Distributed functions of prefrontal and parietal cortices during sequential categorical decisions
Figures
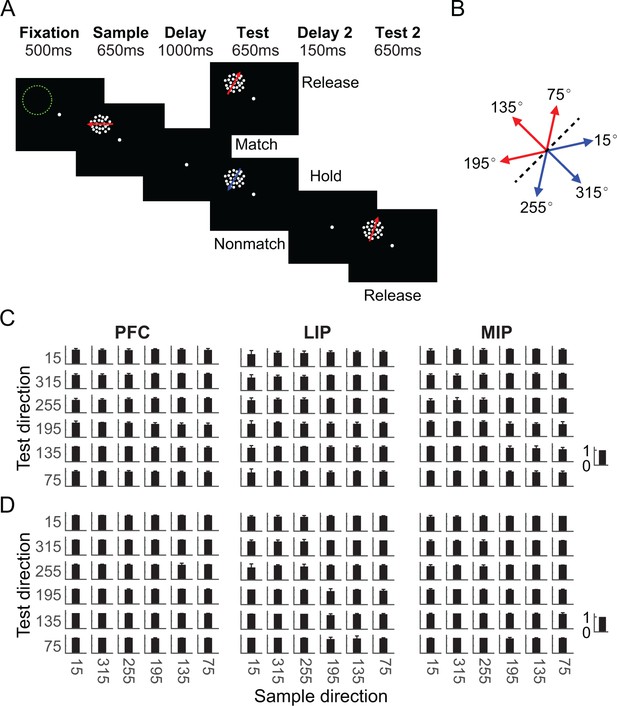
Task and behavioral performance.
(A) Sequence of the delayed match to category (DMC) task. Monkeys needed to release a touch-bar when the categories of sample and test stimuli matched, or hold the bar and wait for the second test stimulus when they did not match. The matching (M)/nonmatching (NM) decision was required to be made during the test period to receive the reward. Stimulus offset occurred immediately after monkeys released the touched-bar. The green dashed circle indicates the position of a neuron’s receptive field. (B) Monkeys needed to group six motion directions into two categories (corresponding to the red and blue arrows) separated by a learned category boundary (black dashed line). (C, D) Two monkeys’ average performance (accuracy) for all stimulus conditions during recordings from prefrontal cortex (PFC), lateral intraparietal (LIP), and medial intraparietal (MIP) recordings are shown separately. Each row corresponds to one monkey. The error bar denotes standard deviation.
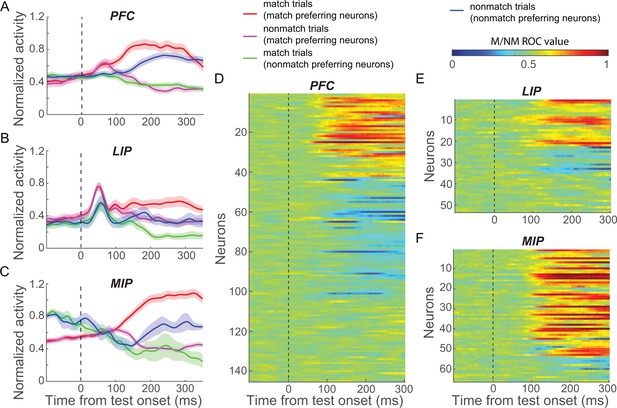
Matching (M)/nonmatching (M/NM) selectivity in prefrontal cortex (PFC), lateral intraparietal (LIP), and medial intraparietal (MIP) areas.
(A–C) The normalized population activity of both match-preferring and nonmatch-preferring neurons in PFC (A), LIP (B), and MIP (C). The shaded area represents ± SEM. (D–F) The strength of the M/NM selectivity was evaluated using receiver-operating characteristic analysis for all neurons in PFC (D), LIP (E), and MIP (F). Values close to 0.0 and 1.0 correspond to strong encoding preference for nonmatch and match, respectively. Values of 0.5 indicate no M/NM selectivity.

Examples of match and nonmatch-preferring neurons in prefrontal cortex (PFC), lateral intraparietal (LIP), and medial intraparietal (MIP) areas.
