Modulation of tonotopic ventral medial geniculate body is behaviorally relevant for speech recognition
Figures
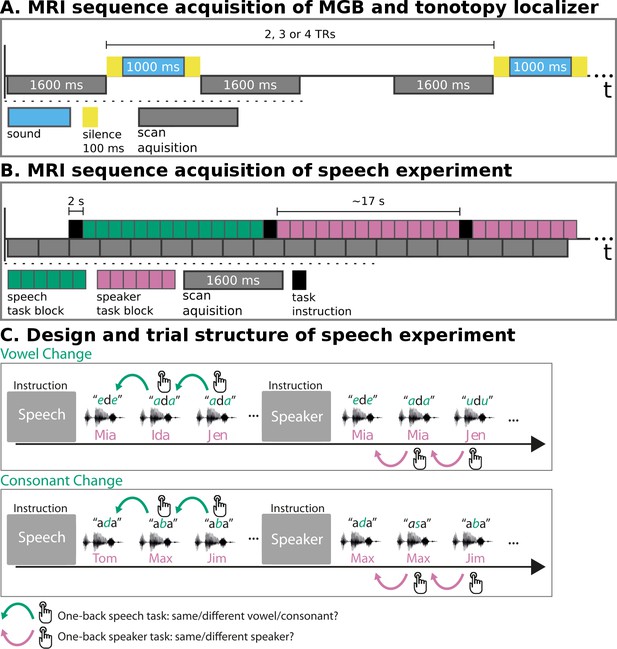
MRI sequence acquisition and experimental design.
(A) MRI sequence acquisition of MGB and tonotopy localizer. Stimuli (‘sound’) were presented in silence periods between scan acquisitions and jittered with 2, 3, or 4 TRs. TR: repetition time of volume acquisition. (B) MRI sequence acquisition of the speech experiment. Each green or magenta rectangle of a block symbolizes a syllable presentation. Blocks had an average length of 17 s. Task instructions (‘speech’, ‘speaker’) were presented for 2 s before each block. MRI data were acquired continuously (‘scan acquisition’) with a TR of 1600 ms. (C) Design and trial structure of speech experiment. In the speech task, listeners performed a one-back syllable task. They pressed a button whenever there was a change in syllable in contrast to the immediately preceding one, independent of speaker change. The speaker task used exactly the same stimulus material and trial structure. The task was, however, to press a button when there was a change in speaker identity in contrast to the immediately preceding one, independent of syllable change. Syllables differed either in vowels or in consonants within one block of trials. An initial task instruction screen informed participants about which task to perform.
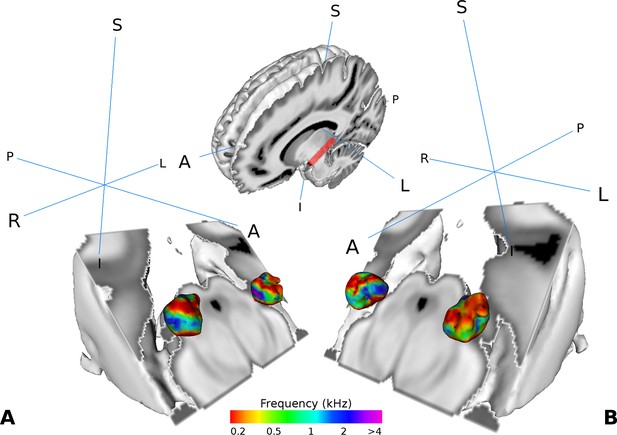
Visualization of the average tonotopy across participants (n = 28) found in the MGB using the tonotopic localizer.
The half-brain image at the top shows the cut through the brain with a red line denoting the −45° oblique plane used in the visualizations in panels A-B. (A) Three dimensional representation of the tonotopy in the left and right MGB with two low-high frequency gradients. (B) Same as in A with a different orientation. Crosshairs denote orientation (A: anterior, P: posterior, L: left, R: right, S: superior, I: inferior).
