Fibroblast mechanotransduction network predicts targets for mechano-adaptive infarct therapies
Figures
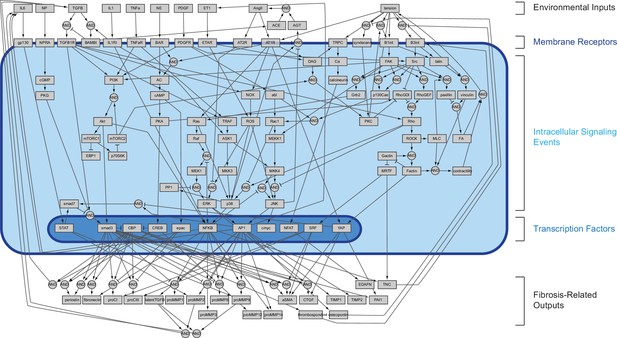
Schematic of expanded logic-based ordinary differential equation model of cardiac fibroblast chemo/mechanotransduction.
Extracellular stimuli, intracellular signaling species, transcription factors, and fibroblast-secreted outputs are represented as nodes (boxes, 109 total). Directed edges represent activating and inhibiting reactions (arrows and T junctions respectively, 174 total) with AND logic indicated by circular nodes.
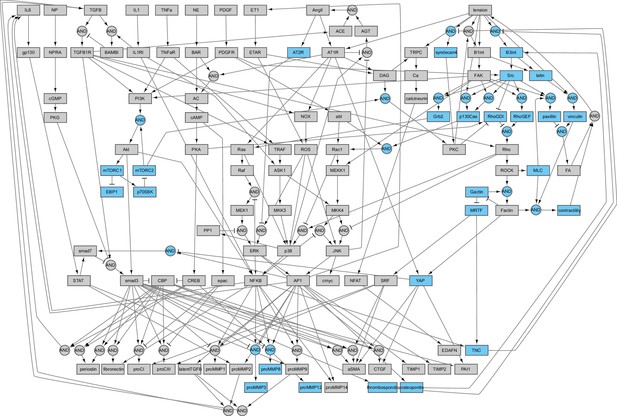
Schematic of fibroblast chemo-mechanotransduction network modifications for current study.
Added nodes relating to mechanotransduction mechanisms, mechano-chemo-crosstalk mechanisms, and additional secreted outputs are highlighted in blue.
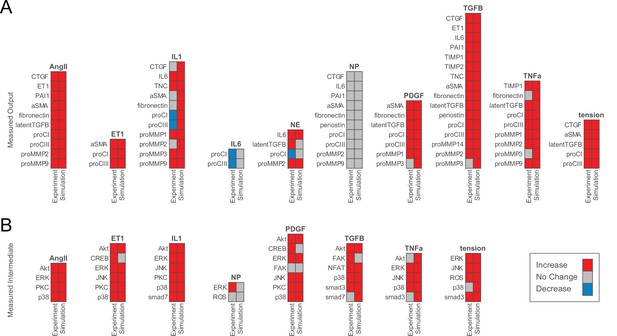
Model accurately predicts qualitative changes in output expression and intermediate activity as measured by independent in vitro studies.
(A) Expression of cell-secreted outputs and phenotypic markers (i.e. αSMA) were predicted in response to single biochemical stimuli and mechanical tension and compared to an independent set of experimental studies found in literature (47 papers total). (B) Activation of intracellular intermediates representing major signaling pathways was predicted in response to single stimuli and compared to independent experimental studies measuring changes in activity (e.g. by phosphorylation, 27 papers total). All model predictions were categorized based on a ±5% change in activity levels compared to baseline conditions.
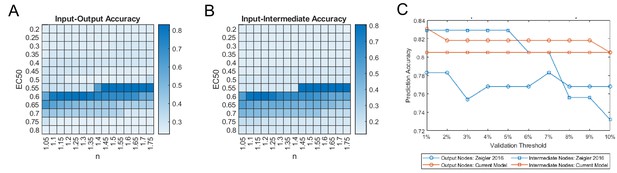
Optimization of reaction parameters for maximizing accuracy of qualitative predictions.
