The effect of climate change on yellow fever disease burden in Africa
Figures
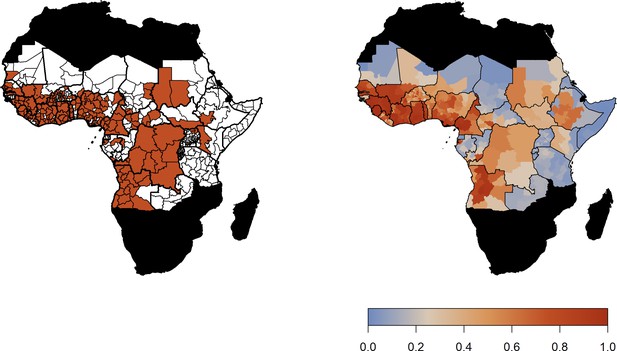
Observed YF occurrence (left) and median probability of a YF report predicted by the GLM.
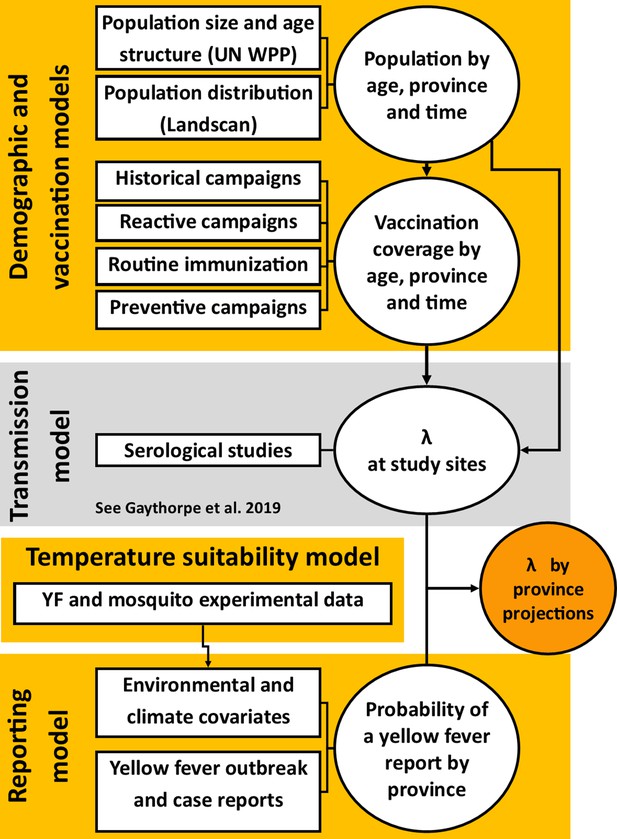
Schematic of data sources and models adapted from Gaythorpe et al., 2019.
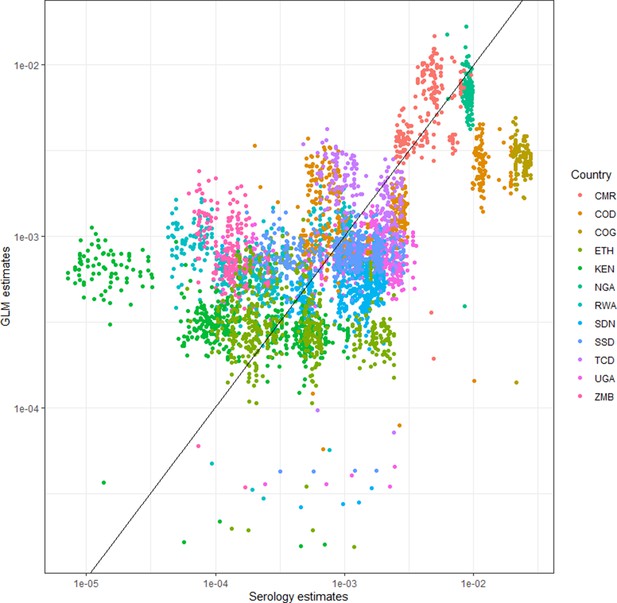
Comparison of force of infection estimates for each admin level 1 unit where we have serological surveys between the estimate from serological surveys only and the GLM within the Bayesian hiearchical model.
In each survey, 100 samples for force of infection are compared.
