Self-organization of modular network architecture by activity-dependent neuronal migration and outgrowth
Figures
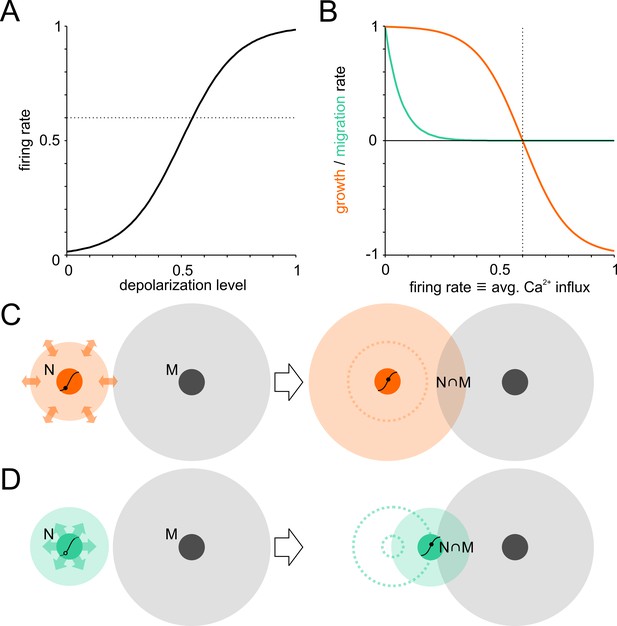
Model of activity-dependent network development.
Neuronal wiring strategies may involve expansion of neurite fields and migration towards other neurons to increase connectivity modeled as neurite field overlap. (A) Transfer function of membrane depolarization between resting and maximal potential to firing rates. Dotted line: target firing rate. (B) Neurite growth (orange) and migration (green) were modulated as a function of [Ca2+]i that corresponded to average firing rates. Neurites grew while the firing rate (corresponding to long-term average Ca2+ influx) was below target and were pruned when above it. Migration rate decreased as neurons approached the target firing rate (dotted line). (C) The area of neurite field overlap, corresponding to connectivity in the model, can be increased by neurite outgrowth and neuronal migration towards neighboring neurons (D).
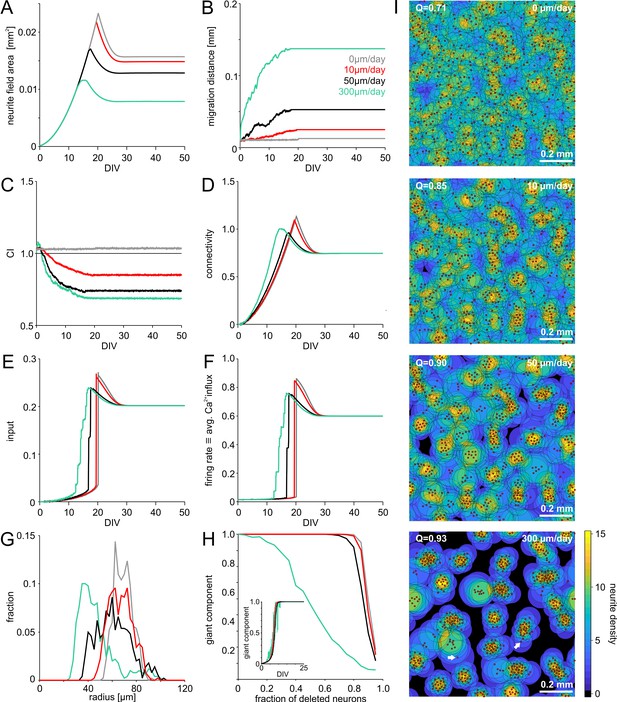
Model of activity-dependent growth and migration.
(A) Activity-dependent growth produced a characteristic overshoot and subsequent pruning of neurite fields. The overall size of developing neurites decreased with increasing migration rates and clustering. (B) Mean migration distance of neurons after seeding (smoothed by 1 hr sliding average). (C) Migration promoted clustering of neurons, which saturated with the onset of network activity and neurite pruning (curves smoothed by 1 hr sliding average). All networks were initialized with the same spatial cell body distribution with CI close to 1. Note that the fluctuations for zero migration results from the random jittering of neuron positions by half the cell body radius (6 µm). (D) Average connectivity increased more rapidly with stronger migration and clustering. (E) Input increased faster with increasing migration rate because clustering initially promoted connectivity. Input levels eventually converged. (F) Firing rates increased sharply once critical input levels were attained. Migration and clustering accelerated the onset of activity. With increasing migration, steps arise because of incremental integration and activation of clusters within the larger network. Note that clustering reduced the developmental overshoot of firing rates. (G) Moderate migration and clustering produced the highest variability of neurite field size across neurons in mature networks. (H) High migration rates increased modularity in mature networks. With increasing migration rate, the giant component more rapidly decreased in clustered networks when a certain fraction of neurons was randomly deleted, indicating that these networks break into disconnected subnets. Inset: the fraction of neurons in the giant cluster, that is the largest connected subnetwork, evolved similarly in different migration conditions. (I) Migration rates crucially determined the mesoscale architecture and modularity (increasing Q indicates stronger modularity) of developing networks. While average neurite fields were small in clustered networks, more isolated neurons generated larger fields (arrows) and formed bottlenecks for activity propagation by connecting otherwise unconnected or weakly connected subnetworks.
