Comprehension of computer code relies primarily on domain-general executive brain regions
Figures
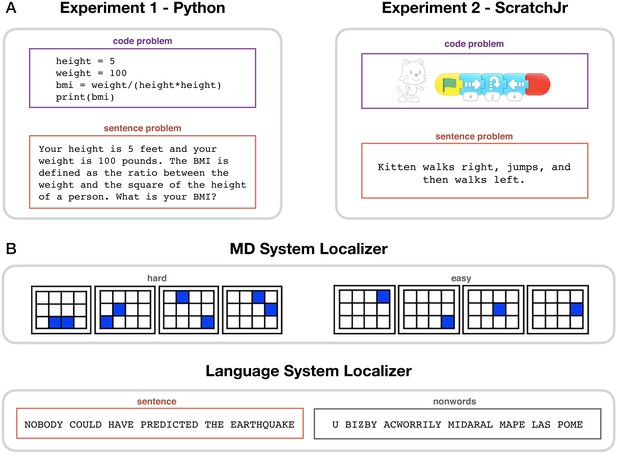
Experimental paradigms.
(A) Main task. During code problem trials, participants were presented with snippets of code in Python (Experiment 1) or ScratchJr (Experiment 2); during sentence problem trials, they were presented with text problems that were matched in content with the code stimuli. Each participant saw either the code or the sentence version of any given problem. (B) Localizer tasks. The MD localizer (top) included a hard condition (memorizing positions of eight squares appearing two at a time) and an easy condition (memorizing positions of four squares appearing one at a time). The language localizer (bottom) included a sentence reading and a nonword reading condition, with the words/nonwords appearing one at a time.
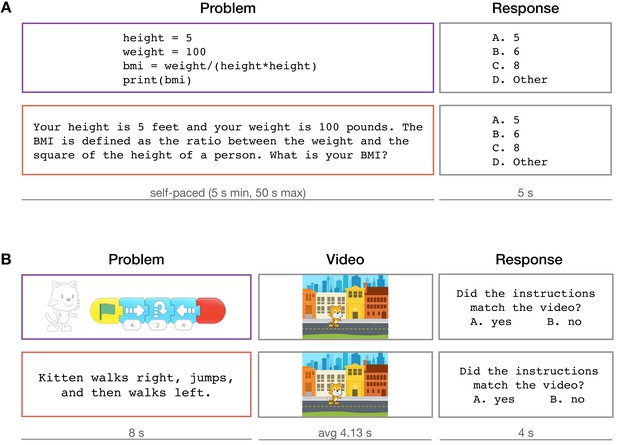
Trial structure of the critical task.
(A) Experiment 1 – Python. (B) Experiment 2 – ScratchJr. All analyses use functional magnetic resonance imaging responses to the ‘problem’ step.
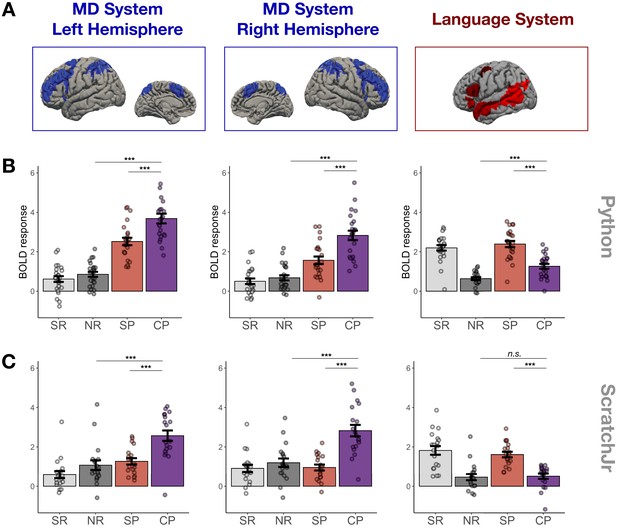
Main experimental results.
(A) Candidate brain systems of interest. The areas shown represent the ‘parcels’ used to define the MD and language systems in individual participants (see Materials and methods and Figure 3—figure supplement 1). (B, C) Mean responses to the language localizer conditions (SR – sentence reading and NR – nonwords reading) and to the critical task (SP – sentence problems and CP – code problems) in systems of interest across programming languages (B – Python, C – ScratchJr). In the MD system, we see strong responses to code problems in both hemispheres and to both programming languages; the fact that this response is stronger than the response to content-matched sentence problems suggests that it reflects activity evoked by code comprehension per se rather than just activity evoked by problem content. In the language system, responses to code problems elicit a response that is substantially weaker than that elicited by sentence problems; further, only in Experiment 1 do we observe responses to code problems that are reliably stronger than responses to the language localizer control condition (nonword reading). Here and elsewhere, error bars show standard error of the mean across participants, and the dots show responses of individual participants.
