Monkey EEG links neuronal color and motion information across species and scales
Figures
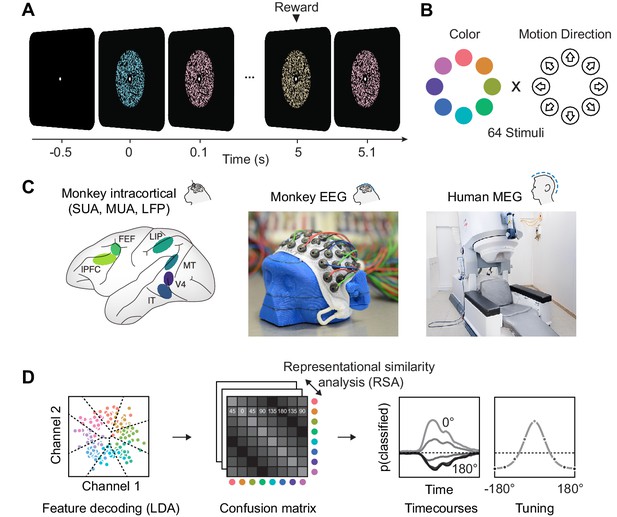
Experimental paradigm, recording and analyses.
(A) We presented a stream of random dot patterns with rapidly changing colors and motion directions. After successfully maintaining fixation for a specified time, a liquid reward or auditory reward cue was delivered. (B) Colors and motion directions were sampled from geometrically identical circular spaces. Colors were uniformly distributed on a circle in L*C*h-space, such that they were equal in luminance and chromaticity, and only varied in hue. (C) We performed simultaneous microelectrode recordings from up to six cortical areas. We used custom 65 channel EEG-caps to record human-comparable EEG in macaque monkeys. MEG was recorded in human participants. (D) We used the same multivariate analysis approach on all signal types: Multi-class LDA applied to multiple recording channels resulted in time-resolved confusion matrices, from which we extracted classifier accuracy time courses and tuning profiles.
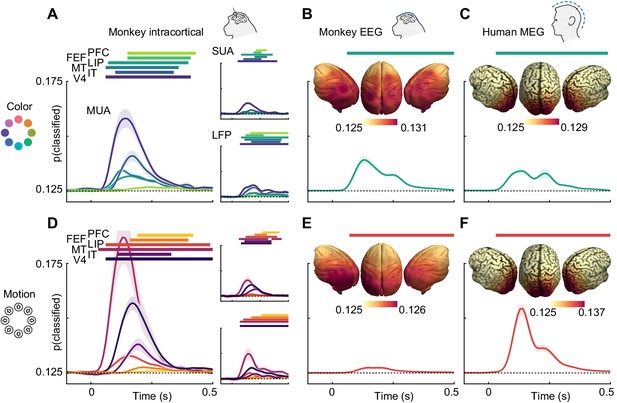
Color and motion direction information across areas and measurement scales.
All panels show classifier accuracy, quantified as the single trial prediction probability for the correct stimulus. Error bars indicate standard error over recording sessions (in macaques) or participants (in humans), horizontal lines show periods of significant information (cluster permutation with p<0.05, corrected for number of regions). (A) Color and (D) motion information is available in most areas in multi-unit, single-unit and LFP data. Information decreases along the cortical hierarchy. Note that MUA color information has very similar timecourses in PFC and FEF, and thus, FEF is barely visible. (B) Color and (E) motion information is available in monkey EEG. Insets: distribution of information in monkey EEG, estimated using source-level searchlight decoding. Information peaks in occipital areas. (C) Color and (F) motion information is available in human MEG. Insets: distribution of information in human MEG, estimated using source-level searchlight decoding. Information peaks in occipital areas.
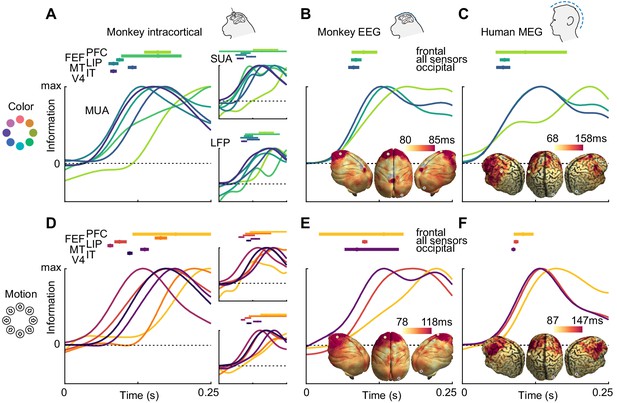
Color and motion direction information latencies across areas and measurement scales.