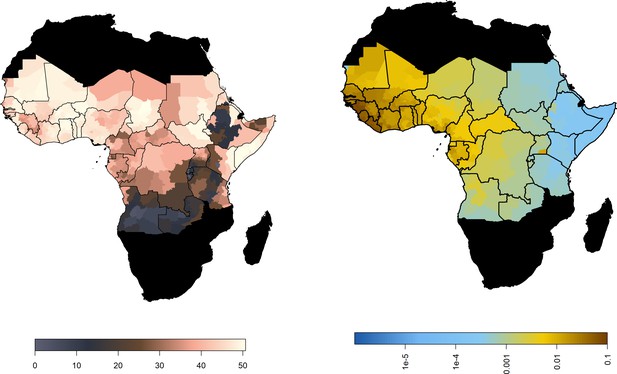
Median predicted model outputs for baseline scenario.
(Left) Median posterior predicted temperature suitability for the African endemic region with average temperature. (Right) Median predicted FOI for the African endemic region at baseline.
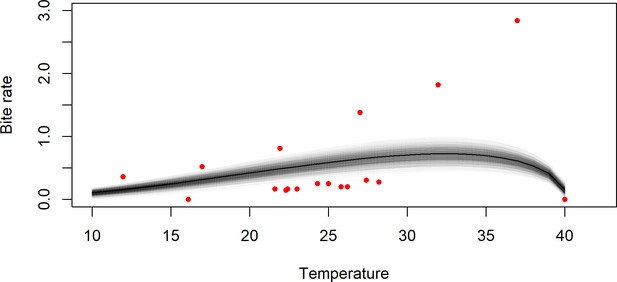
Bite rate per day of Aedes aegypti mosquitos in response to temperature change.
Data is shown with red dots and posterior model samples are shown in grey with the black line indicating median predicted bite rate given temperature in °C. The bite rate of Aedes aegypti mosquitoes is informed by two data sets, that of Mordecai et al. and Martens et al.
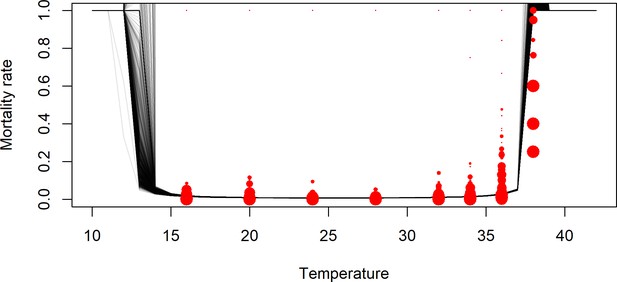
Mortality rate per day of Aedes aegypti mosquitos in response to temperature change.
Data is shown with red dots and posterior model samples are shown in grey with the black line indicating median predicted bite rate given temperature in °C. The data was collected experimentally in Tesla et al.
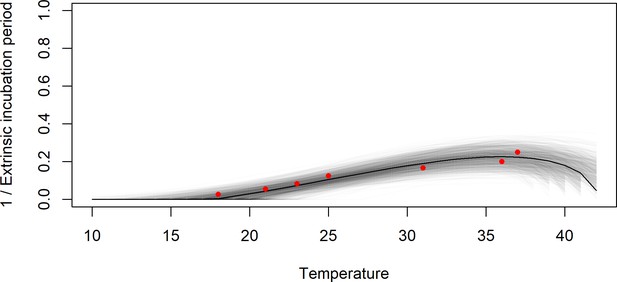
Inverse extrinsic incubation period in response to temperature change.
Data is shown with red dots and posterior model samples are shown in grey with the black line indicating median predicted bite rate given temperature in °C. This is estimated using data from Davis which was calculated specifically for yellow fever in Aedes aegypti. The prediction of the median incubation period at 25°C is in line with that of Johansson et al., 2010 who found it to be 10 days [2.0 - 37] days.
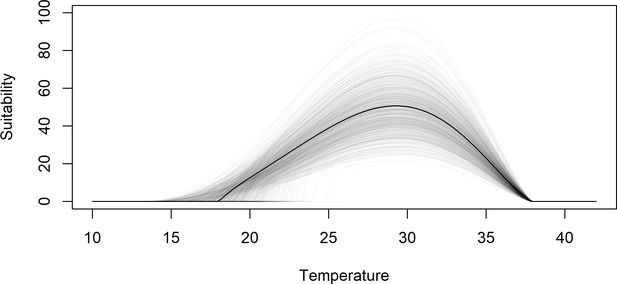
Temperature suitability in response to temperature change.
