Prediction signals in the cerebellum: Beyond supervised motor learning
Figures

Circuit diagram of cerebellar input and output pathways.
Climbing fibers originate in the inferior olive (IO), and excite (plus signs) Purkinje cell dendrites. These inputs serve to instruct heterosynaptic plasticity at synapses from the mossy fiber pathway, an excitatory pathway that originates in the pontine nuclei and elsewhere. The mossy fiber pathway excites granule cells, and terminates in excitatory parallel fiber inputs onto Purkinje cells. Purkinje cells are inhibitory (minus sign), and regulate the activity of output neurons in the cerebellar nuclei.
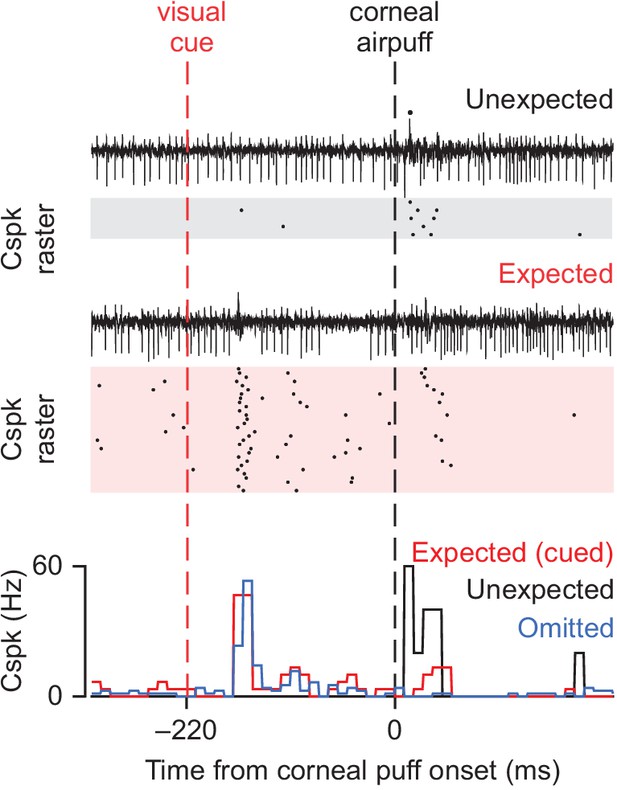
CF input to Purkinje cells obeys the principles of TD learning.
CF-driven Cspks, recorded here after learning, are more probable in response to a US (corneal airpuff) that is unexpected (black) than when the same stimulus is expected (red). Cspks also follow the conditioned stimulus after learning, and are reduced below baseline levels when the expected US does not occur.
© 2015, Springer Nature. Figure 2 was modified from Ohmae and Medina (2015) with permission from JF Medina. It is not covered by the CC-BY 4.0 licence and further reproduction of this panel would need permission from the copyright holder.
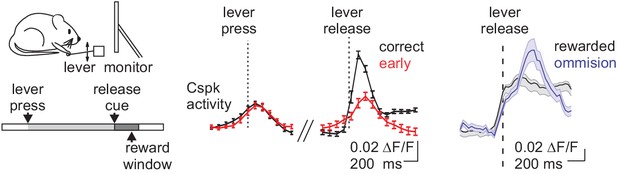
Cspk activity reflects predictions about reward delivery.
Left, mice were trained to press and release a lever as instructed by a visual cue in order to receive reward. Middle, Cspk activity was greatest for lever releases that predicted reward delivery. Right, Cspk activity was enhanced when expected reward was not delivered.
© 2018, Springer Nature. Figure 3 was modified from Heffley et al. (2018). It is not covered by the CC-BY 4.0 licence and further reproduction of this panel would need permission from the copyright holder.
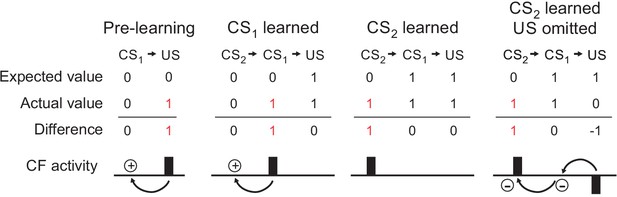
Schematic of CF activity in a TD learning framework.
