Functionally specialized human CD4+ T-cell subsets express physicochemically distinct TCRs
Figures
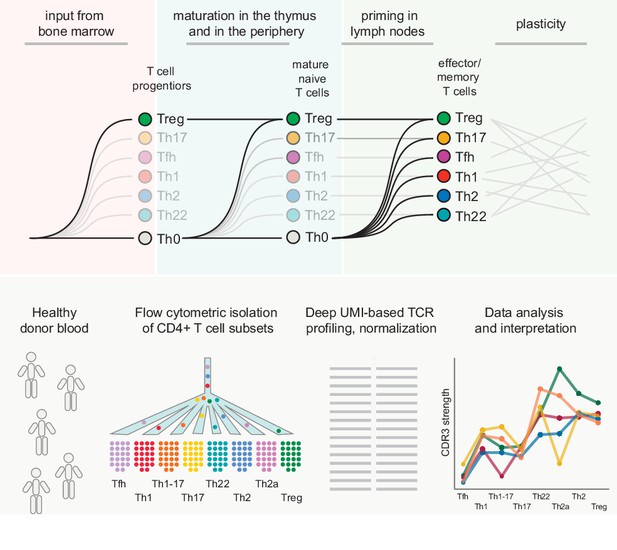
Experimental overview.
Top: schematic representation of the general questions addressed in this study. Bottom: schematic representation of the experimental pipeline. Naive and effector/memory CD4+ T-cell subsets were flow-sorted from peripheral blood samples obtained from healthy donors. Repertoire characteristics were extracted from normalized datasets obtained from each subset via high-throughput sequence analysis of all expressed TCRs.
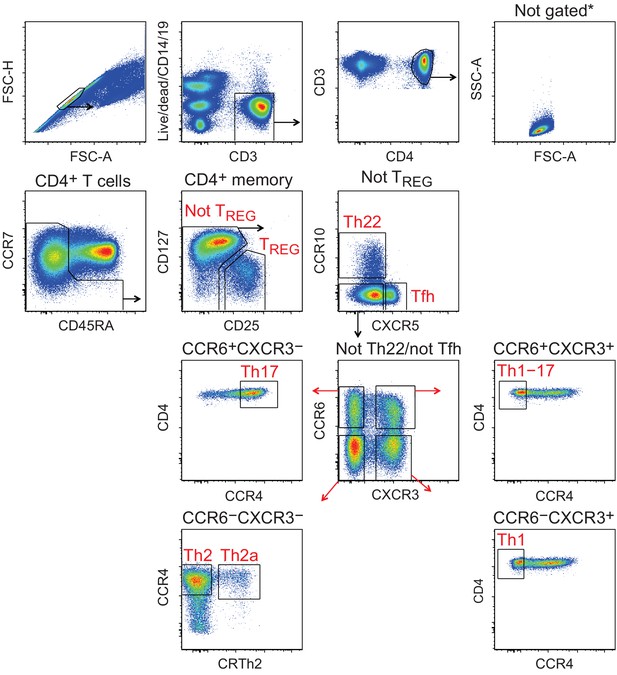
Gating strategy for the identification of effector/memory CD4+ T-cell subsets.
Single lymphocytes were identified in a forward scatter-area (FCS-A) versus forward scatter-height (FSC-H) plot. Viable CD3+CD14−CD19− cells were gated in the CD4+ lineage, and naive cells were excluded as CCR7+CD45RA+ events. Effector/memory subsets were then sorted as Tfh cells (CXCR5+), Th1 cells (non-Tfh/Th22/Treg CCR4−CCR6−CXCR3+), Th1-17 cells (non-Tfh/Th22/Treg CCR4−CCR6+CXCR3+), Th17 cells (non-Tfh/Th22/Treg CCR4+CCR6+CXCR3−), Th22 cells (CCR10+), Th2a cells (non-Tfh/Th22/Treg CCR4+CCR6−CRTh2+CXCR3−), Th2 cells (non-Tfh/Th22/Treg CCR4+CCR6−CRTh2−CXCR3−), or Tregs (CD25highCD127low).
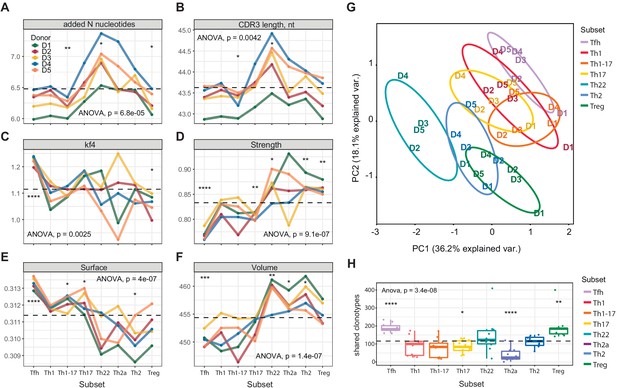
Averaged physicochemical characteristics of CDR3β repertoires from effector/memory CD4+ T-cell subsets.
