A machine-vision approach for automated pain measurement at millisecond timescales
Figures
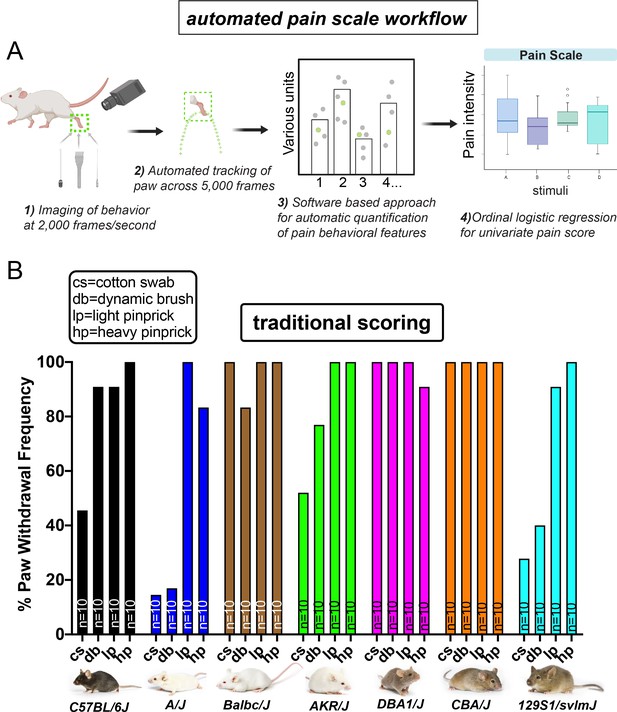
Automated pain assessment workflow in comparison to traditional unidimensional pain scoring.
(A) Workflow pipeline in following order consisting of 1) high-speed videography of freely behaving mice, 2) machine/deep learning-based approaches for automatic tracking of the stimulated paw, 3) PAWS software for automatic quantification of defined pain behavioral features, 4) statistical modeling with ordinal logistic regression for separation of touch versus pain on a trial-by-trial basis. (B) Traditional scoring focused on paw withdrawal frequencies to four mechanical stimuli: cs = cotton swab, db = dynamic brush, lp = light pinprick, hp = heavy pinprick. N = 10 mice per strain. Images from Jackson laboratories.
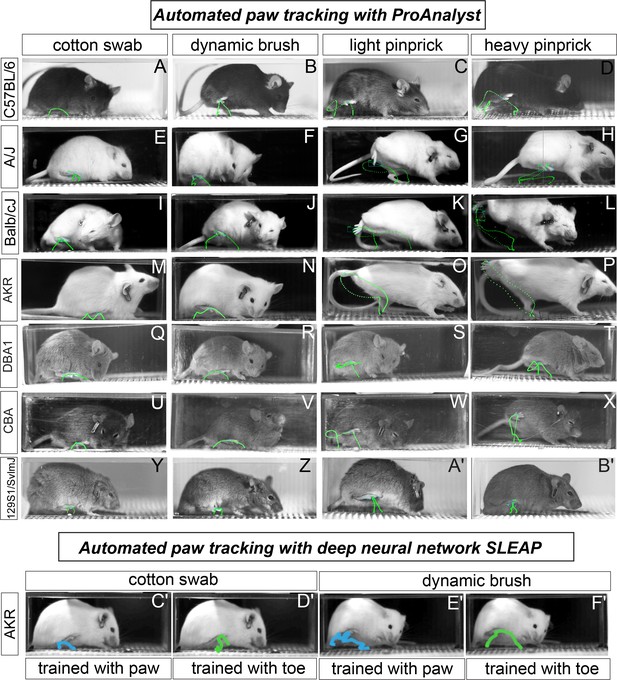
Automated paw tracking with a high-speed recording of behavior.
(a-b′) ProAnalyst machine learning-based paw tracking. (y-z′), SLEAP deep neural network based paw or toe tracking. All still images represent a single frame of ~5000 total frames. Green and blue lines display paw trajectory patterns during the entire behavior. N = 10/mice per stimulus and images shown are representative of each strain.
Automatic paw tracking with the delivery of noxious mechanical stimulus.
Video recording at 2000 fps showing the stimulated paw (received heavy pinprick) successfully tracked throughout the behavioral sequence. Green dots display paw trajectory patterns mapped using ProAnalyst.