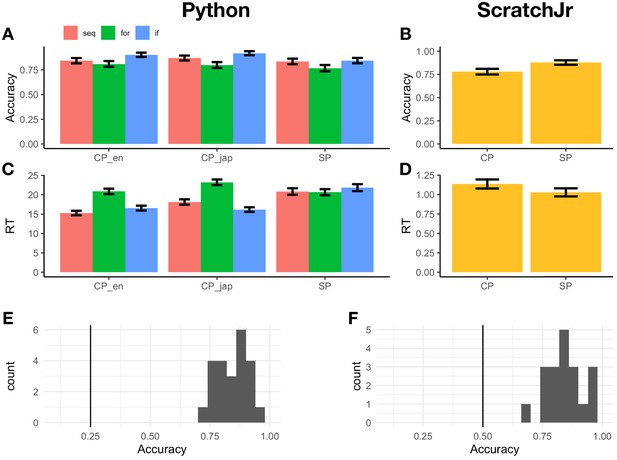
Behavioral results.
(A) Python code problems had mean accuracies of 85.1% and 86.2% for the English-identifier (CP_en) and Japanese-identifier (CP_jap) conditions, respectively, and sentence problems (SP) had a mean accuracy of 81.5%. There was no main effect of condition (CP_en, CP_jap, SP), problem structure (seq – sequential, for – for loops, if – if statements), or problem content (math vs. string); however, there was a three-way interaction among Condition (sentence problems > code with English identifiers), Problem Type (string >math), and Problem Structure (for loop >sequential; p=0.02). Accuracy data from one participant had to be excluded due to a bug in the script. (B) ScratchJr code problems had a mean accuracy of 78.0%, and sentence problems had a mean accuracy of 87.8% (the difference was significant: p=0.006). (C) Python problems with English identifiers had a mean response time (RT) of 17.56 s (SD = 9.05), Python problems with Japanese identifiers had a mean RT of 19.39 s (SD = 10.1), and sentence problems had a mean RT of 21.32 s (SD = 11.6). Problems with Japanese identifiers took longer to answer than problems with English identifiers (β = 3.10, p=0.002), and so did sentence problems (β = 6.12, p<0.001). There was also an interaction between Condition (sentence problems > code with English identifiers) and Program Structure (for >seq; β = −5.25, p<0.001), as well as between Condition (CP_jap > CP_en) and Program Structure (if >seq; β = −2.83, p=0.04). There was no significant difference in RTs between math and string manipulation problems. (D) ScratchJr code problems had a mean RT of 1.14 s (SD = 0.86), and sentence problems had a mean RT of 1.03 s (SD = 0.78); the difference was not significant. The RTs are reported with respect to video offset. Items where >50% participants chose the incorrect answer for the (easy) verbal condition were excluded from accuracy calculations. (E) Mean accuracies for all Python participants were above chance. (F) Mean accuracies for all ScratchJr participants were above chance.
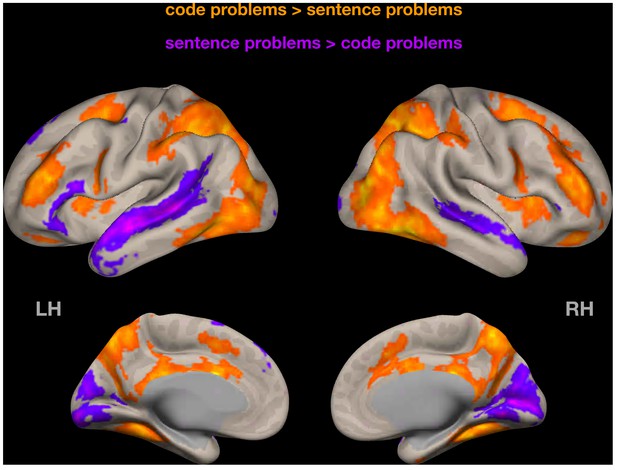
Random-effects group-level analysis of Experiment 1 data (Python, code problems > sentence problems contrast).
Similar to analyses reported in the main text, code-evoked activity is bilateral and recruits fronto-parietal but not temporal regions. Cluster threshold p<0.05, cluster-size FDR-corrected; voxel threshold: p<0.001, uncorrected.