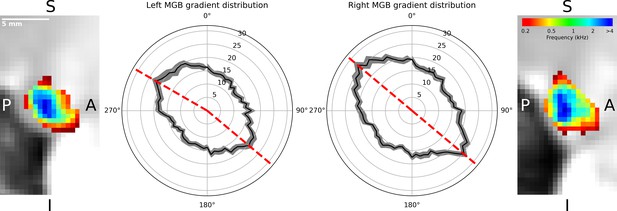
Distribution of gradients in a sagittal plane for ten slices averaged over participants (n = 28).
The mean number of angle counts in 5° steps (black line with standard error of the mean in gray, numbers indicate counts) for the left MGB have maxima at 130° and 300° (red dashed lines). For the right MGB the maximum gradients are at 130° and 310° (red dashed lines). We interpreted these as two gradients in each MGB: one from anterior-ventral to the center (130°) and the other from the center to anterior-dorsal-lateral (300°, 310°). The two outer images display a slice of the mean tonotopic map in the left and right MGB in sagittal view (S: superior, I: inferior, P: posterior, A: anterior).
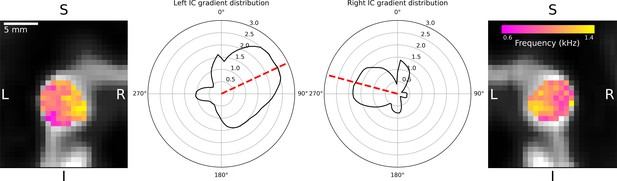
Mean distribution of gradients for the inferior colliculus (IC) in coronal view for three slices averaged over participants (n = 28).
The mean number of angle counts in 5° steps (black line; numbers indicate counts) for the left IC cumulatively point towards 65°. For the right IC the general gradient direction was 285°. The two outer images display one slice of the mean tonotopic map across participants in the left and right IC in coronal view (S: superior, I: inferior, L: left, R: right). Individual tonotopies showed high variability (results not shown). The mean tonotopy revealed a gradient from low frequencies in lateral locations to high frequencies in medial locations.
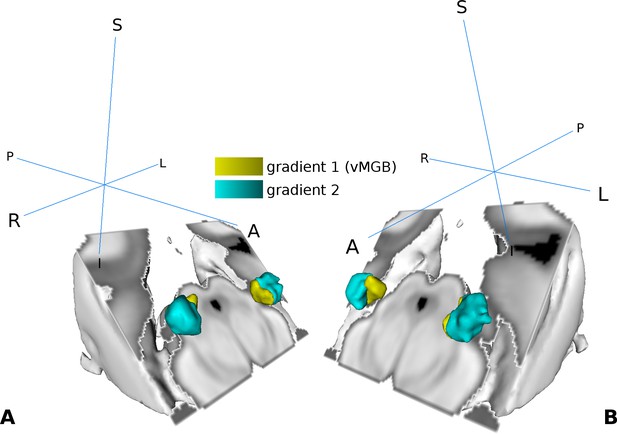
Visualization of the tonotopic gradients found in the MGB based on the tonotopic localizer (see Figure 2).
(A) Three dimensional rendering of the two tonotopic gradients (yellow: ventro-medial gradient 1, interpreted as vMGB, cyan: dorso-lateral gradient 2) in the left and right MGB. (B) Same as in A with a different orientation. Orientation is the same as in Figure 2; crosshairs denote orientation.