(A) The averaged activity of a match-preferring PFC neuron during match trials (red) and nonmatch trials (blue). Shaded area denotes ± SEM. The gray histogram represents the reaction time distribution on match trials. (B) Activity of a nonmatch-preferring PFC neuron. (C, D) The activity of match-preferring (C) and nonmatch-preferring (D) example neurons in LIP. (E, F) The activity of match-preferring (E) and nonmatch-preferring (F) example neurons in MIP.
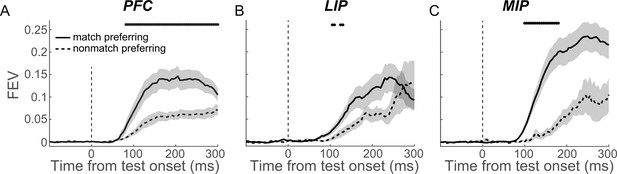
Comparison of the matching/nonmatching (M/NM) selectivity between match-preferring and nonmatch-preferring neurons.
(A) The M/NM selectivity of match-preferring (solid) and nonmatch-preferring (dashed) neurons in prefrontal cortex (PFC) was evaluated using the unbiased fraction of explained variance (FEV). Shaded area denotes ± SEM. (B, C) The M/NM selectivity of neurons in lateral intraparietal (LIP) (B) and medial intraparietal (MIP) (C) areas.
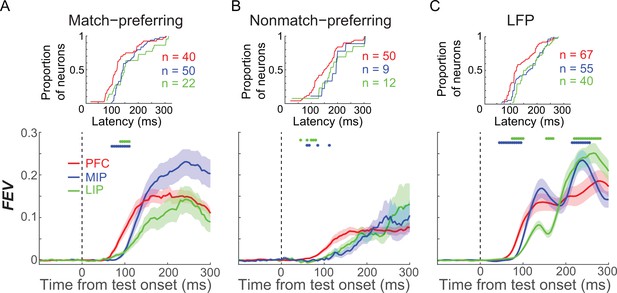
The comparison of matching/nonmatching (M/NM) selectivity between prefrontal cortex (PFC), lateral intraparietal (LIP), and medial intraparietal (MIP) areas.
(A, B) The magnitude and time course of M/NM selectivity was determined using unbiased fraction of explained variance (FEV). Different colors represent different cortical areas, and the shaded area represents ± SEM. The blue dots denote the time points for which there were significant differences between PFC and MIP, while the green dots denote the time points for which there were significant differences between PFC and LIP (p<0.05, Wilcoxon test). The upper inset figures show the cumulative distribution of the latency of M/NM selectivity. (C) The M/NM selectivity of LFP amplitude in PFC, LIP, and MIP, which is shown in the same format as (A). The LFP signal from all recording channels in each area is included.
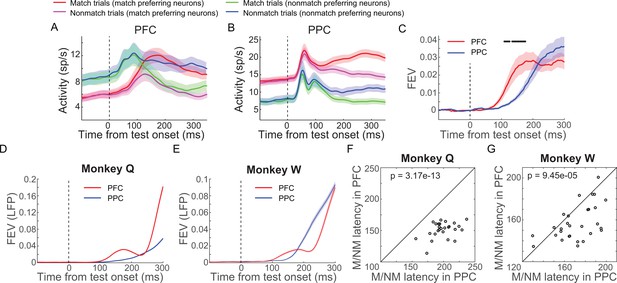
Comparison of matching/nonmatching (M/NM) selectivity between prefrontal cortex (PFC) and PPC in the delayed match to category (DMC) task.
(A, B) The population activity of match-preferring and nonmatch-preferring neurons in PFC (A) and PPC (B) is shown as a function of time. (C) The M/NM selectivity of all PFC (red) and PPC (blue) neurons is evaluated using unbiased fraction of explained variance (FEV). PFC showed significantly earlier M/NM selectivity (p=0.0158, Wilcoxon test). (D, E) The M/NM selectivity of local field potential (LFP) amplitude in PFC (red) and PPC (blue) is shown separately for two monkeys. LFP signals were recorded simultaneously from PFC and PPC by using multi-channel recording. (F, G) Comparisons of the mean time point in which the LFP signal initially showed significant M/NM selectivity between PFC and PPC (p<0.01, one-way ANOVA). Each symbol represents the averaged start time in all recording channels within one recording session. The shaded area represents ± SEM.