Data is shown with red dots and posterior model samples are shown in grey with the black line indicating median predicted bite rate given temperature in °C. The model is also informed by the YF occurrence data within the Bayesian hierarchical framework.
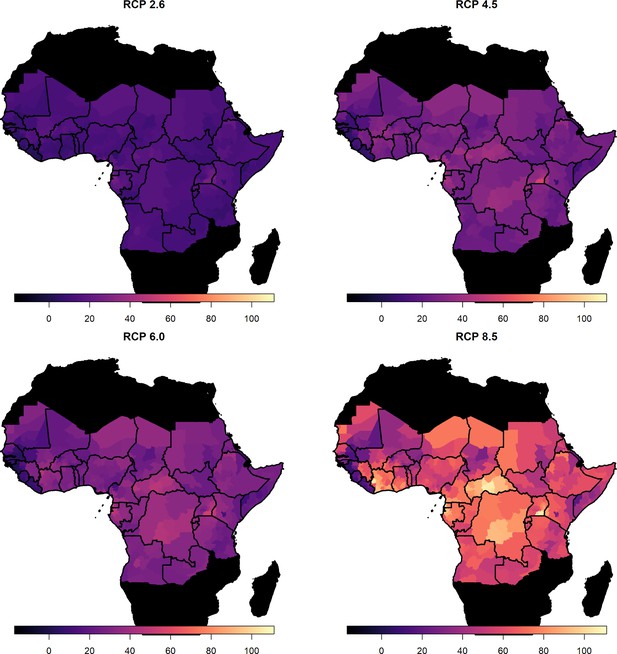
Percentage change in force of infection in 2070.
Median predicted change in force of infection in the African endemic region in 2070 for the four emission scenarios.
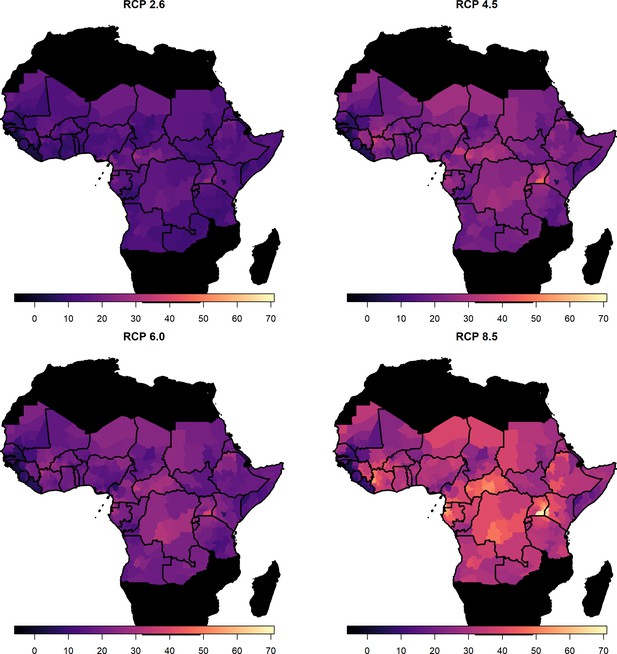
Percentage change in force of infection in 2050.
Median predicted change in FOI in African endemic region in 2050 for the four emission scenarios.
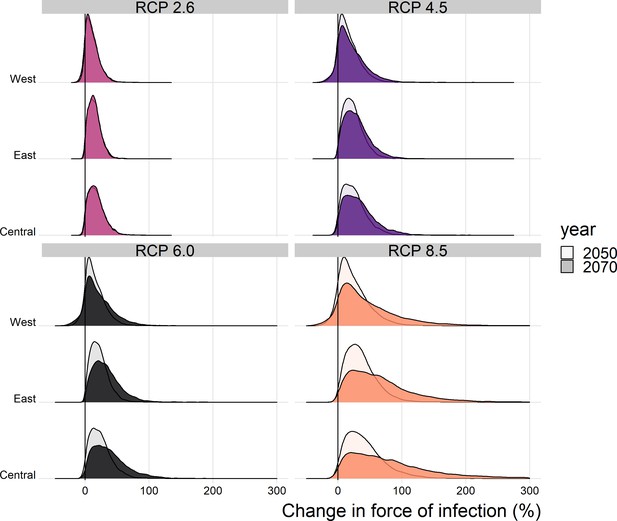
Posterior distribution of the change in the spatial mean force of infection (%) for each region of Africa, year and climate scenario.