(A–F) Averaged physicochemical characteristics were measured for the five amino acids in the middle of the CDR3β sequences obtained from each effector/memory CD4+ T-cell subset (n = 8) from each healthy donor (n = 5). Calculations were weighted by clonotype frequency. Unweighted analyses yielded similar results (data not shown). (A) Non-germline nucleotide (N) additions. (B) CDR3β length (nucleotides). (C) Kidera factor 4 (arbitrary scale). (D) Interaction strength (arbitrary scale). (E) Surface (arbitrary scale). (F) Volume (arbitrary scale). (G) Principal component analysis of the cumulative CDR3α and CDR3β repertoires from each subset of effector/memory CD4+ T cells (n = 28 parameters computed in VDJtools). Top contributing factors to PC1: CDR3β volume, mjenergy, core, beta, length, number of added nucleotides, strength, and alpha. Top contributing factors to PC2: CDR3α disorder, CDR3α Kidera factor 3, CDR3β disorder, CDR3α Kidera factor 1, CDR3α strength, CDR3β Kidera factors 2, 3, 4, and 10, and CDR3β charge. (H) Relative publicity measured for each effector/memory CD4+ T-cell subset as the number of identical or near-identical (maximum n = 1 mismatch) amino acid residue-defined CDR3β variants shared between the top 20,000 most frequent clonotypes in the corresponding repertoires from each pair of donors. Dashed lines indicate means. *p<0.05, **p<0.01, ***p<0.001, and ****p<0.0001 (one-way ANOVA followed by the two-sample Welch t-test with Bonferroni correction for each group versus the mean).
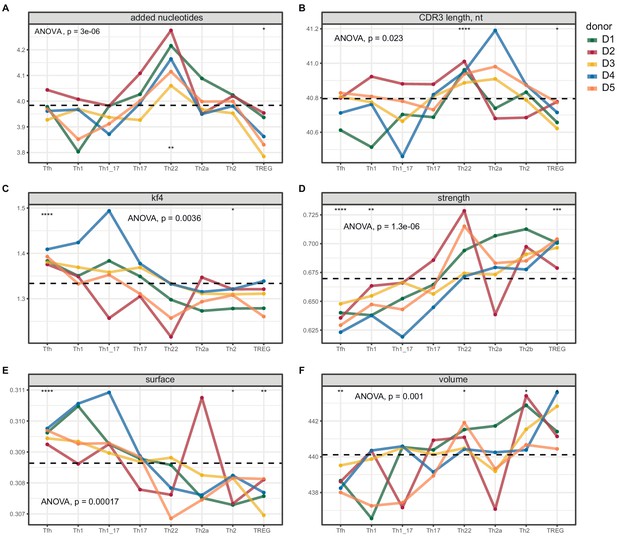
Averaged physicochemical characteristics of CDR3α repertoires from effector/memory CD4+ T-cell subsets.
(A–F) Averaged physicochemical characteristics were measured for the five amino acids in the middle of the CDR3α sequences obtained from each effector/memory CD4+ T-cell subset (n = 8) from each healthy donor (n = 5). Calculations were weighted by clonotype frequency. (A) Non-germline nucleotide (N) additions. (B) CDR3α length (nucleotides). (C) Kidera factor 4 (arbitrary scale). (D) Interaction strength (arbitrary scale). (E) Surface (arbitrary scale). (F) Volume (arbitrary scale). *p<0.05, **p<0.01, ***p<0.001, and ****p<0.0001 (one-way ANOVA followed by the two-sample Welch t-test with Bonferroni correction for each group versus the mean).
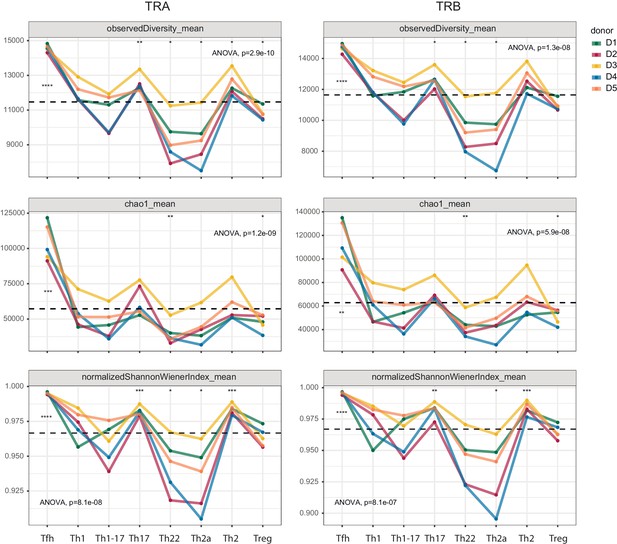
Clonality and diversity of effector/memory CD4+ T-cell subsets.