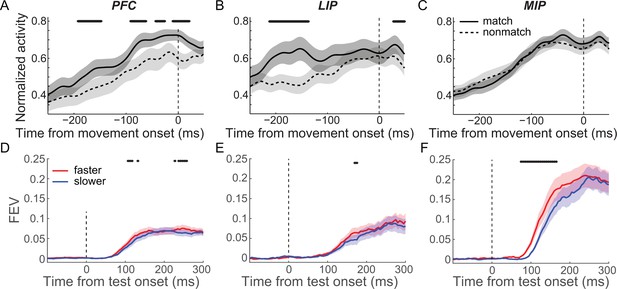
Matching/nonmatching (M/NM) selectivity in medial intraparietal (MIP) cortex but not prefrontal cortex (PFC) and lateral intraparietal (LIP) cortex primarily correlated with monkeys’ hand movement for reporting M/NM decisions.
(A–C) The population activity of match-preferring neurons during match and nonmatch trials when activity was aligned to the start of hand movement. The black stars mark the time periods for which there were significant differences (p<0.01, paired t test). (D–F) The time course and magnitude of the M/NM selectivity in faster trials (red) and slower trials (blue) were evaluated using unbiased fraction of explained variances (FEVs) for PFC (D), LIP (E), and MIP (F). The shaded area represents ± SEM, and the black dots denote the time points for which there were significant differences (p<0.01, paired t-test).
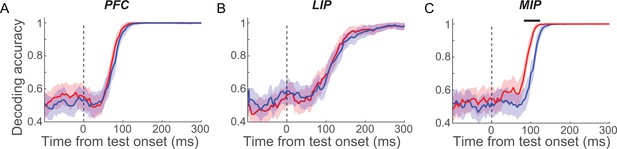
Matching/nonmatching (M/NM) selectivity in medial intraparietal (MIP) cortex correlated with monkeys’ reaction times (RTs).
(A–C) Support vector machine (SVM) decoding performance for M/NM choices in faster (red) and slower trials (blue) for prefrontal cortex (PFC) (A), lateral intraparietal (LIP) (B), and medial intraparietal (MIP) (C) areas. The shaded area represents ± STD, and the black dots mark the time point when there were significant differences (p<0.05, bootstrap).
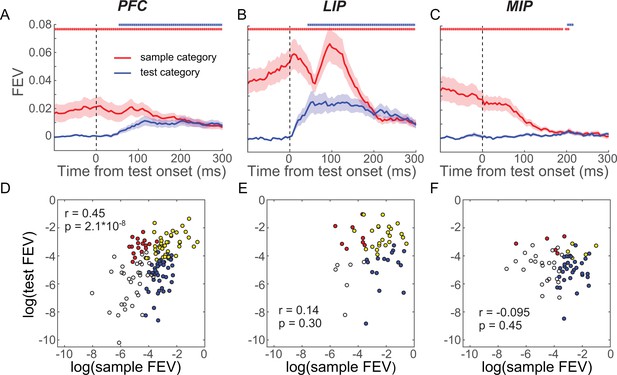
Sample and test category representation in prefrontal cortex (PFC), lateral intraparietal (LIP), and medial intraparietal (MIP) areas.
(A–C) The selectivity of sample category (red) and test category (blue) was evaluated using the unbiased fraction of explained variance (FEV) for all neurons in PFC (A), LIP (B), and MIP (C). The shaded area represents ± SEM. The red and blue dots represent the time points for which the sample and test category selectivity are significantly greater than chance level (p<0.01, paired t-test), respectively. (D–F) The correlations between sample category and test category selectivity (using FEV) during the test period for all neurons in PFC (D), LIP (E), and MIP (F). Each symbol represents a single neuron. Yellow dots denote neurons that showed significantly mixed sample test category selectivity. The blue and red dots denote the neurons that showed only significant sample category or test category selectivity, respectively, and the black circles denote the neurons that did not show significant category selectivity (one-way ANOVA test, p<0.01).
