Single-amino acid variants reveal evolutionary processes that shape the biogeography of a global SAR11 subclade
Figures
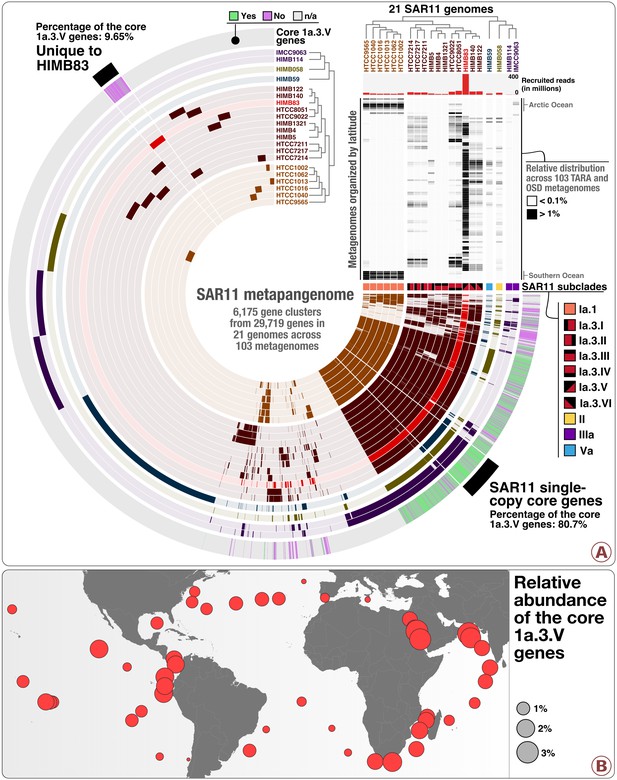
The SAR11 metapangenome.
Panel A describes the pangenome of 21 SAR11 isolate genomes based on the occurrence of 6175 gene clusters, in conjunction with their phylogeny (clade level) and relative distribution of recruited reads in 103 metagenomes ordered by latitude from the North Pole to the South Pole (top right heat map). The relative distributions were displayed for a minimum value of 0.1% and a maximum value of 1%. The layer named ‘Core 1a.3.V genes’ displays the occurrence of the 799 core 1a.3.V genes (in green) and those found in HIMB83 but not in the 1a.3.V lineage (in purple). Panel B describes the relative distribution of reads the 799 core 1a.3.V genes recruited across surface metagenomes from TARA Oceans.
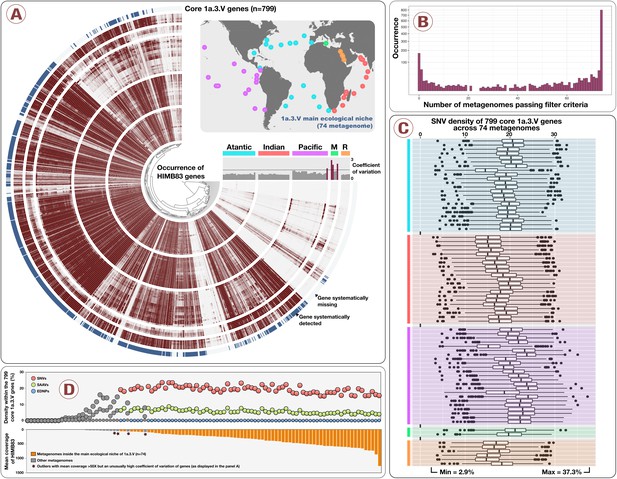
Distribution and diversity of the core 1a.3.
