What do adversarial images tell us about human vision?
Figures
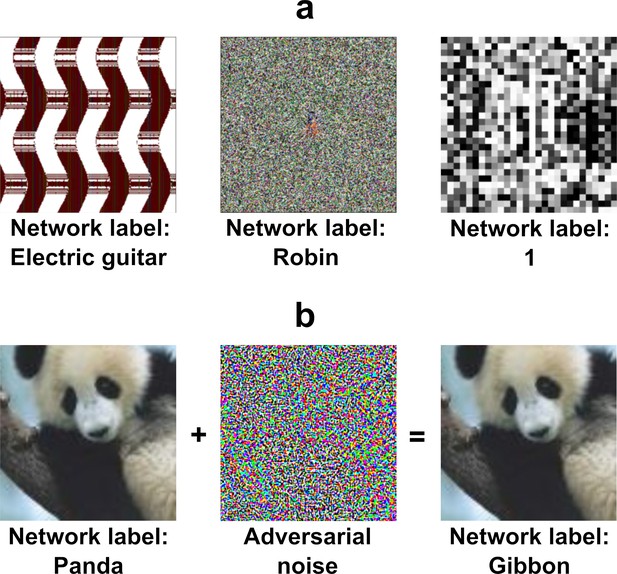
Examples of two types of adversarial images.
(a) fooling adversarial images taken from Nguyen et al., 2015 that do not look like any familiar object. The two images on the left (labelled ‘Electric guitar’ and ‘Robin’) have been generated using evolutionary algorithms using indirect and direct encoding, respectively, and classified confidently by a DCNN trained on ImageNet. The image on the right (labelled ‘1’) is also generated using an evolutionary algorithm using direct encoding and it is classified confidently by a DCNN trained on MNIST. (b) An example of a naturalistic adversarial image taken from Goodfellow et al., 2014 that is generated by perturbing a naturalistic image on the left (classified as ‘Panda’) with a high-frequency noise mask (middle) and confidently (mis)classified by a DCNN (as a ‘Gibbon’).
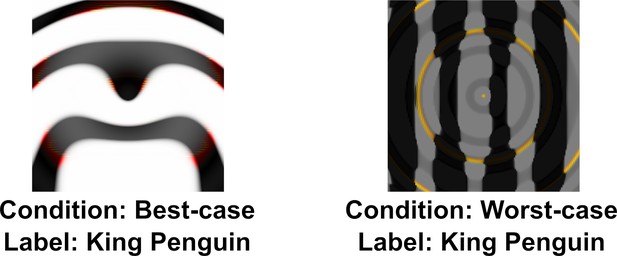
Example of best-case and worst-case images for the same category (‘penguin’) used in Experiment 2.
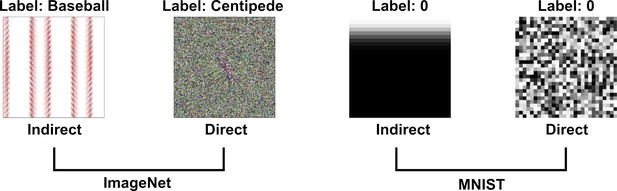
Examples of images from Nguyen et al., 2015 used in the four experimental conditions in Experiment 3.
Images are generated using an evolutionary algorithm either using the direct or indirect encoding and generated to fool a network trained on either ImageNet or MNIST.
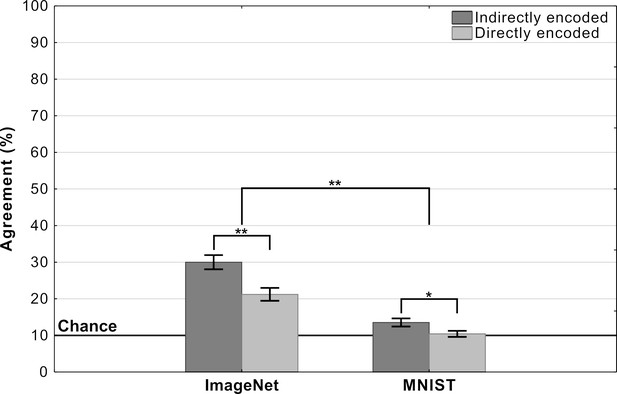
Agreement (mean percentage of images on which a participant choices agree with the DCNN) as a function of experimental condition in Experiment 3 (error bars denote 95% confidence intervals).