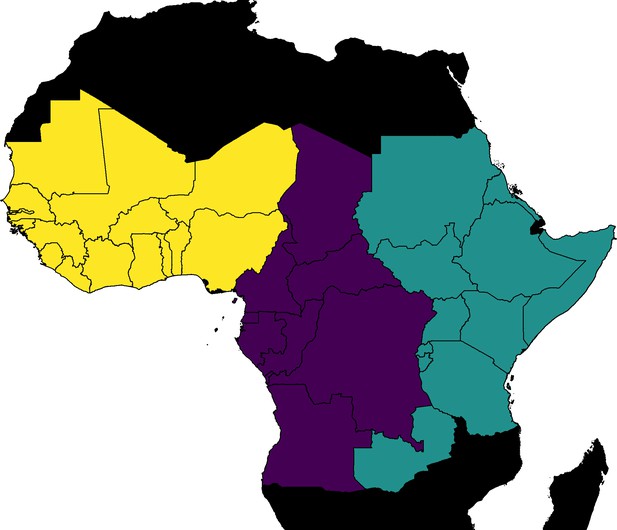
Country groupings in regions used in the manuscript with West, yellow; Central, purple and East, green.
Sudan is a member of the North African region; however, due to the grouping of endemic countries, we include Sudan in the East African region.
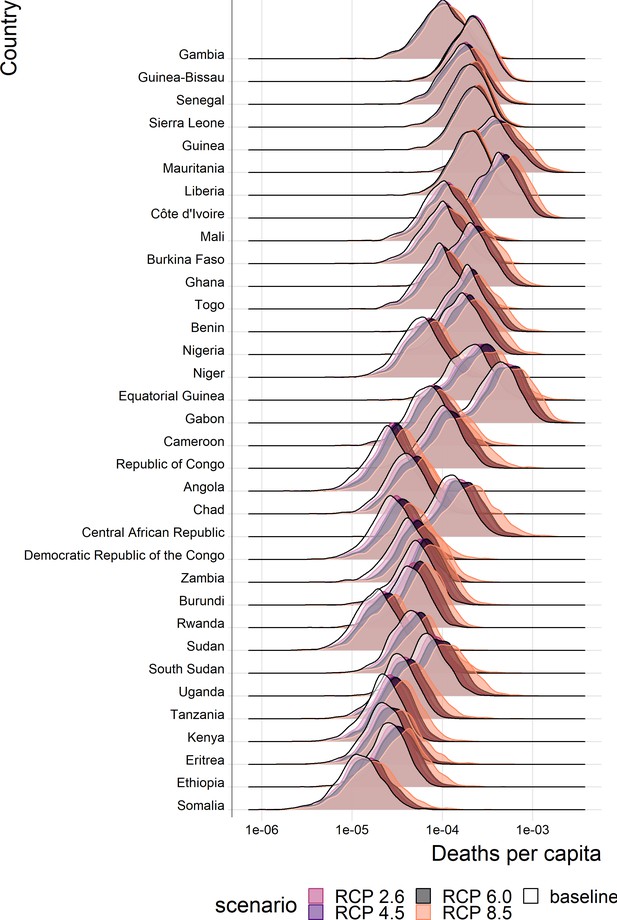
Posterior predicted annual YF deaths per capita for each country in the African endemic region in 2070.
Countries are ordered by longitude.
