A synthetic dataset primer for the biobehavioural sciences to promote reproducibility and hypothesis generation
Figures
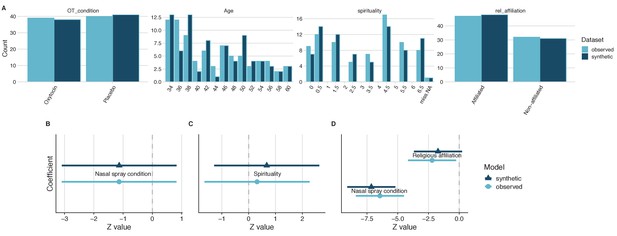
General and specific utility of synthetic data from a study on the impact of intranasal oxytocin on self-reported spirituality.
A comparison of the four variables of interest revealed similar distributions in both the observed and the synthetic datasets, which is indicative of good general utility (A). Direct comparisons of coefficient estimates and 95% confidence intervals from linear models calculated from synthetic and observed datasets revealed no significant differences and high confidence interval overlap (B–D), which is indicative of good specific utility.
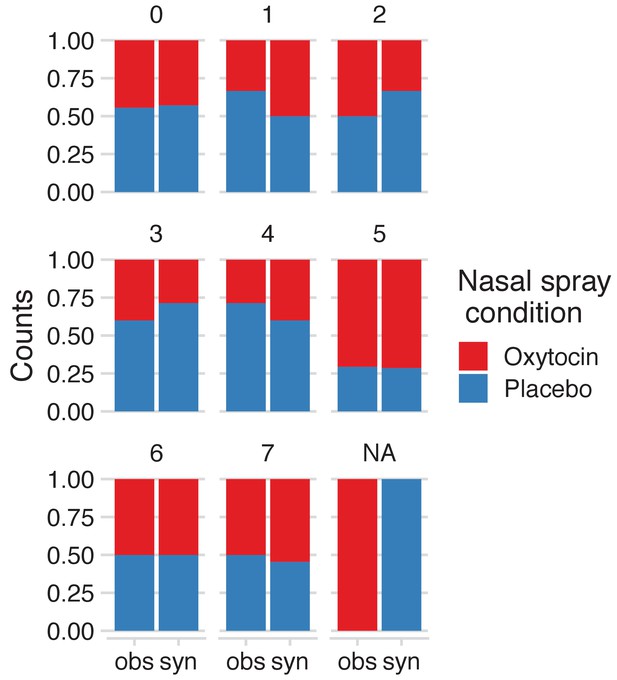
Differences in self-reported spirituality, stratified by nasal spray condition and dataset.
After receiving either the oxytocin or placebo nasal spray (depending on randomization), participants were asked on a scale from 0 (Not at all) to 7 (Completely), “Right now, would you say that spirituality is important for you?”. The difference in counts between the observed dataset (obs) and the synthetic dataset (syn) are shown for each possible response on the scale (0–7). As the counts were similar between datasets for each possible response, this suggests that the synthetic dataset has good utility. There were no missing datapoints (NA).
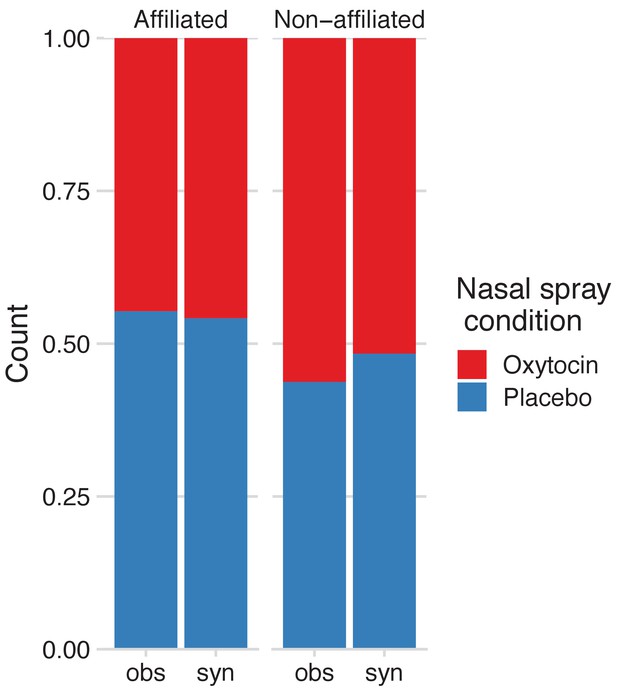
Differences in religious affiliation, stratified by nasal spray condition and dataset.
