Gene-centric functional dissection of human genetic variation uncovers regulators of hematopoiesis
Figures
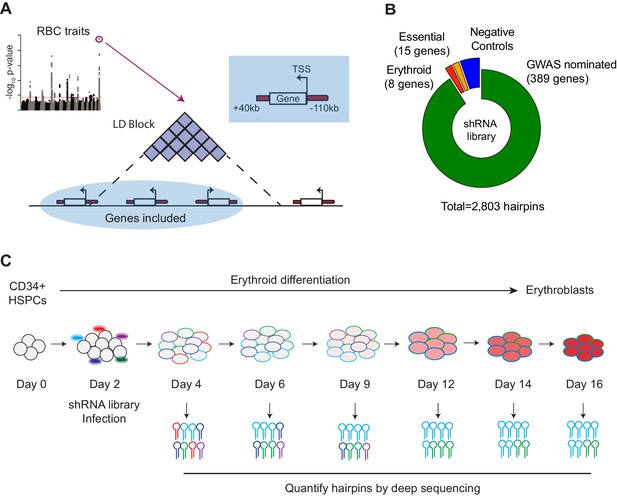
Design and Execution of an shRNA Screen Using Blood Cell Trait GWAS Hits to Identify Genetic Actors in Erythropoiesis.
(A) Overview of shRNA library design.75 loci associated with red blood cell traits (van der Harst et al., 2012) were used as the basis to calculate 75 genomic windows of LD 0.8 or greater from the sentinel SNP. Genes with a start site within 110 kb or end site within 40 kb of the LD-defined genomic windows were chosen as candidates to target in the screen. (B) Compositional makeup of the library, depicted as number of genes and number of hairpins for each of the four included subcategories; GWAS-nominated genes, erythroid genes, essential genes, and negative control genes (Figure 1—source data 2). (C) Primary CD34+hematopoietic stem and progenitor cells (HSPCs) isolated from three independent donors were cultured for a period of 16 days in erythroid differentiation conditions. At day 2, cells were infected with the shRNA library, and the abundances of each shRNA were measured at days 4, 6, 9, 12, 14, and 16 using deep sequencing.
-
Figure 1—source data 1
Table containing annotations and information for the 75 SNPs used to seed the shRNA library.
- https://doi.org/10.7554/eLife.44080.006
-
Figure 1—source data 2
Table containing annotations and information for all hairpins, as well as shRNA counts for each time point and replicate.
- https://doi.org/10.7554/eLife.44080.007
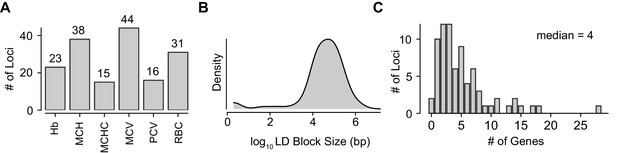
Characteristics of GWAS Loci and Gene Selection for Pooled Screen.
(A) Counts of loci from among the original 75 annotated with linkage to each of the six RBC traits, hemoglobin (Hb), mean corpuscular hemoglobin (MCH), mean corpuscular hemoglobin concentration (MCHC), mean corpuscular volume (MCV), packed cell volume (PCV), and red blood cell count (RBC).Some loci were associated with multiple traits. Detailed information on each loci available in Figure 1—source data 1. (B) Kernel density plot showing the log10 sizes in bp of the LD-defined genomic windows used to find overlapping genes. (C) Histogram showing distribution of number of genes selected using the LD window method at each locus. A median of 4 genes were present at each.
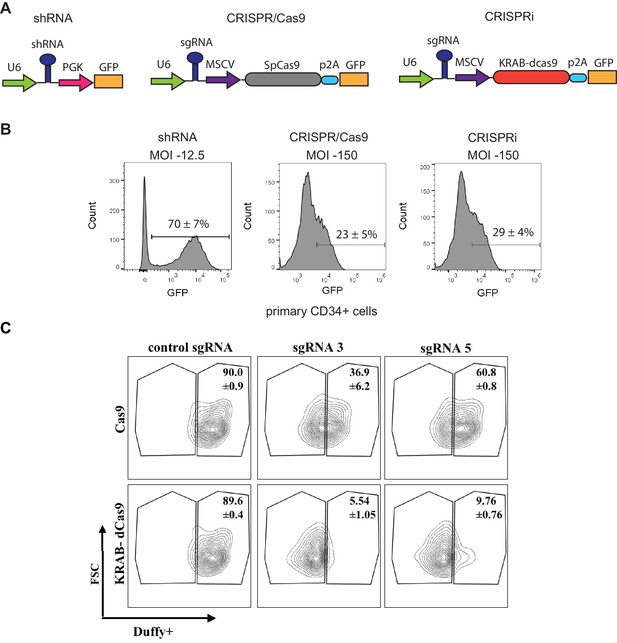
Feasibility of Loss of Function Approaches to Perform Pooled Screens in Primary Hematopoietic Stem and Progenitor Cells (HSPCs).