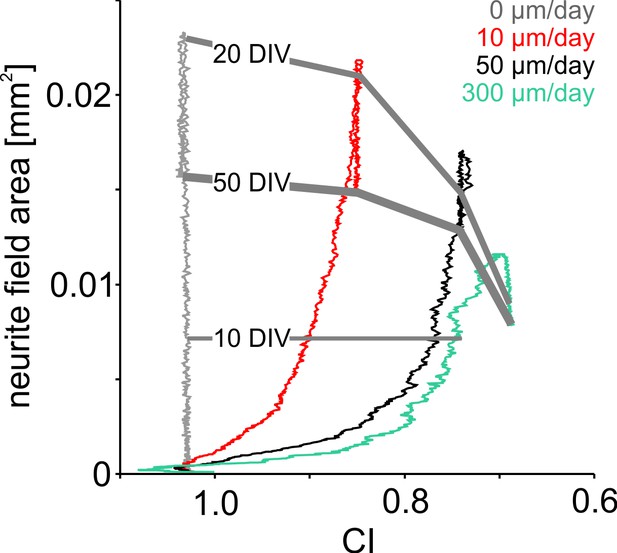
Influence of neuronal clustering on neurite field development.
Increasing migration rates in the simulation promoted neuronal clustering leading to lower final CI and smaller neurite field sizes. At the onset of high network activity, neurite fields were pruned and CI did not change further.
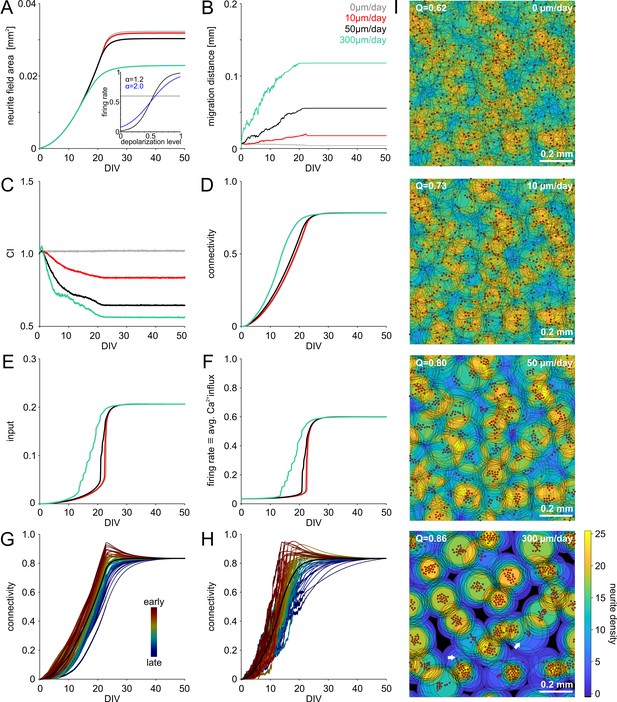
Simulation of saturating network growth.
(A) Decreasing the slope of the sigmoidal transfer function mapping membrane potential to firing rate resulted in saturating growth (inset: blue: = 0.2) instead of an overshoot (inset: black, = 0.12, see Figure 2) in average neurite field size and connectivity. Note that the synaptic weight factor was reduced ( = 0.05 instead of 0.1) to compensate for the increased baseline firing associated with higher . Average neurite fields saturated at different levels depending on the rate of migration, average migration distance (B) and degree of clustering (C). (D) Connectivity increased faster with clustering as in the case of = 0.12. Network-activity, characterized by the average synaptic input (D) and the firing rate (F) increased earlier and more gradually with clustering. (G) Although there was no overshoot of connectivity on average (black line), neurons with faster increase of connectivity showed overshoot and pruning when the network firing rate rapidly increased. In the same network, neurons with slowly increasing connectivity displayed saturating growth. Color indicates the order in which 50 randomly chosen neurons attained 75% of their final connectivity. (H) With migration and clustering, connectivity increased more rapidly with the same dependency of the overshoot on early and late developing connectivity. (I) Saturating growth and migration produced mesoscale network architectures ranging from homogeneous to clustered networks similar to those in Figure 2I. Migration promoted clustering and modularization (increasing Q indicates stronger modularity).
Simulated network development with migration rate 0 µm/day.
https://doi.org/10.7554/eLife.47996.006Simulated network development with migration rate 10 µm/day.
https://doi.org/10.7554/eLife.47996.007Simulated network development with migration rate 50 µm/day.
https://doi.org/10.7554/eLife.47996.008Simulated network development with migration rate 300 µm/day.
https://doi.org/10.7554/eLife.47996.009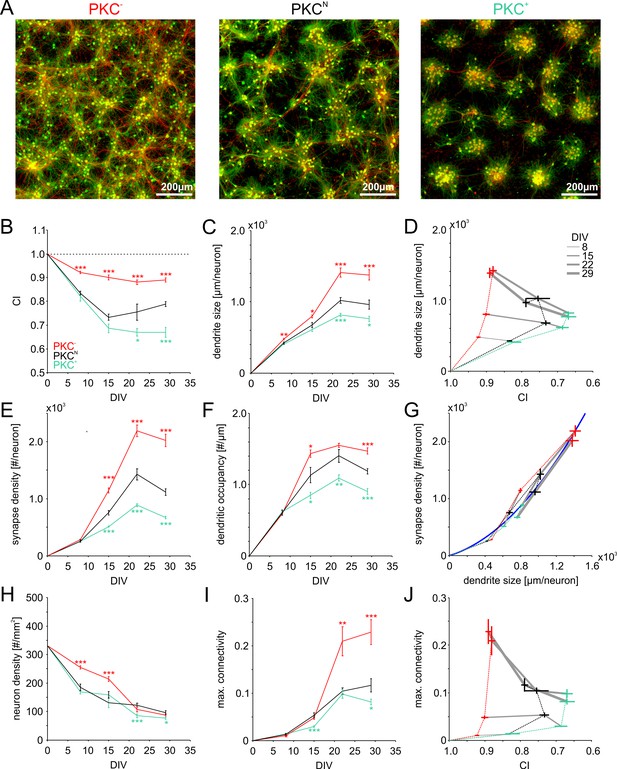
Morphometric analyses of network development.