Parameter sweeps of half-maximal effective concentration (EC50) and Hill coefficients (n) were conducted by simulating changes in output node expression (A) and intermediate node activity (B) in response to single input stimuli. Shading of parameter combinations represents the total proportion of correct qualitative predictions compared to each independent experimental validation set used in Figure 2. The validation accuracy was also tested against the threshold used for defining a model-predicted response or non-response (C).
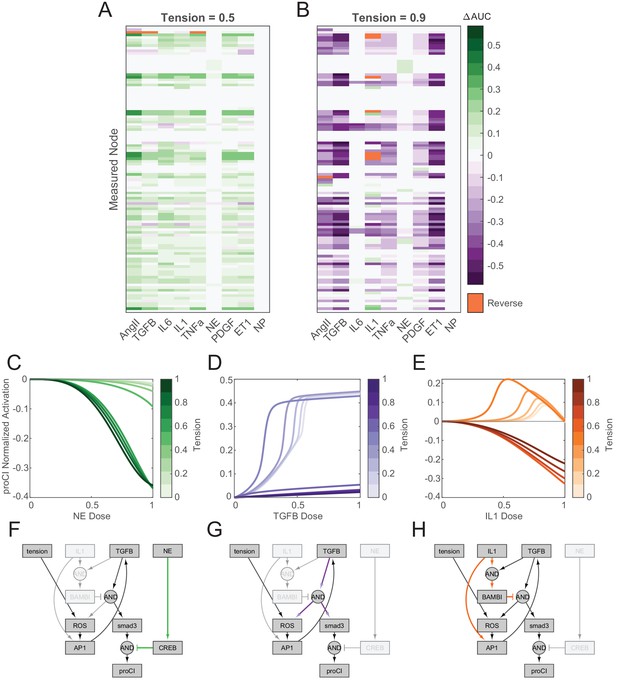
Network-wide responses to biochemical stimuli are dependent on the tensional context.
(A–B) Dose-response curves were simulated under elevated levels of tension with incremental doses of each biochemical input, and changes in area under normalized dose-response curves (ΔAUC) were calculated for all nodes in the model in comparison to basal dose-response simulations (i.e. tension = 0.1). Reversal cases were identified based on opposing AUC signs between basal and elevated tension simulations, that is, nodes that exhibited a positive AUC with basal tension and a negative AUC with elevated tension, and vice versa. (C–E) Examples of mechano-chemo interaction categories identified from network-wide analysis in (A) and (B). Normalized dose-response curves for procollagen I (proCI) expression are shown following simulations with incremental doses of norepinephrine (NE) (A), transforming growth factor-β (TGFβ1) (B), and interleukin-1β (IL1) (C) under static levels of tension. (F–H) Sub-networks regulating the mechano-chemo interactions shown in (C–E) were determined by manual examination of node activity levels following dose-response simulations using NE (F), TGFβ1 (G), and IL1 (H) stimuli under basal and elevated levels of tension.
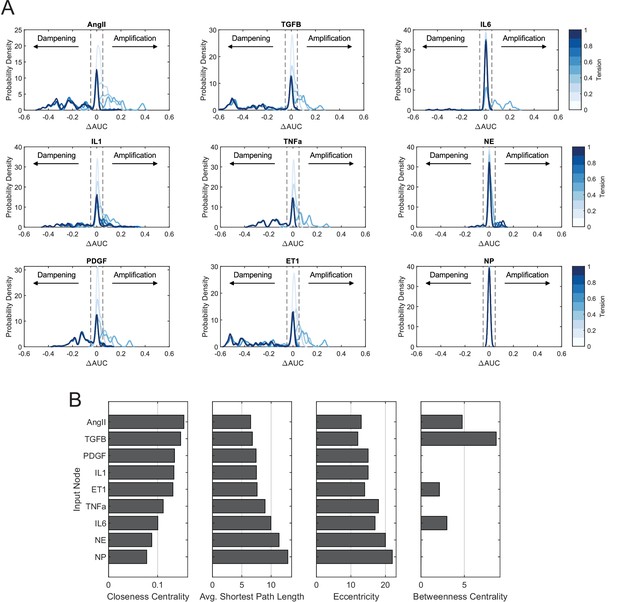
Comparison of mechano-chemo interaction trends across individual biochemical stimuli.
