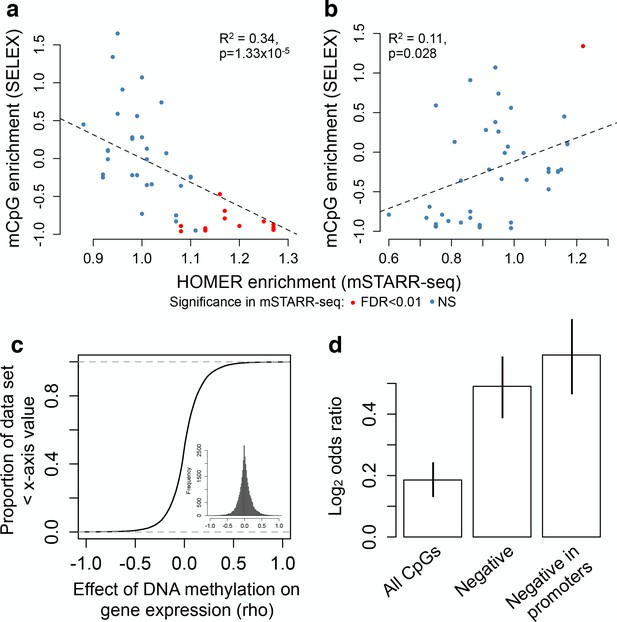
Agreement with in vitro and in vivo estimates of methylation-dependence.
(A–B) Comparison of motif enrichment for mSTARR-seq-identified MD enhancers (x-axis) versus affinity for methylated DNA in SELEX experiments (y-axis: higher values represent preferential binding to methylated DNA). Results are plotted for each TF tested in both mSTARR-seq and in Yin et al. (2017) (A) TFs enriched for MD enhancers that were more active when unmethylated. (B) TFs enriched for MD enhancers that were more active when methylated. Each TF is colored by whether its binding motif was significantly enriched in the given MD enhancer set in mSTARR-seq. (C) CDF of the effect of CpG methylation levels on gene expression levels (rho) for 32,843 gene pairs measured in 1202 human monocyte samples (Reynolds et al., 2014). The mean correlation between CpG methylation levels and the expression of the closest gene is near zero. Inset shows the same distribution plotted as a histogram. (D) Enrichment (Log2 odds ratio from a Fisher’s exact test) of CpG sites with significant DNA methylation-gene expression correlations (FDR < 10%) in MD enhancers relative to non-MD enhancers. Bars show (i) enrichment versus all CpG sites measured in both mSTARR-seq and the monocyte dataset; (ii) enrichment in the subset of CpG sites with negative DNA methylation-gene expression correlations (of any magnitude); and (iii) enrichment in the subset of CpG sites with negative DNA methylation-gene expression correlations located in gene promoters. Error bars represent 95% confidence intervals. Evidence that major environmental perturbations to gene expression (IFNA challenge) leads to correlated changes in DNA methylation is shown in Figure 5—figure supplement 1.
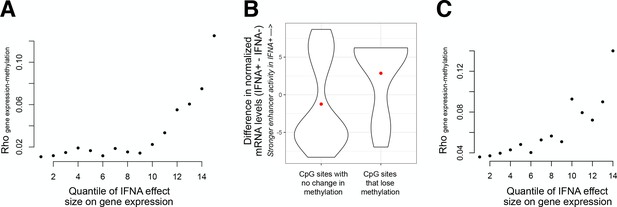
IFNA treatment produces changes in enhancer activity that correlate with changes in post-transfection plasmid DNA methylation levels.
(A) Y-axis: correlation coefficent for the relationship between IFNA-induced changes in enhancer activity and IFNA-induced changes in plasmid methylation levels. Correlations were estimated for the entire data set (n = 2678 200 bp regions), and for regions with progressively stronger evidence for IFNA-mediated induction of enhancer activity. Regions with larger IFNA effect sizes on enhancer activity (gene expression) also are more tightly correlated with mean changes in IFNA-induced DNA methylation levels: for the top 6.67% of effect sizes, rho = 0.125. Mean changes in IFNA-induced DNA methylation levels were estimated across all CpG sites measured in a given 200 bp region. (B) Data underlying the most extreme correlation estimate in A (rho = 0.125), when CpG sites in the 179 most IFNA-responsive regulatory elements are analyzed. Sites that lose DNA methylation after IFNA treatment tend to be located on fragments that specifically increase enhancer activity after IFNA treatment. (C) Same analysis and vizualisation as in panel A, but correlations were estimated between IFNA effect sizes on enhancer activity and changes in IFNA-induced DNA methylation levels for each CpG site individually (n = 7010 CpG sites; largest rho = 0.139).