Observed diversity (top), the Chao1 estimator (middle), and the normalized Shannon-Wiener index (bottom) were calculated for each TCRα (left) and TCRβ repertoire (right) obtained from each effector/memory CD4+ T-cell subset (n = 8) from each healthy donor (n = 5). Dashed lines indicate means. *p<0.05, **p<0.01, ***p<0.001, and ****p<0.0001 (one-way ANOVA followed by the two-sample Welch t-test with Bonferroni correction for each group versus the mean).
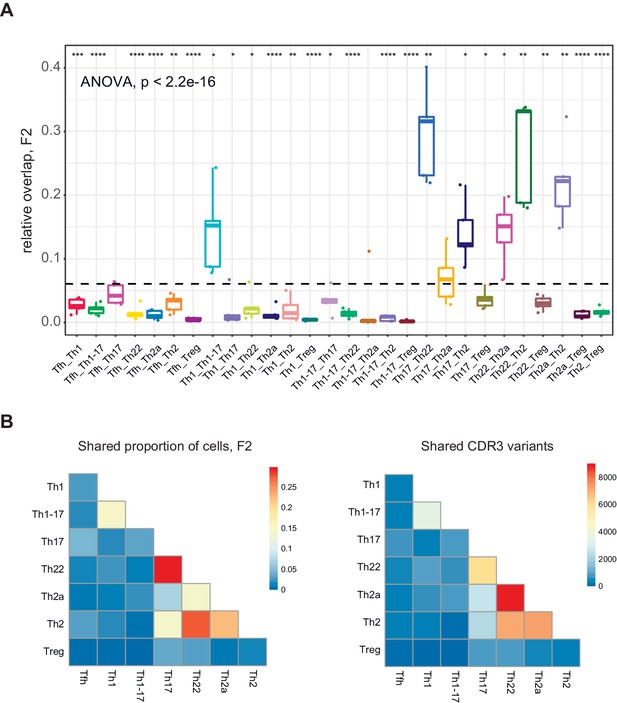
Clonotype overlap among effector/memory CD4+ T-cell subsets.
(A) Relative overlap between nucleotide-defined CDR3β repertoires obtained from donor-matched pairs of effector/memory CD4+ T-cell subsets. Clonotypes were matched on the basis of identical TRBV gene segments and identical CDR3β sequences. Data were normalized to the top 20,000 most frequent clonotypes and weighted by clonotype frequency (F2 metric in VDJtools). The dashed line indicates the mean (n = 5 donors). *p<0.05, **p<0.01, ***p<0.001, and ****p<0.0001 (one-way ANOVA followed by the two-sample Welch t-test with Bonferroni correction for each group versus the mean). (B) Heatmap representations of the weighted overlap (F2 metric in VDJtools, left) and the estimated relative overlap of nucleotide-defined CDR3β clonotypes (calculated via the D metric in VDJtools, right) between donor-matched pairs of effector/memory CD4+ T-cell subsets.
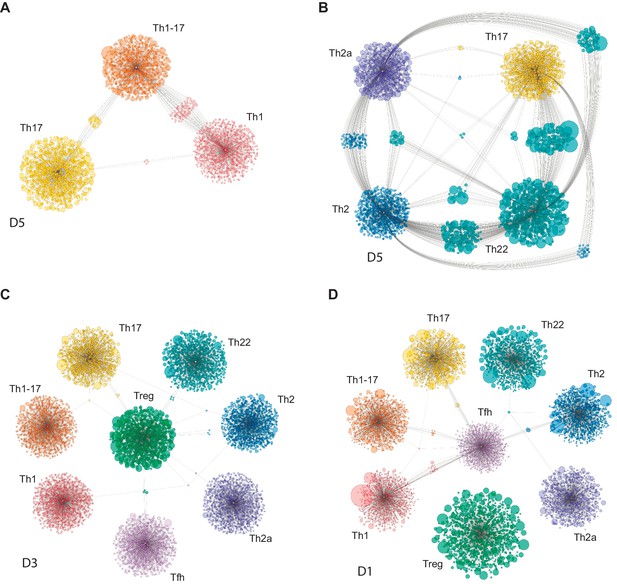
Clonal relatedness among effector/memory CD4+ T-cell subsets.