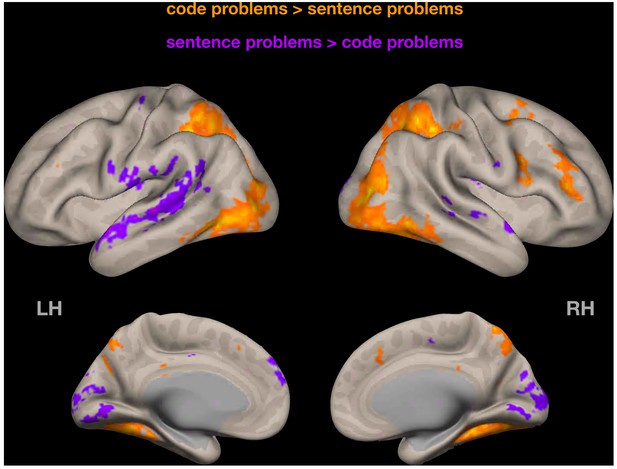
Random-effects group-level analysis of Experiment 2 data (ScratchJr, code problems > sentence problems contrast).
Similar to analyses reported in the main text, ScratchJr-evoked activity has a small right hemisphere bias. Cluster threshold p<0.05, cluster-size FDR-corrected; voxel threshold: p<0.001, uncorrected.
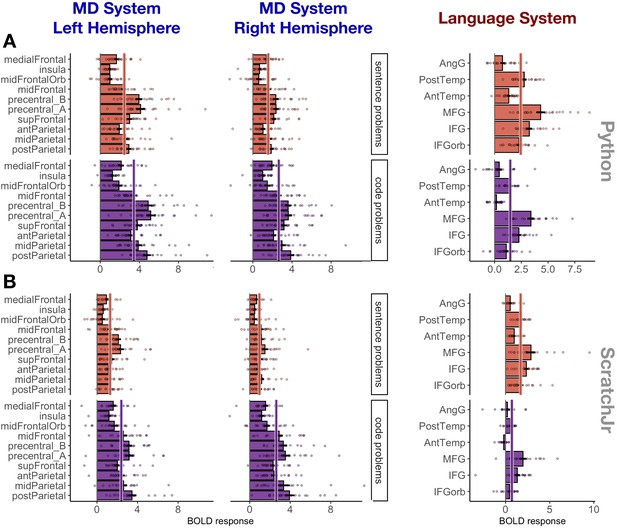
Responses to sentence problems (red) and code problems (purple) during Experiment 1 (Python; A) and Experiment 2 (ScratchJr; B) broken down by region within each system.
Abbreviations: mid – middle, ant – anterior, post – posterior, orb – orbital, MFG – middle frontal gyrus, IFG – inferior frontal gyrus, temp – temporal lobe, AngG – angular gyrus, precentral_A – the dorsal portion of precentral gyrus, precentral_B – the ventral portion of precentral gyrus. A solid line through the bars in each subplot indicates the mean response across the fROIs in that plot.
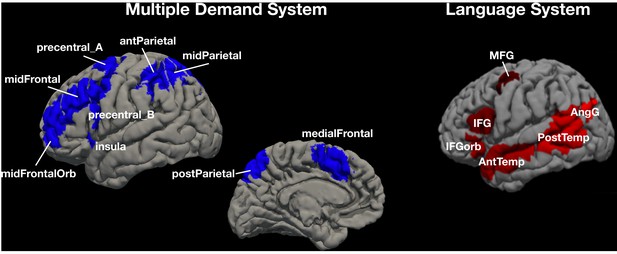
The parcels in the two candidate brain systems of interest, multiple demand (MD) and language.
The parcels are derived from group-level representations of MD and language activity and are used to define the functional regions of interest (fROIs) in individual participants (NB: we show the left hemisphere parcels for the MD system, but the system is bilateral). For each participant, the network of interest is comprised of the top 10% of voxels within each parcel with the highest t-value for the relevant contrast (MD – hard vs. easy spatial working memory task; language – sentence reading vs. nonword reading; see Materials and methods). Abbreviations: mid – middle, ant – anterior, post – posterior, orb – orbital, MFG – middle frontal gyrus, IFG – inferior frontal gyrus, temp – temporal lobe, AngG – angular gyrus.
