Predicting development of adolescent drinking behaviour from whole brain structure at 14 years of age
Figures

Preparation of AUDIT Sum-Scores for two-part latent growth mixture model.
Upper row: data from original scale (Sum Score), zeros are shown in black and indicate non-drinking individuals. Middle row: Transformation of data into consumer and non-consumer without fine-grading of alcohol use scores. Bottom row: Alcohol use score (AUDIT Sum-Score) for individuals who drink at all. Note that to enhance readability of the figure, sum-scales (upper and bottom row) are truncated at a score of 20.
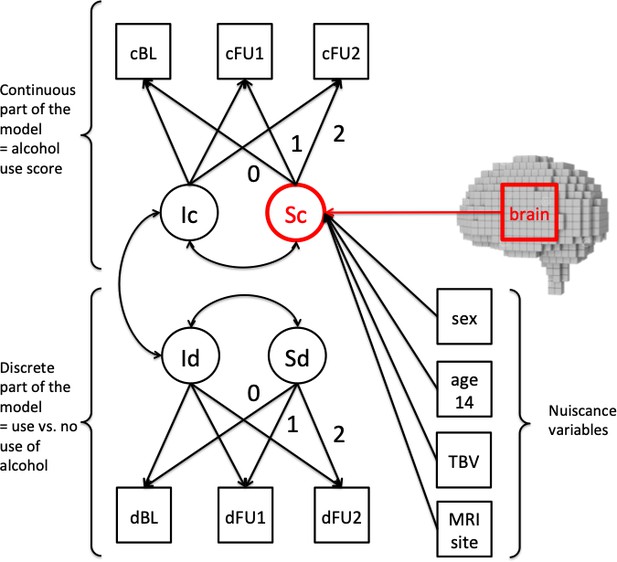
Two-part latent growth mixture model.
c = continuous, d = discrete, BL = baseline, FU = follow up, I = intercept, S = slope, TBV = total brain volume, MRI site was not a single indicator as depicted for reasons of simplicity, but consisted of 9–1 separate indicators dummy coding the different scanners used.
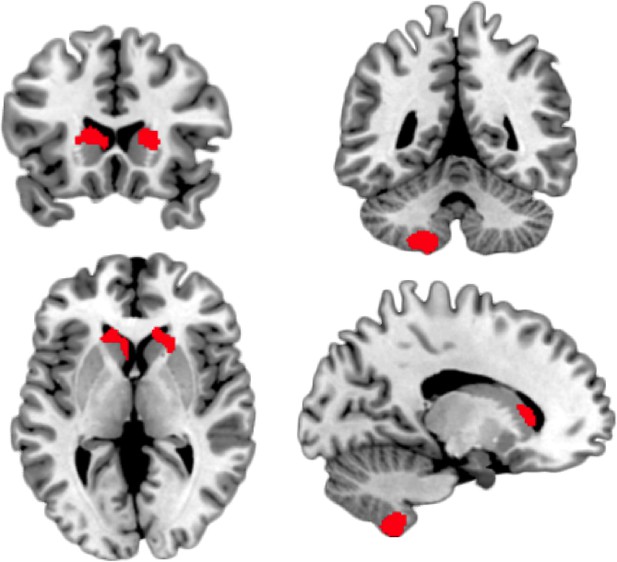
Brain regions showing a significant regression path from brain voxel to the latent slope of alcohol use score increase over time.
The higher the grey matter volume the larger the slope increase.
Tables
Severity of alcohol use at three measurement occasions according to AUDIT.*
https://doi.org/10.7554/eLife.44056.004Baseline n** = 1794 (100%) | Follow-up 1 n = 1439 (100%) | Follow-up 2 n = 1284 (100%) | |
---|---|---|---|
No use at all | 855 (47.7%) | 255 (17.7%) | 95 (7.4%) |
Unproblematic use | 872 (48.7%) | 961 (66.7%) | 823 (64.1%) |
Medium level of alcohol problems | 64 (3.6%) | 218 (15.3%) | 329 (10.7%) |
High level of alcohol problems | 2 (0.1%) | 4 (0.3%) | 28 (2.2%) |
Indicating dependence | 1 (0.1%) | 1 (0.1%) | 9 (0.9%) |
-
*Note: Categorization is based on the interpretation guideline of the World Health Organization: Cut-offs scores are: 0–7 = unproblematic use, 8–15: simple advice focused on the reduction of hazardous drinking warranted, 16–19: brief counseling and continued monitoring warranted, above 20: further diagnostic for alcohol dependence strongly warranted.
**Note: 20 individuals had missing data, in total adding up to 1814.
Estimated parameters in probability of use vs. non-use and alcohol use score with nuisance variables on the clinical data (not yet including brain data)
https://doi.org/10.7554/eLife.44056.005Intercept | Slope | |||
---|---|---|---|---|
Estimate | SE | Estimate | SE | |
Part 1: Prevalence of alcohol drinking (use vs. non-use)=discrete part of the model | ||||
Mean | 0.568** | 0.011 | 0.188** | 0.006 |
Variance | 0.090** | 0.009 | 0.024** | 0.004 |
Part 2: Alcohol use score of AUDIT = continuous part of the model | ||||
Mean | 0.693** | 0.037 | 0.498 | 0.642 |
Variance | 0.618** | 0.087 | 0.218** | 0.046 |
Regression onto Part two slope | ||||
Sex | −0.183** | 0.046 | ||
Age | −0.000 | 0.000 | ||
TBV | 0.000* | 0.000 | ||
Site_London | 0.410* | 0.163 | ||
Site_Nottingham | 0.368* | 0.161 | ||
Site_Dublin | 0.517* | 0.167 | ||
Site_Berlin | 0.091 | 0.170 | ||
Site_Hamburg | 0.122 | 0.162 | ||
Site_Mannheim | 0.038 | 0.163 | ||
Site_Paris | 0.079 | 0.163 | ||
Site_Dresden | −0.044 | 0.163 | ||
Covariances | ||||
Covariance between intercept and slope in Part 1 | −0.033** | 0.005 | ||
Covariance between intercept and slope in Part 2 | −0.078 | 0.050 | ||
Covariance between the intercepts of Part 1 and Part 2 | 0.124** | 0.012 |
-
*p < 0.05 , **p<0.001, SE = standard error, TBV = total brain volume
Additional files
-
Transparent reporting form
- https://doi.org/10.7554/eLife.44056.008