Cytoscape network analysis schemes represent the number and size (frequency) of nucleotide-defined clonotype variants shared among the top 2000 most frequent CDR3β clonotypes in each subset. Each bubble represents one CDR3β clonotype. The size of each bubble is proportional to the frequency of each CDR3β clonotype in the corresponding repertoire. Shared clonotypes are depicted as connected clouds among the corresponding subsets. The size of each bubble in these clouds is proportional to the frequency of each CDR3β clonotype averaged across the maternal subsets. Representative plots were selected for illustrative purposes from donors D1, D3, and D5. (A) Th1/Th1-17/Th17. (B) Th17/Th22/Th2a/Th2. (C) Tregs versus other subsets. Only clonotypes shared with Tregs are shown. (D) Tfh cells versus other subsets. Only clonotypes shared with Tfh cells are shown.
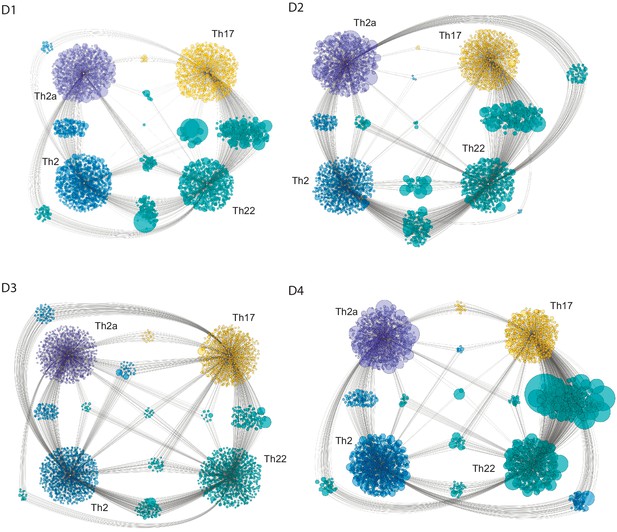
Clonal relatedness among the Th17, Th22, Th2a, and Th2 subsets of effector/memory CD4+ T-cells.
Cytoscape plots for each donor represent the number and size (frequency) of nucleotide-defined clonotype variants shared among the top 2000 most frequent CDR3β clonotypes in each subset. Details as in Figure 5.
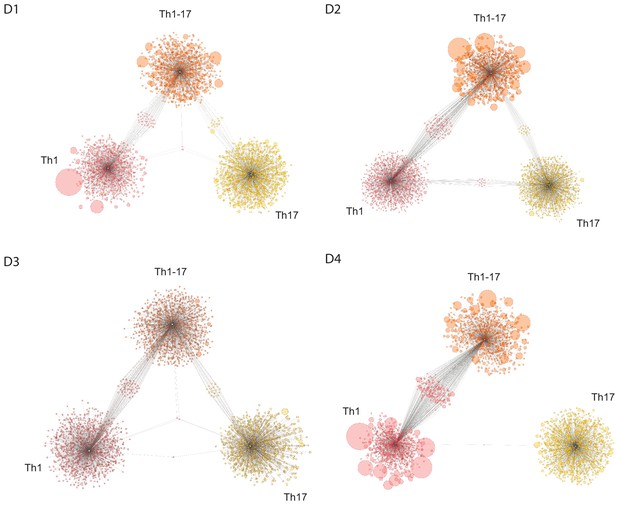
Clonal relatedness among the Th1, Th1-17, and Th17 subsets of effector/memory CD4+ T-cells.
Cytoscape plots for each donor represent the number and size (frequency) of nucleotide-defined clonotype variants shared among the top 2000 most frequent CDR3β clonotypes in each subset. Details as in Figure 5.
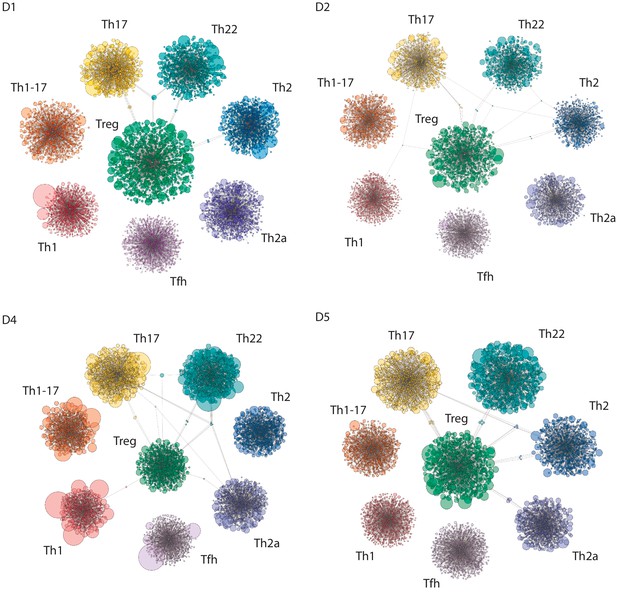
Clonal relatedness among Tregs and other subsets of effector/memory CD4+ T-cells.
