Evidence accumulation, not ‘self-control’, explains dorsolateral prefrontal activation during normative choice
Figures
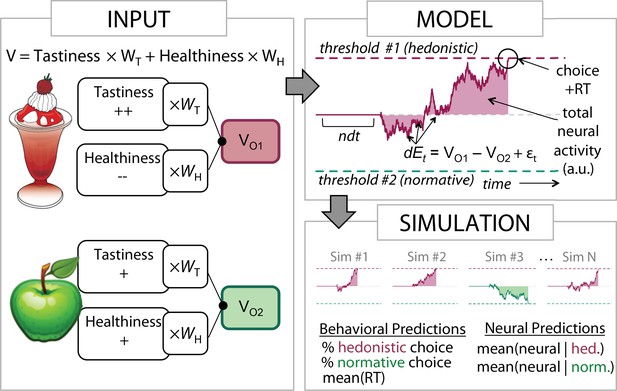
Attribute-based drift diffusion model (DDM) of normative choice.
Input. Each option’s hedonic and normative attributes (e.g., high tastiness and low healthiness for the sundae) are weighted by their current importance (e.g., wTaste [wT] and wHealth [wH]) and summed to construct relative option values [VO1 – VO2]. Model. In the computational model (upper right panel), these values, corrupted by momentary noise at time t [εt], serve as the inputs to an evidence accumulation process (Et) representing the two options (Sunday vs. apple). This evidence accumulates over time, moving by an increment dE at each moment, until it hits one of two predefined thresholds, determining the simulated response time (RT), the simulated choice, and the simulated neural activity (the sum of the absolute value of the evidence from t=0 to t=RT). Choices are classified as normative if the option with higher normative attribute value is selected (in this example, higher healthiness, i.e., the apple in option 2). Simulation. Simulating this process thousands of times (lower right panel) allows distributions to be constructed for the expected frequency of hedonistic and normative choices, RTs, and average neural activity, mean(neural), for each type of choice.
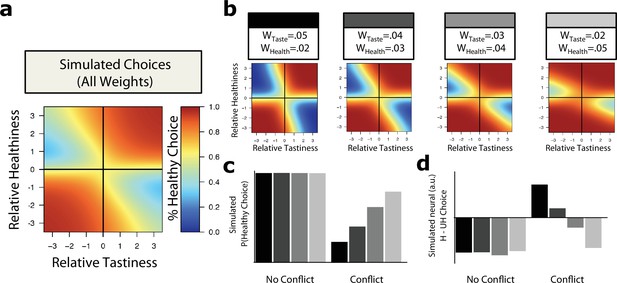
Simulating the dilemma of self-control.
An evidence accumulation model (drift diffusion model [DDM]) can be used to simulate decision making for any self-control context requiring an integration of normative and hedonistic considerations (dietary choices displayed, integrating relative tastiness and healthiness). Heat maps display simulated healthy/unhealthy choices for binary choices consisting of a proposed option vs. a default option. Both options are characterized by attribute values such as the perceived tastiness and healthiness of the two food options, with plot axes capturing the relative value difference in tastiness (x-axis) and healthiness (y-axis) of the proposal vs. the default. For example, for each heat map, the upper right-hand quadrant shows likelihood of a healthy choice when the proposed option was both tastier and healthier than the default. The upper left-hand quadrant illustrates the likelihood of a healthy choice when proposed foods were healthier but less tasty than the default and so forth. Warmer colors indicate a higher likelihood of choosing the healthier option. (a) On average across multiple different goals (i.e., different weights on different attributes), the likelihood of a healthy choice depends on the relative attribute values of one option vs. another. Healthy choices are less likely when tastiness and healthiness conflict (conflict trials: one option is clearly better on one attribute, but worse on the other). (b) Specific goals prioritizing tastiness or prioritizing healthiness alter the frequency of healthy choice, although it still depends on the relative values of both tastiness and healthiness. Four example decision makers with different weights on healthiness and tastiness (wTaste, wHealth) are displayed for comparison. (c) The overall likelihood of a healthy choice (averaged separately for all combinations of conflict or no-conflict choices) depends on attribute weights. Goals prioritizing tastiness (darker bars) produce fewer healthy choices than goals prioritizing healthiness (lighter bars), but only when tastiness and healthiness conflict. (d) The drift diffusion model can also simulate expected neural activity (i.e., aggregate activity summed over decision time: ) when choosing healthy [H] or unhealthy [UH] options, as a function of relative attribute values of available options and different goals. Bars display the overall difference in neural activity for H compared to UH choices for goals prioritizing tastiness (darker bars) and healthiness (lighter bars), divided as a function of attribute conflict. In no-conflict trials, healthy choices elicit less activity regardless of goal (i.e., Activity H < Activity UH). In conflict trials, however, healthy choices elicit more activity (i.e., Activity H > Activity UH), but only when goals prioritize tastiness (dark gray bars). Identical results are obtained when substituting response time (RT) for neural response (see Figure 2—figure supplement 1). Similar results are also obtained when using a different formulation of the evidence accumulation process (see Appendix 2 and Appendix 1—figure 1).