V genes. Panel A displays the relative distribution of HIMB83 genes across 78 metagenomes, along with their coefficient of variation and the selection of 799 core 1a.3.V genes (blue outer circle). A world map provides the location of 74 metagenomes corresponding to the main ecological niche of 1a.3.V metagenomes (four metagenomes were disregarded due to high coefficients of variation). Panel B shows the number of metagenomes in which genes were consistently present. Genes were considered to be present in a metagenome consistently only if their coverage was within a factor of 5 of the average coverage of all genes for that metagenome. Those that passed the filter criteria in all 74 metagenomes (far right) were defined as the core 1a.3.V genes. Panel C displays the SNV density of core 1a.3.V genes across these 74 metagenomes. SNV density varied between 2.9% and 37.3% across genes and metagenomes. Panel D summarizes the heterogeneity extent of the core 1a.3.V genes within the population main ecological niche. Specifically, the panel displays the density of single nucleotide variants (>1% from consensus), environmentally disconnected nucleotide position (i.e., positions stable in the environment but differing from the reference,<1% from consensus) and single amino acid variants (>10% from consensus) within the core genes of 1a.3.V across 103 metagenomes as a function of the mean coverage of HIMB83.
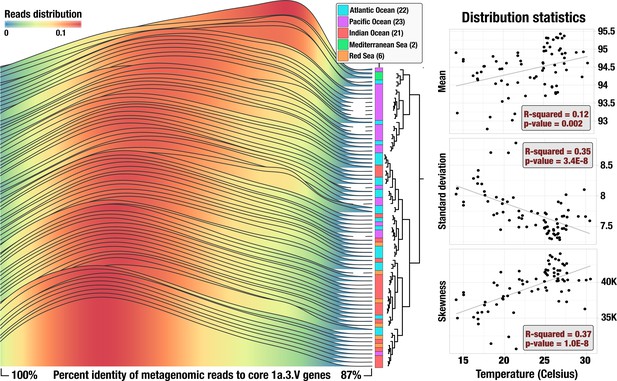
Statistics of recruited reads.
Left panel shows percent identity distributions in each of the 74 metagenomes. Curves are colored based on height. Metagenomes are ordered according to how the percent identity distributions hierarchically cluster based on Euclidean distance (dendrogram). Right panels display a summary of distribution statistics for each percent identity distribution compared against in situ temperature in a linear regression (correlations to all other available parameters are summarized in Figure 2—figure supplement 2). Each point is a metagenome and black lines are lines of best fit. For visual clarity, the data in left panel considers only the median read length and interpolates between data points, whereas the data in right panels consider all read lengths with no interpolation.
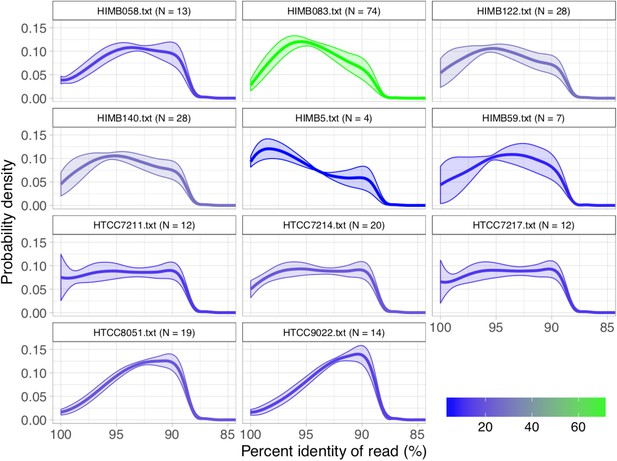
Percent identity distributions resulting from the competitive mapping experiment of the metagenomic short reads onto the 21 SAR11 reference genomes.
For each reference genome, metagenomes were only included if they recruited at least 50X coverage. 10 references failed to recruit 50X coverage in any metagenome and were excluded from the plot. Curves were colored according to , the number of metagenomes passing the 50X threshold, and each curve represents the mean distribution of these metagenomes, where the shaded area reflects the ± standard deviation. For visual clarity, the data only include reads equal to the median read length.
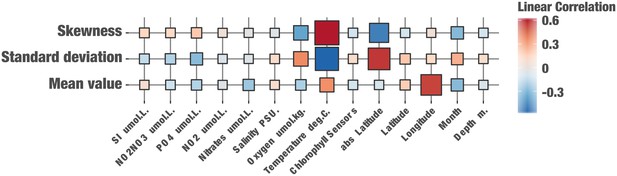
A matrix illustrating the degree of correlation (via linear regression) between oceanic metadata and the statistics (mean, standard deviation, skewness) of the percent read identity distributions of reads recruited by HIMB83 for the 74 metagenomes in which HIMB83 was covered at least 50X.