(A) Dense networks established characteristic mesoscale architectures for the different PKC conditions. PKC− networks had a more homogeneous distribution of axons (red), dendrites (green) and cell bodies (green) than PKCN networks. In PKC+ networks, neurons formed well-delineated clusters. Note that the following morphometric analyses are based on sparser cultures. (B) Decreasing CI during development reflects cell migration and ongoing clustering of neuronal cell bodies until ~15 DIV. PKC+ promoted and PKC− diminished clustering during development. (C) Dendrite size increased until 22 DIV, with boosted growth in PKC− and diminished growth in PKC+ networks. (D) In late development, dendrite size scaled inversely with the degree of clustering. For visualization the CI axis was inversed, so the degree of clustering increases from left to right. (E) The synapse density increased concurrently with dendrite growth. After 22 DIV synapse densities decreased in PKCN and PKC+ networks, indicating synaptic pruning. (F) Dendritic occupancy with synapses differed slightly between conditions and decreased after 22 DIV. (G) The number of synapse per neurons increased with the dendrite size. Gray lines connect networks of the same age. The blue line illustrates a proposed quadratic scaling rule between dendrite size and synapse densities. (H) Neuron density declined with DIV to about one third of the seeding density. (I) Estimated upper bounds for connectivity based on the synapse density and the total number of neurons (on 113 mm2 cover slips). PKC−at least doubled average connectivity. (J) In mature networks, maximum connectivity scaled inversely with clustering. All parameters are presented as mean ± SEM. Data from 4 to 24 images (Table 1, area 3.5 mm2) taken in each of 2 networks per condition and age. Asterisks indicate p-values ≤0.05 (*), ≤0.01 (**) and ≤0.001 (***) tested against PKCN.
-
Figure 3—source data 1
Source data and Matlab script for Figure 3B,C,E,F,H,I.
- https://doi.org/10.7554/eLife.47996.013
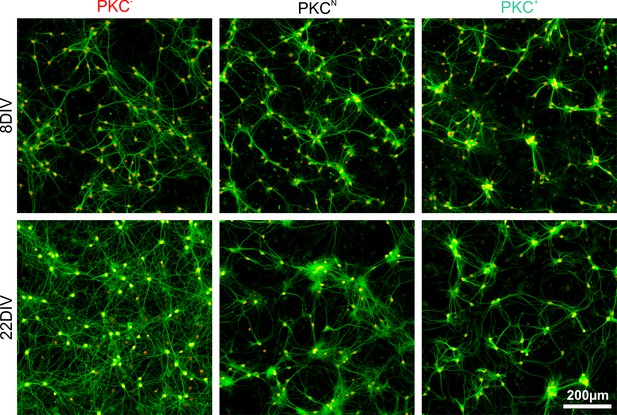
Clustering and dendrite development in sparse networks.
Exemplary images with neuronal nuclei (NeuN, red) and dendrites (MAP2, green) stained at 8 and 22 DIV. PKC inhibition diminished and PKC stimulation promoted neuronal migration and clustering of neurons during development. Dendrite growth changed with the spatial arrangement of cell bodies. Homogeneous cell body arrangements correlated with larger dendrites, whereas clusters had local dendrite tangles and dendrite bundles reaching to other clusters.
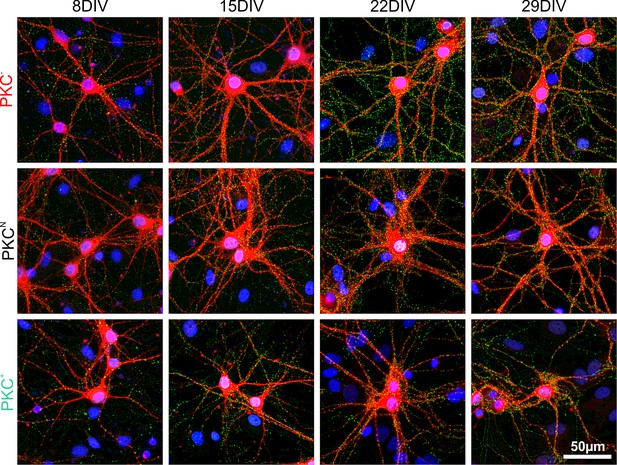
Development of synapses in sparse networks.
Immunohistochemical staining of dendrites (MAP2, red), presynaptic compartments (synapsin, green) and cellular nuclei (DAPI, blue). Synapse densities on dendrites increased in all PKC conditions up to 22 DIV and slightly decreased afterwards. Overall synapse densities were highest in the PKC− and lowest in PKC+ networks.
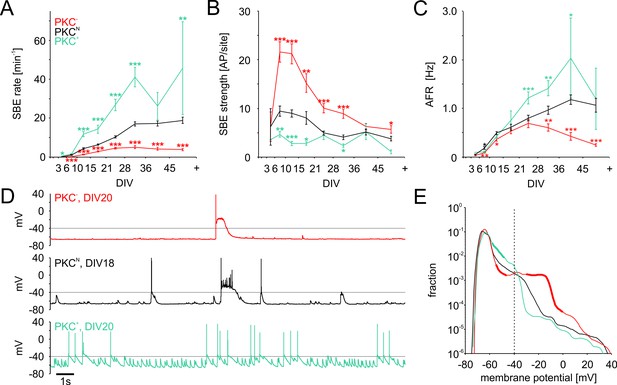
Development of spontaneous network activity.