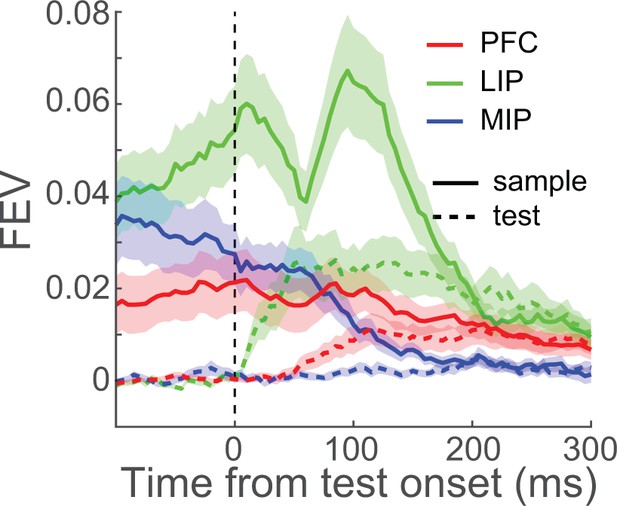
Comparisons of category selectivity among prefrontal cortex (PFC), lateral intraparietal (LIP), and medial intraparietal (MIP) areas.
Both sample (solid) and test (dashed) category selectivity of PFC (red), LIP (green), and MIP (blue) neurons were evaluated using unbiased fraction of explained variance (FEV). The shaded area represents ± SEM.
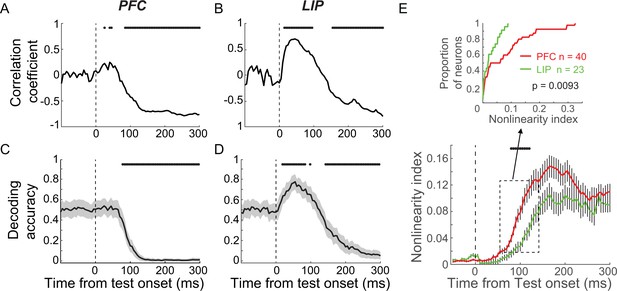
Mixed category selectivity was more nonlinear in prefrontal cortex (PFC) than in lateral intraparietal (LIP) cortex.
(A, B) The correlation coefficient between the test category selectivity (using receiver-operating characteristic [ROC] value) of two sample category conditions is shown for both PFC (A) and LIP (B). The black dots mark the time points for which the correlation was statistically significant (p<0.01, t-test). (C, D) The decoding performance of a test category classifier using neuronal activity in PFC (C) and LIP (D). The support vector machine (SVM) classifier was trained by using activity from one sample category condition (e.g., S1T1 vs. S1T2) and tested with activity from the other sample category conditions (e.g., S2T1 vs. S2T2). The shaded area represents ± STD, and the black stars mark the time points for which the decoder performance is significantly different from chance level (bootstrap, p<0.05). (E) The nonlinearity index of mixed category-selective neurons in both PFC (red) and LIP (green). The shaded area represents ± SEM, and the black stars denote the time points for which there is a significant difference between LIP and PFC (unpaired t-test, p<0.05). The upper panel shows the cumulative distribution of nonlinearity index shortly after test onset (50–150 ms after test onset) for both PFC and LIP neurons.
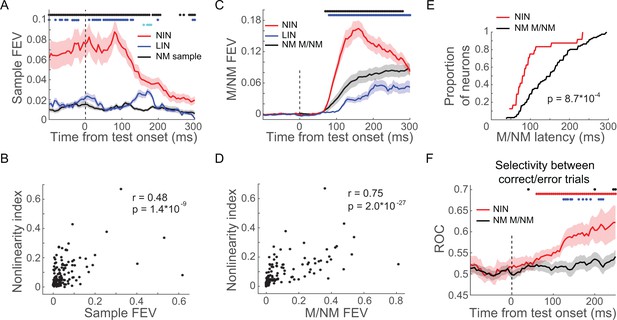
Nonlinearly integrating neurons (NIN) in prefrontal cortex (PFC) were more engaged in the delayed match to category (DMC) task.