All panels show normalized classifier accuracy, and latency estimates as well as confidence intervals (bootstrap, 95%). (A) Color and (D) motion information rises first in early visual areas, and last in frontal areas. (B) Color and (E) motion latencies in monkey EEG are comparable to those in early visual areas. Insets: distribution of latencies in monkey EEG, estimated using source-level searchlight decoding. Information rises later in frontal sources than in occipital sources. Marked positions indicate sources for which time courses are shown. (C) Color and (F) motion latencies in human MEG are comparable to those of early visual areas in the macaque brain. Insets: distribution of latencies in human MEG, estimated using source-level searchlight decoding. Information rises later in frontal sources than in occipital sources.
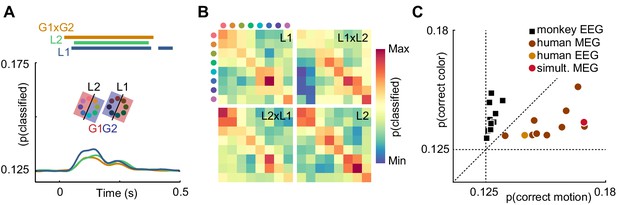
Control experiments.
(A) Time-resolved classifier accuracy. Accuracy is highest when trained and tested on high-luminance stimuli (L1), and lower when trained and tested on low-luminance stimuli (L2). Training on half of the color space in low luminance, and half of the color space in high luminance, and testing on the remainder (G1 x G2), results in accuracy comparable to the low-luminance stimuli alone. (B) Confusion matrices for low-luminance and high luminance stimuli, as well as classifiers trained on low-luminance and tested on high-luminance stimuli and vice versa (L1 x L2, L2 x L1). (C) Maximum color and motion classifier accuracies for all individual sessions. Color is better classified in monkey EEG, motion is better classified in human MEG and EEG. Simultaneously recorded human MEG and EEG results in overall higher accuracy in MEG, but more motion than color information in both.
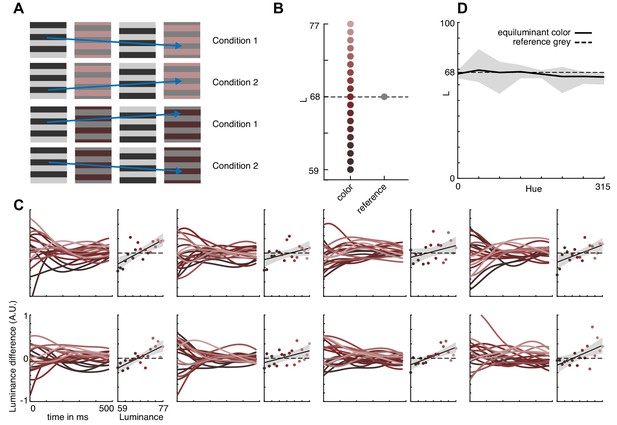
Perceptual equiluminance control.
(A) To account for the possibility of luminance differences between stimuli due to different color sensitivities in macaques and humans, we performed a psychophysical experiment using a no-report minimum motion technique based on eye-movements in a third macaque monkey. We presented moving gratings consisting of alternating frames with luminance and color contrasts, respectively, with each frame being shifted by a quarter cycle for 500 ms. Color contrasts consisted of a reference gray and a color to be probed. If the probed color was darker than the reference gray, motion in one direction was perceived, if it was brighter, in the opposite direction. A color equiluminant to the reference gray should not evoke any stable motion percept. Stimuli were presented in two conditions: either a brighter probe color elicited upwards motion, or downwards motion. (B) This technique was applied to colors of 8 different hues, at 19 luminance levels. (C) We computed a luminance difference index based on eye position measurements, by calculating the difference in vertical eye trace curvature between conditions. For a color equiluminant to the reference gray, this measure was expected to be 0. The left plots show the luminance difference measure resolved in time, the right plots show the average over time. For all colors, 0 was crossed within the range of luminances probed, suggesting that this range included colors equiluminant to the reference gray. There was a significantly positive slope (pearson correlation, p<0.05) for 6 out of 8 colors, validating our approach. (D) Estimates of the zero-crossing for all eight hues are close to the L value of the reference gray, suggesting that psychophysically equiluminant colors in the macaque are close to those derived from human L*C*h - space. Shaded area shows 95% bootstrap confidence interval.