(A) SBE rates gradually increased during development until 28 DIV, which was accelerated in clustered PKC+ networks and decelerated in homogeneous PKC− networks. In result, SBE rates differed considerably in mature networks and increased with the degree of clustering. X-axis ticks indicate bin boundaries. (B) PKC− networks generated stronger SBEs with more APs per site than clustered networks, compensating lower SBE rates to some extent. Burst strength increased initially but declined later on, putatively because of the maturation of inhibition. (C) AFR increased comparably during early development in the different PKC conditions, indicating that stronger bursting compensated lower SBE rates. Later in development, AFRs were increased in PKC+ and reduced in PKC− networks. (D) Neurons in PKC− networks showed strong depolarization during SBEs that reached well above the spiking threshold around −40 mV causing depolarization block of spiking. In clustered networks, neurons displayed higher membrane potential fluctuations below threshold that occasionaly passed the threshold leading to spikes. (E) Membrane potential distribution. Thick lines indicate regions significantly different from PKCN (p≤0.05). The fraction of time in which neuronal membrane potentials were above the spiking threshold (dashed line) was significantly increased in PKC− networks compared to PKCN and PKC+ networks. Data in A-D and F show mean ± SEM derived from 1 hr recording sessions. Asterisks indicate p-values ≤0.05 (*), ≤0.01 (**) and ≤0.001 (***) tested against PKCN. The number of recordings per age and condition is provided in Table 2.
-
Figure 4—source data 1
Source data and Matlab script for Figure 4A–C,E.
- https://doi.org/10.7554/eLife.47996.019
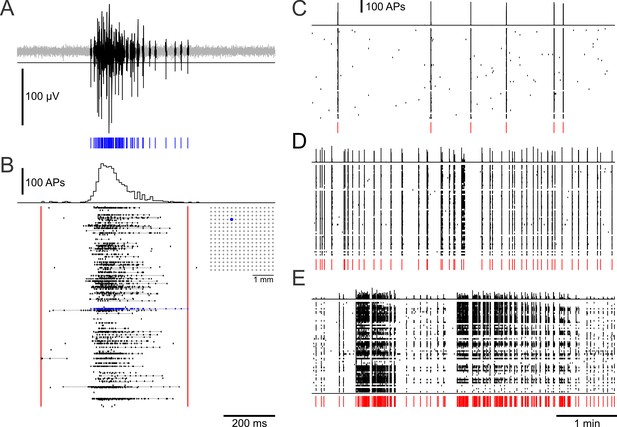
Sample MEA recordings from dense networks.
(A) SBE spike activity on a MEA electrode. Spike times (blue) were detected with a voltage threshold on high-pass filtered raw data. (B) Global spike histogram (10 ms bins) and spike activity of a PKCN network recorded with a 16 × 16 MEA (inset) at 11 DIV. Most spikes occurred in bursts (gray lines connect spikes assigned to a burst) that were typically organized in SBEs. Red lines indicate SBE onset and offset. Blue: electrode shown in A. (C–E) Global spike histogram (top row) and raster plot of typical activity in networks with different mesoscale architecture at 22–23 DIV (58–59 active sites; 6 × 10 MEAs). C SBEs (red ticks) in PKC− networks had high PFRs and typically occurred in regular intervals at low rates. (D) PKCN networks displayed a wide range of dynamics. The example shows SBEs occuring at intermediate rates and in irregular intervals. (E) Bursts of bursts, that is superbursts, occurred in PKCN and were very prominent in PKC+ (shown), but not in PKC− networks.
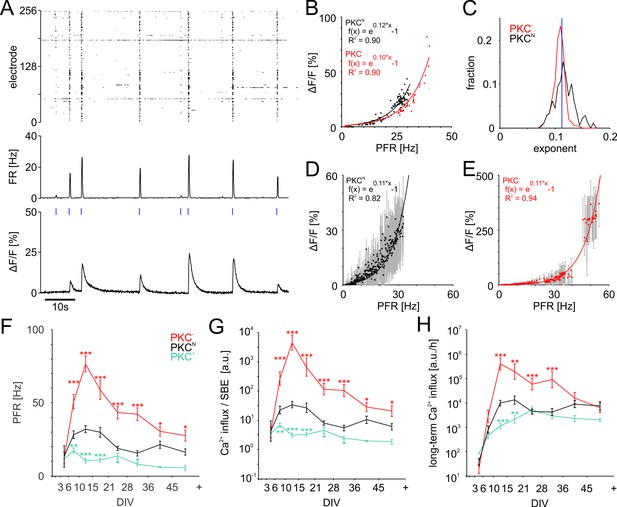
PFR-dependent Ca2+ gain.