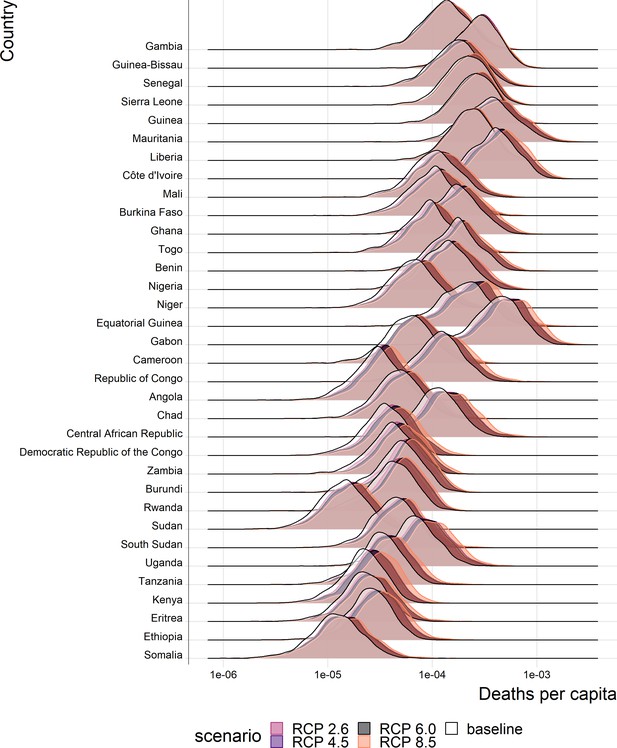
Posterior predicted deaths per capita for each country in the African endemic region in 2050.
Countries are ordered by longitude.
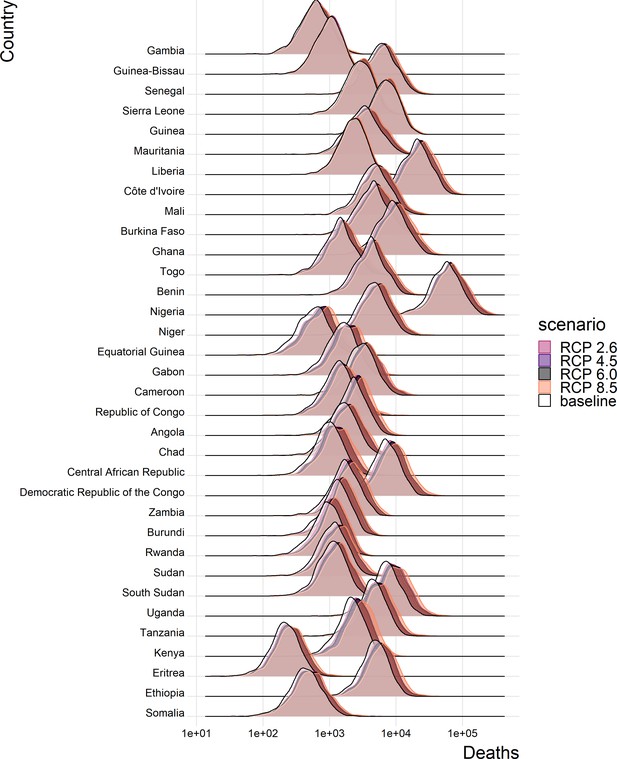
Posterior predicted deaths for each country in the African endemic region in 2050.
Countries are ordered by longitude.
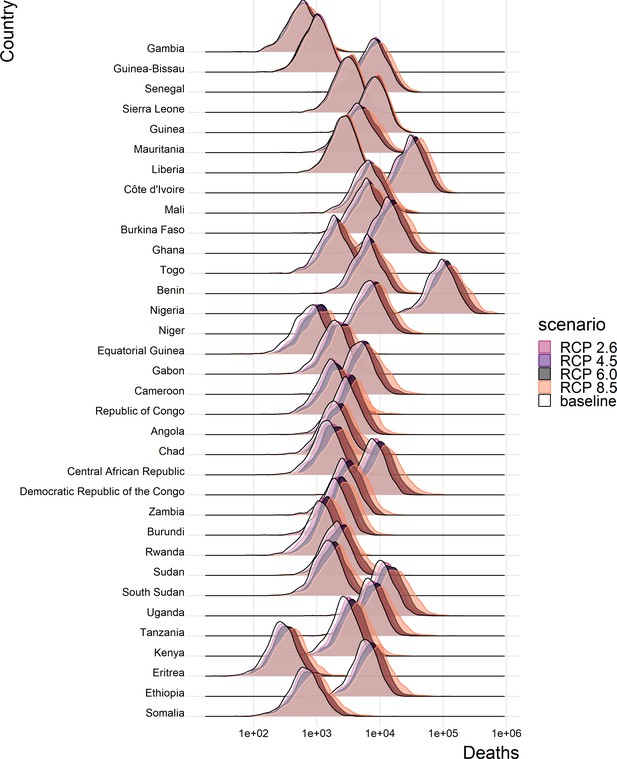
Posterior predicted deaths for each country in the African endemic region in 2070.
Countries are ordered by longitude.