For example, cells in the temperature column of this matrix quantify the linear correlation coefficients of the scatterplots shown in Figure 2B. Cell colors correspond to their linear correlation coefficient and sizes are proportional to R-squared values. We did not correct for multiple testing due to the potential for strong inter-dependence of the parameters. Supplementary file 1k reports full numerical statistics including p-values.
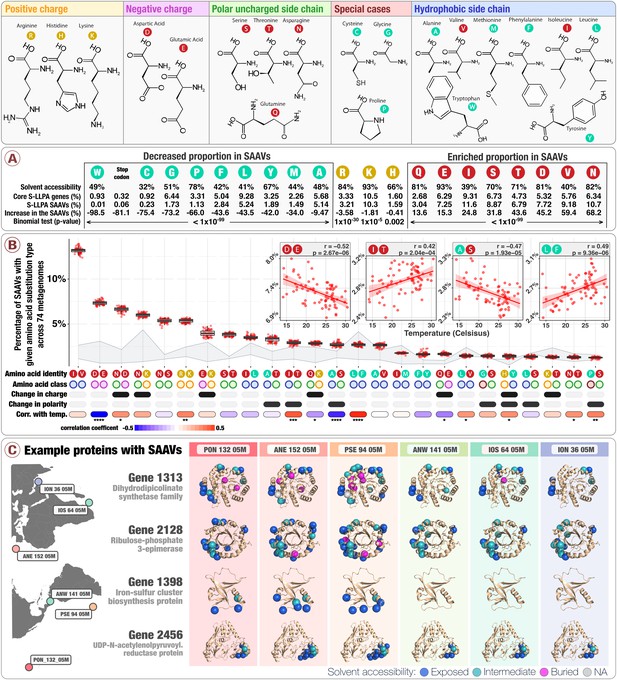
Physico-chemical properties of amino acid variants.
The top panel describes the structure of 20 amino acids grouped by their main chemical properties. Panel A describes the solvent accessibility of amino acids, their relative distribution in both the core 1a.3.V genes and SAAVs, and their percentage increase in SAAVs as compared to the core 1a.3.V genes. The solvent accessibility of amino acids derives from the analysis of 55 proteins (Bordo and Argos, 1991). Panel B describes the relative abundance of the top 25 most prevalent amino acid substitution types (AASTs) across 74 metagenomes (boxplots), along with the classes their amino acids belong to and the correlation coefficient between AAST prevalence and in situ temperature calculated via linear regression (see Figure 3—figure supplement 2 for p-values). The area shaded in light gray shows bounds for the expected frequency distribution given strictly neutral processes. The upper bound is Model one and the lower bound is Model 2 (see Materials and methods). The four insets example the relationship between AAST prevalence and in situ temperature for the AASTs 'aspartic/glutamic acid', 'isoleucine/threonine', 'alanine/serine', and 'leucine/phenylalanine' (Figure 3—figure supplement 2 illustrate similar plots for all 25 of the most prevalent AASTs). The 25 AASTs included in the analysis cover 87.1% of all SAAVs. Panel C displays SAAVs on the predicted protein structures of four core 1a.3.V genes across six metagenomes from distant locations.
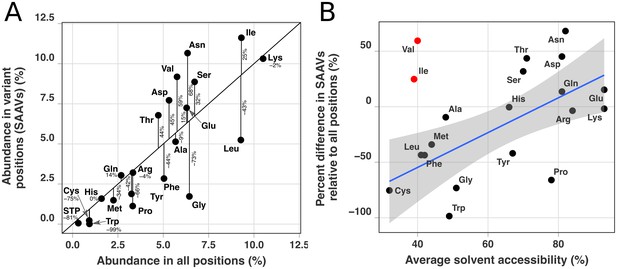
Panel A shows a direct comparison between the amino acid composition in all positions compared to the amino acid composition within SAAVs.