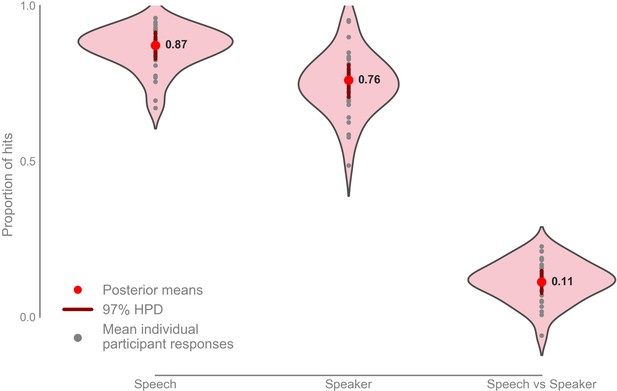
Mean proportion of correct button presses for the speech and speaker task behavioral scores, as well as the difference between the speech and speaker task (n = 33).
Mean speech task: 0.872 with 97% HPD [0.828, 0.915], mean speaker task: 0.760 with 97% HPD [0.706, 0.800], mean speech vs speaker task: 0.112 with 97% HPD [0.760, 0.150]. Raw data provided in the Source Data File.
-
Figure 5—source data 1
Raw behavioral correct button presses and total correct answers expected.
- https://doi.org/10.7554/eLife.44837.011
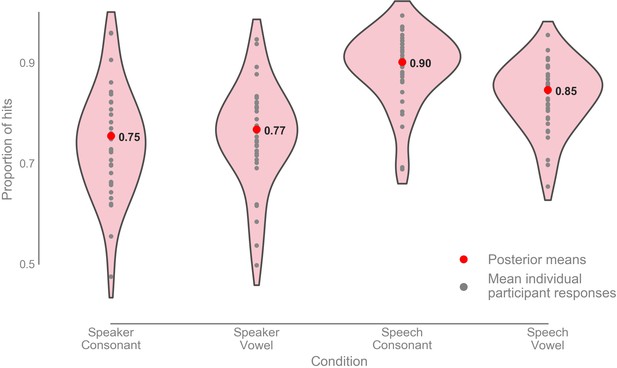
Mean proportion of correct button presses for each condition.
The red dots and black numbers indicate estimated means, and the gray dots are individual participant responses averaged over the experiment. The violin plots summarize the distribution of data points. Raw data are provided in the Source Data File.
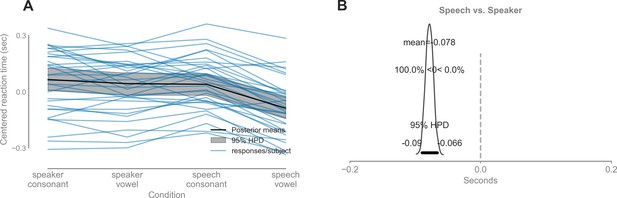
Results from the reaction time analysis.
(A) Reaction times per condition summarized as a mean (black line) and 95% highest posterior density interval (gray area). The blue lines indicate averaged individual reaction times per condition. (B) The speech task reaction time is on average 78 ms quicker than the speaker task reaction time indicating that there is no speed-accuracy trade-off.
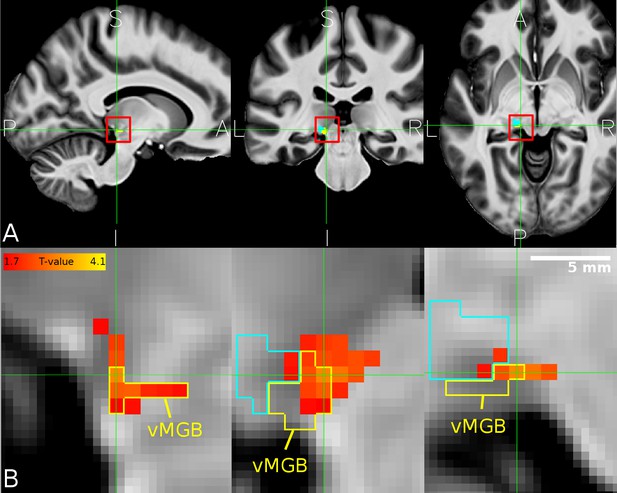
Overlap between MGB divisions and the behaviourally relevant task-dependent modulation.