(A) Probability density distributions of changes in area under normalized dose-response curves (ΔAUC) with incremental levels of tension. Distributions were estimated from data using a kernel density smoothing function with a bandwidth of 0.01, and dashed lines represent a ±5% threshold used to identify nodes that exhibited amplified or dampened responses toward biochemical stimuli with added tension. (B) Comparison of topological measures of centrality for biochemical inputs. Topological analysis of the full network was conducted using the NetworkAnalyzer plugin for Cytoscape.
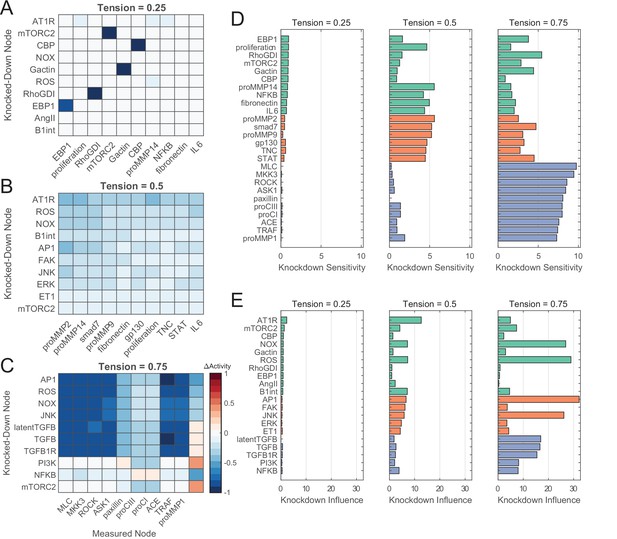
Network perturbation analysis reveals influential pathways of fibroblast mechanotransduction.
(A–C) Changes in activity levels for top 10 sensitive nodes with simulated knockdown of top 10 influential nodes at low (A), medium (B), and high (C) levels of tension. Nodes were ranked based on knockdown influence (rows) and knockdown sensitivity metrics (columns), and changes in activity levels with perturbations are compared un-perturbed (i.e. no knockdown) conditions at each tension level. (D–E) Comparison of top-ranked sensitive and influential nodes between levels of tension. Nodes shown reflect all unique nodes that ranked in the top 10 based on knockdown sensitivity (D) or knockdown influence (E) between all levels of tension simulated, and colors reflect nodes that were top-ranked starting at low tension (green), medium tension (orange), and high tension (purple).
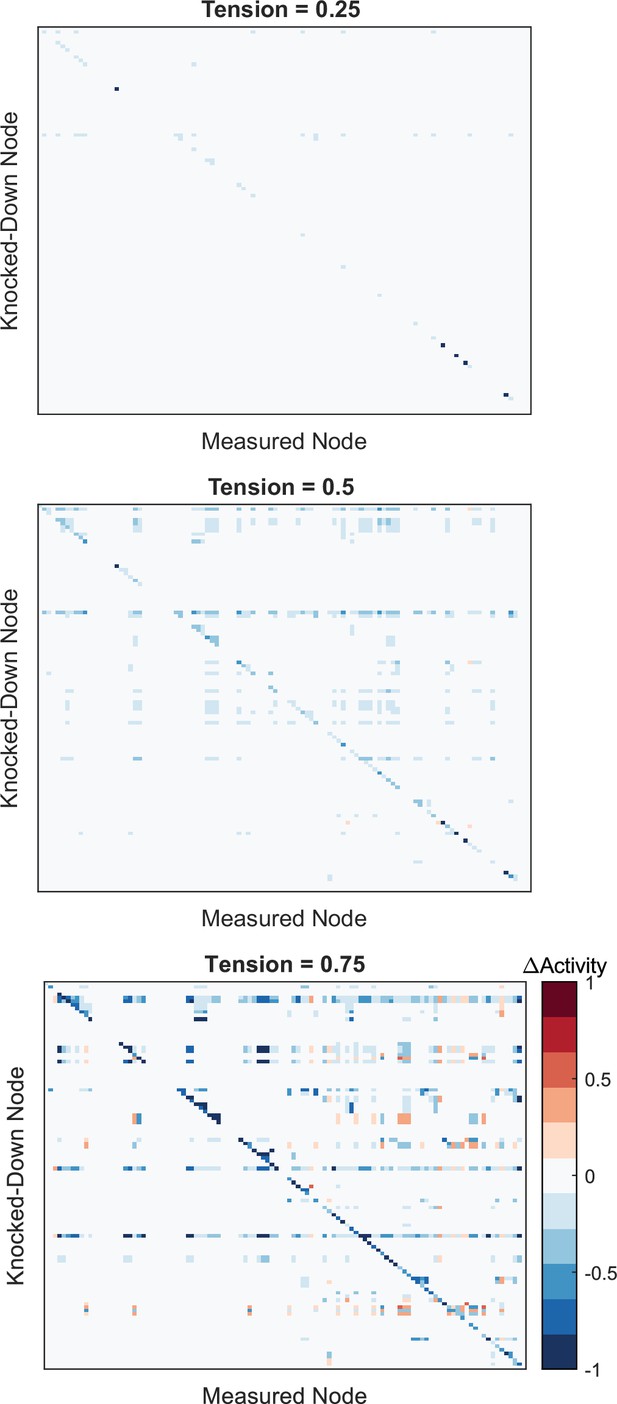
Full network perturbation analysis results.