(A) The sample category selectivity of the NIN, linearly integrating neurons (LIN), and nonmixed sample category-selective neurons in PFC were compared using fraction of explained variance (FEV). The shaded area denotes ± SEM. The blue and black dots denote the time point for which the NINs were significantly different from LINs and nonmixed sample category-selective neurons (NM sample), respectively; while the cyan dots denote the time point for which there was significant difference between LIN and NM sample (p<0.05, Wilcoxon test). (B) The correlation between sample category selectivity and nonlinearity indices of PFC neurons. Each dot denotes one single neuron. (C) The matching/nonmatching (M/NM) selectivity of the NINs, LINs, and the nonmixed M/NM-selective neurons (NM M/NM) in PFC were compared using FEV. The colored dots denote the statistical significance in the same format as in (A). (D) Correlation between M/NM selectivity and nonlinearity index of PFC neurons. (E) The cumulative distribution of the latency of M/NM selectivity for NINs and NM M/NM. (F) The change in activity on incorrect match trials relative to correct match trials was evaluated using receiver-operating characteristic (ROC) for both NINs and NM M/NM neurons. The shaded area denotes ± SEM. The red and black dots denote the time points for which the activity changes of NINs and NM M/NM neurons were statistically significant (p<0.05, paired t-test), respectively; while the blue dots denote the time points for which there were significant differences between NINs and the NM M/NM neurons (p<0.05, Wilcoxon test).

Selectivity profile of prefrontal cortex (PFC) (A) and lateral intraparietal (LIP) (B) neurons.
A two-way ANOVA was performed on test-period activity with sample and test categories as factors. Linearly integrating neurons (LIN) were defined as those that exhibited main effects of both sample and test categories (p<0.01), but a nonsignificant interaction term (4); while nonlinearly integrating neurons (NIN) were defined as those that exhibited main effects of both sample and test categories (p<0.01), as well as a significant interaction term (p<0.01) (7). Nonmixed sample category-selective neurons were defined as those that exhibited main effects of only sample but not test categories (p<0.01), including 1 and 5. Nonmixed matching/nonmatching (M/NM)-selective neurons were defined as that exhibited a significant interaction term (p<0.01), but not mixed sample and test category selectivity, including 3, 5, and 6.
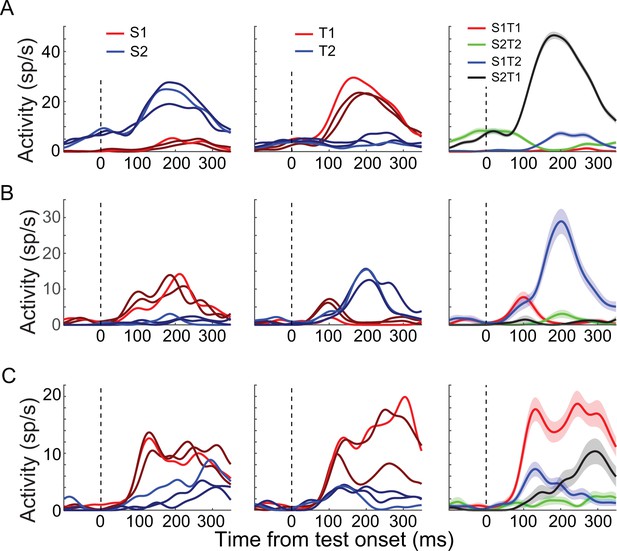
Nonlinearly integrating neurons (NIN) in prefrontal cortex (PFC).
(A–C) The activity of three example NINs. The first and second columns show the condition averaged activity sorted by sample direction and test direction, respectively. The lighter colors correspond to directions in the center of each category, and darker colors correspond to all other directions. The third column shows the mean activity of each sample test category combination. The shaded area denotes ± SEM. S: sample category; T: test category.