(A) Schematic of the loss of function lentiviral constructs tested for pooled screens in primary CD34+ cells. (B) FACS plots showing the proportion of infected GFP+ cells 4 days after transduction with the respective constructs (MOI -multiplicity of infection). FACS analysis was performed in independent analyzers. (C) Efficient silencing of Duffy surface antigen in primary CD34+ derived erythroid cells by targeting the promoter region using CRISPRi compared to CRISPR constructs.
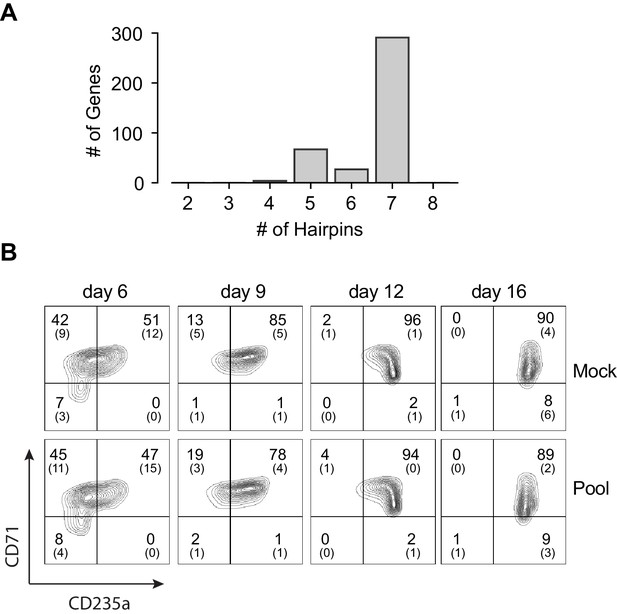
Pooled shRNA screen in primary HSPCs undergoing erythroid differentiation.
(A) Histogram showing distribution of number of independent hairpins included in the library to target each of the candidate’s genes. (B) Representative FACS plots of erythroid cell surface markers CD71 (transferrin receptor) and CD235a (Glycophorin A) expression at various time points during erythroid differentiation at which deep sequencing of shRNAs was performed. Percentages in each quadrant is represented as mean and standard deviation of 3 experiments from independent donors that were uninfected (Mock) or infected with the shRNA library (Pool).
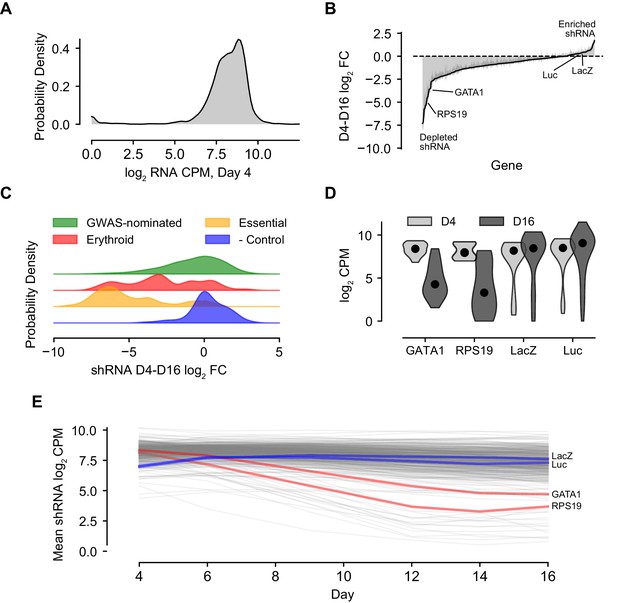
Summary Characterization of shRNA Screen Outcomes.
(A) Kernel density plot showing library representation as log2 shRNA CPM across all hairpins. (B) shRNA abundance log2 fold changes from day 4 to day 16. Represented values are the mean of hairpin abundance log2 fold changes across hairpins for each gene and two standard deviations. (C) Kernel density plots representing the day 4 to day 16 log2 fold changes of hairpin abundances for each of the subcategories of the library, including GWAS-nominated genes, known erythroid essential genes, essential genes to cell viability, and orthogonal genes serving as negative controls. (D) Violin plot of day 4 and day 16 log2 CPM for known actors GATA1 and RPS19 and negative controls LacZ and luciferase. (E) Log2 hairpin counts averaged for known actors GATA1 and RPS19 as well as negative controls LacZ and luciferase across the course of the experiment. Gray lines depict the universe of all other gene traces in the library for context.
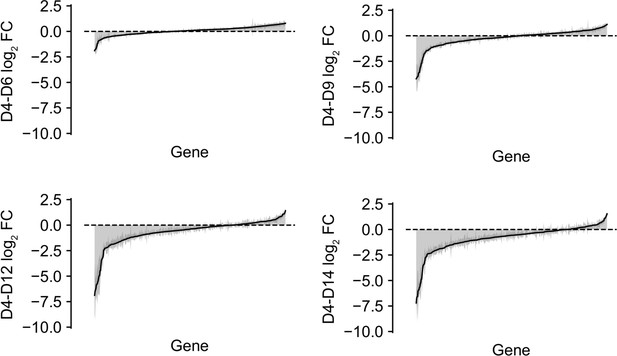
shRNA abundance log2 fold changes from day four to each of the other time points.