Before learning, CFs are driven by an unexpected US. After learning, CFs are driven by a CS that accurately predicts the US (CS1). If a new CS occurs earlier in time (CS2), CF activity can then be driven by this higher order stimulus. Finally, if the expected US is omitted, CF activity is reduced (negative prediction error), serving to extinguish associations with the no longer appropriate CS1 and CS2.
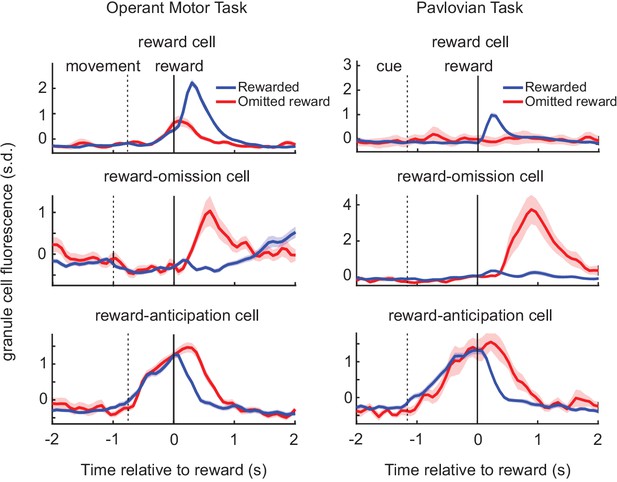
Granule cells develop reward-predictive activity in both operant and Pavlovian conditioning tasks.
Left, population level calcium imaging in mice reveals distinct populations of granule cells that represent reward delivery (top), reward omission (middle), and the anticipation of reward delivery (bottom) after learning in an operant forelimb task. Right, the same categories of responses arise following learning in a Pavlovian task where a neutral cue (CS) is associated with reward.
© 2017, Springer Nature. Figure 5 was modified from Wagner et al. (2017) with permission from M Wagner and L Luo. It is not covered by the CC-BY 4.0 licence and further reproduction of this panel would need permission from the copyright holder.
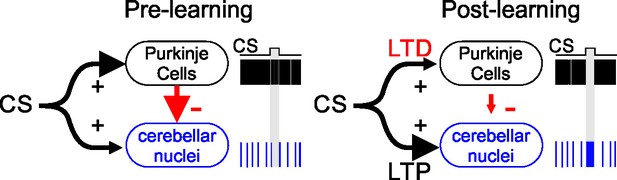
LTD is thought to provide a key mechanism for reducing Purkinje cell output to enable learning.
Parallel fibers (Figure 1) in the mossy fiber pathway carrying CS input are thought to be depressed when paired with CF input to Purkinje cells. This enables a well-timed reduction in Purkinje cell inhibition of CbN cells. LTP of mossy fiber input to CbN cells may also facilitate learning (Pugh and Raman, 2006).
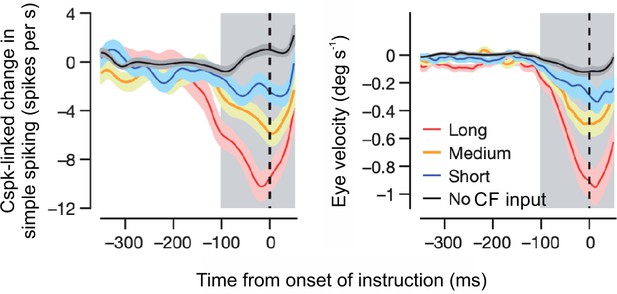
Cspk activity is correlated with both the depression of Purkinje cell simple spiking and learning on a trial-by-trial basis.
Left, Cspks on the preceding trial lead to a depression of Purkinje cell simple spiking on the next trial that is proportional to the duration of the Cspk. Right, trial over trial changes in eye velocity obey the same relationship to Cspk duration as the depression in simple spiking.
© 2014, Springer Nature. Figure 7 was modified from Yang and Lisberger (2014) with permission from SG Lisberger. It is not covered by the CC-BY 4.0 licence and further reproduction of this panel would need permission from the copyright holder.