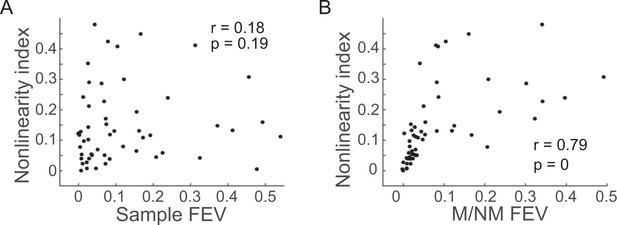
Correlation between nonlinearly integrative encoding and encoding of task variables in lateral intraparietal (LIP) cortex.
(A) Correlation between nonlinearity indices and sample category selectivity. (B) Correlation between nonlinear indices and matching/nonmatching (M/NM) selectivity.
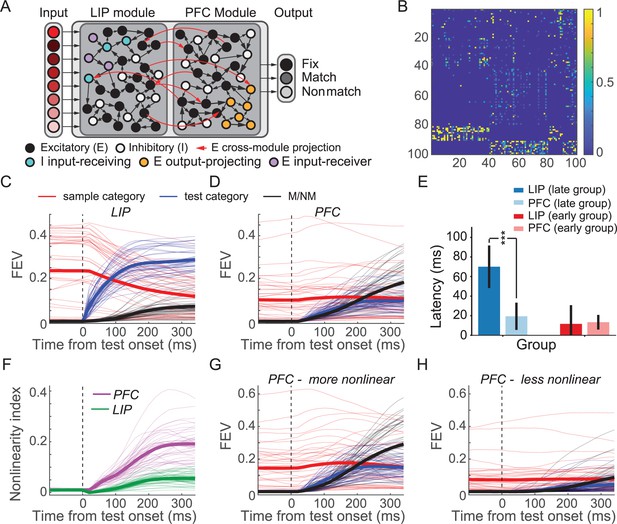
Two-module recurrent neural networks (RNNs) showed similar patterns of activity and dynamics as in neural data.
(A) Model schematic of the two-module ‘frontoparietal’ RNNs. Each RNN consists of 24 motion direction turned input units, 100 hidden units, and 3 response units. The hidden layer of each RNN consists of two modules simulating lateral intraparietal (LIP) and prefrontal cortex (PFC), respectively, with half of the units designated to each module (and E/I proportion maintained). Both the excitatory and inhibitory units in each module are recurrently connected within each module. The cross-module connections are more sparse than recurrent connection within each module. Only excitatory units project to the units in the other module. (B) Example recurrent connectivity matrix of an example two-module RNN. Inhibition is strictly local to each module, as is emphasized by the block-diagonal structure in the bottom fifth of rows. Excitatory projections between modules are sparse, while excitatory projections within modules are denser. Each row/column represents one unit. The 1–40th and 41–80th represent PFC and LIP excitatory units, respectively; while the 81–90th and 91–100th represent LIP and PFC inhibitory units, respectively. (C) The averaged sample category selectivity, test category selectivity, and matching/nonmatching (M/NM) selectivity of units in the LIP modules of the 41 successfully trained RNNs were quantified using fraction of explained variance (FEV). Each thin line denotes the result from one RNN. The thick lines denote the average of all the RNNs. (D) The sample category selectivity, test category selectivity, and M/NM selectivity of PFC modules. (E) The comparison of the latencies of M/NM selectivity between LIP and PFC modules. All RNNs were separated into the late and early group based on the latency of the M/NM selectivity in LIP module. The error bar denotes STD. (F) The averaged nonlinearity index of units in LIP (green) and PFC (pink) modules. The thick lines denote the average across all the RNNs. (G, H) The task variable encodings (sample, test, and M/NM) of the more-nonlinear (G) and less-nonlinear (H) groups of units in the PFC module are shown separately.