(A) The mean structural image across participants (n = 33) in MNI space. The red squares denote the approximate location of the left MGB and encompass the zoomed in view in B. (B) Overlap of correlation between the speech vs speaker contrast and the mean percent correct in the speech task (hot color code) across participants within the left vMGB (yellow). The tonotopic gradient two is shown in cyan. Panels correspond to sagittal, coronal, and axial slices (P: posterior, A: anterior, S: superior, I: inferior, L: left, R: right). Crosshairs point to the significant voxel using SVC in the vMGB mask (MNI coordinate −11,–28, −5).
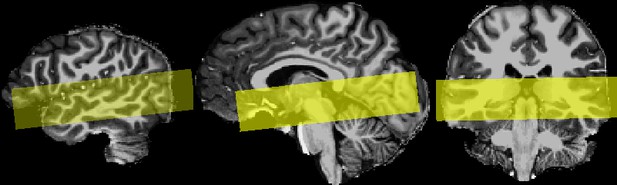
Example of the orientation and volume covered by the 28 slices of the functional MRI measurements.
From left to right: left lateral sagittal view, medial sagittal view, and coronal view (left hemisphere is on the left).
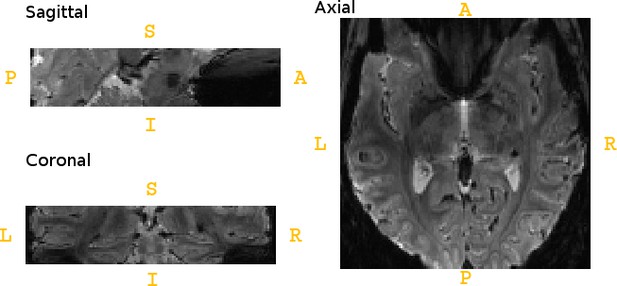
An example of an echo planar image in sagittal, axial and coronal view, as recorded in the speech experiment.
Wrapping artifacts were avoided by using appropriate phase over-sampling (33% larger FoV in phase-encoding direction A-P; A: anterior, P: posterior, L: left, R: right, S: superior, I: inferior).
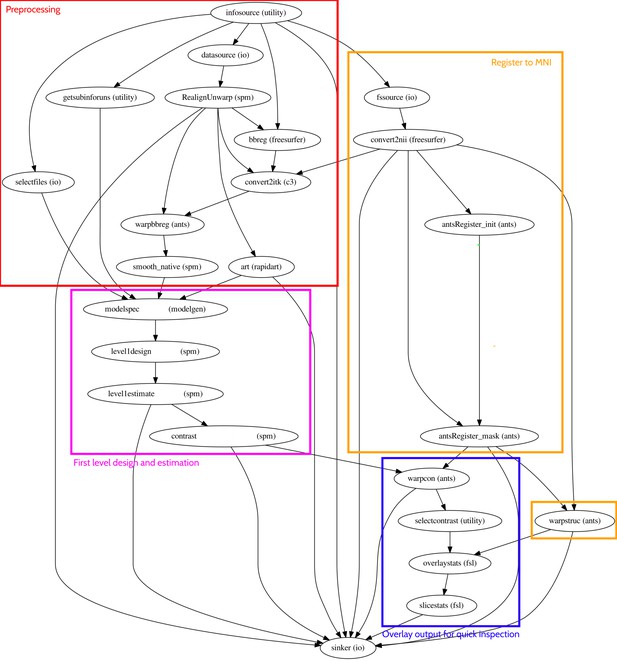
Example workflow for the functional MRI analysis of the speech experiment as coded in nipype depicting different processing stages (preprocessing, Register to MNI, First level design and estimation, overlay output for quick inspection).