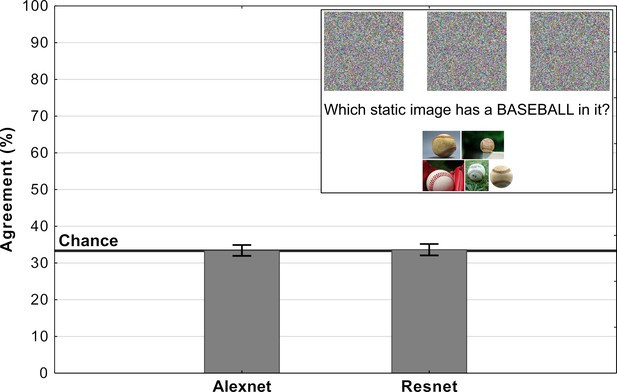
Average levels of agreement in Experiment 4 (error bars denote 95% confidence intervals).
The inset depicts a single trial in which participants were shown three fooling adversarial images and naturalistic examples from the target category. Their task was to choose the adversarial image which contained an object from the target category.
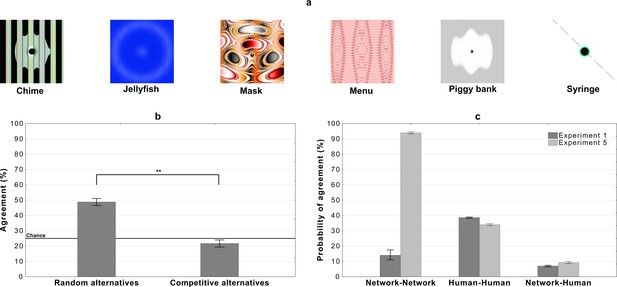
Results for images that are confidently classified with high network-to-network agreement on Alexnet, Densenet-161, GoogLeNet, MNASNet 1.0, MobileNet v2, Resnet 18, Resnet 50, Shufflenet v2, Squeezenet 1.0, and VGG-16.
(a) Examples of images used in the experiment - for all the stimuli see Appendix 2—figures 4 and 5, (b) average levels of agreement between participants and DCNNs under the random and competitive alternatives conditions in Experiment 5, and (c) probability of network, human, and network to human agreement in the competitive alternatives condition of Experiment 1 and Experiment 5 (error bars denote 95% confidence intervals).
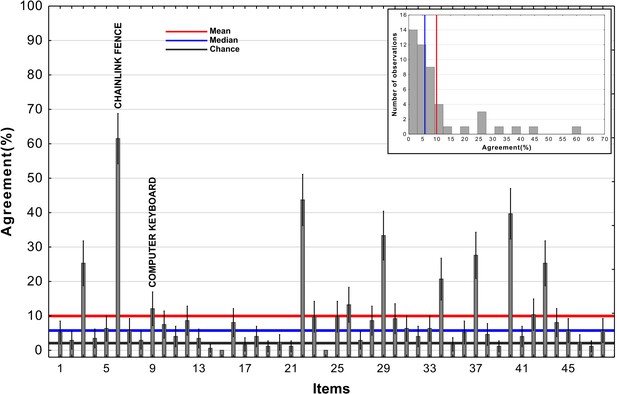
Agreement across adversarial images from Experiment 3b in Zhou and Firestone, 2019.
The red line represents the mean, the blue line represents the median, and the black reference line represents chance agreement. The inset contains a histogram of agreement levels across the 48 images.
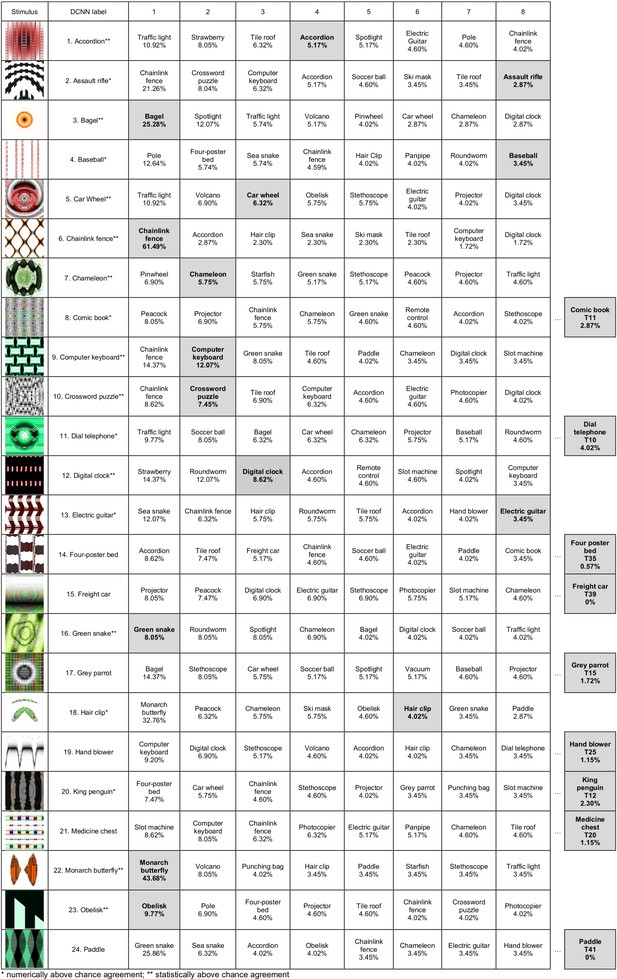
Participant responses ranked by frequency (Experiment 3b).