The difference in counts between the observed dataset (obs) and the synthetic dataset (syn) are shown for two religious affiliation categories: affiliated with a religion and non-affiliated with any religion. As the counts were similar between datasets for both categories, this suggests that the synthetic dataset has good utility.
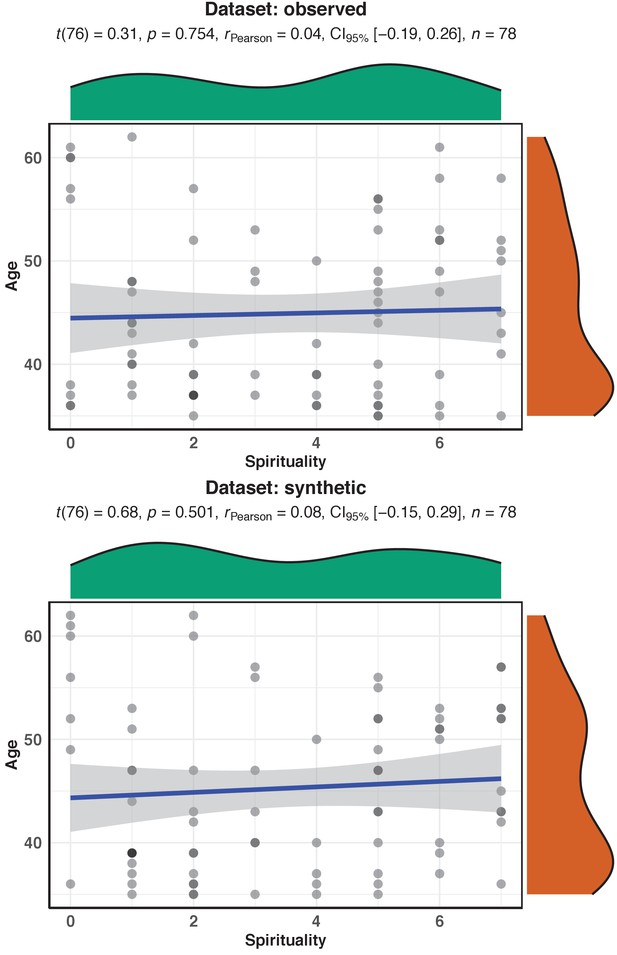
The relationship between age and self-reported spirituality in the observed and synthetic datasets.
As the scatterplot and density plots appear similar between the observed and synthetic datasets, this suggests that the synthetic dataset has good utility.
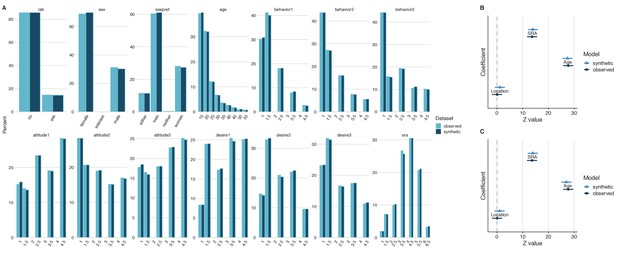
General and specific utility of synthetic data from an investigation on sociosexual orientation.
A comparison of the fourteen variables of interest revealed similar distributions in both the observed and the synthetic datasets, which is indicative of good general utility (A). Direct comparisons of coefficient estimates and 95% confidence intervals from a linear model calculated from synthetic and observed datasets revealed no significant differences and high confidence interval overlap (B), which is indicative of good specific utility. The coefficient estimates and 95% confidence intervals of the same model derived from the synthetic dataset with 213 replicated individuals removed also demonstrated high confidence interval overlap (C). This demonstrates that reducing disclosure risk has little effect on specific utility.