(A) Spiking raster and firing rate averaged across all electrodes within 40 ms bins (synchronized to the frame times of the Ca2+ measurement) and Ca2+ signal for one neuronal soma at 19 DIV in a PKCN network. Blue ticks: SBE onsets. (B) The amplitude of Ca2+ transients (shown for the PKCN neuron in A and a PKC− neuron at 20 DIV) scaled exponentially (solid line) with PFR. (C) Exponents had a narrow distribution and were slightly higher in PKCN (p=3.2*10−18) than in PKC− conditions (PKCN: 179 neurons, 5 networks at 19 DIV, 714 SBEs total, mean and standard deviation of exponent = 0.12 ± 0.02; PKC−: 622 neurons, 4 networks at 20 DIV, 248 SBEs total, exponent = 0.11 ± 0.01). Blue: average exponent (0.11 ± 0.01) for the entire data set. (D) Ca2+ amplitudes scaled exponentially with PFRs across many neurons in these PKCN and PKC− networks (E). The data represent median (data points) and standard deviation (error bars) of Ca2+ amplitudes, averaged across neurons for a given PFR range (bin size 0.1 Hz). (F) PFR assessed during SBEs were higher in homogeneous networks and lower in clustered networks. PFR decreased after week 3, putatively with the maturation of inhibition. (G) Prediction of the development of average Ca2+ influx per SBE estimated as (Figure 5D). (H) Average Ca2+ influx per minute, estimated from all SBEs in 1 hr recording sessions, suggests that long-term average Ca2+ influx in different PKC conditions converged at network maturation. Data in G and H are presented as mean ± SEM. Asterisks indicate p-values ≤0.05 (*), ≤0.01 (**) and ≤0.001 (***) tested against PKCN.
-
Figure 5—source data 1
Source data and Matlab script for Figure 5B–E,F.
- https://doi.org/10.7554/eLife.47996.022
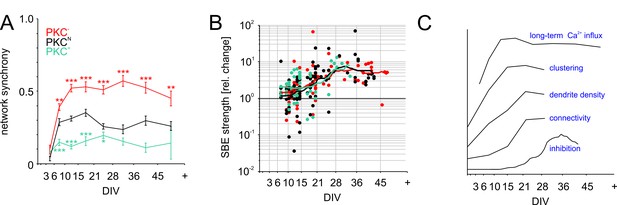
Functional aspects of network maturation.
(A) Network synchrony (average spike train correlation for all electrode pairs determined with 30 ms time bins, mean ± SEM) stabilized early in development in all PKC conditions but was significantly higher in homogeneous networks and significantly lower in clustered networks. Asterisks indicate p-values ≤0.05 (*), ≤0.01 (**) and ≤0.001 (***) tested against PKCN. (B) Inhibition was probed by acute blockade of GABA-A receptors with PTX. In early networks, PTX had highly variable impact on SBE strength (<14 DIV) but significantly increased it after 21 DIV in all network types. The maturation of inhibition was comparable across PKC conditions. (C) Illustration of the time course of key differentiation processes shown in detail in Figures 3 and 5 in relation to the development of long-term average Ca2+ influx. Morphological parameters that showed a similar time course across PKC conditions were normalized to final levels to visualize the relative change during development. The maturation of inhibition is shown as average relative change in SBE strength upon PTX appliction. Y-axis scaling is linear for all graphs. An offset was added for visualization.
-
Figure 6—source data 1
Source data and Matlab script for Figure 6A,B.
- https://doi.org/10.7554/eLife.47996.024
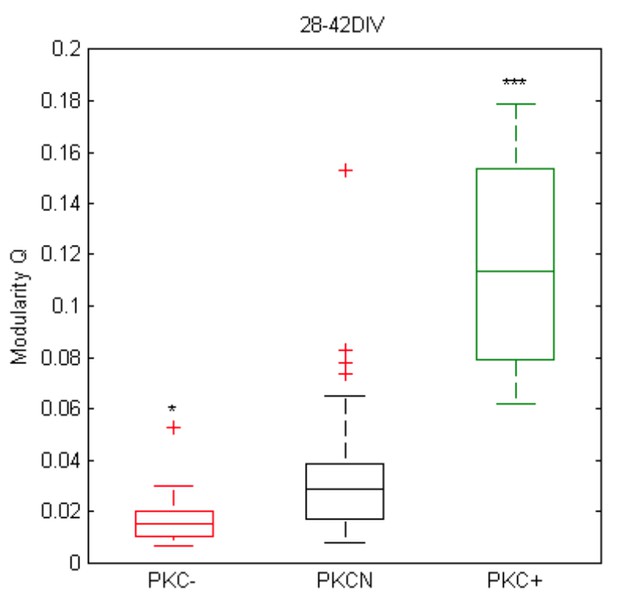
Tables
Morphometric analysis of network development under different PKC conditions.
Results are presented as mean ± standard error of mean (SEM). Significance was determined against PKCN, or between specified developmental time windows, using independent Student’s t-test. N specifies the number of analyzed images taken from two networks per PKC condition and age.