Each bubble represents a node with a descriptive name and the software package or utility in parentheses (io: input/output utility). Arrows indicate directional connections between nodes. The infosource utility takes the subject names and passes them on to the nodes that require this information (datasource, selectfiles, bbreg, fssource, gestubinforuns). Datasource and selectfiles select the files needed for the analysis. The functional runs are first realigned and unwarped (RealignUnwarp). The mean realigned image together with the structural image are used in the bbreg step to perform the boundary based registration. Convert2itk (c3: c3d converts 3D images between common file formats, https://sourceforge.net/p/c3d/git/ci/master/tree/doc/c3d.md) converts the rotation/translation matrix from bbreg to ITK format in order to be able to use ANTs to apply the coregistration transformations of the functional to structural runs in the warpbbreg step. The coregistered runs are then smoothed (smooth_native). Outliers are computed in the art (rapidart) step. Model generation is implemented in modelspec, where smoothed images, regressors of interest (onsets, durations convolved with HRF from getsubinforuns), and regressors of no interest (realignment parameters, outliers, physiological regressors, button presses) are gathered. These are modeled in the level1design step in SPM, estimated in level1estimate, and contrasts calculated in the contrast step. The two ANTs registrations to MNI (antsRegister_init and antsRegister_mask) are computed in parallel. Contrast warping to MNI space is computed in the warpcon step, and individual structural images are warped to MNI space in the warpstruc step. Using FSL statistical maps are overlayed in overlaystats and slicestats for quick visual inspection. The sinker collects desired files written in the temporary directory and organizes them according to subject name and computational step for easy access. Data processing workflows for MGB and tonotopic localizer follow the one for the speech experiment. Transformation matrices to MNI space were taken from the speech experiment computation, as data from all experiments need to be in the same space.
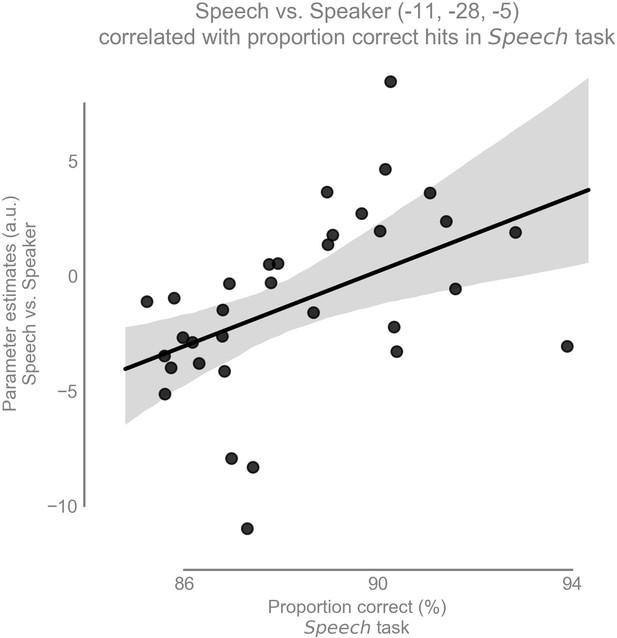
Task-dependent modulation of left vMGB correlates with proportion correct responses in the speech task over participants (n = 33): the better the behavioral score in the speech task, the stronger the BOLD response difference between speech and speaker task in the left vMGB (maximum statistic at MNI coordinate [−11,–28, −5].
The line represents the best fit with 97% bootstrapped confidence interval (gray shaded region).