Represented values are the mean of hairpin abundance log2 fold changes across hairpins for each gene and two standard deviations.
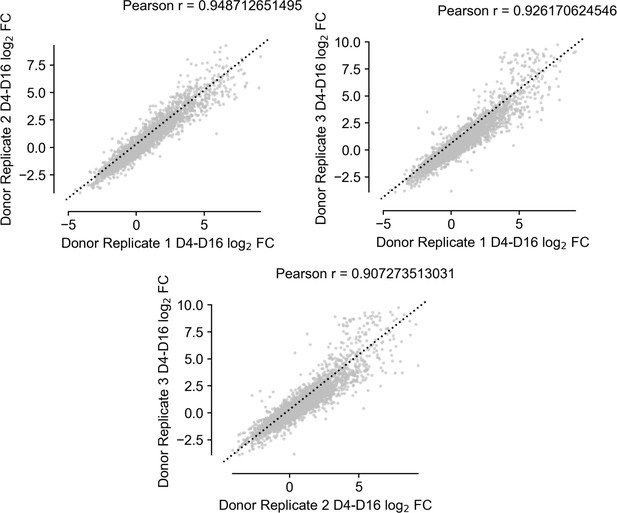
Scatter plots showing agreement of replicate observations across independent CD34+ donor populations.
https://doi.org/10.7554/eLife.44080.010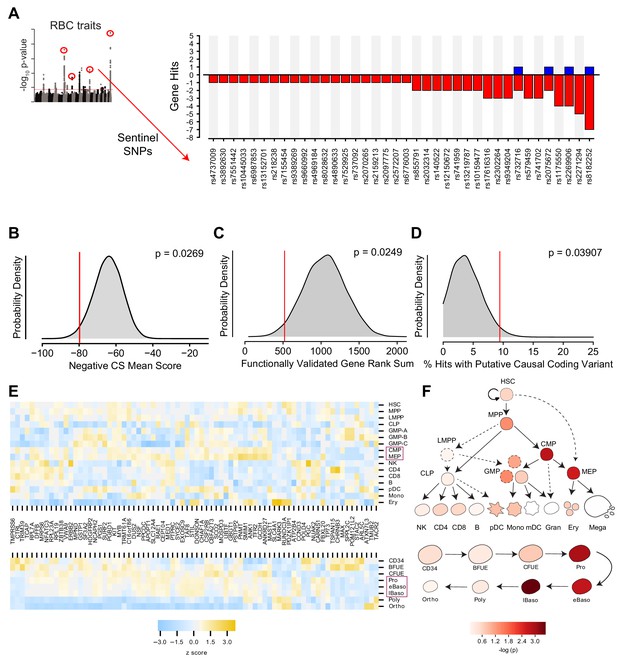
Statistical Modeling of Gene Effect Accounting for Off-target shRNA Confounders.
(A) Bar graph showing the 38 of 75 loci in the screen with at least one corresponding statistically significant (FDR < 0.1, β >0.1) gene effect causing either a positive or negative log2 fold change in shRNA abundance.Statistical model output for each gene in screen available in Figure 3—source data 1. (B) Kernel density plot showing the expected distributions of K562 essentiality scores using permuted gene hit sets from the library. (C) Hairpin rank sums for permuted sets of 5 genes. The red line indicates the enriched rank sums for 5 ‘gold standard’ genes included in the library, CCND3, SH2B3, MYB, KIT, and RBM38, for each which a genetic basis of action has already been established. (D) Permuted distribution of % inclusion of predicted coding variants among the set of identified hits. (E) Heat map depicting strength of expression (as z scores within each gene) for each of the 77 identified hit genes across hematopoietic lineages (top) and throughout the specific stages of adult erythropoiesis (bottom). Purple boxes highlight the cell types that were enriched for expression of hit genes. (F) Calculated enrichment of the identified hit genes for expression across hematopoietic lineages (top) and throughout the specific stages of adult erythropoiesis (bottom). In both cases, cellular states corresponding to those along the erythropoietic lineage had elevated probability of expressing genes from the hit set as compared to other genes from the library.
-
Figure 3—source data 1
Table containing the R model output for each gene.
- https://doi.org/10.7554/eLife.44080.015
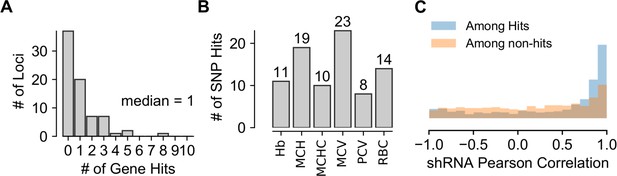
Additional Characterization of Modeling Outcomes.
