Unsupervised learning of haptic material properties
Figures
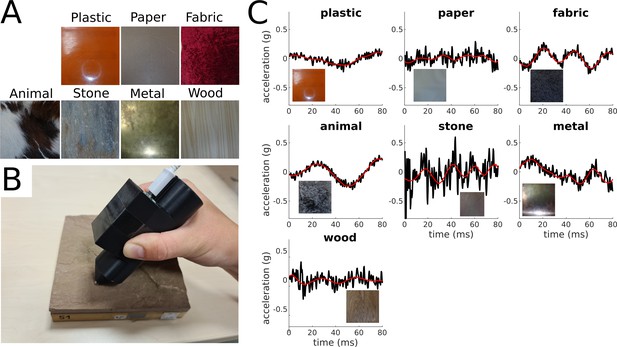
Materials and vibratory signals.
(A) Examples of materials. One example per category is shown. (B) Accelerometer mounted on a 3D-printed pen with a steel tip used for acquisition of vibratory signals. (C) Original and reconstructed acceleration traces for one example material per category. The black lines represent the original amplitude signals (y-axis) over time (x-axis), and the red line represents the corresponding reconstructed signals.
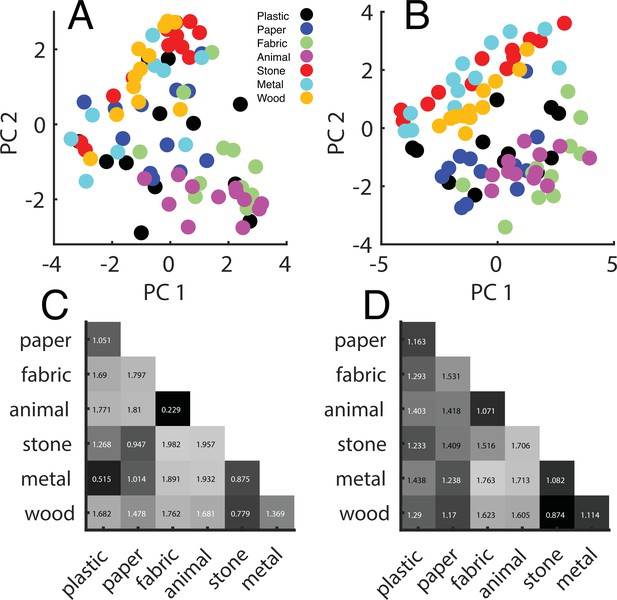
Perceptual ratings.
(A) Perceptual representation within the first (x-axis) and the second (y-axis) principal components space. Principal components analysis (PCA) was performed on the z-transformed ratings pooled over participants. Each point represents one material, and different colors represent different categories. (B) Same representation as in (A) based on barehand explorations (adapted from Figure 5 of Baumgartner et al., 2013). (C) Distance matrix based on the ratings from our experiment and (D) based on Baumgartner et al., 2013 data.
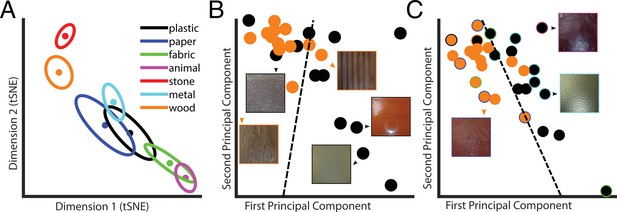
Latent space representation of the Autoencoder.
(A) Centers of categories in the t-distributed stochastic neighbor embedding (t-SNE). Each color represents a different category, as indicated in the legend. Dots represent material categories averaged across material samples, with the two-dimensional errors represented by error ellipses (axes length given by 1/2 of the eigenvalues of the covariance matrix; axes directions correspond to the eigenvectors). (B) Example of material category classification in the two first principal components of the latent PCs space, based on the ground truth material category labels. The black data points are the 2D representation of plastic; wood is in orange. The icons represent examples of material samples. The dashed line is the classification line learned by a linear classifier, which could tell apart the two categories with 75% accuracy. (C) Same as in (B), but based on perceptual labels. Black and orange data points indicate the perceptual categories plastic and wood, respectively. The data points surrounded by a colored rim represent material samples that were perceived as wood or plastic but belong to a different ground truth category (indicated by the color of the rim with the same color code as in A). The dashed line is the classification line learned by a linear classifier, which could tell apart the two categories with 87% accuracy. Note that the classification results reported in the main text are based on 10 principal components.