Changes in activity for all nodes in the network were measured following comprehensive knockdown of individual noes (Ymax = 0.1) under given levels of tension. Values reflect changes in node activity between perturbed and un-perturbed conditions at each tension level. Refer to Supplementary file 1 for the order of nodes perturbed/measured.
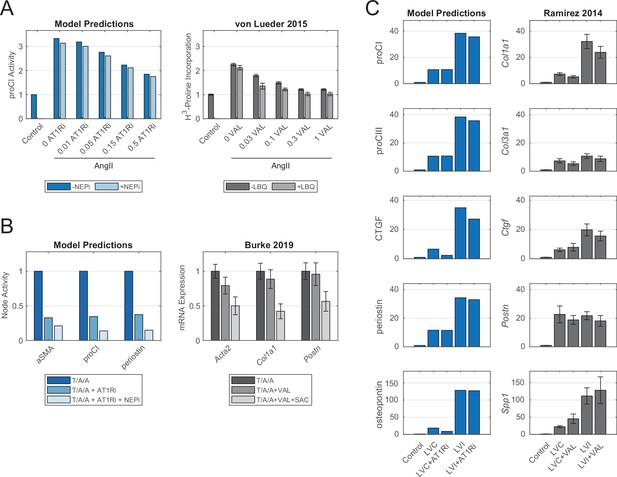
Model-predicted effects of current post-myocardial infarction (MI) drug treatments reflect experimental evidence from independent case studies.
(A) Comparison of model-predicted effect of angiotensin receptor blocker (ARB) doses with or without neprilysin inhibitor (NEPi) treatment on procollagen I expression with measurements of collagen synthesis in cardiac fibroblasts in vitro. Doses for model predictions reflect negative modifiers of the Ymax parameter of AT1R, doses for the in vitro study reflect doses of valsartan in μM, and values reflect fold changes compared to control conditions. (B) Comparison of model-predicted effects of ARB and ARB-NEPi treatments on three output nodes with corresponding gene expression measurements of cardiac fibroblasts in vitro. Values reflect fold changes compared to TGFβ1/AngII/atrial NP (T/A/A) conditions. (C) Comparison of model-predicted effects of ARB treatment on five output nodes in representative remote (LVC) and infarct (LVI) zones with corresponding gene expression measurements in post-MI mouse hearts. Values reflect fold changes compared to control (non-infarcted) conditions. All experimental data are represented as mean ± SEM.