(A) Histogram showing the number of gene hits identified at each of the 40 loci with at least one significant gene effect detected. Statistical model output for each gene in screen available in Figure 3—source data 1. (B) Bar graph showing the number of gene hits identified for each of the six red blood cell traits used in the original GWAS to identify the studied loci. (C) Density-normalized histogram showing the Pearson correlation of hairpin measurements for both genes nominated as hits and genes not nominated as hits.
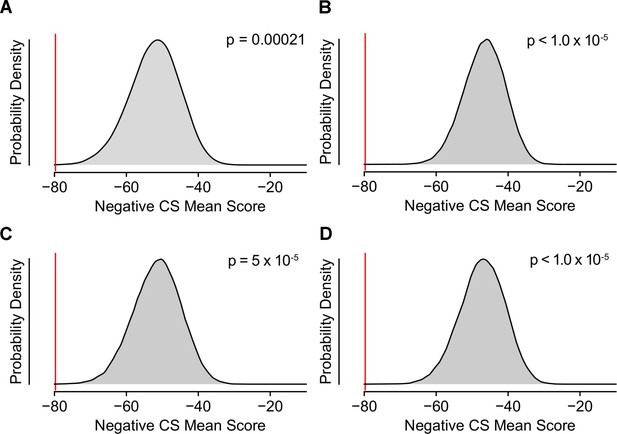
K562 Essentiality Scores Comparing Hit Genes vs.Genes Implicated by Other Traits.
(A) Permuted enrichment of essentiality among the set of hit genes vs. randomly chosen sets of genes from the human genome. (B) Permuted enrichment of essentiality among the set of hit genes vs. genes implicated by a separate GWAS for LDL cholesterol levels. (F) Permuted enrichment of essentiality among the set of hit genes vs. genes implicated by a separate GWAS for HDL cholesterol levels. (C) Permuted enrichment of essentiality among the set of hit genes vs. genes implicated by a separate GWAS for blood triglyceride levels.
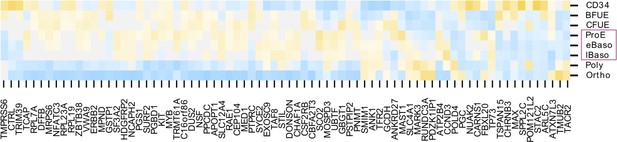
Heat map depicting strength of expression (as z scores within each gene) for each of the 77 identified hit genes throughout the specific stages of fetal erythropoiesis.
Purple boxes highlight the cell types that were enriched for expression of hit genes.
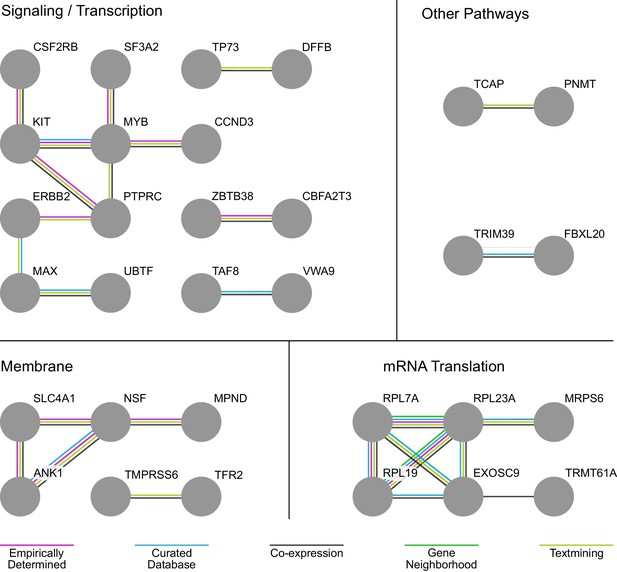
Analysis of Interactions Among Members of the Hit Set Identifies Signaling/Transcription, Membrane, and mRNA Translation-Related Subnetworks Important to Erythropoiesis.
STRING interaction network analysis identifies signaling/transcription, membrane, and mRNA translation-related subnetworks important to erythropoiesis embedded in the genes identified in the screen hit set. Edges connecting the network are color-coded according to the evidence supporting the interaction. In STRING, this evidence can derive from empirical determination, curation in a database, co-expression of the respective gene nodes, genomic proximity, and text-mining of published literature.
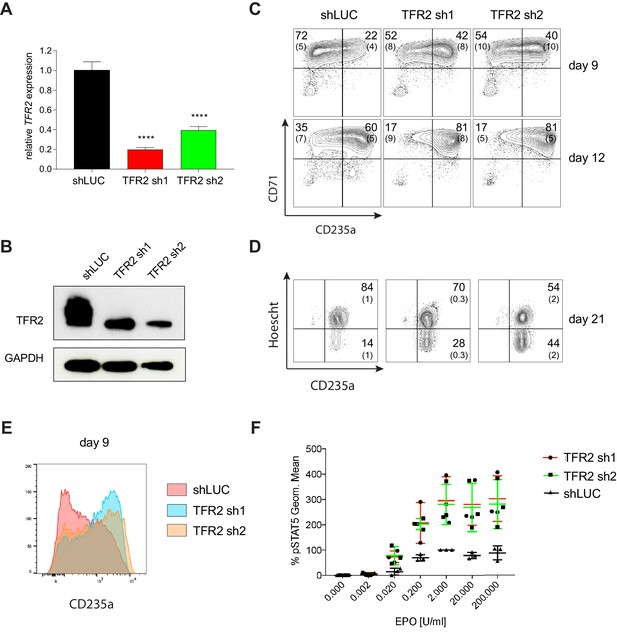
Transferrin receptor two is a Negative Regulator of Human Erythropoiesis.