The amino acid composition of all positions (y-axis) was quantified by calculating the frequency that amino acids appeared within the core 1a.3.V genes of the reference sequence, HIMB83, whereas amino acid composition in SAAVs (x-axis) was quantified by calculating the frequency that an amino acid was one of the two dominant alleles across the 738,324 SAAVs. The black diagonal line signifies a one-to-one correspondence between these two variables, and black vertical lines illustrate the deviation of amino acids from this null expectation. Vertical lines are labeled with percent differences between frequencies of amino acids in SAAVs relative to all positions. The linear correlation coefficient of panel A is 0.81, with an R-squared of 0.65, and a probability that no correlation exists of . Panel B shows a comparison between the average solvent accessibility of amino acids (x-axis) to the percent difference between frequencies of amino acids in SAAVs relative to all positions (y-axis). Average solvent accessibilities for amino acids were taken from Table 2 of Bordo and Argos (1991). The linear correlation coefficient of panel B excluding isoleucine and valine (in red) is 0.64, with an R-squared of 0.41, and a probability that no correlation exists of 0.004. Including isoleucine and valine, these values are 0.40, 0.16, and 0.08, respectively. The blue line shows the line of best fit excluding isoleucine and valine, with shaded in regions representing 95% confidence intervals.
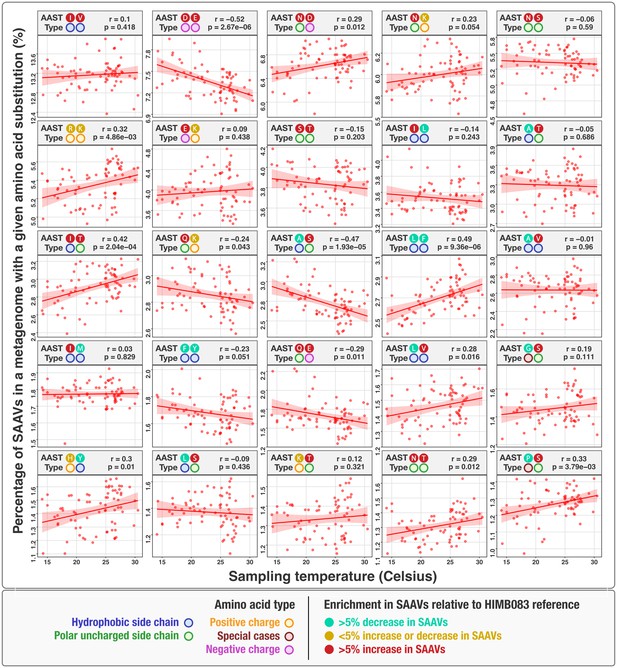
The top 25 most abundant amino acid substitution types (AASTs) and their relationship with in situ temperature.
Each dot represents a metagenome, the x-axis is in situ temperature, and the y-axis is the percentage of SAAVs that were a given AAST. The red line is the line of best fit and the shaded-in region illustrates the 95% confidence interval. The linear correlation coefficient is given as , and the probability of no relationship with temperature is given as (uncorrected for multiple testing). Corrected p-values can be obtained by dividing by 25, the number of linear regressions performed (Bonferonni multiple testing).
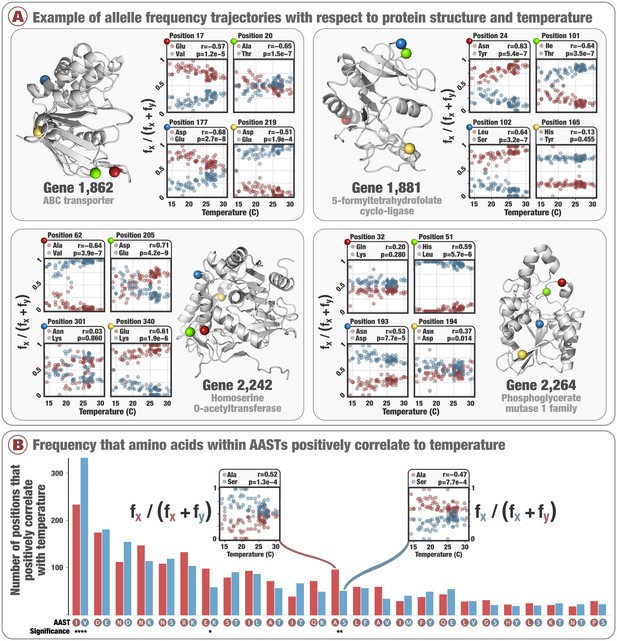
Allele frequency trajectories and in situ temperature.