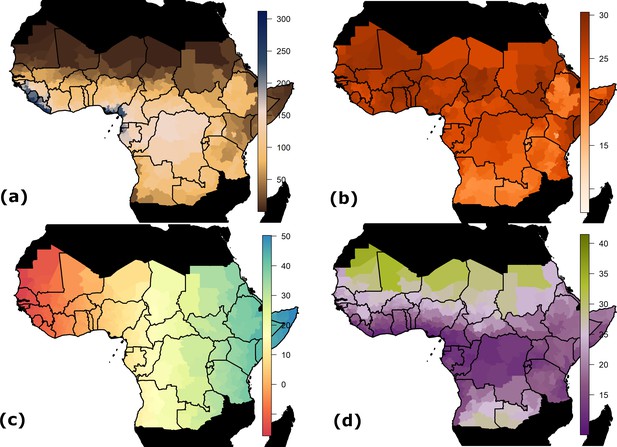
Spatial data inputs for generalised linear model.
Countries shown in black are not considered endemic for YF. (a) Estimated mean monthly rainfall (mm) for baseline/current scenario. (b) Average temperature at baseline/current scenario in °C. (c) Longitude. (d) Range in temperature at baseline/current scenario in °C.
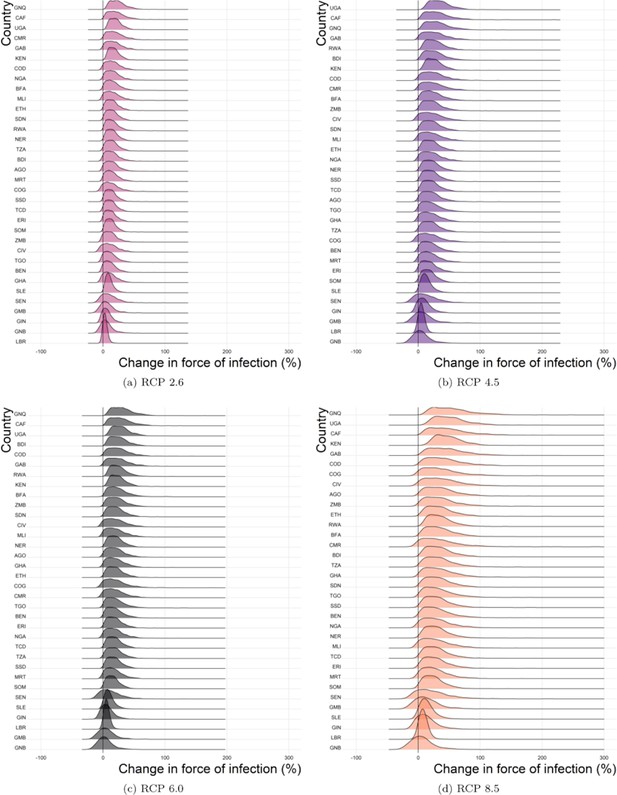
Predicted force of infection change (%) for each country and scenario in 2050.
Note, countries are ordered by difference and may vary in position. (a) RCP 2.6, (b) RCP 4.5, (c) RCP 6.0, (d) RCP 8.5.
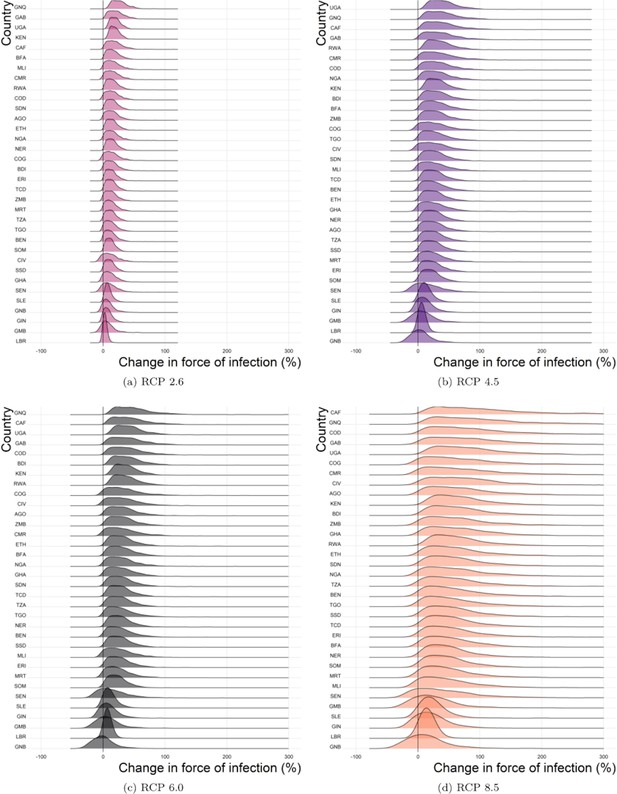
Predicted force of infection change (%) for each country and scenario in 2070.