DIV | PKC- | PKCN | PKC+ | unit | |
---|---|---|---|---|---|
clustering index | |||||
8 | 0.92 ± 0.01 (1.8*10−10) | 0.84 ± 0.01 | 0.82 ± 0.02 (6.1*10−1) | CI | |
15 | 0.9 ± 0.01 (1.5*10−6) | 0.73 ± 0.01 | 0.69 ± 0.02 (1.1*10−1) | CI | |
22 | 0.88 ± 0.01 (4.4*10−4) | 0.75 ± 0.03 | 0.67 ± 0.02 (3.3*10−2) | CI | |
29 | 0.89 ± 0.01 (8.0*10−9) | 0.79 ± 0.01 | 0.67 ± 0.02 (2.1*10−5) | CI | |
8 vs. 22 | −4.49 (3.5*10−4) | −9.65 (3.1*10−2) | −18.6 (1.2*10−5) | % change | |
22 vs. 29 | 1.04 (4.9*10−1) | 4.5 (2.7*10−1) | 0.01 (1.0) | % change | |
Dendrite size | |||||
8 | 476 ± 9 (1.3*10−3) | 421 ± 12 | 408 ± 8 (3.4*10−1) | µm | |
15 | 797 ± 22 (1.2*10−2) | 676 ± 37 | 610 ± 30 (2.1*10−1) | µm | |
22 | 1413 ± 64 (7.9*10−5) | 1021 ± 41 | 816 ± 24 (3.6*10−4) | µm | |
29 | 1380 ± 74 (1.9*10−4) | 962 ± 65 | 760 ± 37 (1.3*10−2) | µm | |
8 vs. 22 | 196.59 (4.0*10−20) | 142.46 (9.1*10−12) | 100.08 (1.4*10−15) | % change | |
22 vs. 29 | −2.38 (7.4*10−1) | −5.81 (5.0*10−1) | −6.86 (2.5*10−1) | % change | |
Synapse density | |||||
8 | 281 ± 11 (2.2*10−1) | 255 ± 18 | 254 ± 14 (9.4*10−1) | # | |
15 | 1142 ± 44 (2.3*10−4) | 754 ± 39 | 510 ± 9 (2.4*10−5) | # | |
22 | 2188 ± 100 (2.1*10−5) | 1427 ± 99 | 885 ± 27 (3.4*10−5) | # | |
29 | 2019 ± 110 (4.5*10−8) | 1114 ± 56 | 669 ± 21 (5.7*10−8) | # | |
8 vs. 22 | 678.77 (4.7*10−24) | 458.69 (2.1*10−10) | 248.68 (1.2*10−17) | % change | |
22 vs. 29 | −7.75 (2.7*10−1) | −21.93 (6.7*10−3) | −24.35 (1.2*10−6) | % change | |
Dendritic occupancy | |||||
8 | 0.59 ± 0.02 (6.4*10−1) | 0.6 ± 0.04 | 0.62 ± 0.03 (7.5*10−1) | #/µm | |
15 | 1.44 ± 0.05 (1.7*10−2) | 1.13 ± 0.11 | 0.85 ± 0.04 (1.7*10−2) | #/µm | |
22 | 1.55 ± 0.03 (9.6*10−2) | 1.4 ± 0.09 | 1.09 ± 0.04 (5.7*10−3) | #/µm | |
29 | 1.47 ± 0.04 (3.0*10−5) | 1.19 ± 0.04 | 0.9 ± 0.04 (2.3*10−5) | #/µm | |
8 vs. 22 | 163.83 (7.2*10−28) | 132.26 (9.0*10−8) | 76.65 (3.7*10−10) | % change | |
22 vs. 29 | −5.06 (1.5*10−1) | −15.41 (2.3*10−2) | −17.45 (3.8*10−3) | % change | |
Neuron density | |||||
8 | 255 ± 6 (9.6*10−7) | 185 ± 11 | 168 ± 7 (2.0*10−1) | #/mm2 | |
15 | 214 ± 9 (5.9*10−4) | 131 ± 17 | 158 ± 12 (2.0*10−1) | #/mm2 | |
22 | 107 ± 8 (1.9*10−1) | 123 ± 6 | 85 ± 6 (5.7*10−4) | #/mm2 | |
29 | 87 ± 5 (3.0*10−1) | 96 ± 7 | 77 ± 4 (2.6*10−2) | #/mm2 | |
8 vs. 22 | −58.03 (7.3*10−17) | −33.66 (1.1*10−4) | −49.42 (1.0*10−8) | % change | |
22 vs. 29 | −18.93 (4.5*10−2) | −21.71 (1.3*10−2) | −9.79 (2.6*10−1) | % change | |
Maximum connectivity | |||||
8 | 0.01 ± 0.001 (3.5*10−2) | 0.013 ± 0.001 | 0.014 ± 0.001 (6.3*10−1) | fraction | |
15 | 0.048 ± 0.003 (4.6*10−1) | 0.053 ± 0.006 | 0.029 ± 0.002 (9.2*10−4) | fraction | |
22 | 0.209 ± 0.031 (8.2*10−3) | 0.104 ± 0.007 | 0.098 ± 0.009 (5.9*10−1) | fraction | |
29 | 0.229 ± 0.026 (9.2*10−4) | 0.116 ± 0.014 | 0.081 ± 0.006 (3.8*10−2) | fraction | |
8 vs. 22 | 1987.43 (5.9*10−10) | 701.18 (2.6*10−11) | 604.32 (1.4*10−10) | % change | |
22 vs. 29 | 9.31 (6.3*10−1) | 11.48 (5.2*10−1) | −17.23 (1.3*10−1) | % change | |
N | |||||
8 | 24 | 11 | 15 | ||
15 | 9 | 4 | 7 | ||
22 | 15 | 11 | 11 | ||
29 | 17 | 16 | 15 |
-
Table 1—source data 1
Source data and Matlab script.
- https://doi.org/10.7554/eLife.47996.015
-
Table 1—source data 2
Source data and Matlab script.
- https://doi.org/10.7554/eLife.47996.016
Electrophysiological characterization of network activity during development.
Data were pooled within defined developmental time windows. Significance was determined against PKCN using independent Student’s t-test. N specifies the number of recorded networks per PKC condition and age.