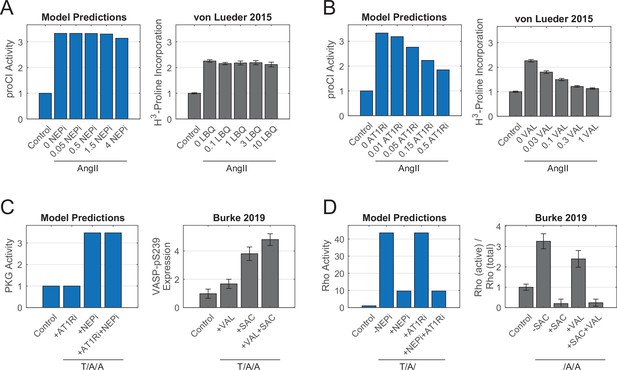
Comparisons of model-predicted and experimentally measured changes in procollagen expression and signaling intermediate activity.
(A) Simulated and experimental measurements of procollagen I synthesis in response to increasing doses of neprilysin inhibitor (NEPi) LBQ657 (LBQ). Simulated concentrations of NEPi represent positive modifiers added to Ymax parameters, and experimental concentrations represent concentrations of LBQ657 in µM. (B) Simulated and experimental measurements of procollagen I synthesis in response to increasing doses of angiotensin receptor blocker valsartan (VAL). Simulated concentrations represent negative modifiers subtracted from Ymax parameters, and experimental concentrations represent concentrations of valsartan in µM. (C) Simulated and experimental measurements of PKG activity in response to valsartan and/or NEPi sacubitril (SAC). Experimental values reflect phosphorylation of PKG analog VASP (vasodilator-stimulated phosphoprotein). (D) Simulated and experimental measurements of Rho activity in response to valsartan and/or sacubitril. All experimental data represent mean levels ± SEM.
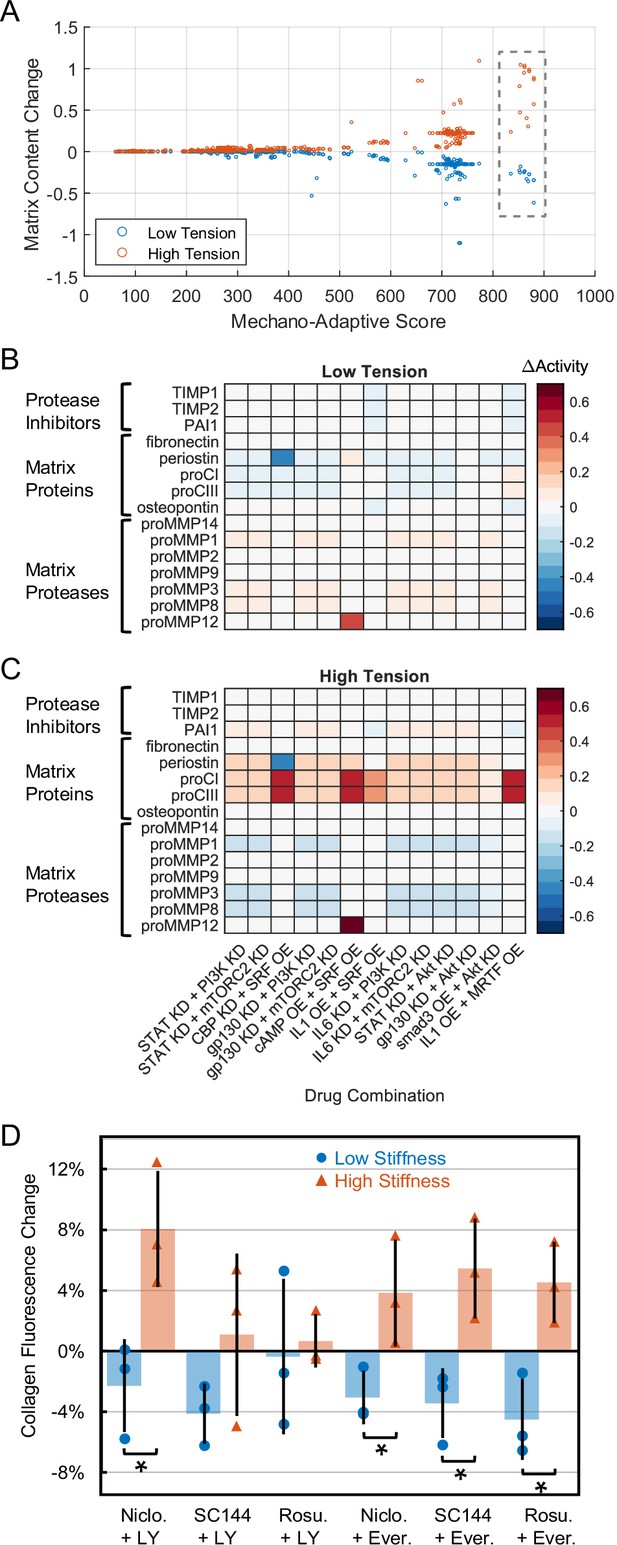
Comprehensive drug screen identifies mechano-adaptive candidates for post-myocardial infarction (MI) fibrosis.
(A) Target combinations meeting preliminary threshold for mechano-adaptive behavior (i.e. promoted negative change in matrix content in low tension and positive change in matrix content in high tension) were scored based on rankings of matrix content changes in low- and high-tension simulations. Boxed values represent scores of 13 candidates examined in panels (B) and (C). (B–C) Changes in output expression predicted with mechano-adaptive candidates under tensional contexts, expressed as changes in node activity compared to non-perturbed conditions for each level of tension. Bracketed groups in panels (B/C) represent categories used for calculations of matrix content in (A). (D) Follow-up in vitro experiments treated human cardiac fibroblasts with pharmacological compound combinations that inhibited the model-identified targets. Four of six combinations successfully decreased collagen content under low mechanical stimulation (8 kPa stiffness) and increased collagen under high mechanical stimulation (64 kPa stiffness). * indicates p<0.05 using Student's t-tests with Bonferroni multiple comparison adjustments.
-
Figure 6—source data 1
In vitro validation experimental results.
- https://cdn.elifesciences.org/articles/62856/elife-62856-fig6-data1-v2.xlsx
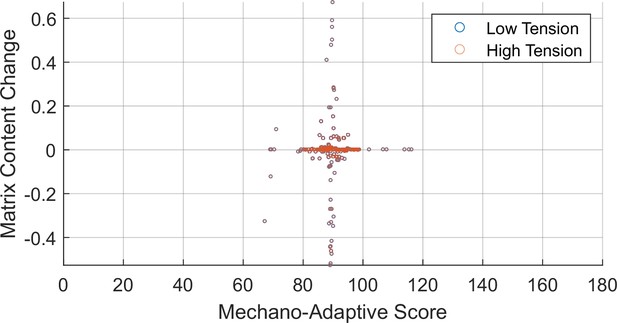
Screen of individual drug targets for mechano-adaptive matrix expression.
Changes in matrix content as a function of output node expression were measured following comprehensive knockdown and overexpression of individual nodes in low- or high-tension contexts. Perturbations were scored for mechano-adaptive behavior based on matrix content change values. Overlapping low- and high-tension values indicate perturbations that equality altered matrix content regardless of the tensional context.
Tables
Statistical tests of tension dependencies.
Results of Kolmogorov-Smirnov (K-S) tests with Benjamini-Hochberg correction comparing changes in area under normalized dose-response curves (ΔAUC) distributions for inputs across levels of tension.