(A) Quantitative RT-PCR and (B) Western blot showing the expression of TFR2 in human CD34+ cells five days post-infection with the respective lentiviral shRNAs targeting TFR2 (TFR2 sh1 and sh2) and a control luciferase gene (shLUC). (C) Representative FACS plots of erythroid cell surface markers CD71 (transferrin receptor) and CD235a (Glycophorin A) expression at various time points during erythroid differentiation. Percentages in each quadrant are represented as mean and standard deviation of 3 independent experiments (D) Hoechst staining showing more enucleated cells after TFR2 knockdown at day 21 of erythroid culture. (E) Representative histogram plots showing increased expression of CD235a (Glycophorin A) after TFR2 knockdown (F) Enhanced pSTAT5 response after TFR2 knockdown in UT7/EPO cells.
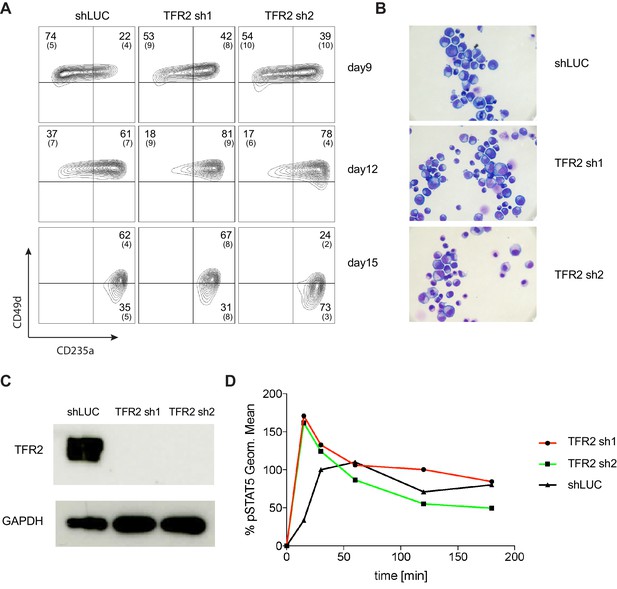
Additional Analysis Showing Transferrin Receptor two is a Negative Regulator of Human Erythropoiesis.
(A) Representative FACS plots of alternate erythroid cell surface markers CD49d (α4 integrin) and CD235a (Glycophorin A) expression at various time points during erythroid differentiation. (B) May-Grunwald Giemsa staining showing more differentiated erythroid cells after TFR2 knockdown at day 18 of erythroid culture. (C) Western blot showing downregulation of TFR2 in UT7/EPO cells. (D) Time-dependent absolute value of Mean Fluorescence Intensity (MFI) of STAT5 in UT7/Epo cells after TFR2 knockdown.
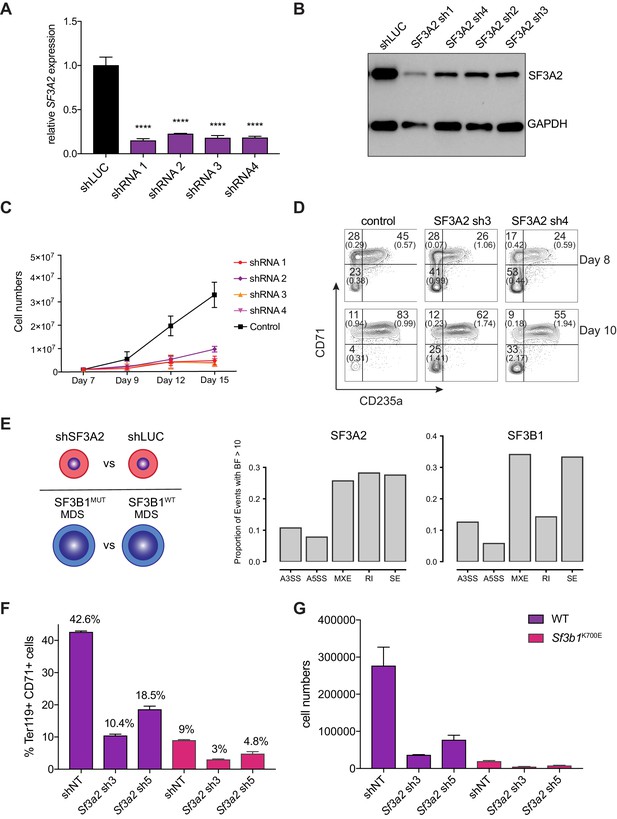
SF3A2 is a Key regulator of Human Erythropoiesis and Modulates Erythropoiesis Defects in a Murine Model of MDS.