Note, countries are ordered by difference and may vary in position. (a) RCP 2.6, (b) RCP 4.5, (c) RCP 6.0, (d) RCP 8.5.
Videos
Percentage change in deaths from 2020 to 2070 in three regions in the African Endemic region under 4 climate change scenarios.
100 samples of the posterior predicted trajectories are shown.
Tables
Predicted percentage change in deaths in the African endemic region in 2050 and 2070 compared to the baseline/current scenario.
Year | Scenario | 95% CrI low | 50% CrI low | Median | 50% CrI high | 95% CrI high |
---|---|---|---|---|---|---|
2050 | RCP 2.6 | −2.36 | 4.49 | 10.84 | 18.58 | 37.91 |
2050 | RCP 4.5 | −2.40 | 7.32 | 16.71 | 28.16 | 57.43 |
2050 | RCP 6.0 | −2.78 | 6.79 | 15.49 | 25.86 | 51.85 |
2050 | RCP 8.5 | −2.17 | 11.03 | 24.92 | 41.84 | 88.33 |
2070 | RCP 2.6 | −0.74 | 4.11 | 9.99 | 17.03 | 34.10 |
2070 | RCP 4.5 | −2.76 | 7.77 | 19.28 | 33.56 | 71.08 |
2070 | RCP 6.0 | −4.56 | 8.63 | 21.35 | 36.70 | 77.70 |
2070 | RCP 8.5 | −2.90 | 16.08 | 39.57 | 72.43 | 178.63 |
Generalised linear model covariates.
Covariate | Interpretation |
---|---|
log(survey quality) | Log of the survey quality for countries in YFSD. |
adm05 | Country factors for countries not in YFSD. |
longitude | Longitude of province centroid |
temperature suitability | Temperature suitability at average suitability of province. |
temperature range | Temperature range in province. |
rainfall | Mean Precipitation in province. |
log(pop) | Log of the human population size of the province |
Projected change in global mean surface air temperature and CO2 concentrations by 2100 relative to the reference period of 1986–2005 (Stocker, 2013).
Scenario | Temperature rise (°C) [range] | CO2 concentrations (ppm) |
---|---|---|
RCP 2.6 | 1 [0.3 to 1.7] | 421 |
RCP 4.5 | 1.8 [1.1 to 2.6] | 538 |
RCP 6.0 | 2.2 [1.4 to 3.1] | 670 |
RCP 8.5 | 3.7 [2.6 to 4.8] | 936 |
Characteristics of included serological surveys. Recreated from Gaythorpe et al., 2019.
Location | Sample size | Year | Reference |
---|---|---|---|
Nigeria | 184 | 1990 | Omilabu et al., 1990 |
Democratic Republic of the Congo | 140 | 1985 | Werner and Huber, 1984 |
Republic of the Congo | 360 | 1985 | Merlin et al., 1986 |
Cameroon (North) | 840 | 1987 | Tsai et al., 1987 |
Cameroon (South) | 256 | 2001 | Kuniholm et al., 2006 |
Uganda (zones) | 584 | 2012 | |
Rwanda (zones) | 1286 | 2012 | |
Zambia (zones) | 3679 | 2013 | |
Sudan (zones) | 1814 | 2012 | |
Kenya (zones) | 1960 | 2013 | |
Ethiopia (zones) | 1645 | 2014 | Mengesha Tsegaye et al., 2018 |
Democratic republic of the Congo (zones) | 479 | 2014 | |
South Sudan (zones) | 1480 | 2014 | |
Chad (zones) | 352 | 2014 |
Parameter estimates with low and high ends of the 95% credible interval.
