The brain in motion: How ensemble fluidity drives memory-updating and flexibility
Figures
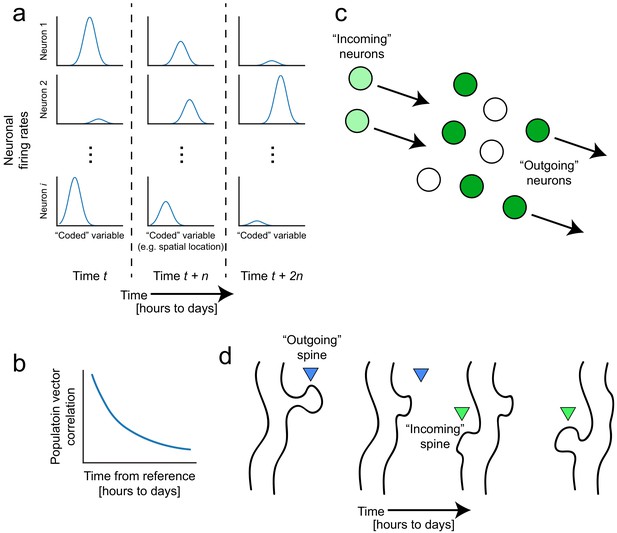
Representational drift and intrinsic dynamics supply neural substrates for memory-updating over time.
(a) Example tuning curves of a neuronal population changing its firing patterns over time with respect to an arbitrary external variable (e.g. spatial location) (Mau et al., 2018; Ziv et al., 2013). Each column corresponds to a certain point in time. Some neurons will lose their field while others gain one. This occurs even when the animal is performing stereotyped behavior (Chambers and Rumpel, 2017; Rokni et al., 2007; Rule et al., 2019). (b) Schematic of population similarity over time. As a result of (a), the similarity of population activity to time t decreases over time (Mankin et al., 2012; Mau et al., 2018; Ziv et al., 2013). (c) Schematic of fluidity in ensembles. Intrinsic fluctuations that result in increased excitability in certain neurons (light green circles) bring them up to par with the currently active ensemble (dark green circles) relative to non-active ensembles (white circles). These ‘incoming’ neurons become more likely to encode future memories (Rogerson et al., 2014). At the same time, other ‘outgoing’ neurons lose their association with the network or their synapses are pruned to make room for the incoming cells. (d) Schematic of dynamic synapses. Even in the absence of neuronal activity, synapses are known to be continuously formed and eliminated (Minerbi et al., 2009; Yasumatsu et al., 2008), meaning this is an intrinsic process that occurs regardless of input. Perhaps the underlying source of drift at the population level is intrinsic synaptic volatility (Holtmaat and Svoboda, 2009; Ziv and Brenner, 2018).
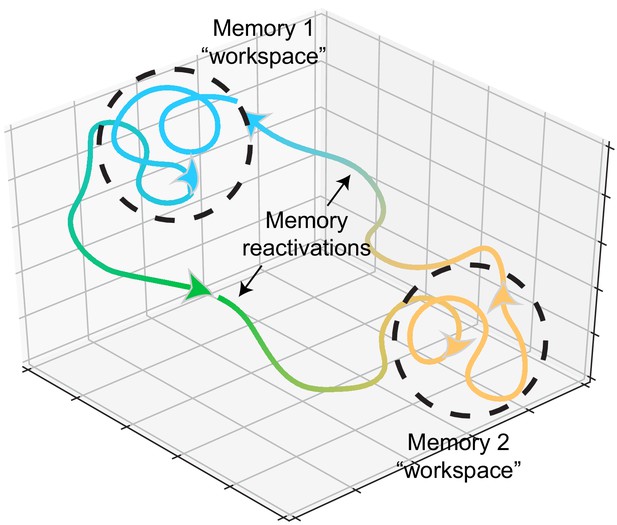
Memory representations occupy regions of state space during experience and learning.
Example trajectories of network states during recollection of two memories, depicted on a neural subspace. The network state is expressed in the firing activity of large neuronal populations. During situations where the environment or context is relatively stationary, the network state exhibits slow drift that constrains learning and plasticity locally (dotted black circles). Upon a major contextual shift, the network state responds with a fast, commensurate shift to a new regime (from Memory 1 to Memory 2, green trajectory) that recalls another memory. The network resides there until another contextual shift kicks it back to Memory 1. In experimental conditions, these contextual shifts are usually experimenter-defined (e.g., placing animals in different enclosures). However, in the wild, they may be shaped by major changes in the animal’s surroundings. In humans, and probably in non-human animals as well, contextual shifts may be internally motivated (i.e., spontaneous recall). Compartmentalization in network state space ensures that learning does not corrupt existing memories while allowing mechanisms for memory modification within local state space regions.
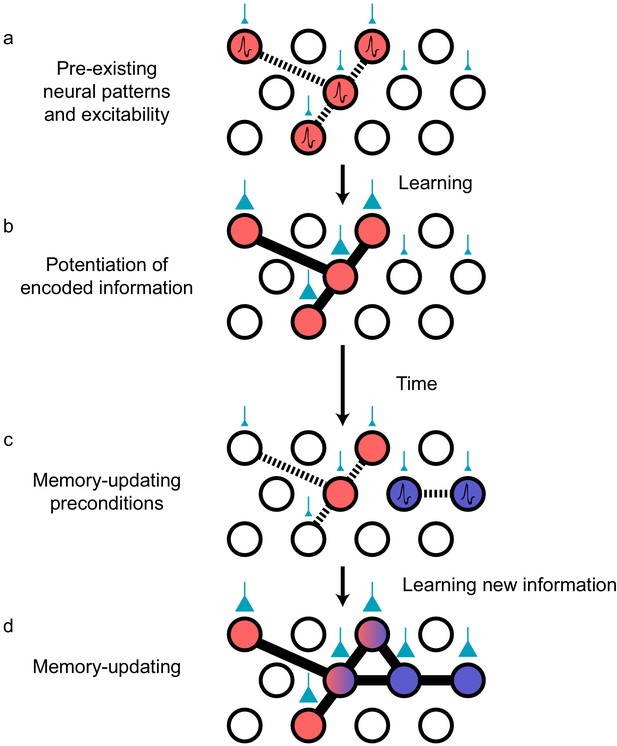
Preconfigured synaptic weights and excitability bias the allocation of memories to certain neuronal populations.
(a) Prior to an encoding event, some neurons will happen to be at the higher end of a distribution of excitability (red circles). These cells may be synaptically connected with each other, or receive shared input from upstream regions (e.g., CA1 receiving input from CA3; cyan boutons with bouton size representing synaptic weight) that result in high functional connectivity (dashed lines). Other cells may also be receiving input but have low excitability (empty circles). (b) During learning, these highly excitable cells increase their functional connectivity (bold lines connecting red circles) through synaptic plasticity. This could be achieved through potentiation of direct synaptic connections in the case of recurrent brain regions (e.g., CA3), or through potentiation of synapses in upstream regions that achieve appropriately timed co-activation of downstream (e.g., CA1) ensembles. (c) Over time, synaptic weights may weaken but some may persist to allow partial reactivation of a prior ensemble during memory recall (red circles). At the same time, just as in (a), a new population of cells may exhibit above-average excitability and functional connectivity (blue circles and dashed lines) at the time of a second learning episode. (d) Learning may potentiate functional connections between the red and blue ensembles to link the two memories.