(A) Quantitative RT-PCR and (B) Western blot showing the expression of SF3A2 in human CD34+ cells five days post-infection with the respective lentiviral shRNAs targeting SF3A2 (sh1-4) and a control luciferase gene (shLUC). (C) Growth curves showing that downregulation of SF3A2 results in reduced total cell numbers during erythroid differentiation from three independent experiments. (D) Representative FACS plots of erythroid cell surface markers CD71 (transferrin receptor) and CD235a (Glycophorin A) expression at various time points during erythroid differentiation. Percentages in each quadrant are represented as mean and standard deviation of three independent experiments (E) Altered splicing events identified by RNA-Seq analysis of stage matched erythroid cells (shSF3A2 vs. shLUC). Overlapping changes observed in SF3B1 mutant BM cells from MDS patients (Obeng et al) (Figure 6—source data 5 and 6). Differentially expressed genes and pathway analysis available in Figure 6—source data 1–4. (F) Lineage negative bone marrow cells from wildtype (WT) and Sf3b1K700E mice were infected with shRNAs targeting murine Sf3a2 gene co-expressing a reporter GFP gene. Percentage of Ter119+ CD71+ erythroid cells within the GFP compartment after 48 hr in erythroid differentiation. (G) Total cell numbers of GFP+ erythroid cells after 48 hr in erythroid differentiation.
-
Figure 6—source data 1
Table containing the DESeq2 output for differentially expressed genes in cells undergoing SF3A2 knockdown or control shRNA treatment.
- https://doi.org/10.7554/eLife.44080.022
-
Figure 6—source data 2
Table containing the DESeq2 output for differentially expressed genes in MDS patients with and without mutations in SF3B1.
- https://doi.org/10.7554/eLife.44080.023
-
Figure 6—source data 3
Tables containing the GO component (Table 1) and function (Table 2) enrichments calculated using GOrilla for cells undergoing SF3A2 knockdown or control shRNA treatment.
- https://doi.org/10.7554/eLife.44080.024
-
Figure 6—source data 4
Tables containing the GO component (Table 1) and function (Table 2) enrichments calculated using GOrilla for MDS patient samples with and without mutations in SF3B1.
- https://doi.org/10.7554/eLife.44080.025
-
Figure 6—source data 5
Tables containing the differential splicing analysis for cells undergoing SF3A2 knockdown or control shRNA treatment.
Categories of splice mutations presented in each table are alternative 3’ splice sites, alternative 5’ splice sites, mutually exclusive exons, retrained introns, and skipped exons, respectively.
- https://doi.org/10.7554/eLife.44080.026
-
Figure 6—source data 6
Tables containing the differential splicing analysis for MDS patient patient samples with and without mutations in SF3B1.
Categories of splice mutations presented in each table are alternative 3’ splice sites, alternative 5’ splice sites, mutually exclusive exons, retrained introns, and skipped exons, respectively.
- https://doi.org/10.7554/eLife.44080.027
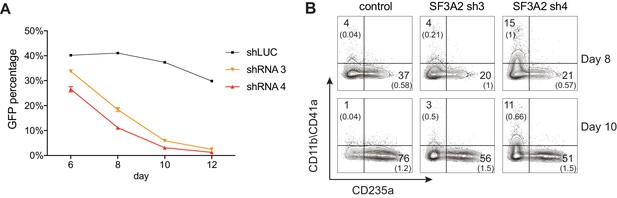
Additional Analysis Showing SF3A2 is Required for Human Erythropoiesis.
(A) shRNAs targeting SF3A2 co-expressing a reporter GFP gene was infected into human CD34+ cells and cultured in erythroid conditions. GFP expression at various time points from three independent experiments show that downregulation of SF3A2 results in reduced cell numbers. (B) Representative FACS plots of erythroid (CD235a) and non-erythroid cell surface markers (CD11b/CD41 a) and at various time points showing an increase in non-erythroid lineages upon SF3A2 downregulation. Cells were gated on the GFP positive population.
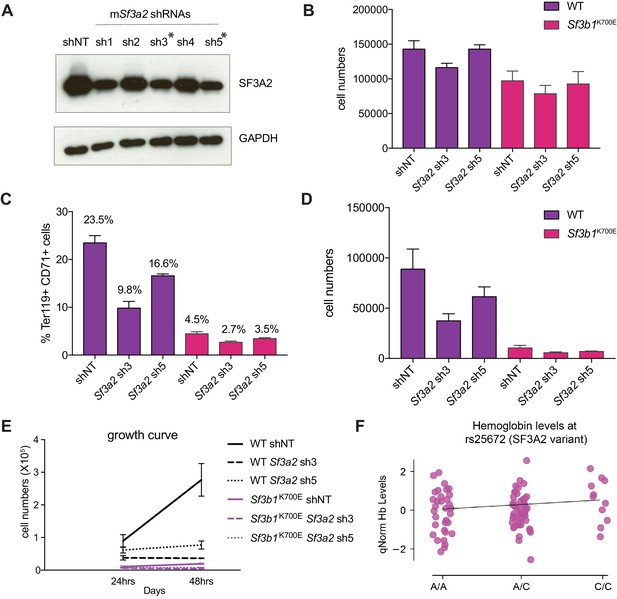
Additional Analysis of Erythropoiesis Defects Observed in Sf3b1K700E Murine Erythroid Cells upon SF3A2 knockdown.