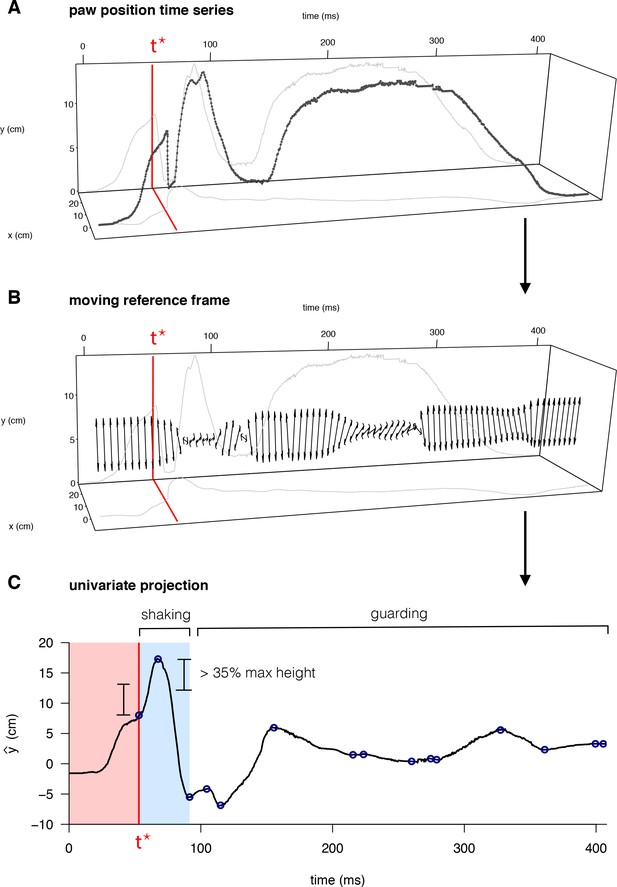
Quantification of behavioral features for mouse pain state.
(A) Raw paw positions (x, y) measured in the camera reference frame (anterior/posterior displacement and vertical height, respectively) as a function of time, with the time of the first peak in paw height, t*, marked in red. (B) The principal axis of paw displacement in a moving time window (double-sided arrow), shown as a function of time. (C) Displacement in the principal axis moving reference frame. Local maxima and minima are indicated by blue circles – the first of which occurs at time t*. Periods of paw shaking were identified based on sequences of displacements between maxima and minima above a given threshold relative to the maximum paw height displacement. Guarding periods were defined simply as periods without shaking, in which the paw remained elevated above its final resting point.
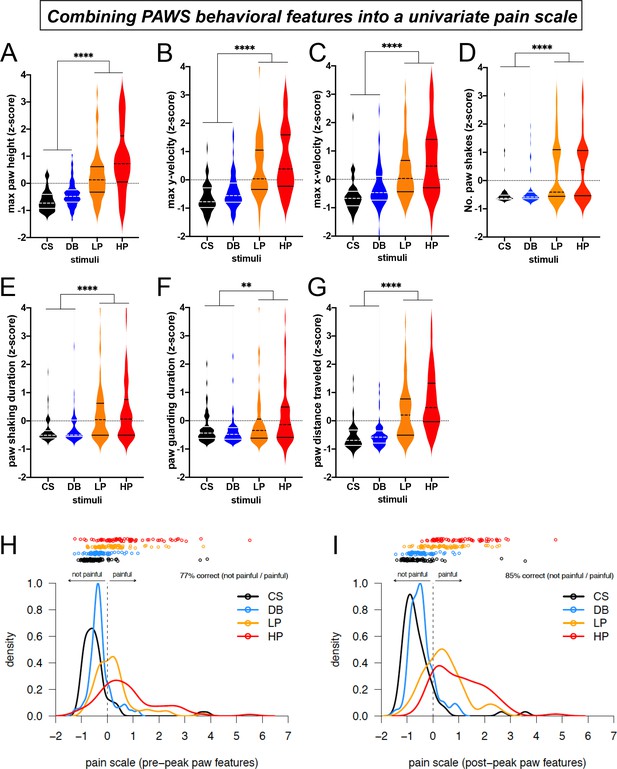
Automated measurement of pain behavioral features across the seven strains with new software PAWS.