Parameter | 95% CrI low | Median | 95% CrI high | Meaning |
---|---|---|---|---|
a_c | 0.0002 | 0.0003 | 0.0003 | Bite rate |
a_T0 | 0.2205 | 2.9285 | 7.2004 | Bite rate |
a_Tm | 40.0223 | 40.1368 | 40.2981 | Bite rate |
adm05AGO | 1.1382 | 1.7656 | 2.3960 | GLM coefficients |
adm05BDI | −1.1566 | −0.3275 | 0.4671 | GLM coefficients |
adm05ERI | −0.9901 | −0.1074 | 0.7519 | GLM coefficients |
adm05ETH | −1.2878 | −0.5366 | 0.1882 | GLM coefficients |
adm05GNB | −1.4959 | −0.7566 | −0.0692 | GLM coefficients |
adm05KEN | −1.1264 | −0.3510 | 0.3722 | GLM coefficients |
adm05MRT | −1.1837 | −0.4218 | 0.2927 | GLM coefficients |
adm05RWA | −1.1411 | −0.3175 | 0.4826 | GLM coefficients |
adm05SDN | −0.8870 | −0.1106 | 0.6377 | GLM coefficients |
adm05SOM | −1.0177 | −0.1425 | 0.7144 | GLM coefficients |
adm05SSD | −0.9086 | −0.0796 | 0.7140 | GLM coefficients |
adm05TZA | −1.3990 | −0.6442 | 0.0812 | GLM coefficients |
adm05UGA | −0.6618 | −0.0081 | 0.6163 | GLM coefficients |
adm05ZMB | −1.2049 | −0.3840 | 0.3975 | GLM coefficients |
Intercept | −16.4268 | −13.2731 | −10.2753 | GLM coefficients |
log.surv.qual.adm0 | 0.3209 | 0.5048 | 0.6917 | GLM coefficients |
logpop | 0.9133 | 1.1466 | 1.3913 | GLM coefficients |
lon | −1.1806 | −0.9173 | −0.6557 | GLM coefficients |
mu_c | −0.8003 | −0.7578 | −0.7166 | Mortality |
mu_T0 | 12.2133 | 12.7137 | 13.1498 | Mortality |
mu_Tm | 38.0341 | 38.0481 | 38.0532 | Mortality |
iEIP_c | 0.0001 | 0.0001 | 0.0002 | Inverse EIP |
iEIP_T0 | 10.9412 | 17.6724 | 22.2418 | Inverse EIP |
iEIP_Tm | 39.0737 | 42.1075 | 45.5927 | Inverse EIP |
temp_suitability | 0.0101 | 0.1523 | 0.3863 | GLM coefficients |
worldclim_rainfall | 0.2338 | 0.4629 | 0.6969 | GLM coefficients |
worldclim_temp_range | −0.1912 | 0.0368 | 0.2687 | GLM coefficients |
Deaths in the African endemic region in 2050 and 2070 compared to the baseline/constant scenario.
Year | Scenario | Median | 95% Cr interval |
---|---|---|---|
2050 | RCP 2.6 | 191309 | [62462, 468985] |
2050 | RCP 4.5 | 200470 | [66330, 499615] |
2050 | RCP 6.0 | 198096 | [65113, 489494] |
2050 | RCP 8.5 | 214427 | [69699, 554842] |
2050 | baseline | 172668 | [58177, 395300] |
2070 | RCP 2.6 | 273582 | [90275, 653145] |
2070 | RCP 4.5 | 298822 | [99694, 735109] |
2070 | RCP 6.0 | 301001 | [99551, 739950] |
2070 | RCP 8.5 | 349157 | [108913, 933389] |
2070 | baseline | 249556 | [84877, 560186] |
Probability of increase (%) in deaths in the African endemic region in 2050 and 2070 compared to the baseline/constant scenario.
Year | Scenario | Median | 95% Cr interval |
---|---|---|---|
2050 | RCP 2.6 | 92.97 | [92.7, 93.23] |
2050 | RCP 4.5 | 94.85 | [94.61, 95.07] |
2050 | RCP 6.0 | 94.89 | [94.64, 95.13] |
2050 | RCP 8.5 | 95.98 | [95.78, 96.17] |
2070 | RCP 2.6 | 95.47 | [95.24, 95.7] |
2070 | RCP 4.5 | 94.64 | [94.41, 94.88] |
2070 | RCP 6.0 | 94.10 | [93.85, 94.35] |
2070 | RCP 8.5 | 95.94 | [95.72, 96.15] |