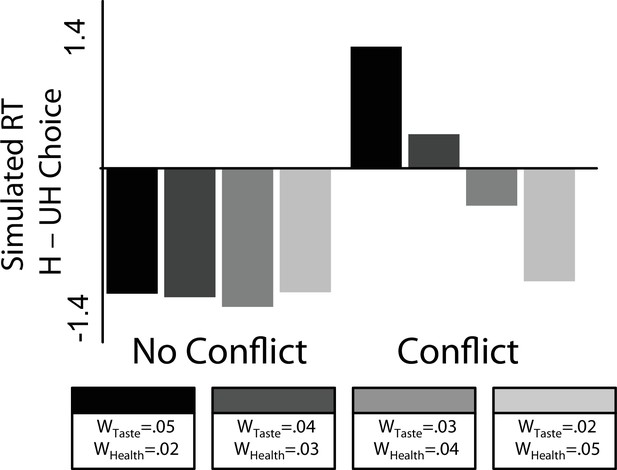
Computational simulations of response time (RT).
Similar to neural response, model simulations suggest that RTs when making normative (e.g., healthy, H) choices instead of hedonistic (e.g., unhealthy, UH) ones (i.e., RTH – RTUH) depends on the relative weighting of normative (healthiness) and hedonic (tastiness) choice attributes. Bars display the overall difference in RT for H compared to UH choices for goals prioritizing tastiness (darker bars) and healthiness (lighter bars), divided as a function of attribute conflict. In no-conflict trials, healthy choices elicit faster RTs regardless of goal (i.e., RTH < RTUH). In conflict trials, however, healthy choices take longer (i.e., RTH > RTUH), but only when goals prioritize tastiness.
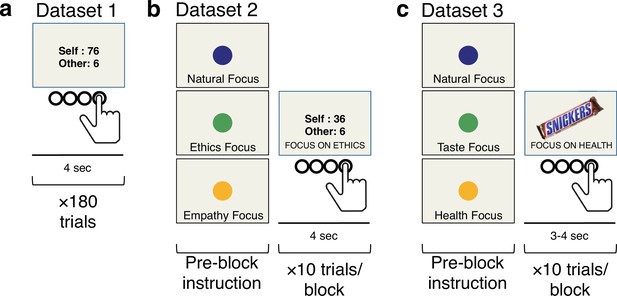
fMRI task designs.
(a) In Dataset 1, participants made choices involving tradeoffs between monetary payoff for another person ($Other; normative attribute) and for themselves ($Self; hedonic attribute) in an Altruistic Choice Task. (b) In Dataset 2, participants made choices similar to the Altruistic Choice Task in Study 1, while we manipulated the weights on normative and hedonic attributes using instructions presented at the beginning of each task block. These instructions asked participants to focus on different pro-social motivations (ethical considerations, partner’s feelings) as they made their choice. (c) In Dataset 3, we examined the generalizability of the model-based predictions in another choice domain. Here, participants performed a Food Choice Task in which the weights on food’s healthiness (normative attribute) and tastiness (hedonic attribute) were manipulated. In all studies, participants had 4 s to decide, and gave their response on a 4-point scale from ‘Strong No’ to ‘Strong Yes’.