Measurements are converted to Z-score to reveal deviation from the mean of individual measures and to standardize units across the seven measures. (A-C) Pain measurements of the stimulated paw from lift to max height. (D-G) Pain measurements of the stimulated paw from max height to paw return. N = 10/mice of each strain given each stimulus once. Statistical significance was computed by comparing CS+DB versus LP+HP with Wilcoxon matched-pairs signed-rank test. ** represents p-value ≤0.01. **** represents p-value ≤0.0001. On the violin plots, black or white horizontal lines represent quartiles and black or white dashed horizontal lines represent the median. (H) The univariate linear scale that best separates the four pain states studied: CS, DB, LP, and HP, for pre-peak features only. Kernel density estimates and the original data decomposed by pain state are shown projected onto the univariate measure of pain identified by ordinal logistic regression. Here, we use the threshold between no-pain and pain (DB and LP) inferred by ordinal logistic regression as the zero point, and we rescale by the threshold between low pain (LP) and high pain (HP), fixing this point at one. (I) Same as a but for post-peak paw features only.
-
Figure 4—source data 1
Raw data values automatically computed from PAWS showing each mouse and stimulus across all eight inbred lines tested.
p=pain, NP = no pain.
- https://cdn.elifesciences.org/articles/57258/elife-57258-fig4-data1-v2.xlsx
-
Figure 4—source data 2
Raw data values automatically computed from PAWS showing each mouse and stimulus across four inbred lines tested before and after unilateral CFA paw injection.
p=pain, NP = no pain.
- https://cdn.elifesciences.org/articles/57258/elife-57258-fig4-data2-v2.xlsx
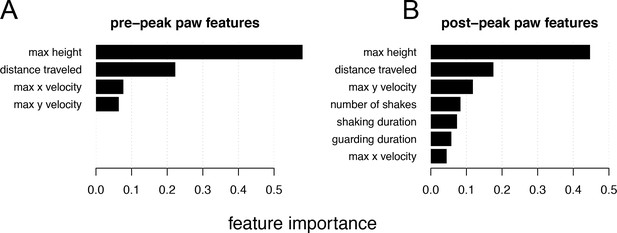
Relative feature importance for individual behavioral features that contribute to the univariate pain score.
(A) Magnitude of the weights assigned by ordinal logistic regression to the standardized (mean subtracted, variance scaled) pre-peak paw behavioral features. (B) Same as a but for post-peak paw features.
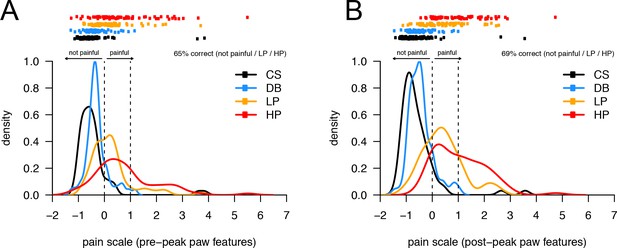
The univariate linear scale that best separates the four pain states studied: CS, DB, LP, and HP.
Related to panels 4 H, I. Here, we are asking the model to distinguish between three conditions: no pain (CS or DB), light pain (LP), or heavy pain (HP).
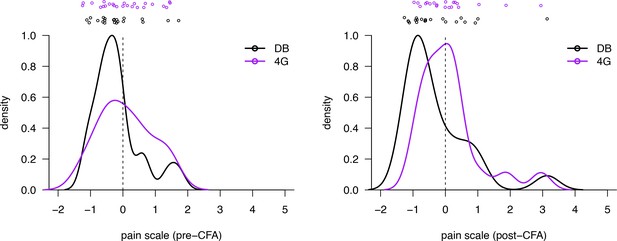
Univariate linear scale using post-paw peak features before and after CFA injection and application of dynamic brush or 4 g von Frey hair filament.
Kernel density estimates and the original data decomposed by pain state are shown projected onto the univariate measure of pain identified by ordinal logistic regression. As in Figure 2, we use the threshold between no-pain and pain (DB and LP) inferred by ordinal logistic regression as the zero point, and we rescale by the threshold between low pain (LP) and high pain (HP), fixing this point at one. Please note that we are not plotting an outlier DB data point in the pre-CFA panel (pain score was >10).
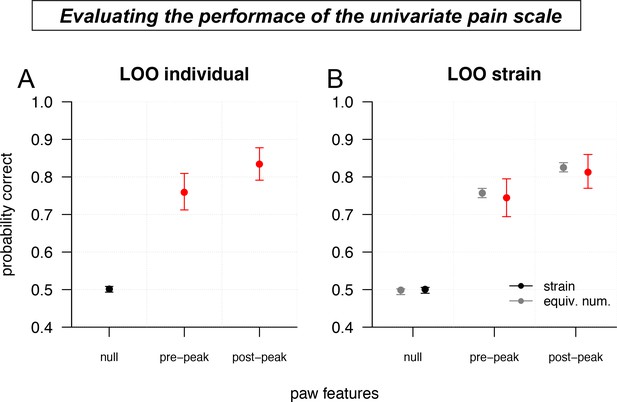
Validating the accuracy of the univariate pain scale.