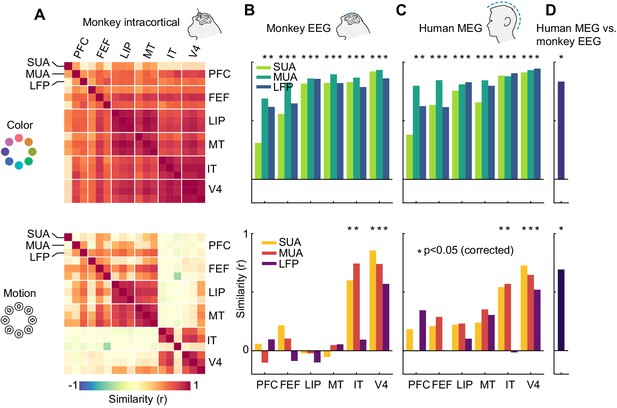
Representational similarity between areas and measurement scales.
(A) Similarity between SUA, MUA and LFP color (top) and motion direction representations (bottom) in six areas of the macaque brain, masked at p<0.05 (uncorrected). Color representations are highly similar between all areas; motion representations are split between frontal/dorsal and ventral areas. (B) and (C) Similarity between monkey EEG and human MEG color and motion representations and those in SUA, MUA and LFP in six areas. Non-invasive color representations are similar to all areas, motion representations are similar to IT and V4 representations (p<0.05, random permutation test, corrected for number of areas). (D) Color and motion representations are similar between human MEG and monkey EEG (both p<0.001, random permutation test).
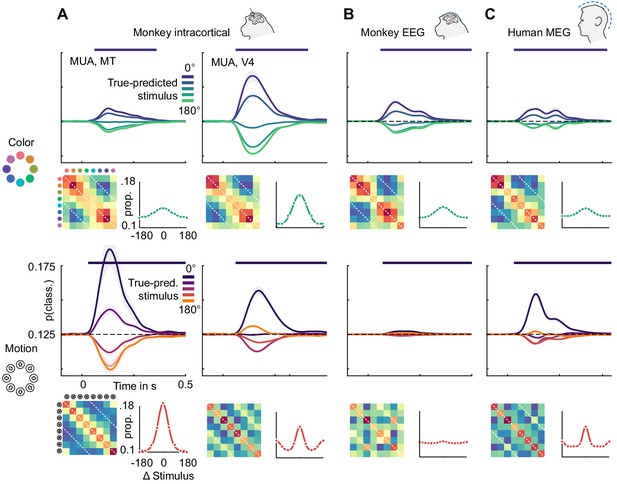
Color and motion direction tuning.
Color and motion direction tuning in (A) MT (left), V4 (right), (B) monkey EEG and (C) human MEG. Shown is, for each area or signal type, first: the temporally resolved prediction probability as a function of the distance between true and predicted stimulus. The dark blue line indicates the probability of a stimulus being predicted correctly (classifier accuracy), the green (color) and orange (motion) lines the probability of a stimulus being predicted as the opposite in the circular stimulus space. Second: the confusion matrix, indicating prediction probabilities for all stimulus combinations. Third: A representation tuning curve, indicating prediction probabilities as a function of distance between true and predicted stimulus at the time of peak accuracy. For color, tuning is always unimodal, with opposite-classifications having the lowest probability. For motion direction, V4, EEG and MEG, but not MT tuning is bimodal, with opposite-classifications having a higher probability than some intermediate stimulus distances.
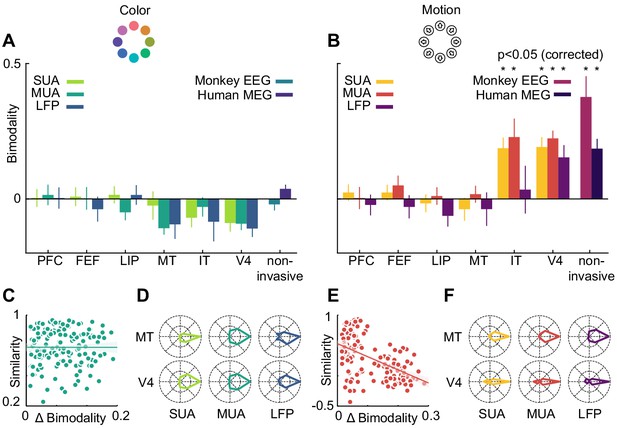
Motion direction tuning bimodality across areas and measurement scales explains representational similarity.
Representations of color (A) do not show bimodality; representations of motion (B) do in IT, V4 and non-invasive data, but not in frontal and dorsal areas. (C, E). Correlation of representational similarity between SUA, MUA and LFP in all areas with differences in bimodality. In case of color (C), there is no strong correlation, in case of motion (E), there is a strong anticorrelation. (D, F). Average tuning of individual single units, multi units or LFP channels in MT and V4. Color tuning is unimodal in both areas, motion tuning is bimodal in area V4.
Additional files
-
Transparent reporting form
- https://doi.org/10.7554/eLife.45645.011