Panel A illustrates allele frequency trajectories across 74 metagenomes with respect to temperature for 16 manually chosen SAAV positions in the context of protein structures predicted from the core 1a.3.V genes. Only the two most abundant amino acids were considered for each SAAV. The linear correlation coefficient is denoted as and the probability that no correlation exists is denoted as (Benjamini–Hochberg corrected p-values <0.05 (i.e., false discovery rate of 5%)). is defined such that a positive value refers to the first amino acid (in dark red) positively correlating with temperature. Panel B shows the 4,592 positions within the core 1a.3.V genes that had temperature-correlated allele frequency trajectories (Benjamini–Hochberg corrected p-value <0.05), and the number of times these positions corresponded to the top 25 most prevalent AASTs. On the x-axis are the 25 most prevalent AASTs, where red and blue bars indicate the number of times the first and second amino acid, respectively, had allele frequency trajectories that positively correlated with temperature. The insets illustrate two example allele frequency trajectories for the AAST 'alanine/serine'.
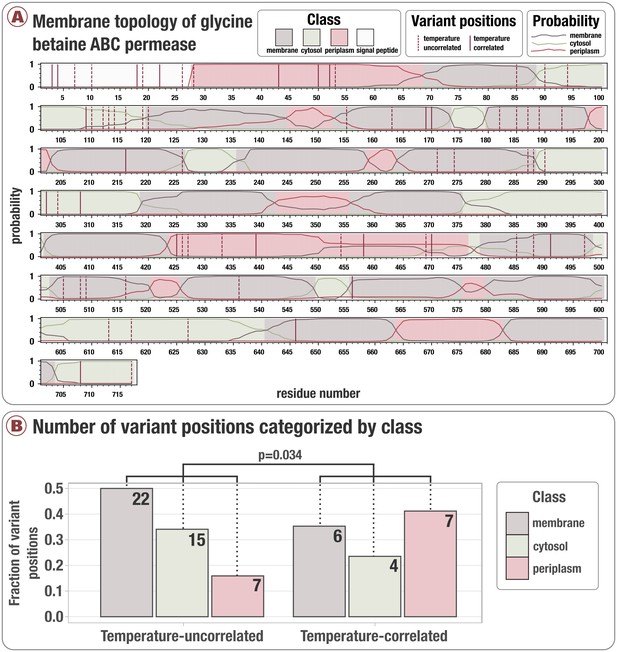
Analysis of how temperature-correlated variant positions distribute within Gene 1727, a glycine betaine ATP-binding cassette permease subunit identified for its rare proportion of temperature-correlated variant positions.
Panel A illustrates the membrane topology predicted by Phobius (See Materials and methods), which associates to each position a probability it is periplasmic, cytosolic, transmembrane, or within the signaling peptide (colored lines). We categorized each position according to the maximum probability (shaded regions). Temperature-correlated and uncorrelated variant positions are denoted by solid red and dashed red vertical lines, respectively. Panel B illustrates the frequency that variant positions are found in the membrane, cytosol, and periplasm, based on whether or not they correlate with temperature. The y-axis is the fraction of variant positions observed (excluding positions observed in the signaling peptide), and numbers within each bar denote the number of variant positions observed within each class. The probability that positions are distributed independent of temperature correlation was 0.034. Formally, this is the probability that temperature-correlated positions were distributed according to a trinomial distribution with a probability vector equal to the empirical distribution observed in temperature-uncorrelated positions.