Input | Tension levels | K-S test p-value | Tension levels | K-S test p-value | Tension levels | K-S test p-value |
---|---|---|---|---|---|---|
AngII | 0.2 vs. 0.5 | 2.2e-17 | 0.2 vs. 0.9 | 2.37e-37 | 0.5 vs. 0.9 | 1.00e-32 |
TGFβ | 0.2 vs. 0.5 | 1.88e-13 | 0.2 vs. 0.9 | 6.10e-32 | 0.5 vs. 0.9 | 5.20e-31 |
IL6 | 0.2 vs. 0.5 | 1.87e-28 | 0.2 vs. 0.9 | 9.56e-6 | 0.5 vs. 0.9 | 9.90e-23 |
IL1 | 0.2 vs. 0.5 | 5.38e-21 | 0.2 vs. 0.9 | 5.88e-16 | 0.5 vs. 0.9 | 5.88e-16 |
TNFα | 0.2 vs. 0.5 | 3.17e-21 | 0.2 vs. 0.9 | 1.55e-39 | 0.5 vs. 0.9 | 1.55e-39 |
NE | 0.2 vs. 0.5 | 1.07e-22 | 0.2 vs. 0.9 | 1.19e-3 | 0.5 vs. 0.9 | 7.47e-13 |
PDGF | 0.2 vs. 0.5 | 5.38e-21 | 0.2 vs. 0.9 | 6.10e-32 | 0.5 vs. 0.9 | 6.10e-32 |
ET1 | 0.2 vs. 0.5 | 1.15e-15 | 0.2 vs. 0.9 | 8.30e-34 | 0.5 vs. 0.9 | 1.70e-32 |
NP | 0.2 vs. 0.5 | 1.18e-17 | 0.2 vs. 0.9 | 2.02e-12 | 0.5 vs. 0.9 | 6.97e-4 |
Model parameters used for drug effect simulations.
Minimum/maximum AT1R inhibitor (AT1Ri) values represent modifiers subtracted from the Ymax parameter of the AT1R node, and minimum/maximum neprilysin inhibitor (NEPi) values represent modifiers added to the Ymax parameter of the NP node. Non-specified input levels were set to a default value of 0.1. Int.: interpolated from measurements of post-MI cytokine levels in vivo.
Study | TGFβ (w) | AngII (w) | NP (w) | Tension (w) | AT1Ri minimum | AT1Ri maximum | NEPi minimum | NEPi maximum |
---|---|---|---|---|---|---|---|---|
von Lueder et al., 2015 | – | 0.4 | – | 0.3 | 0.01 | 0.5 | 0.05 | 4 |
Burke et al., 2019 | 0.4 | 0.4 | 0.6 | 0.3 | 0.5 | 0.5 | 4 | 4 |
Ramirez et al., 2014 | Int. | Int. | Int. | 0.1/0.6 | 0.5 | 0.5 | – | – |
Experimental group culture media conditions.
Control | Media (0.5% FBS)+ TGFβ (10 ng/mL)+ TNFα (10 ng/mL)+ L-ascorbic acid (50 ng/mL) |
---|---|
PI3K + STAT inhibitors | Control + LY294002 (10 μM)+ Niclosamide (0.5 μM) |
PI3K + gp130 inhibitors | Control + LY294002 (10 μM)+ SC144 (10 μM) |
PI3K + IL6 inhibitors | Control + LY294002 (10 μM)+ Rosuvastatin (10 μM) |
mTOR + STAT inhibitors | Control + Everolimus (0.5 μM)+ Niclosamide (0.5 μM) |
mTOR + gp130 inhibitors | Control + Everolimus (0.5 μM)+ SC144 (10 μM) |
mTOR+ IL6 inhibitors | Control + Everolimus (0.5 μM)+ Rosuvastatin (10 μM) |
Additional files
-
Supplementary file 1
Fibroblast mechano-chemo signal transduction model.
Database detailing all nodes, reactions, model parameters, and references used for construction of the signaling network.
- https://cdn.elifesciences.org/articles/62856/elife-62856-supp1-v2.xlsx
-
Supplementary file 2
Model validation database.
Database detailing all qualitative input-output and input-intermediate relationships and references used for validation of the signaling network with independent experimental studies.
- https://cdn.elifesciences.org/articles/62856/elife-62856-supp2-v2.xlsx
-
Transparent reporting form
- https://cdn.elifesciences.org/articles/62856/elife-62856-transrepform1-v2.docx