Tables
Current methods for testing the causal relationship between DNA methylation and gene regulation.
https://doi.org/10.7554/eLife.37513.002Approach | Method | Throughput | Output |
---|---|---|---|
Episomal reporter | mSTARR-seq | Genome-scale (105–106 query fragments) | Expression |
Luciferase reporter assay (Klug and Rehli, 2006) | Single locus | Expression | |
Endogenous editing | TALE fusion to TET1 or DNMT3a effector domains (Maeder et al., 2013; Bernstein et al., 2015) | Single locus | Expression |
CRISPR-dCas9 fusion to TET1 or DNMT3a effector domains (Liu et al., 2016; Vojta et al., 2016) | Single locus | Expression | |
ZF domain fusion to DMNT3a or DNA glyocosylase effector (Rivenbark et al., 2012) | Single locus | Expression | |
Inducible overexpression of an artificial ZF-DNMT3A fusion protein (Ford et al., 2017)* | Genome-scale (104 query fragments) | Expression | |
In vitro TF binding | Electrophoretic mobility shift assay | Single locus | TF binding |
Protein-binding microarray (Mann et al., 2013) | Genome-scale (104–105 query fragments) | TF binding | |
DNA affinity purification sequencing (DAP-seq) (O'Malley et al., 2016) | Genome-scale (105–106 query fragments) | TF binding | |
Methylation sensitive and bisulfite-SELEX (Yin et al., 2017) | Genome-scale (104 query fragments) | TF binding | |
Methyl-Spec-seq (Zuo et al., 2017) | Genome-scale (102–103 query fragments) | TF binding |
-
*This method is currently limited to testing off-target effects in the MCF-7 cell line, which contains an inducible artificial ZF-DNMT3A fusion protein.
Reagent type (species)or resource | Designation | Source or reference | Identifiers | Additional |
---|---|---|---|---|
Cell line (human) | K562 | ATCC | ATCC CCL-243 | |
Recombinant DNA reagent | pmSTARRseq1 | Addgene | Plasmid #96945 | |
Peptide, recombinant protein | IFN-α | Abcam | ab48750 | |
Chemically competent E. coli cells | GT115 strain | Invivogen | ChemiComp GT115 | |
Electrically competent E. coli cells | GT115 strain | Intact Genomics | Custom order to grow Invivogen's ChemiComp GT115 strain and prepare cells for electroporation |
Additional files
-
Supplementary file 1
Table describing the samples sequenced as part of this study (contains source data for Figure 1E).
- https://doi.org/10.7554/eLife.37513.020
-
Supplementary file 2
Table showing that plasmid transfection does not induce a strong type I interferon responses in the K562 cell line (by testing GO enrichments of the strongest mSTARR-seq identified enhancers).
- https://doi.org/10.7554/eLife.37513.021
-
Supplementary file 3
Table of results from nested model testing for enhancer activity.
- https://doi.org/10.7554/eLife.37513.022
-
Supplementary file 4
Table of results from interaction model testing for MD enhancer activity (contains source data for Figure 3A).
- https://doi.org/10.7554/eLife.37513.023
-
Supplementary file 5
Table describing.
(A) luciferase reporter assay construct information and (B) luciferase reporter assay results (contains source data for Figure 2C).
- https://doi.org/10.7554/eLife.37513.024
-
Supplementary file 6
Table of random forests analysis results (contains source data for Figure 3F).
- https://doi.org/10.7554/eLife.37513.025
-
Supplementary file 7
Table of TF motif enrichment results for MD enhancers with greater activity in the unmethylated condition in the K562 cell line (contains source data for Figure 4A).
- https://doi.org/10.7554/eLife.37513.026
-
Supplementary file 8
Table of TF motif enrichment results for MD enhancers with greater activity in the methylated condition in the K562 cell line (contains source data for Figure 4B).
- https://doi.org/10.7554/eLife.37513.027
-
Supplementary file 9
Table of mean methylation levels for CpG sites in ChIP-seq identified TF binding sites.
- https://doi.org/10.7554/eLife.37513.028
-
Transparent reporting form
- https://doi.org/10.7554/eLife.37513.029