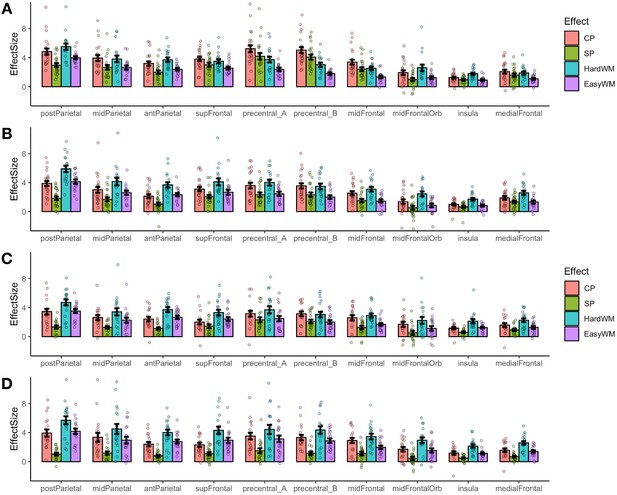
ROI-level responses in the multiple demand system to the critical task (CP – code problems, SP – sentence problems) and the spatial working memory task (HardWM – hard working memory task, EasyWM – easy working memory task).
(A) Experiment 1, Python; left hemisphere fROIs; (B) Experiment 1, Python; right hemisphere fROIs; (C) Experiment 2, ScratchJr; left hemisphere fROIs; (D) Experiment 2, ScratchJr; right hemisphere fROIs. No fROIs prefer both Python and ScratchJr code problems over the spatial working memory task.
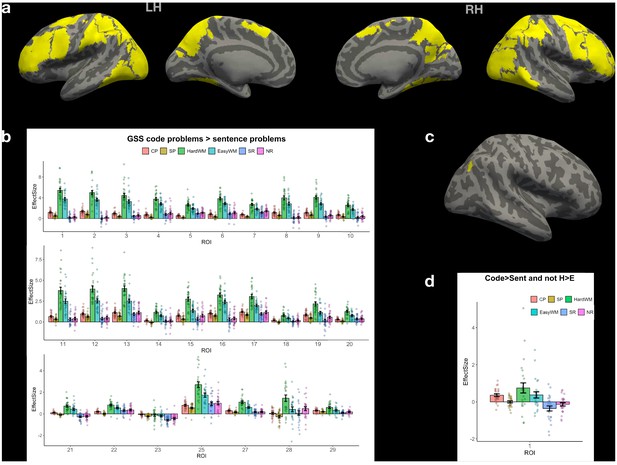
Whole-brain group-constrained subject-specific (GSS) analysis (Fedorenko et al., 2010) based on data from Experiment 1 shows the absence of code-only brain regions.
(a) Parcels defined at the group level using the code problems > sentence problems contrast, p threshold 0.001, inter-subject overlap ≥70%. (b) Activation profile for the top 10% of voxels within each parcel in (a) across conditions. All code-sensitive regions exhibit high activity during the spatial working-memory task, suggesting that they belong to the MD system. (c) Parcels defined using the contrast above plus the ‘not hard working-memory task >easy working-memory task’ contrast, p=0.5. Only one parcel was significant (right hemisphere). (d) Even that parcel’s response profile shows high activity in response to the working-memory task, modulated by difficulty, rather than a code-specific response. Abbreviations: CP – code problems; SP – sentence problems; HardWM – hard working memory task; EasyWM – easy working memory task; SR – sentence reading; NR – nonword reading.
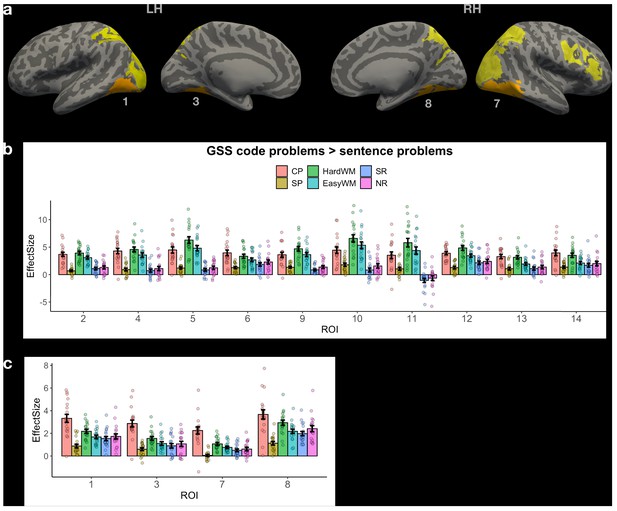
Whole-brain group-constrained subject-specific (GSS) analysis (Fedorenko et al., 2010) based on data from Experiment 2.