(A) Knockdown efficiency of shRNAs targeting SF3A2 in murine erythroleukemia (MEL) cells by western blot. (B) Total cell numbers of GFP +shRNA expressing bone marrow cells from wildtype (WT) and Sf3b1K700E mice at the start of murine erythroid differentiation. (C) Percentage of Ter119+ CD71+ erythroid cells within GFP compartment and (D) Total cell numbers of GFP+ erythroid cells after 24 hr in erythroid differentiation. (E) Growth curves of GFP+ erythroid cells during erythroid culture. (F) Putative but insignificant interaction between SF3A2 variant alleles (rs25672) and hemoglobin levels in MDS patients with SF3B1 mutations.
Tables
Reagent type (species) or resource | Designation | Source or reference | Identifiers | Additional information |
---|---|---|---|---|
Biological sample (Homo sapiens) | CD34 + mobilized peripheral blood | Fred Hutchinson Cancer Research Center | ||
Cell line (Homo sapiens) | UT-7/EPO | NA | RRID:CVCL_5202 | maintained in Sankaran laboratory |
Cell line (Mus musculus) | MEL | NA | maintained in Sankaran laboratory | |
Genetic reagent (Mus musculus) | Sf3b1K700E | Obeng et al., 2016 | Dr. Benjamin L. Ebert (Brigham Women's Hospital, Boston MA) | |
Recombinant DNA reagent (lentiviral shRNA) | PLKO.1-Puro (plasmid) | Sigma-Aldrich | RRID :Addgene_10878 | Pol III based shRNA backbone |
Recombinant DNA reagent (lentiviral shRNA) | PLKO-GFP (plasmid) | this paper | GFP version of pLKO.1-Puro | |
Recombinant DNA reagent (lentiviral shRNA) | SFFV-Venus-mir30 shRNA (plasmid) | this paper | Pol II based shRNA backbone | |
Antibody | mouse monoclonal anti-human CD235a-APC | Thermo Fisher Scientific | Cat#: 17-9987-42; RRID:AB_2043823 | FACS (5 ul per test) |
Antibody | mouse monoclonal anti-human CD71-FITC | Thermo Fisher Scientific | Cat#: 11-0719-42; RRID:AB_1724093 | FACS (5 ul per test) |
Antibody | mouse monoclonal anti-human CD71-PEcy7 | Thermo Fisher Scientific | Cat#: 25-0719-42; RRID:AB_2573366 | FACS (5 ul per test) |
Antibody | mouse monoclonal ant-human CD49d-PE | Miltenyi Biotec | Cat#: 130-093-282; RRID:AB_1036224 | FACS (10 ul per test) |
Antibody | mouse monoclonal anti-human CD41a-PE | Thermo Fisher Scientific | Cat#: 12-0419-42; RRID:AB_10870785 | FACS (5 ul per test) |
Antibody | mouse monoclonal anti-human CD11b-PE | Thermo Fisher Scientific | Cat#: 12-0118-42; RRID:AB_2043799 | FACS (5 ul per test) |
Antibody | Rat monoclonal anti-mouse Ter119-APC | Thermo Fisher Scientific | Cat#: 17-5921-82; RRID:AB_469473 | FACS (0.25 ug/test) |
Antibody | Rat monoclonal anti-mouse CD71-PE | Thermo Fisher Scientific | Cat#: 12-0711-82; RRID:AB_465740 | FACS (0.5 ug/test) |
Antibody | mouse monoclonal anti-phospho STAT5 Alexa Fluor-647 | BD Bioscience | Cat#: 612599; RRID:AB_399882 | FACS (1:20) |
Antibody | mouse monoclonal anti-GAPDH | Santa Cruz Biotechnology | sc-32233; RRID:AB_627679 | Western (1:20,000) |
Antibody | mouse monoclonal anti-TFR2 | Santa Cruz Biotechnology | sc-32271; RRID:AB_628395 | Western (1:200) |
Antibody | mouse monoclonal anti-SF3A2 | Santa Cruz Biotechnology | sc-390444 | Western (1:1000) |
Sequence-based reagent | shLUC | Sigma-Aldrich | TRCN0000072259 | 5’- CGCTGAGTACTTCGAAATGTC-3’ |
Sequence-based reagent | TFR2 sh1 (human) | Sigma-Aldrich | TRCN0000063628 | 5’-GCCAGATCACTACGTTGTCAT-3’ |
Sequence-based reagent | TFR2 sh2 (human) | Sigma-Aldrich | TRCN0000063632 | 5-CAACAACATCTTCGGCTGCAT-3’ |