(A) Leave-one-out (LOO) cross-validation performance (binary classification accuracy of not pain/pain) of the pre-peak and post-peak univariate pain scales, compared to a null model (random assignment), for individual mice. Error bars show nonparametric bootstrap 95% confidence intervals. (B) Same as a, but leaving out an entire strain (colors) or an equivalent number of mice chosen uniformly at random (gray).
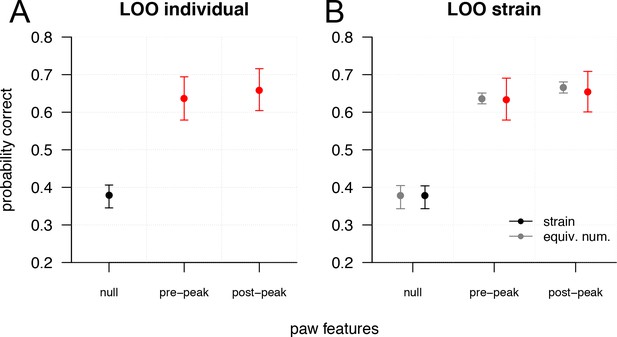
Validating the accuracy of the univariate pain scale.
Related to Figure 5. Plots show ternary classification accuracy (no pain/LP/HP).
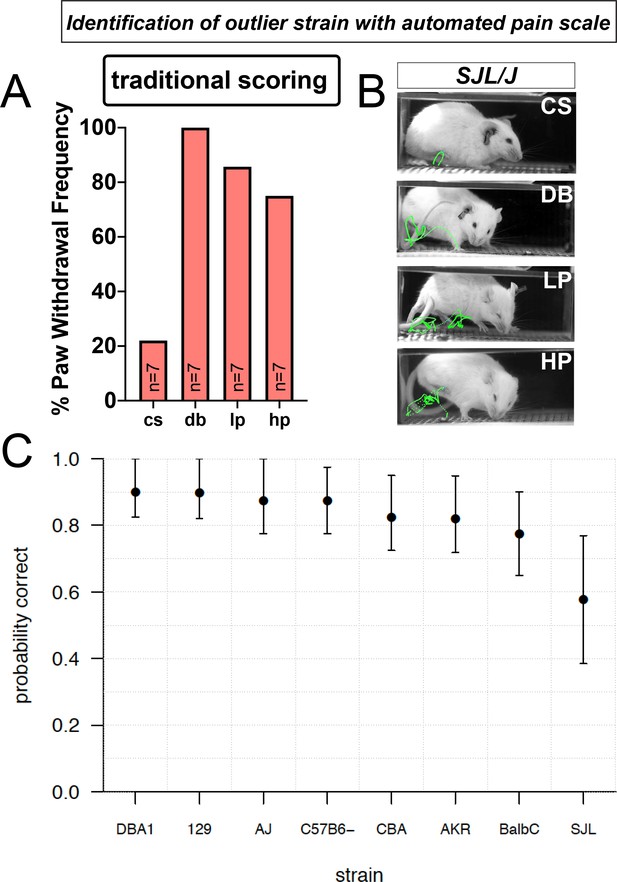
Automated pain assessment platform uncovers an outlier strain.
(A) Traditional scoring focused on paw withdrawal frequencies to four mechanical stimuli: cs = cotton swab, db = dynamic brush, lp = light pinprick, hp = heavy pinprick. N = 7 mice for the SJL strain. (B) ProAnalyst tracking showing SJL mice have pain-like response to dynamic brush and heightened pinprick responses. (C) Leave-one-out (LOO) cross validation by strain shows stimulus prediction for SJL mice is poor, likely because their responses typically map outside of the normal range of the other seven strains. Error bars show nonparametric bootstrap 95% confidence intervals.