(a) Parcels defined at the group level using the code problems > sentence problems contrast, p threshold 0.001, inter-subject overlap ≥70%. Parcels where the responses to ScratchJr code were stronger than responses to all other tasks are labeled and marked in orange; they include parts of early visual cortex and parts of the ventral visual stream. (b) Activation profile for the top 10% of voxels within each parcel in (a) marked in yellow. All regions exhibit high activity during the spatial working-memory task, suggesting that they belong to the MD system. (c) Activation profile for the top 10% of voxels within each parcel in (a) marked in orange. These fROIs exhibit higher responses to ScratchJr problems compared to a working memory task; given that they are located in the visual cortex, we can infer that they respond to low-level visual properties of ScratchJr code. A follow-up conjunction analysis using the contrast in (a) plus the ‘not hard working-memory task >easy working-memory task’ contrast, p=0.5, revealed no significant parcels, indicating the lack of code-selective response. Abbreviations: CP – code problems; SP – sentence problems; HardWM – hard working memory task; EasyWM – easy working memory task; SR – sentence reading; NR – nonword reading.
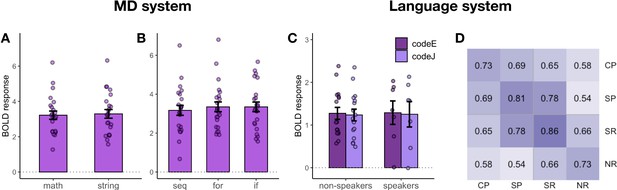
Follow-up analyses of responses to Python code problems.
(A) MD system responses to math problems vs. string manipulation problems. (B) MD system responses to code with different structure (sequential vs. for loops vs. if statements). (C) Language system responses to code problems with English identifiers (codeE) and code problems with Japanese identifiers (codeJ) in participants with no knowledge of Japanese (non-speakers) and some knowledge of Japanese (speakers) (see the ‘Language system responses...' section for details of this manipulation). (D) Spatial correlation analysis of voxel-wise responses within the language system during the main task (SP – sentence problems and CP – code problems) with the language localizer conditions (SR – sentence reading and NR – nonwords reading). Each cell shows a correlation between the activation patterns for each pair of conditions. Within-condition similarity is estimated by correlating activation patterns across independent runs.
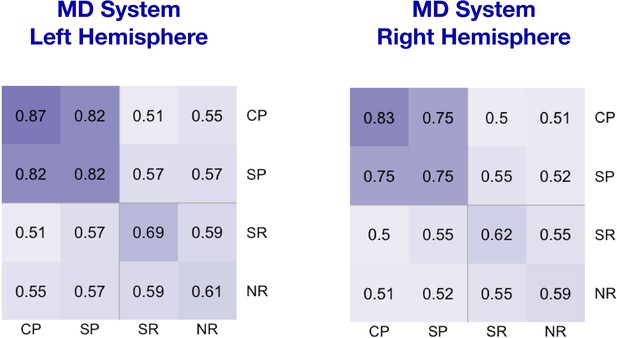
Spatial correlation analysis of voxel responses within the MD system during the Python experiment (CP – code problems and SP – sentence problems) with the language localizer conditions for the same participants (SR – sentence reading and NR – nonword reading).
Each cell shows a correlation between voxel-level activation patterns for each condition. Within-condition similarity is estimated by correlating activation patterns across independent runs. Code problems correlate with sentence problems much more strongly than with sentence reading (β = −0.59, p<0.001) and with nonword reading (β = −0.55, p<0.001), but substantially weaker than with other code problems (β = 0.11, p<0.001). There was no main effect of hemisphere, but there was an interaction between some of the conditions and hemisphere (sentence reading: β = 0.17, p<0.001, nonword reading: β = 0.13, p=0.002), indicating that the correlation patterns of code/sentence problems were somewhat less robust in the right hemisphere.
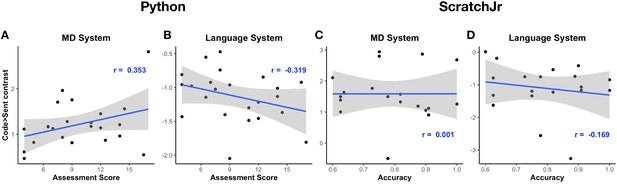
The effect of programming expertise on code-specific response strength within the MD and language system in Experiment 1, Python (A, B) and Experiment 2, ScratchJr (C, D).
Python expertise was evaluated with a separate 1-hr-long Python assessment (see the paper’s website, https://github.com/ALFA-group/neural-program-comprehension); ScratchJr expertise was estimated with in-scanner response accuracies. No correlations were significant.
Additional files
-
Supplementary file 1
Statistical analysis of functional ROIs in the multiple demand system.
Table 1 – Experiment 1 (Python); Table 2 – Experiment 2 (ScratchJr).
- https://cdn.elifesciences.org/articles/58906/elife-58906-supp1-v1.docx
-
Transparent reporting form
- https://cdn.elifesciences.org/articles/58906/elife-58906-transrepform-v1.pdf