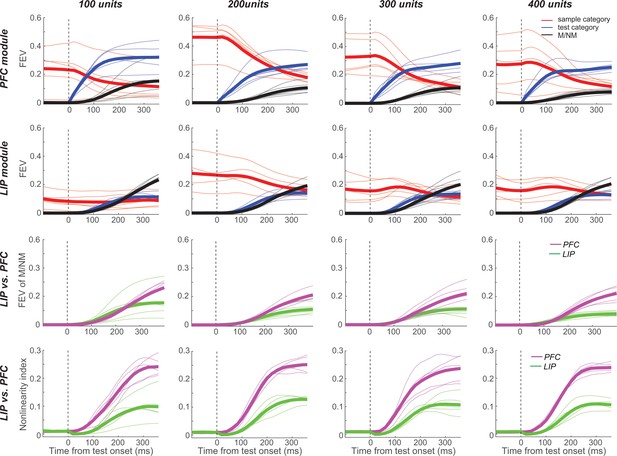
We observe similar patterns of activity and task variable encoding across recurrent neural networks (RNNs) with different sizes (50, 100, 150, and 200 units in each module, 100, 200, 300, and 400 units for the whole networks, respectively).
The first row shows the averaged sample category selectivity, test category selectivity, and matching/nonmatching (M/NM) selectivity of units in the lateral intraparietal (LIP) modules of each group of RNNs across different sizes, quantified using fraction of explained variance (FEV). Each thin line denotes the result from one RNN, while the thick lines denote the average across all five example RNNs. The second row shows identical information (selectivity for sample category, test category, and M/NM) in the prefrontal cortex (PFC) module. The third row shows the comparison of the M/NM selectivity between LIP (green) and PFC (pink) modules. The fourth row shows the averaged nonlinearity index of units in LIP (green) and PFC (pink) modules.
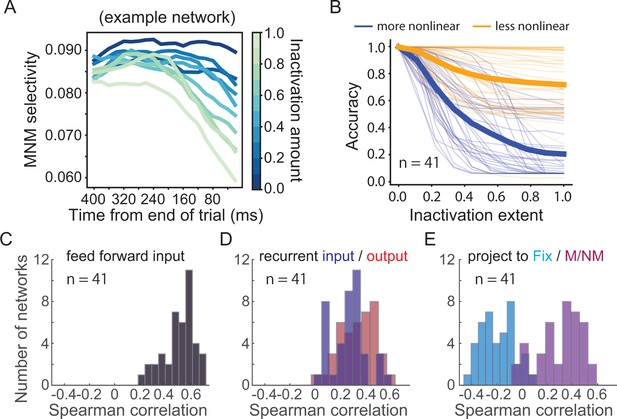
Circuit mechanisms underlying nonlinear integration of task variables to form matching/nonmatching (M/NM) decisions.
(A) The M/NM selectivity of units in an example lateral intraparietal (LIP) module is shown as a function of time after increasingly inactivating the feedback projection from prefrontal cortex (PFC) module during the test period. Different colors denote different inactivation levels. (B) The behavioral performance of the recurrent neural networks (RNNs) after gradually inactivating the more-nonlinear and less-nonlinear groups of units in the PFC module. Each thin line denotes the result from one RNN. The thick lines denote the averaged performance for all 41 RNNs. (C) The Spearman rank correlations between the feedforward input weights from the LIP modules and the nonlinear integrative index values of PFC module units. (D) The Spearman rank correlations between the recurrent connection weights of units within the PFC module and their nonlinear integrative index values. (E) The Spearman rank correlations between the output weights to different types of response units and the nonlinear integrative indexes of units in the PFC module. The M and NM units were responsible for reporting the match and nonmatch decisions, respectively.

Behavioral performance of recurrent neural networks (RNNs) after gradually inactivating the more-nonlinear and less-nonlinear groups of units in the prefrontal cortex (PFC) module.
The thick lines denote the averaged performance of all 41 RNNs. The units that directly projected to the response output units were not included in the inactivation experiment. Each thin line denotes the result from one RNN. The thick lines denote the averaged performance of all 41 RNNs.
Additional files
-
Supplementary file 1
Both monkeys’ delayed match to category (DMC) task accuracy during match and nonmatch trials.
- https://cdn.elifesciences.org/articles/58782/elife-58782-supp1-v1.docx
-
Transparent reporting form
- https://cdn.elifesciences.org/articles/58782/elife-58782-transrepform-v1.docx