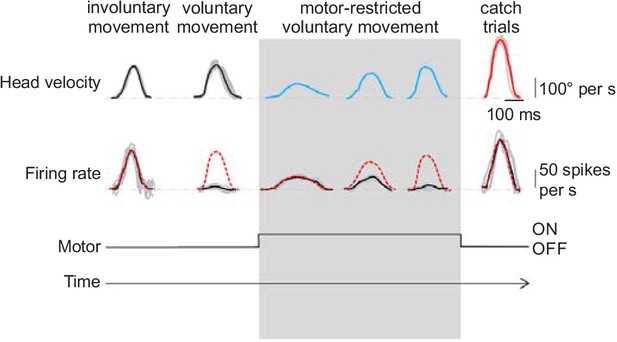
CbN neuron spiking is proportional to mismatch between actual and predicted head velocity.
Top, head velocity traces for a monkey making voluntary and involuntary (imposed via rotational turntable) head movements. When force is applied from an external motor (gray interval), the monkey must slowly adapt its head movement to account for the oppositional force and recover normal head velocity. Catch trials are interleaved where no motor-restriction is applied. Middle, a representative CbN neuron fires only when head movement differs from expectation, and its firing rate is directly proportional to the difference between actual and expected head movement across time.
© 2015, Springer Nature. Figure 8 was modified from Brooks et al. (2015) with permission from K Cullen. It is not covered by the CC-BY 4.0 licence and further reproduction of this panel would need permission from the copyright holder.
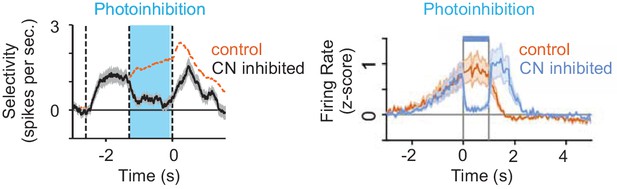
Inhibiting cerebellar CbN output abolishes ramping activity in ALM.
Left, inhibition of the CbN (fastigial) prevents ALM ramping in a whisker-based sensory discrimination task. Right, inhibition of the CbN (dentate) prevents ALM ramping in a virtual reality conditioning task. Modified from Gao et al. (2018) (Left) and Chabrol et al. (2019) (Right) with permission from N Li, K Svoboda, CI DeZeeuw (left) and T Mrsic-Flogel (right).
© 2018, Springer Nature. Left panel modified from Gao et al. (2018) with permission from N Li, K Svoboda, CI DeZeeuw. It is not covered by the CC-BY 4.0 licence and further reproduction of this panel would need permission from the copyright holder.

CbN projections to the VTA signal during a social behavioral context.
Top, behavioral chamber where a test mouse can explore either a novel object (green area) or novel animal (yellow area). Fiber photometry was used to measure the activity of VTA projecting CbN neurons. Bottom, VTA projecting CbN neurons are preferentially active when the test mouse explores the novel animal.
© 2019, AAAS. Modified from Carta et al. (2019) with permission from K Khodakhah. It is not covered by the CC-BY 4.0 licence and further reproduction of this panel would need permission from the copyright holder.
Tables
Open questions.
Can learned climbing fiber activity drive higher order conditioning to establish motor (or other) sequences? |
Does climbing fiber activity in reward-based learning paradigms follow the same rules that have been shown for VTA dopamine neurons? |
How are reward-related climbing fiber signals generated? Can they be computed locally in the IO, or inherited from upstream brain regions? If inherited, from where? |
How are reward-related signals in the granule cells and climbing fibers integrated to mediate learning? How might cerebellar reward-based learning be used by downstream brain regions? |
Does the granule cell layer generate sparse representations of sensorimotor input, and how do local synaptic computations establish such representations? |
Can climbing fibers generate a graded representation of the magnitude of behavioral errors? |
How does cerebellar learning interact with neocortical circuits, and how to do these circuits bi-directionally modulate one another to guide behavior? |
How does cerebellar learning modify output to the mesolimbic dopamine system during goal directed behaviors? |
How does cerebellar circuit dysfunction modulate neocortical developmental and adult neocortical circuit processing in cognitive disease states such as Autism Spectrum Disorders? |
Can cerebellar learning harness different mechanisms and region-specific computations to achieve different goals? Does cerebellar output depend on behavioral or cognitive requirements? |