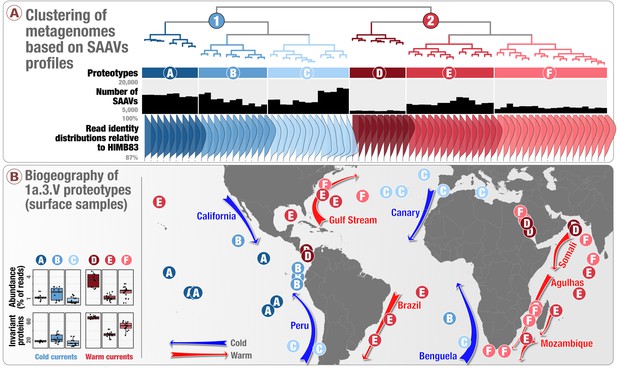
Biogeography of SAR11 subclade 1a.3.V based on single amino acid variants.
Panel A describes the organization of 74 metagenomes based on 57,277 codon position-specific AASTs affiliated with 37,415 unique codon positions and summarizes the number of detected SAAVs and percent identity of reads HIMB83 recruited for each metagenome. The world map in panel B displays the geographic partitioning of the two main metagenomic groups and six proteotypes. Panel B also describes the relative abundance of 1a.3.V and the number of invariant proteins across the six proteotypes.
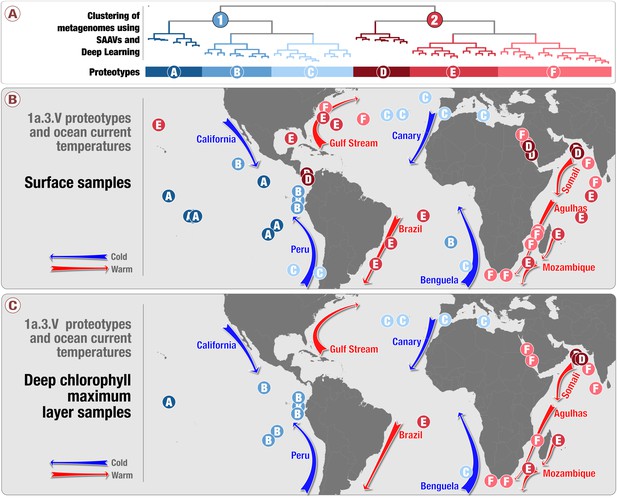
A comparison of the geographic partitioning of the 1a.3.
V groups and proteotypes between metagenomes sampled from the surface versus those sampled from the deep maximal chlorophyll layer. Panels A and B contain the same information as shown in Figure 4, and panel C shows the locations of deep maximal chlorophyll layer metagenomes and the proteotypes to which they belong.
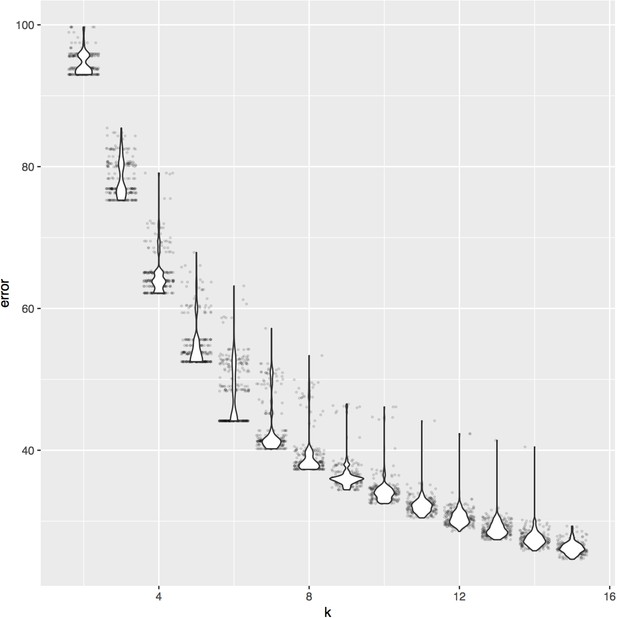
K-means clustering results (250 iterations) of the Deep Learning distance metric of 74 metagenomes based on the coordinates and identity of 738,324 SAAVs.