Each row contains the adversarial image, the DCNN label for that image, the top eight participant responses. Shaded cells contain the DCNN choice, when not ranked in the top 8, it is shown at the end of the row along with the rank in brackets.
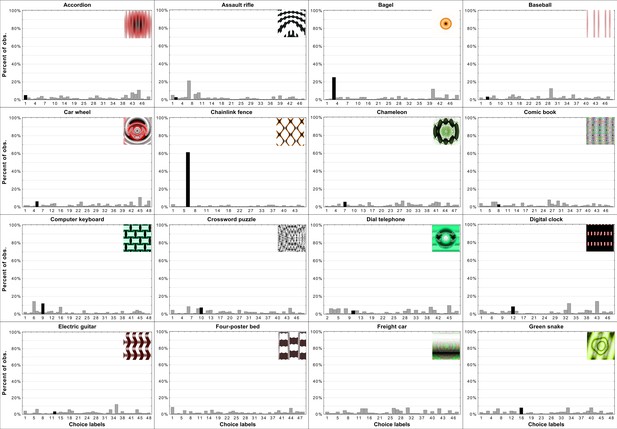
Per-item histograms of response choices from Experiment 3b in Zhou and Firestone, 2019.
Each histogram contains the adversarial stimuli and shows the percentage of responses per each choice (y-axis). The choice labels (x-axis) are ordered the same way as in Appendix 1—figures 2 and 3 from 1 to 48. Black bars indicate the DCNN choice for a particular adversarial image.
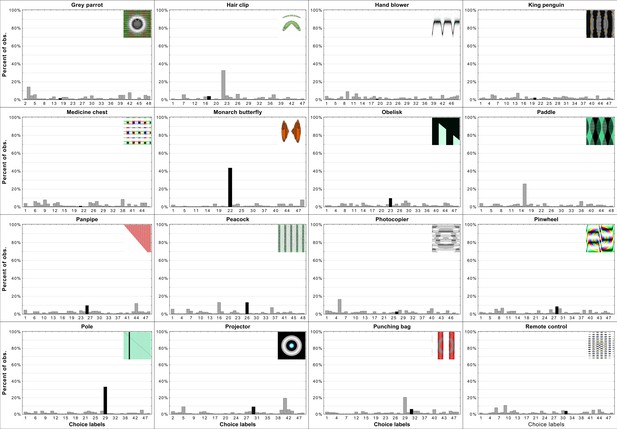
Per-item histograms of response choices from Experiment 3b in Zhou and Firestone, 2019.
Continued.
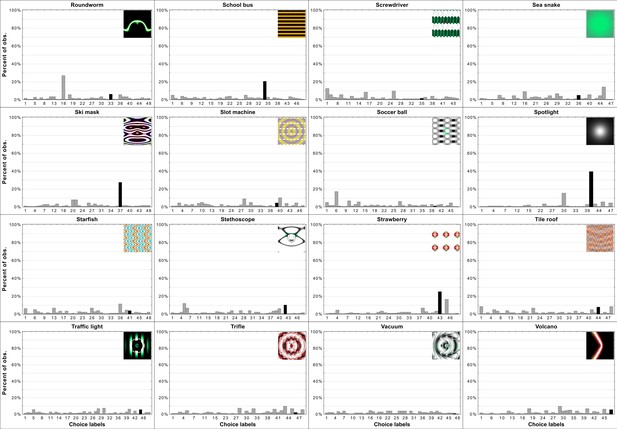
Per-item histograms of response choices from Experiment 3b in Zhou and Firestone, 2019.
Continued.