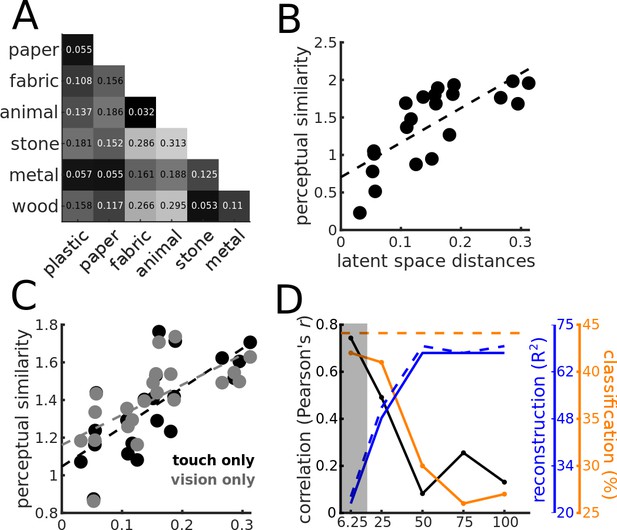
Similarity between the perceptual and the latent representation of the Autoencoder.
(A) Distance matrix based on the distances between the category centers in the (10D) latent PCs space. (B) Relationship between perceptual distances (y-axis) and distances between categories in the latent PCs space (x-axis). The black dashed line indicates the linear regression line. (C) Same as (B), but based on the rating experiments from Baumgartner et al., 2013, for the touch-only (black data points) and vision-only (gray data points) conditions. Again, the dashed lines represent regression lines, and in black and gray for the touch-only and the vision-only conditions, respectively. (D) Effect of compression. Correlation between distances in the latent PCs space and perceptual distances (black y-axis), reconstruction performance (blue y-axis), and classification performance (orange y-axis), as a function of compression rate (x-axis). Black line and data points indicate Pearson’s r correlation coefficient, and orange line and data points indicate classification accuracy (%). The blue lines represent the reconstruction performance computed as R2 and expressed in percentage of explained variance; the dashed blue line represents the reconstruction performance for the training data set, and the continuous line for the validation data set. The horizontal orange dashed line represents the between-participants agreement expressed as classification accuracy. The gray shaded area indicates the compression level corresponding to the Autoencoder used for the analyses depicted in Figure 3 and Figure 4A–C.
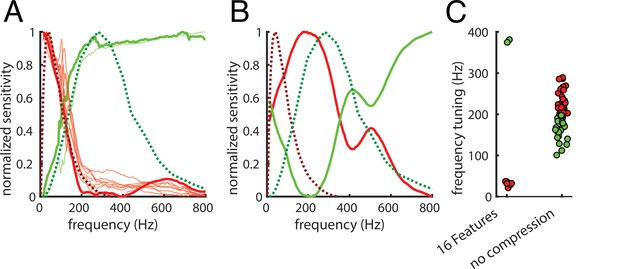
Frequency tuning.
(A) Comparison between temporal tuning of the dimensions of the 10D latent PCs space and the temporal tuning of the Pacinian (PC) and rapidly adapting (RA) afferents (green and red dashed lines, respectively). Tuning is given by sensitivity (y-axis) as a function of temporal frequency (x-axis). RA is optimally tuned at 40–60 Hz, and the PC is optimally tuned at 250–300 Hz. The first two principal components of the latent PCs space are represented by thick lines; thinner lines represent the other components. For a group of components (in red), sensitivity peaks around the RA afferents peak, the other group (in green) reaches the maximum sensitivity in proximity of the PC peak. (B) same as (A), but for the Autoencoder with no compression (100% compression rate). Here, only the first two principal components of the latent PCs space are shown as the tuning profiles tend to differ from each other. (C) Frequency tuning for the 16 features (6.25% compression rate) and the no compression (100% compression rate) Autoencoder. Tuning is again defined as average frequency weighted by sensitivity, and colors are assigned by the k-means clustering algorithm.