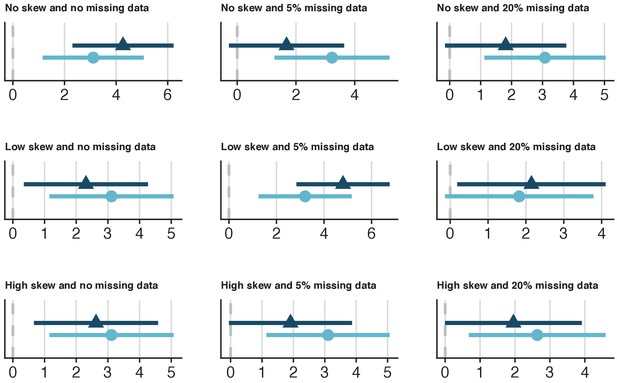
Specific utility of synthetic data from a range of simulated datasets with 100 cases that model the relationship between Heart Rate Variability (HRV) and fitness.
Nine datasets with 100 cases were simulated, which varied on skewness for the HRV variable (none, low, high) and missingness for all variables (0%, 5%, 20%). The x-axes values represent Z-values for the HRV coefficient. The dark-blue triangles and confidence intervals represent the HRV estimates for the synthetic data and the light-blue circles and confidence intervals represent the HRV estimates for the observed data. In general, there was a high overlap between the synthetic and original estimates (Supplementary file 1). The confidence interval range overlap between the synthetic and observed estimates from the dataset with normally distributed HRV and 5% missing data were 60.5%. While the standardized difference was not statistically significant (p=0.12), caution would be warranted in terms of specific utility in this case, given the relatively low confidence interval range overlap.
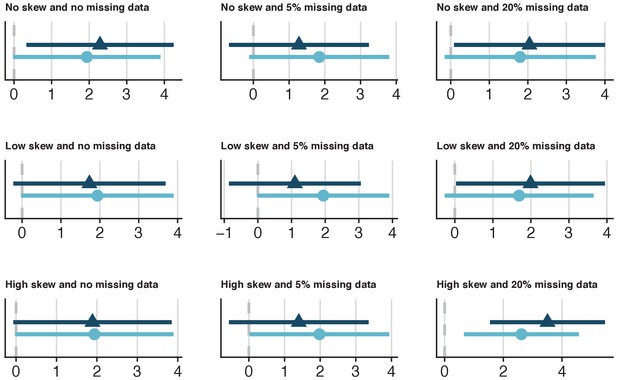
Specific utility of synthetic data from a range of simulated datasets with 40 cases that model the relationship between Heart Rate Variability (HRV) and fitness.
Nine datasets with 40 cases were simulated, which varied on skewness for the HRV variable (none, low, high) and missingness for all variables (0%, 5%, 20%). The x-axes represent Z-values for the HRV coefficient. The dark-blue triangles and confidence intervals represent the HRV estimates for the synthetic data and the light-blue circles and confidence intervals represent the HRV estimates for the observed data. There was high overlap between the synthetic and original estimates for the samples and the standardized differences between models derived from the synthetic and observed datasets were not statistically significant (Supplementary file 1). Thus, these synthetic datasets demonstrate good specific utility.
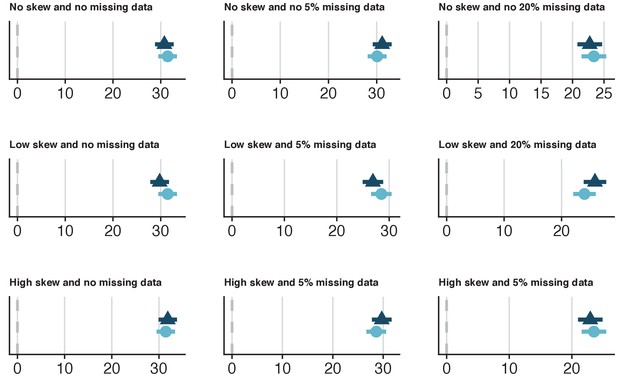
Specific utility of synthetic data from a range of simulated datasets with 10,000 cases that model the relationship between Heart Rate Variability (HRV) and fitness.