Cytoscape plots for each donor represent the number and size (frequency) of nucleotide-defined clonotype variants shared among the top 2000 most frequent CDR3β clonotypes in each subset. Only clonotypes shared with Tregs are shown. Details as in Figure 5.
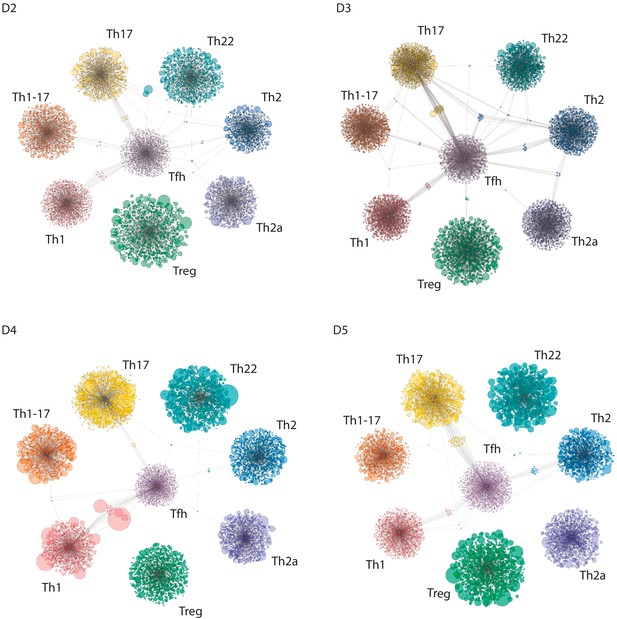
Clonal relatedness among Tfh cells other subsets of effector/memory CD4+ T-cells.
Cytoscape plots for each donor represent the number and size (frequency) of nucleotide-defined clonotype variants shared among the top 2000 most frequent CDR3β clonotypes in each subset. Only clonotypes shared with Tfh cells are shown. Details as in Figure 5.
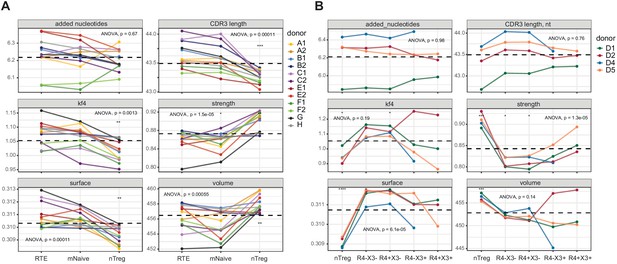
Averaged physicochemical characteristics of CDR3β repertoires from naive CD4+ T-cell subsets.
(A) Repertoire analysis of RTEs (CD25−CD31+), mature naive T cells (mNaive; CD25−CD31−), and naive Tregs (nTreg; CD25high) from healthy donors (n = 12). Matched letters in the key indicate twin pairs. (B) Repertoire analysis of naive Th1-like cells (non-Treg CCR4−CXCR3+), naive Th2-like cells (non-Treg CCR4+CXCR3−), naive Tregs (CD25highCD127low), and the corresponding non-Treg CCR4−CXCR3− and non-Treg CCR4+CXCR3+ populations from healthy donors (n = 4) matching those shown in Figure 2. Averaged physicochemical characteristics were measured for the five amino acids in the middle of the CDR3β sequences obtained from each naive CD4+ T-cell subset. Calculations were weighted by clonotype frequency. Parameter details as in Figure 2. Dashed lines indicate means. *p<0.05, **p<0.01, ***p<0.001, and ****p<0.0001 (one-way ANOVA followed by the two-sample Welch t-test with Bonferroni correction for each group versus the mean).
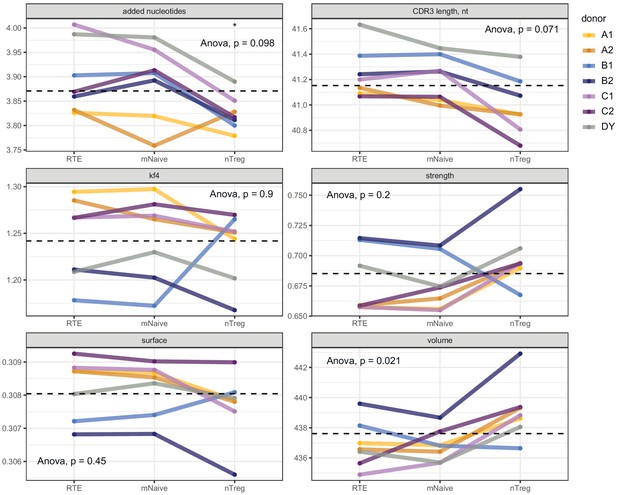
Averaged physicochemical characteristics of CDR3α repertoires from naive CD4+ T-cell subsets.