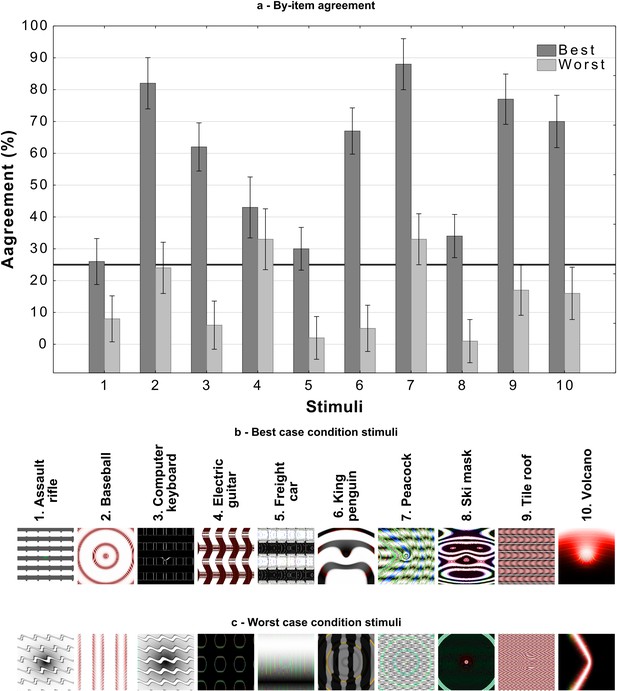
An item-wise breakdown of agreement levels in Experiment 2 as a function of experimental condition and category.
Average agreement levels for each category in each condition with 95% CI are presented in (a) with the black line referring to chance agreement. The best case stimuli are presented in (b), these stimuli were judged as containing the most features in common with the target category (out of 5 generated by Nguyen et al., 2015). The worst case stimuli are presented in (c), these were judged to contain the least number of features in common with the target category.
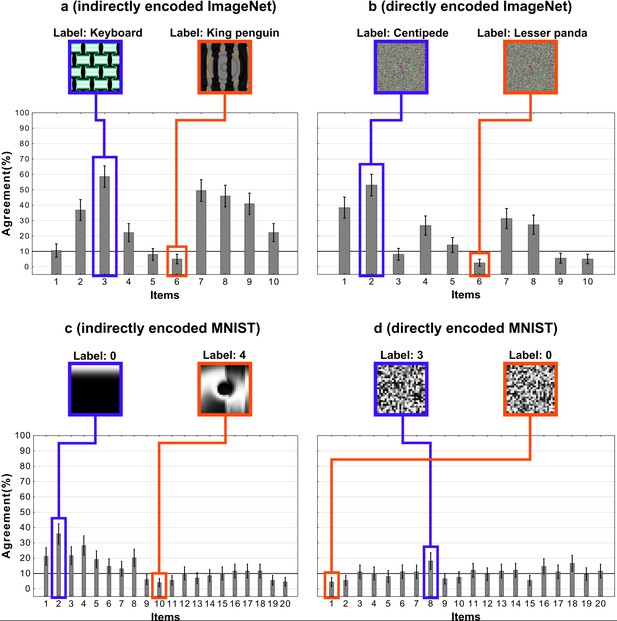
An item-wise breakdown of agreement levels for the four conditions in Experiment 3.
Each bar shows the agreement level for a particular image, that is, the percentage of participants that agreed with DCNN classification for that image. Each sub-figure also shows the images that correspond to the highest (blue) and lowest (red) levels of agreement under that condition.
Tables
Mean DCNN-participant agreement in the experiments conducted by Zhou and Firestone, 2019
Exp. | Test type | Mean agreement | Chance |
---|---|---|---|
1 | Fooling 2AFC N15 | 74.18% (35.61/48 images) | 50% |
2 | Fooling 2AFC N15 | 61.59% (29.56/48 images) | 50% |
3a | Fooling 48AFC N15 | 10.12% (4.86/48 images) | 2.08% |
3b | Fooling 48AFC N15 | 9.96% (4.78/48 images) | 2.08% |
4 | TV-static 8AFC N15 | 28.97% (2.32/8 images) | 12.5% |
5 | Digits 9AFC P16 | 16% (1.44/9 images) | 11.11% |
6 | Naturalistic 2AFC K18 | 73.49% (7.3/10 images) | 50% |
7 | 3D Objects 2AFC A17 | 59.55% (31.56/53 images) | 50% |
-
* To give the readers a sense of the levels of agreement observed in these experiments, we have also computed the average number of images in each experiment where humans and DCNNs agree as well as the level of agreement expected if participants were responding at chance.
† Stimuli sources: N15 - Nguyen et al., 2015; P16 - Papernot et al., 2016; K18 - Karmon et al., 2018; A17 - Athalye et al., 2017.