Nine datasets with 10,000 cases were simulated, which varied on skewness for the HRV variable (none, low, high) and missingness for all variables (0%, 5%, 20%). The x-axes represent Z-values for the HRV coefficient. The dark-blue triangles and confidence intervals represent the HRV estimates for the synthetic data and the light-blue circles and confidence intervals represent the HRV estimates for the observed data. There was high overlap between the synthetic and original estimates for the samples in which HRV was normally distributed (top row) and highly skewed (bottom row). For the datasets in which HRV had a low skew, the standardized differences between models derived from the synthetic and observed datasets were associated with p-values that can be considered on the border of statistical significance (Supplementary file 1) and the confidence interval range overlap ranged from 53.5% to 28.9% (Supplementary file 1). Altogether, evidence for specific utility in these samples in which HRV had a low skew would not be considered to be strong.
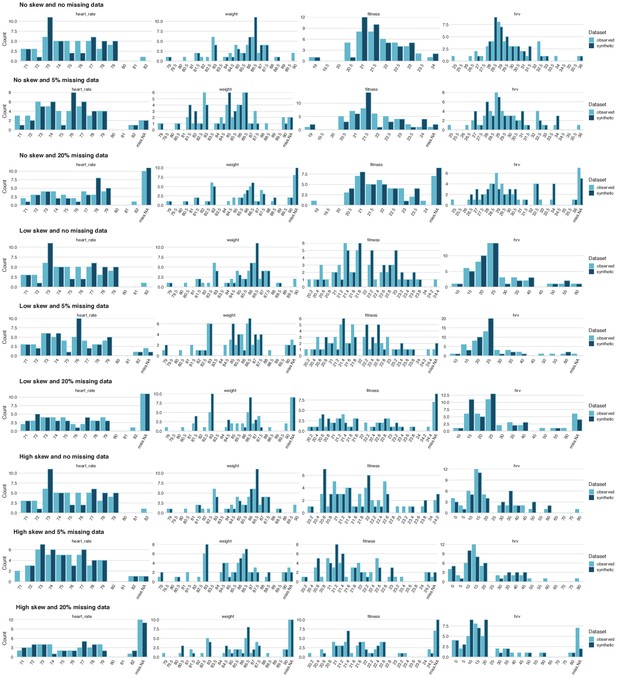
General utility of nine simulated datasets with 40 cases.
Datasets varied on the shape of the distribution of heart rate variability (HRV) and the percentage of missing data.
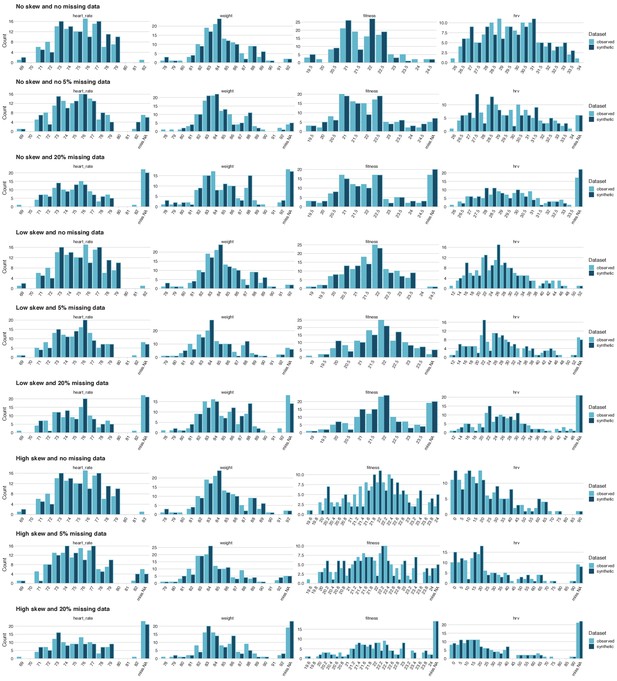
General utility of nine simulated datasets with 100 cases.
Datasets varied on the shape of the distribution of heart rate variability (HRV) and the percentage of missing data.
Additional files
-
Supplementary file 1
Specific utility of simulated datasets.
- https://cdn.elifesciences.org/articles/53275/elife-53275-supp1-v2.xlsx
-
Transparent reporting form
- https://cdn.elifesciences.org/articles/53275/elife-53275-transrepform-v2.docx