Repertoire metrics are shown for RTEs (CD25−CD31+), mature naive T cells (mNaive; CD25−CD31−), and naive Tregs (nTreg; CD25high) from healthy donors (n = 7). Matched letters in the key indicate twin pairs. Averaged physicochemical characteristics were measured for the five amino acids in the middle of the CDR3α sequences obtained from each naive CD4+ T-cell subset. Calculations were weighted by clonotype frequency. Parameter details as in Figure 2. Dashed lines indicate means. *p<0.05, **p<0.01, ***p<0.001, and ****p<0.0001 (one-way ANOVA followed by the two-sample Welch t-test with Bonferroni correction for each group versus the mean).
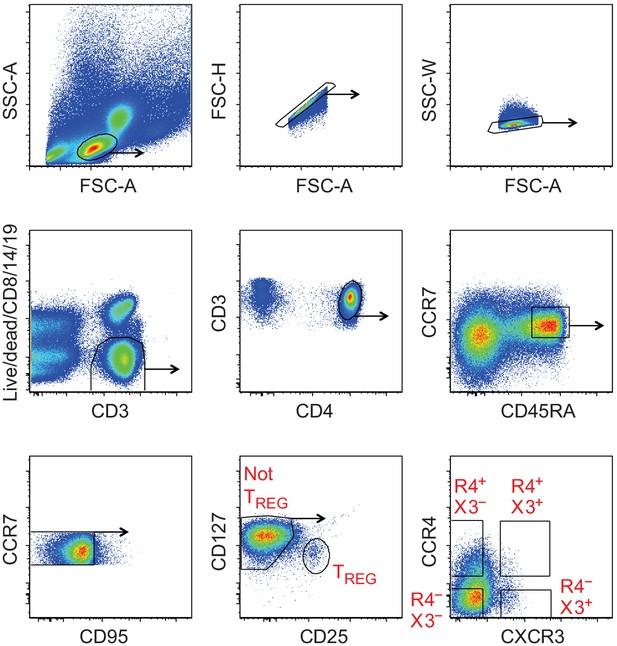
Gating strategy for the identification of naive CD4+ T-cell subsets.
Lymphocytes were identified in a forward scatter-area (FSC-A) versus side scatter-area (SSC-A) plot, and single cells were identified in FSC-A versus forward scatter-height (FSC-H) and FSC-A versus side scatter-width (SSC-W) plots. Naive cells were gated as viable CD3+CD4+CD8−CD14−CD19−CCR7+CD45RA+CD95− events, and subsets were sorted as naive Th1-like cells (non-Treg CCR4−CXCR3+), naive Th2-like cells (non-Treg CCR4+CXCR3−), or naive Tregs (CD25highCD127low), alongside the corresponding non-Treg CCR4−CXCR3− and non-Treg CCR4+CXCR3+ populations.
Tables
Gating strategy for the identification of effector/memory CD4+ T-cell subsets.
Gates 1 and 2 | Gate 3 | Gate 4 | Gate 5 | Gate 6 | Gate 7 | Gate 8 | Subset |
---|---|---|---|---|---|---|---|
Live single CD3+ CD14− CD19− lymphocytes | CD4+ | Exclude CCR7+ CD45RA+ | CD25high CD127low | Treg | |||
CD25low CD127+ | CXCR5+ | Tfh | |||||
CCR10+ | Th22 | ||||||
CXCR5− CCR10− | CXCR3+ CCR6− | CCR4− | Th1 | ||||
CXCR3− CCR6+ | CCR4+ | Th17 | |||||
CXCR3+ CCR6+ | CCR4− | Th1-17 | |||||
CXCR3− CCR6− | CCR4+ CRTh2− | Th2 | |||||
CCR4+ CRTh2+ | Th2a |
-
See also Figure 1—figure supplement 1.
Frequencies of sorted effector/memory CD4+ T-cell subsets.
Donor | Tfh | Th1 | Th1-17 | Th17 | Th22 | Th2a | Th2 | Treg |
---|---|---|---|---|---|---|---|---|
D1 | 5.44 | 1.91 | 1.44 | 3.06 | 2.60 | 1.04 | 4.86 | 3.99 |
D2 | 5.82 | 3.29 | 3.50 | 3.14 | 6.64 | 1.53 | 9.24 | 6.92 |
D3 | 2.05 | 0.19 | 0.31 | 1.31 | 0.81 | 0.26 | 1.93 | 1.84 |
D4 | 6.70 | 2.33 | 2.11 | 4.22 | 2.02 | 0.57 | 7.19 | 3.95 |
D5 | 4.39 | 1.16 | 1.17 | 3.32 | 2.12 | 0.82 | 3.96 | 3.99 |
Mean | 4.88 | 1.78 | 1.71 | 3.01 | 2.84 | 0.84 | 5.44 | 4.14 |
SD | 1.79 | 1.17 | 1.19 | 1.06 | 2.23 | 0.48 | 2.84 | 1.81 |
-
Shown as % of live CD3+CD4+CD14−CD19− non-naive cells. Details in Figure 1—figure supplement 1.