DIV | PKC− | PKCN | PKC+ | |
---|---|---|---|---|
AFR | ||||
3–5 | 0.03 ± 0.01 (1.0*10−1) | 0.05 ± 0.01 | 0.09 (1.4*10−1) | |
6–9 | 0.08 ± 0.01 (2.6*10−3) | 0.18 ± 0.03 | 0.1 ± 0.02 (4.0*10−2) | |
10–14 | 0.36 ± 0.04 (1.8*10−2) | 0.49 ± 0.03 | 0.42 ± 0.04 (2.0*10−1) | |
15–20 | 0.53 ± 0.06 (3.6*10−1) | 0.61 ± 0.06 | 0.76 ± 0.19 (3.6*10−1) | |
21–27 | 0.69 ± 0.08 (2.5*10-1) | 0.8 ± 0.06 | 1.21 ± 0.12 (7.3*10−4) | |
28–35 | 0.6 ± 0.07 (2.9*10−3) | 0.96 ± 0.09 | 1.41 ± 0.15 (6.9*10−3) | |
36–44 | 0.42 ± 0.08 (2.4*10−7) | 1.18 ± 0.1 | 2.04 ± 0.83 (4.3*10−2) | |
45+ | 0.24 ± 0.02 (9.9*10−8) | 1.06 ± 0.14 | 1.2 ± 0.63 (8.3*10−1) | |
SBE rate (SBE/min) | ||||
3–5 | 0.17 ± 0.04 (2.9*10−1) | 0.11 ± 0.03 | 0.54 (1.5*10−2) | |
6–9 | 0.18 ± 0.03 (5.4*10−6) | 1.02 ± 0.15 | 1.46 ± 0.19 (7.4*10−2) | |
10–14 | 1.21 ± 0.13 (2.0*10−8) | 4.26 ± 0.44 | 11.69 ± 1.27 (3.4*10−10) | |
15–20 | 2.83 ± 0.35 (1.4*10−8) | 6.36 ± 0.43 | 14.31 ± 2.03 (4.7*10−7) | |
21–27 | 4.58 ± 0.44 (2.4*10−10) | 10.3 ± 0.62 | 26.82 ± 3 (5.5*10−12) | |
28–35 | 4.98 ± 0.81 (2.2*10−13) | 16.97 ± 1.07 | 41.1 ± 5.07 (7.9*10-9) | |
36–44 | 4.08 ± 0.75 (9.0*10−12) | 17.25 ± 1.28 | 26.21 ± 7.05 (8.7*10−2) | |
45+ | 3.74 ± 0.56 (2.2*10−13) | 18.85 ± 1.62 | 45.76 ± 23.86 (2.0*10−3) | |
SBE strength (APs per burst) | ||||
3–5 | 6.2 ± 3 (1.0*100) | 6.2 ± 3.8 | 3.5 (7.6*10−1) | |
6–9 | 21.6 ± 2.1 (9.4*10−7) | 9.5 ± 1 | 4.6 ± 0.6 (1.2*10−3) | |
10–14 | 21.2 ± 2.1 (5.7*10−9) | 9 ± 0.8 | 2.7 ± 0.5 (1.5*10−7) | |
15–20 | 15.2 ± 1.9 (2.1*10−3) | 8 ± 1.3 | 2.7 ± 0.3 (1.2*10−2) | |
21–27 | 10.1 ± 1 (6.1*10−8) | 4.9 ± 0.4 | 4.4 ± 0.9 (5.4*10−1) | |
28–35 | 8.9 ± 0.9 (2.9*10−6) | 4 ± 0.5 | 2.3 ± 0.3 (1.7*10−2) | |
36–44 | 6.2 ± 0.6 (2.2*10−1) | 5.1 ± 0.6 | 5.1 ± 1.6 (9.8*10−1) | |
45+ | 5.6 ± 0.8 (4.2*10−2) | 3.8 ± 0.5 | 1.1 ± 0.4 (2.2*10−1) | |
PFR (Hz) | ||||
3–5 | 12.3 ± 3.8 (9.0*10−1) | 13.2 ± 7.3 | 11.5 (9.2*10−1) | |
6–9 | 50.8 ± 4.8 (6.4*10−5) | 28.3 ± 2.7 | 17.4 ± 1.7 (4.9*10−3) | |
10–14 | 76.6 ± 5.4 (6.7*10−14) | 32.1 ± 2.3 | 10.3 ± 1.4 (2.1*10−9) | |
15–20 | 59.1 ± 6.5 (5.3*10−5) | 29.8 ± 3.4 | 10.9 ± 1.3 (6.4*10−4) | |
21–27 | 43.3 ± 4.1 (7.4*10−10) | 18.9 ± 1.5 | 13.7 ± 2 (4.4*10−2) | |
28–35 | 42.3 ± 4.4 (2.4*10−8) | 15.5 ± 2 | 8.1 ± 1.2 (1.8*10−2) | |
36–44 | 30.5 ± 3.1 (2.7*10−2) | 21.4 ± 2.6 | 6.1 ± 0.4 (1.3*10−1) | |
45+ | 27.5 ± 3.8 (1.5*10−2) | 16.4 ± 2.3 | 5.6 ± 1.7 (2.8*10−1) | |
Network synchrony | ||||
3–5 | 0.1 ± 0.03 (2.6*10−1) | 0.04 ± 0.02 | 0.08 (3.4*10−1) | |
6–9 | 0.39 ± 0.02 (3.3*10−3) | 0.29 ± 0.02 | 0.15 ± 0.02 (3.1*10−4) | |
10–14 | 0.52 ± 0.03 (2.5*10−10) | 0.31 ± 0.02 | 0.12 ± 0.02 (1.4*10−10) | |
15–20 | 0.53 ± 0.04 (4.6*10−5) | 0.35 ± 0.02 | 0.16 ± 0.03 (1.7*10−5) | |
21–27 | 0.51 ± 0.03 (5.8*10−13) | 0.26 ± 0.02 | 0.2 ± 0.02 (4.8*10−2) | |
28–35 | 0.57 ± 0.04 (2.0*10−10) | 0.24 ± 0.03 | 0.15 ± 0.03 (7.6*10−2) | |
36–44 | 0.53 ± 0.04 (1.3*10−5) | 0.3 ± 0.03 | 0.11 ± 0.03 (1.3*10−1) | |
45+ | 0.45 ± 0.05 (2.5*10−3) | 0.26 ± 0.03 | 0.14 ± 0.11 (3.8*10−1) | |
N | ||||
3–5 | 7 | 3 | 1 | |
6–9 | 33 | 40 | 24 | |
10–14 | 70 | 92 | 47 | |
15–20 | 53 | 65 | 27 | |
21–27 | 77 | 121 | 56 | |
28–35 | 47 | 62 | 29 | |
36–44 | 38 | 57 | 4 | |
45+ | 38 | 36 | 2 |
Reagent type (species) or resource | Designation | Source or reference | Identifiers | Additional information |
---|---|---|---|---|
Strain, strain background (Rattus norvegicus domestica) | wildtype wistar rat pups | CEMT, University, Freiburg | ||