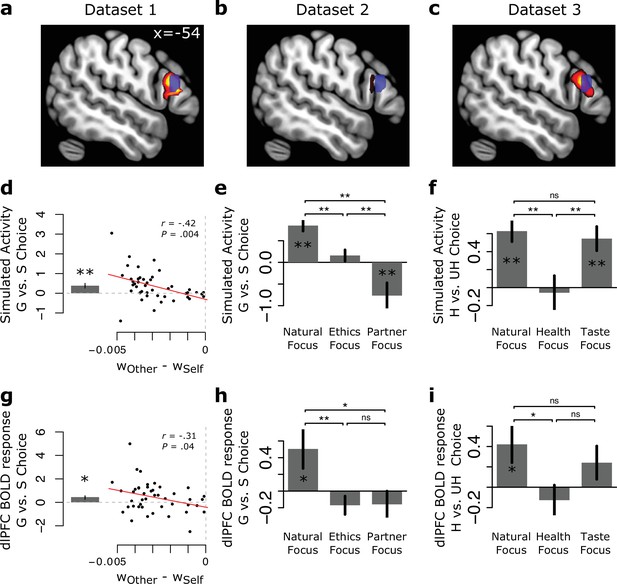
BOLD responses in the left dorsolateral prefrontal cortex (dlPFC) during self-control dilemmas.
Top (dlPFC): Trial-by-trial BOLD response in the dlPFC correlates significantly with predicted activity of the drift diffusion model (DDM) across three separate datasets. This includes responses during altruistic choice (a, b) and dietary choice (c). Hot colors display DDM-related responses thresholded for display purposes at p<0.001 (a and c) and p<0.005 (b), uncorrected. The location of this activity was anatomically similar to an area of the dlPFC associated with inhibitory control in a meta-analysis (Dockès et al., 2020) (blue region of interest [ROI] displayed in each panel), which served as our primary, independently selected ROI to test predictions of the computational model (d-f). Middle (Model Simulations): Given participants’ observed attribute weights, we simulated expected neural response during normative vs. hedonistic choice, using the DDM, for all trials in Dataset 1 (d), as well as separately for each condition in Dataset 2 (e) and Dataset 3 (f). Bottom (dlPFC Results): Within the independently defined dlPFC ROI associated with inhibitory control (blue voxels in a–c), BOLD response during normative choice vs. hedonistic choice when attributes conflict, in (g) Dataset 1 (N=50) for all trials, as well as in (h) Dataset 2 (N=49), and (i) Dataset 3 (N=113) as a function of regulatory goals. Regulatory goals were designed to decrease hedonic weights and increase normative weights on choice (Dataset 2: Ethics, Partner; Dataset 3: Health) or to emphasize weights on hedonic attributes (Dataset 3: Taste). Only conflict trials are considered across datasets. As predicted, normative choices activate the dlPFC, but only when goals result in a greater weight on hedonistic than normative attributes. Contexts that increase normative attribute weights yield less dlPFC activity during normative choices (Dataset 2: Ethics, Partner; Dataset 3: Health). *p<0.05 based on one-sample t-tests against 0, or paired-tests between conditions; **p<0.01. Error bars show standard error of the mean. Similar results were obtained from a left dlPFC region associated with dietary self-control in dieters (Hare et al., 2009), as well as a right-hemisphere version of the inhibitory control ROI (see Appendices 2-3 and Appendix 2—figure 1 and Appendix 3—figure 1 for details).
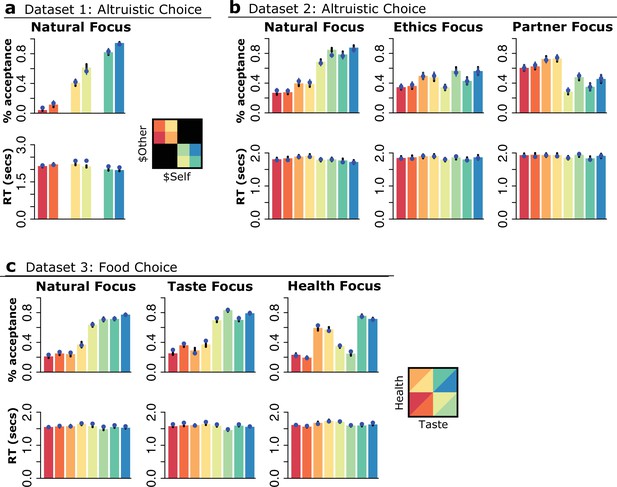
Model fits to behavior.