Frequencies of sorted naive CD4+ T-cell subsets.
Donor | Th1-like CCR4−CXCR3+ | Th2-like CCR4+CXCR3− | CCR4− CXCR3− | CCR4+ CXCR3+ | Treg CD25high CD127low |
---|---|---|---|---|---|
D1 | 1.75 | 5.46 | 44.80 | 0.23 | 0.73 |
D2 | 0.77 | 6.77 | 20.40 | 0.32 | 0.57 |
D3 | 0.15 | 5.67 | 42.60 | 0.19 | 1.70 |
D4 | 0.16 | 6.33 | 33.10 | 0.05 | 1.11 |
-
Shown as % of live CD3+CD4+CD8−CD14−CD19− naive cells. Details in Figure 6—figure supplement 2.
property | column used | compare to | subset | p adjusted (BH method) | p | signif | test | p adjusted (BH method) | p | signif | test |
---|---|---|---|---|---|---|---|---|---|---|---|
cdr3_length | zscore | .all. | Th2 | 5.1e-01 | 0.43258 | ns | T-test | 0.600 | 0.4481 | ns | Wilcoxon |
cdr3_length | zscore | .all. | Tfh | 2.4e-01 | 0.14003 | ns | T-test | 0.370 | 0.2333 | ns | Wilcoxon |
cdr3_length | zscore | .all. | Th2a | 7.4e-01 | 0.72099 | ns | T-test | 0.770 | 0.7726 | ns | Wilcoxon |
cdr3_length | zscore | .all. | Th1 | 4.3e-01 | 0.34963 | ns | T-test | 0.770 | 0.7451 | ns | Wilcoxon |
cdr3_length | zscore | .all. | Th1-17 | 9.2e-02 | 0.03070 | * | T-test | 0.250 | 0.0829 | ns | Wilcoxon |
cdr3_length | zscore | .all. | TREG | 4.3e-01 | 0.34053 | ns | T-test | 0.600 | 0.4481 | ns | Wilcoxon |
cdr3_length | zscore | .all. | Th17 | 7.5e-01 | 0.75446 | ns | T-test | 0.670 | 0.5633 | ns | Wilcoxon |
cdr3_length | zscore | .all. | Th22 | 1.1e-01 | 0.04145 | * | T-test | 0.120 | 0.0171 | * | Wilcoxon |
added_nucleotides | zscore | .all. | Th2 | 3.1e-01 | 0.21194 | ns | T-test | 0.330 | 0.1935 | ns | Wilcoxon |
added_nucleotides | zscore | .all. | Tfh | 1.4e-01 | 0.07180 | ns | T-test | 0.330 | 0.1699 | ns | Wilcoxon |
added_nucleotides | zscore | .all. | Th2a | 3.7e-01 | 0.26285 | ns | T-test | 0.330 | 0.1935 | ns | Wilcoxon |
added_nucleotides | zscore | .all. | Th1 | 2.6e-01 | 0.16583 | ns | T-test | 0.540 | 0.3860 | ns | Wilcoxon |
added_nucleotides | zscore | .all. | Th1-17 | 2.2e-02 | 0.00365 | ** | T-test | 0.120 | 0.0251 | * | Wilcoxon |
added_nucleotides | zscore | .all. | TREG | 1.1e-01 | 0.04517 | * | T-test | 0.310 | 0.1485 | ns | Wilcoxon |
added_nucleotides | zscore | .all. | Th17 | 7.4e-01 | 0.70804 | ns | T-test | 0.670 | 0.5633 | ns | Wilcoxon |
added_nucleotides | zscore | .all. | Th22 | 7.6e-02 | 0.01900 | * | T-test | 0.073 | 0.0060 | ** | Wilcoxon |
strength | zscore | .all. | Th2 | 1.1e-02 | 0.00135 | ** | T-test | 0.160 | 0.0362 | * | Wilcoxon |
strength | zscore | .all. | Tfh | 1.8e-06 | 7.3e-08 | **** | T-test | 0.049 | 0.0021 | ** | Wilcoxon |
strength | zscore | .all. | Th2a | 4.3e-01 | 0.34108 | ns | T-test | 0.470 | 0.3118 | ns | Wilcoxon |
strength | zscore | .all. | Th1 | 3.9e-01 | 0.28682 | ns | T-test | 0.610 | 0.4700 | ns | Wilcoxon |