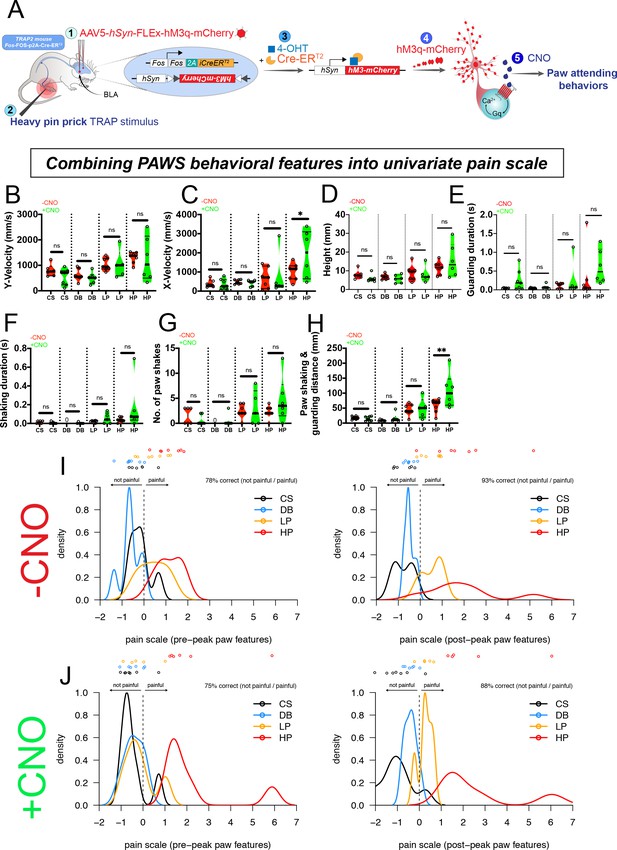
Pain hypersensitivity with chemogenetic activation of the BLA pain ensemble automatically captured via PAWS.
(A) Schematic to permanently tag pain-active neurons in the BLA with an excitatory DREADD in transgenic painTRAP2hM3 mice. (B-D) Automatic measurement of pre-paw peak features comparing mice at baseline (-CNO) to mice administered CNO. (E-H) Automatic measurement of post-paw peak features comparing mice at baseline (-CNO) to mice administered CNO. Stimulus abbreviations are same as above. Statistical significance was computed with student’s t-test. *p-value ≤0.05. **p-value ≤0.01. Raw values were plotted instead of z-scores as shown in Figure 3 because we are plotting only six mice in this figure. (I-J) Ordinal logistic regression reveals separation of innocuous versus noxious stimuli at baseline (-CNO) and further segregation of the HP group after CNO, only when using all seven paw features. N = 6 mice tested in these experiments. The second panel in J omits one outlier mouse (HP treatment) that produced pain score >25.
-
Figure 7—source data 1
Raw data values automatically computed from PAWS showing each painTRAP2hM3 mouse combined with sensory stimuli before and after CNO administration.
p=pain, NP = no pain.
- https://cdn.elifesciences.org/articles/57258/elife-57258-fig7-data1-v2.xlsx
Tables
Reagent type (species) or resource | Designation | Source or reference | Identifiers | Additional information |
---|---|---|---|---|
Genetic reagent (M. musculus) | C57BL/6J | Jackson Laboratory | RRID:IMSR_JAX:000664 | |
Genetic reagent (M. musculus) | A/J | Jackson Laboratory | RRID:IMSR_JAX:000646 | |
Genetic reagent (M. musculus) | 129S1/SvlmJ | Jackson Laboratory | Stock No: 002448 | |
Genetic reagent (M. musculus) | BALB/cJ | Jackson Laboratory | Stock No: 000651 | |
Genetic reagent (M. musculus) | DBA/1J | Jackson Laboratory | Stock No: 000670 | |
Genetic reagent (M. musculus) | AKR/J | Jackson Laboratory | RRID:IMSR_JAX:000648 | |
Genetic reagent (M. musculus) | CBA/J | Jackson Laboratory | Stock No: 000656 | |
Genetic reagent (M. musculus) | SJL/J | Jackson Laboratory | Stock No: 000686 | |
Genetic reagent (M. musculus) | Fos-FOS-2A-iCre-ERT2 | Jackson Laboratory | Stock no: 030323 | PMID:28912243 |
Chemical compound, drug | Clozapine N-oxide | Sigma | C0832 | 3 mg/kg |
Chemical compound, drug | Complete Freund’s Adjuvant | Sigma | F5881 | 10 μL/paw |
Software, algorithm | PAWS | this paper | https://github.com/crtwomey/paws | |
Software, algorithm | ProAnalyst | https://www.xcitex.com/proanalyst-motion-analysis-software.php | ||
Software, algorithm | SLEAP | https://sleap.ai/ | PMID:30573820 |