(a) Choices and response times (RTs) for observed behavior (colored bars) and model simulations (blue dots) for different choice types in Dataset 1 (N = 50). (b) Observed and model-simulated choices and RTs in Dataset 2 (N = 49), separately by regulatory condition. (c) Observed and model-simulated choices and RTs in Dataset 3 (N = 113), separately by regulatory condition. Error bars show standard error of the mean.
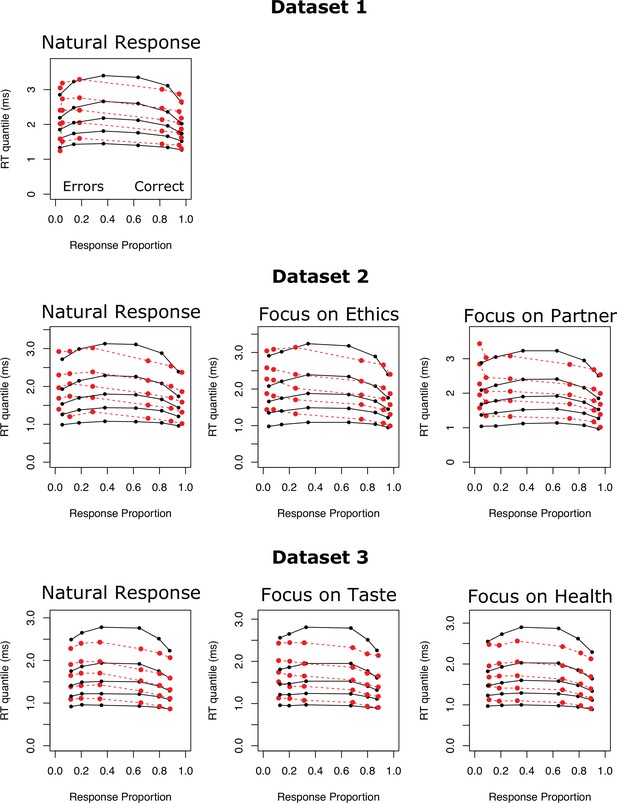
Alternative visualization of model fits to behavior.
Q-P plots of predicted and observed response proportions and response times (RTs) for (a) Study 1, (b) Study 2, and (c) Study 3. Black dots represent model predicted choice proportions (x-axis) for low-, medium-, and high-difficulty trials, separated into five response time quantiles (y-axis), separately for the 10%, 30%, 50%, 70%, and 90% quantiles. Error responses correspond to response proportions below 50%. Red dots represent the observed choice proportions and RTs for these same trials.
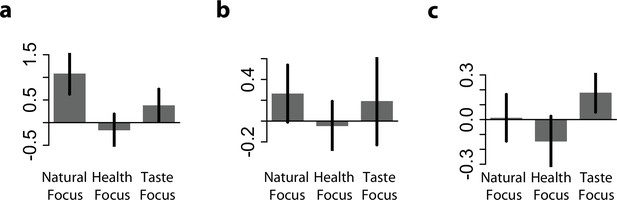
Healthy vs. unhealthy choices by sample in Dataset 3.
BOLD responses in the left dorsolateral prefrontal cortex (dlPFC) during healthy vs. unhealthy choices in Dataset 3, separately by (a) Sample 3a, N=3622; (b) Sample 3b, N=336; and (c) Sample 3c, N=4431. Error bars show standard error of the mean.
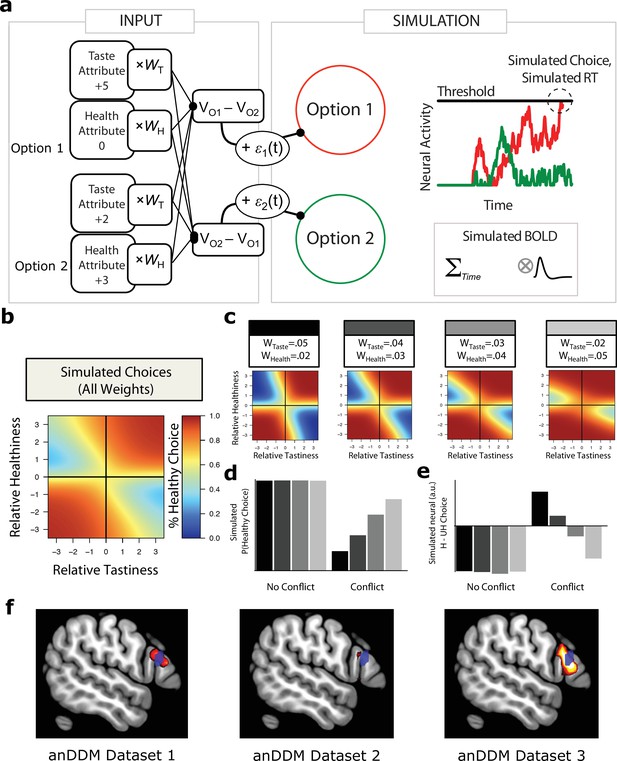
Simulating the dilemma of self-control using the attribute-based neural drift diffusion model (anDDM).