strength | zscore | .all. | Th1-17 | 2.6e-01 | 0.16136 | ns | T-test | 0.370 | 0.2333 | ns | Wilcoxon |
strength | zscore | .all. | TREG | 1.5e-02 | 0.00219 | ** | T-test | 0.310 | 0.1386 | ns | Wilcoxon |
strength | zscore | .all. | Th17 | 1.0e-02 | 0.00104 | ** | T-test | 0.310 | 0.1292 | ns | Wilcoxon |
strength | zscore | .all. | Th22 | 1.0e-01 | 0.03680 | * | T-test | 0.190 | 0.0556 | ns | Wilcoxon |
surface | zscore | .all. | Th2 | 9.2e-02 | 0.03016 | * | T-test | 0.120 | 0.0251 | * | Wilcoxon |
surface | zscore | .all. | Tfh | 2.0e-06 | 1.3e-07 | **** | T-test | 0.049 | 0.0019 | ** | Wilcoxon |
surface | zscore | .all. | Th2a | 2.4e-01 | 0.14789 | ns | T-test | 0.330 | 0.1935 | ns | Wilcoxon |
surface | zscore | .all. | Th1 | 1.2e-01 | 0.05902 | ns | T-test | 0.670 | 0.5392 | ns | Wilcoxon |
surface | zscore | .all. | Th1-17 | 5.5e-02 | 0.01270 | * | T-test | 0.310 | 0.1485 | ns | Wilcoxon |
surface | zscore | .all. | TREG | 3.1e-01 | 0.21607 | ns | T-test | 0.430 | 0.2785 | ns | Wilcoxon |
surface | zscore | .all. | Th17 | 1.1e-01 | 0.04722 | * | T-test | 0.330 | 0.1814 | ns | Wilcoxon |
surface | zscore | .all. | Th22 | 1.6e-01 | 0.08850 | ns | T-test | 0.190 | 0.0603 | ns | Wilcoxon |
volume | zscore | .all. | Th2 | 5.5e-02 | 0.01168 | * | T-test | 0.120 | 0.0208 | * | Wilcoxon |
volume | zscore | .all. | Tfh | 4.6e-03 | 0.00039 | *** | T-test | 0.120 | 0.0229 | * | Wilcoxon |
volume | zscore | .all. | Th2a | 7.8e-02 | 0.02114 | * | T-test | 0.310 | 0.1485 | ns | Wilcoxon |
volume | zscore | .all. | Th1 | 1.4e-01 | 0.06982 | ns | T-test | 0.310 | 0.1120 | ns | Wilcoxon |
volume | zscore | .all. | Th1-17 | 1.2e-01 | 0.05435 | ns | T-test | 0.190 | 0.0603 | ns | Wilcoxon |
volume | zscore | .all. | TREG | 5.3e-01 | 0.46185 | ns | T-test | 0.670 | 0.6131 | ns | Wilcoxon |
volume | zscore | .all. | Th17 | 2.3e-01 | 0.13201 | ns | T-test | 0.480 | 0.3294 | ns | Wilcoxon |
volume | zscore | .all. | Th22 | 3.8e-02 | 0.00721 | ** | T-test | 0.120 | 0.0140 | * | Wilcoxon |
kf4 | zscore | .all. | Th2 | 6.3e-01 | 0.56570 | ns | T-test | 0.770 | 0.7726 | ns | Wilcoxon |
kf4 | zscore | .all. | Tfh | 3.5e-07 | 7.3e-09 | **** | T-test | 0.049 | 0.0031 | ** | Wilcoxon |
kf4 | zscore | .all. | Th2a | 6.3e-01 | 0.57609 | ns | T-test | 0.680 | 0.6387 | ns | Wilcoxon |
kf4 | zscore | .all. | Th1 | 5.0e-01 | 0.41381 | ns | T-test | 0.670 | 0.6131 | ns | Wilcoxon |
kf4 | zscore | .all. | Th1-17 | 4.3e-01 | 0.32935 | ns | T-test | 0.670 | 0.5879 | ns | Wilcoxon |
kf4 | zscore | .all. | TREG | 9.2e-02 | 0.02848 | * | T-test | 0.170 | 0.0431 | * | Wilcoxon |
kf4 | zscore | .all. | Th17 | 1.2e-01 | 0.05305 | ns | T-test | 0.310 | 0.1386 | ns | Wilcoxon |
kf4 | zscore | .all. | Th22 | 7.1e-01 | 0.66248 | ns | T-test | 0.670 | 0.5879 | ns | Wilcoxon |