The elbow of this curve is k = 6, which informed our decision to define six proteotypes.
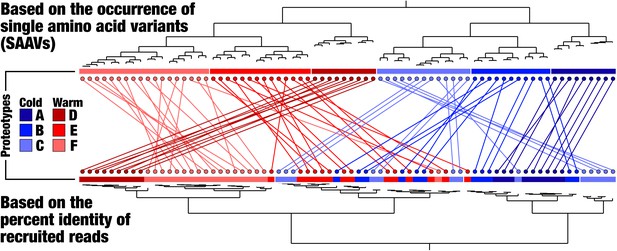
A comparison of dendrograms that organize metagenomes based on the genomic variability observed in the core 1a.3.
V genes. Above, the metagenomes are organized by applying a novel graph-based activity regularization technique for competitive learning from hyper-dimensional data to the 738,324 SAAVs (see Materials and methods). Below, the metagenomes are organized by a less comprehensive approach in which the percent identity distributions are hierarchically clustered via Euclidean distance. The first method therefore is sensitive to the identity and positions of amino acid variability, whereas the second method is based solely on a summary statistic that quantifies the degree of sequence divergence at the DNA level and is agnostic to position and identity. Each metagenome is connected to itself through a straight line and is colored according to the proteotype to which it belongs.
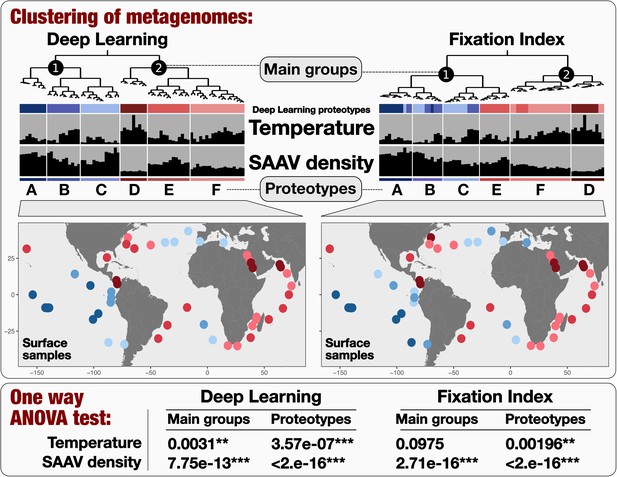
Biogeography of SAR11 subclade 1a.3.
V based on single amino acid variants using Deep Learning (left) and Fixation Index (right) (see Material and methods). The world maps display the geographic partitioning of six proteotypes based on the two methodologies. Finally, ANOVA tests determine whether there are statistically significant differences between the means of either in situ temperature or SAAV density across (1) the two main groups and (2) the six proteotypes as inferred from the two methodologies.
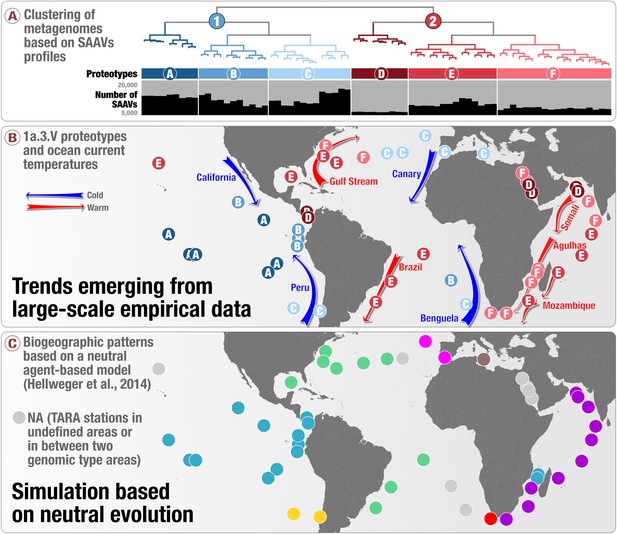
Geographic partitioning of SAR11 by matching surface metagenomes analyzed in our study to simulated results determined using a neutral-agent based model (Hellweger et al., 2014).