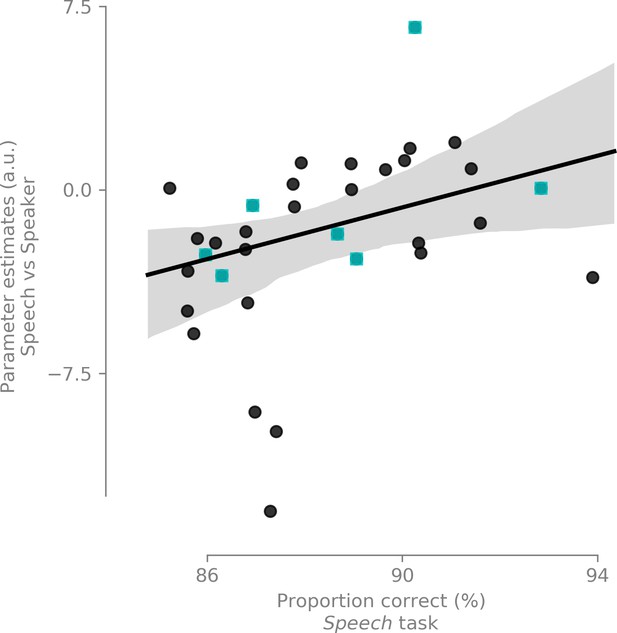
Correlation of parameter estimates (Speech vs. Speaker) for the significant voxel in the vMGB in the speech vs. speaker task with percent correct behavioral score in the speech task.
The cyan squares denote those participants who scored below 50% in the reading speed and comprehension test. A lower reading speed and comprehension score in these typically developed participants did not imply a lower vMGB response.
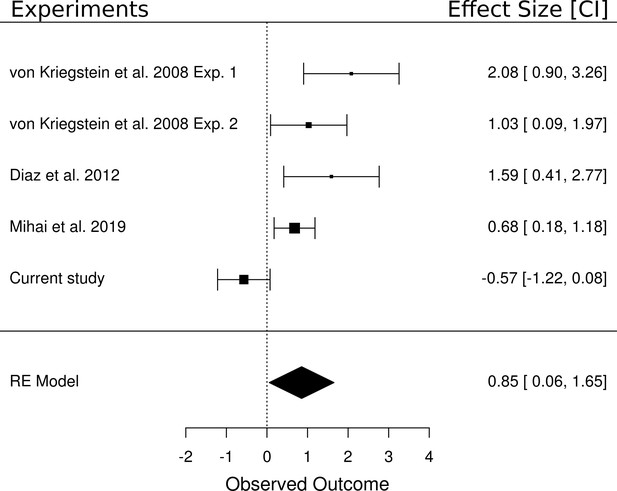
Meta-analysis of five experiments that investigated the difference between a speech and a non-speech control task.
Experiment 1 of von Kriegstein et al. (2008b) tested a speech task vs loudness task contrast (n = 16). All other experiments included a speech task vs speaker task contrast (i.e., Experiment 2 of von Kriegstein et al., 2008b (n = 17), the control participants of Díaz et al., 2012 (n = 14), the result of a recent experiment (Mihai et al., 2019) (n = 17) as well as the current study (n = 33)). The meta-analysis yielded an overall large effect size of d = 0.85 [0.06, 1.65], p=0.036. The area of the squares denoting the effect size is directly proportional to the weighting of the particular study when computing the meta-analytic overall score.
Tables
Center of mass (COM) and volume of each MGB mask used in the analysis.
https://doi.org/10.7554/eLife.44837.007Mask | COM (MNI coordinates mm) | Volume (mm³) |
---|---|---|
Left Gradient 1 (ventro-medial) | (−12.7,–26.9, −6.3) | 37.38 |
Left Gradient 2 (dorso-lateral) | (−14.8,–25.9, −5.4) | 77.38 |
Right Gradient 1 (ventro-medial) | (12.7,–27.6, −4.4) | 45.00 |
Right Gradient 2 (dorso-lateral) | (14.7,–25.8, −4.3) | 67.38 |
Additional files
-
Supplementary file 1
Montreal neurological institute (MNI) coordinates, p-values, T-values and parameter estimates (β) and 90% confidence intervals (CI) for voxels within regions for which we did not have an a-priori hypothesis.
Family-wise error corrected p-values were calculated using small volume correction for the voxels within the masks.
- https://doi.org/10.7554/eLife.44837.020
-
Transparent reporting form
- https://doi.org/10.7554/eLife.44837.021