Genetic reagent | AAV9.CAG.GCaMP6s.WPRE.SV40 | Penn Vector Core, University of Pennsylvania | V3296TI-R | titer 1e11 |
Antibody | anti-MAP2 (chicken polyclonal) | Abcam, Cambridge, UK | ab92434 RRID:AB_2138147 | 1:500 |
Antibody | anti-NeuN (rabbit polyclonal) | Abcam, Cambridge, UK | ab128886 RRID:AB_2744676 | 1:500 |
Antibody | anti-Neurofilament (mouse monoclonal) | Abcam, Cambridge, UK | ab24571 RRID:AB_448148 | 1:10 |
Antibody | anti-Synapsin (mouse monoclonal) | Synaptic Systems GmbH, Germany | 106001 RRID:AB_887805 | 1:200 |
Antibody | anti-chicken-Cy2 (goat polyclonal) | Abcam, Cambridge, UK | ab6960 RRID:AB_955003 | 1:200 |
Antibody | anti-rabbit-Cy3 (goat polyclonal) | Abcam, Cambridge, UK | ab6939 RRID:AB_955021 | 1:200 |
Antibody | anti-mouse-Cy5 (goat polyclonal) | Abcam, Cambridge, UK | ab6563 RRID:AB_955068 | 1:200 |
Chemical compound, drug | 4,6-diamidino-2-phenyindole, diclactate (DAPI) | Sigma-Aldrich, Germany | D9562 | 1:5000 |
Chemical compound, drug | Gödecke6976 | Tocris Bioscience, Bristol, UK | 2253 | 1 µM |
Chemical compound, drug | Phorbol-12-Myristate-13-Acetate (PMA) | Sigma-Aldrich, Munich, Germany | P1585 | 1 µM |
Chemical compound, drug | Picrotoxin | Tocris Bioscience, Bristol, UK | 1128 | 10 µM |
Chemical compound, drug | DMSO | Sigma-Aldrich, Munich, Germany | D8418 | 0.1% |
Chemical compound, drug | DNase (type IV) | Sigma-Aldrich, Munich, Germany | D5025 | 50 g/ml |
Chemical compound, drug | minimal essential medium | Invitrogen, Karlsruhe, Germany | 21090055 | |
Chemical compound, drug | horse serum (heat-inactivated) | Invitrogen, Karlsruhe, Germany | 26050088 | 20% |
Chemical compound, drug | phosphate buffered saline (PBS) | Invitrogen, Karlsruhe, Germany | 21600010 | |
Chemical compound, drug | glucose | Sigma-Aldrich, Munich, Germany | G7528 | 20 mM |
Chemical compound, drug | L-glutamine | Invitrogen, Karlsruhe, Germany | 25030024 | 0.5 mM |
Chemical compound, drug | gentamycin | Invitrogen, Karlsruhe, Germany | 15750060 | 20 µg/ml |
Chemical compound, drug | potassiumD-gluconate | Sigma-Aldrich, Munich, Germany | G4500 | 125 mM |
Chemical compound, drug | EGTA | Carl Roth, Karlsruhe, Germany | 3054 | 5 mM |
Chemical compound, drug | KCl | Sigma-Aldrich, Munich, Germany | P4504 | 20 mM |
Chemical compound, drug | Na2-ATP | Carl Roth, Karlsruhe, Germany | K054 | 2 mM |
Chemical compound, drug | Hepes | Carl Roth, Karlsruhe, Germany | 9105 | 10 mM |
Chemical compound, drug | CaCl2 | Sigma-Aldrich, Munich, Germany | C3881 | 0.5 mM |
Chemical compound, drug | KOH | Sigma-Aldrich, Munich, Germany | P4504 | |
Chemical compound, drug | MgCl2 | Sigma-Aldrich, Munich, Germany | MO250 | 2 mM |
Software | MC Rack software | Multi Channel Systems, Germany | versions 3.3–4.5 RRID:SCR_014955 | |
Software | Spike2 software | Cambridge Electronics Design Ltd., Cambridge, UK. | RRID:SCR_000903 | |
Software | Zen | Carl Zeiss, Jena, Germany | RRID:SCR_013672 | |
Software | MEA-Tools | Egert et al., 2002 (PMID 12084562) | version 2.8 | |
Software | FIND toolbox | Meier et al., 2008 (PMID 18692360) | ||
Software | ImageJ | Schneider et al., 2012 (PMID 22930834) | RRID:SCR_003070 | |
Software | Matlab | Mathworks, Natick, MA, USA | versions 2014a – 2017a |
Additional files
-
Transparent reporting form
- https://doi.org/10.7554/eLife.47996.025