The figure emphasizes biogeographic differences between this simulation focused on neutral evolution and our large-scale empirical analysis.
Additional files
-
Supplementary file 1
Details of SAR11 genomes, marine metagenomes, and metagenomic read recruitment results.
(a) Summary of 103 Tara Oceans and Ocean Sampling Day metagenomes. (b) Features of 21 SAR11 isolate genomes, clades to which they belong, and their genome-level metagenomic read recruitment summaries. (c) Comprehensive summary of metagenomic read recruitment results per SAR11 genome, including the number of recruited reads, mean coverage, relative distribution, as well as the detection of 21 SAR11 genomes across 103 metagenomes, along with the total number of single nucleotide variants (SNVs) and single amino acid variants (SAAVs) identified in each metagenome. (d) Summary of the SAR11 metapangenome and gene cluster membership statistics. (e) Presence/absence summary of gene clusters across SAR11 genomes. Distances between SAR11 genomes based on (f) their full-length 16S ribosomal RNA genes and (g) whole genome average nucleotide identity. (h) Relative distribution and abundance of 21 SAR11 genomes, 31 Prochlorococcus genomes, and 957 additional marine population genomes across from three studies across 103 metagenomes. (i) SAR11 genomes with more than 50X coverage across Tara Oceans Project and Ocean Sampling Day metagenomes. (j) Detection of individual HIMB83 genes across metagenomes and whether they belong to 1a.3.V core or not. (k) Summary of the degree of correlation between percent identity histograms of metagenomics reads recruited by HIMB83 and environmental data reported per metagenome. (l) SNV and SAAV density of HIMB83 across metagenomes. This table also reports the predicted functions of individual HIMB83 genes and their DNA sequences, and functional summary of HIMB83 genes that represent core 1a.3.V genes and those that belong to environmental accessory genes of HIMB83.
- https://doi.org/10.7554/eLife.46497.018
-
Supplementary file 2
Details and raw data for single-nucleotide variants, single-amino acid variants, and amino acid substitution types.
(a) SNV density of core 1a.3.V genes, (departure from consensus of >1%). (b) SNV density of core 1a.3.V genes (departure from consensus of >10%) (c) SAAV density of core 1a.3.V genes (departure from consensus of >10%). (d) Number of SAAVs among core 1a.3.V genes. (e) Distribution of invariant core 1a.3.V proteins across metagenomes. (f) Frequency and proportion of amino acids in SAAVs. (g) Comprehensive summary statistics and ratios for amino acid substitution types (AASTs) across metagenomes. (h) Unique coordinates per AAST in 738,324 core 1a.3.V genes (cAASTs) (1) that were covered more than 20X across 74 metagenomes, (2) and in which a divergence >10% from consensus was observed in the frequency of amino acids. (i) cAASTs across metagenomes. This table also reports BLOSUM estimates per AAST, and BLOSUM AAST summary statistics across core 1a.3.V genes.
- https://doi.org/10.7554/eLife.46497.019
-
Supplementary file 3
Relationships between SAAVs and protein structures and temperature.
(a) SAAV characteristics, including solvent accessibility for each SAAV belonging to a core 1a.3.V gene with a successfully predicted protein structure. (b) The correlation of allele frequencies to temperature for each position containing at least one SAAV. (c) The summary of the proportion of temperature-correlated and temperature-uncorrelated SAAV positions per core 1a.3.V gene.
- https://doi.org/10.7554/eLife.46497.020
-
Supplementary file 4
Details of proteotypes within 1a.3.V.
(a) Deep Learning-estimated distances between 74 metagenomes based on cAASTs reconstructed from 738,324 SAAVs. (b) Six 1a.3.V proteotypes. (c) Summary of all data per metagenome to estimate significant determinants of proteotype organization. (d) ANOVA test statistic per sample data category given the six proteotypes.
- https://doi.org/10.7554/eLife.46497.021
-
Transparent reporting form
- https://doi.org/10.7554/eLife.46497.022