Sequence-based reagent | SF3A2 sh1 (human) | Sigma-Aldrich | TRCN0000000060 | 5’-CTACGAGACCATTGCCTTCAA-3’ |
Sequence-based reagent | SF3A2 sh2 (human) | Sigma-Aldrich | TRCN0000000061 | 5’-CCTGGGCTCCTATGAATGCAA-3’ |
Sequence-based reagent | SF3A2 sh3 (human) | Sigma-Aldrich | TRCN0000000062 | 5’-CAAAGTGACCAAGCAGAGAGA-3’ |
Sequence-based reagent | SF3A2 sh4 (human) | Sigma-Aldrich | TRCN0000000063 | 5’-ACATCAACAAGGACCCGTACT-3’ |
Commercial assay or kit | RNeasy Mini Kit | QIAGEN | Cat#: 74104 | |
Commercial assay or kit | iScript cDNA synthesis Kit | Bio-Rad | Cat#: 1708891 | |
Commercial assay or kit | iQ SYBR Green Supermix | Bio-Rad | Cat#: 170–8882 | |
Commercial assay or kit | NucleoSpin Blood XL-Maxi kit | Clonetch | Cat#: 740950.1 | |
Commercial assay or kit | Lineage Cell Depletion Kit (mouse) | Miltenyi | Cat#: 130-090-858 | |
Commercial assay or kit | Nextera XT DNA Library Preparation Kit | Illumina | Cat#: FC-131–1096 | |
Commercial assay or kit | NextSeq 500/550 High Output Kit v2.5 (75 Cycles) | Illumina | Cat#: 20024906 | |
Commercial assay or kit | Bioanalyzer High Sensitivity DNA Analysis | Agilent | Cat#: 5067–4626 | |
Commercial assay or kit | Agencourt AMPure XP | Beckman-Coulter | Cat#: A63881 | |
Commercial assay or kit | TaKaRa Ex TaqDNA Polymerase | Takara | Cat#: RR001B | |
Commercial assay or kit | Qubit dsDNA HS Assay Kit | Thermo Fisher | Cat#: Q32854 | |
Chemical compound, drug | Human Holo-Transferrin | Sigma Aldrich | Cat#: T0665-1G | |
Peptide, recombinant protein | Humulin R (insulin) | Lilly | NDC 0002-8215-01 | |
Peptide, recombinant protein | Heparin | Hospira | NDC 00409-2720-01 | |
Peptide, recombinant protein | Epogen (recombinant erythropoietin) | Amgen | NDC 55513-267-10 | |
Peptide, recombinant protein | Recombinant human stem cell factor (SCF) | Peprotech | Cat#: 300–07 | |
Peptide, recombinant protein | Recombinant human interleukin-3 (IL-3) | Peprotech | Cat#: 200–03 | |
Peptide, recombinant protein | Recombinant mousestem cell factor (SCF) | R&D systems | Cat# 455-MC-010 | |
Peptide, recombinant protein | recombinant mouse Insulin like Growth Factor 1 (IGF1) | R&D systems | Cat# 791 MG-050 | |
Chemical compound, drug | Hoechst 33342 | Life Technologies | Cat#: H1399 | FACS (1:1000) |
Chemical compound, drug | Fixation Buffer | BD Bioscience | Cat#: 554655 | |
Chemical compound, drug | Perm Buffer III | BD Bioscience | Cat#: 558050 | |
Chemical compound, drug | May-Grünwald Stain | Sigma-Aldrich | Cat#: MG500 | |
Chemical compound, drug | Giemsa Stain | Sigma-Aldrich | Cat#: GS500 | |
Software, algorithm | STAR | Dobin et al., 2013 | RRID:SCR_015899 | |
Software, algorithm | MISO | Katz et al., 2010 | RRID:SCR_003124 | |
Software, algorithm | R | The R Foundation | RRID:SCR_001905 | |
Software, algorithm | Salmon | Patro et al., 2017 | RRID:SCR_017036 | |
Software, algorithm | GOrilla | Eden et al., 2009 | RRID:SCR_006848 | |
Software, algorithm | VEP | McLaren et al., 2016 | RRID:SCR_007931 | |
Software, algorithm | FlowJo version 10 | FlowJo | RRID:SCR_008520 | |
Software, algorithm | GraphPad Prism 7 | GraphPad Software Inc | RRID:SCR_002798 | |
Software, algorithm | Python 2, 3 | Python Software Foundation | RRID:SCR_008394 | |
Software, algorithm | PLINK | Chang et al., 2015 | RRID:SCR_001757 | |
Software, algorithm | PoolQ | Broad Institute | https://portals.broadinstitute.org/gpp/public/software/poolq |
Additional files
-
Transparent reporting form
- https://doi.org/10.7554/eLife.44080.028