(a) An evidence accumulation model (anDDM) can be used to simulate decision making for any self-control context requiring an integration of normative and hedonistic considerations (dietary choices displayed, integrating relative tastiness, and healthiness). (b) On average across multiple different goals, the likelihood of a healthy choice depends on the relative attribute values of one option vs. another, and is less likely when tastiness and healthiness conflict. Warmer colors indicate a higher likelihood of choosing the healthier option. (c) As with the DDM, specific goals prioritizing tastiness or prioritizing healthiness alter the frequency of healthy choice, although it still depends on the relative values of both tastiness and healthiness. Four example, decision makers with different weights on healthiness and tastiness are displayed for comparison. (d) The overall likelihood of a healthy choice (averaged separately for all combinations of conflict or no-conflict choices) depends on weights. Goals prioritizing tastiness (darker bars) produce fewer healthy choices than goals prioritizing healthiness (lighter bars), but only when tastiness and healthiness conflict. (e) The computational model can also simulate expected neural activity (i.e., aggregate activity in the two neuronal pools, summed over decision time: ) when choosing healthy [H] or unhealthy [UH] options, as a function of relative option values and different goals. Bars display the overall difference in neural activity for H compared to UH choices for goals prioritizing tastiness (darker bars) and healthiness (lighter bars), divided as a function of attribute conflict. In no-conflict trials, healthy choices elicit less activity regardless of the goal (i.e., Activity H Activity UH), but only when goals prioritize tastiness. (f) Neural activation in the left dlPFC match estimates of the anDDM on that trial (displayed at p < 0.001, uncorrected in left and right-most images, p = 0.005, uncorrected in middle image). Blue region of interest (ROI) indicates the left dlPFC area identified as correlating with self-control using the meta-analytic database NeuroQuery (Dockès et al., 2020).
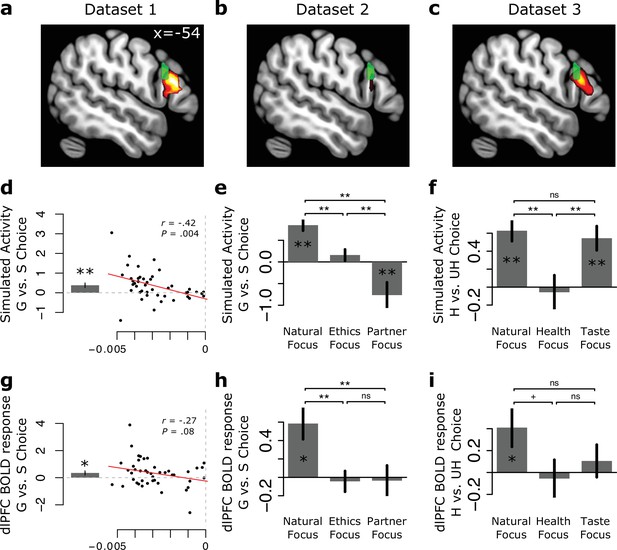
BOLD responses in a left dorsolateral prefrontal cortex (dlPFC) region associated with self-control in dieters.
Top (dlPFC): Trial-by-trial BOLD response in the dlPFC correlates significantly with predicted activity of the drift diffusion model (DDM) across three separate datasets. This includes responses during altruistic choice (a, b) and dietary choice (c). Hot colors display DDM-related responses thresholded for display purposes at p < 0.001 (a and c) and p < 0.005 (b), uncorrected. The location of this activity was anatomically similar to an area of the dlPFC associated with self-control in dieters (Hare et al., 2009) (green region of interest [ROI] displayed in each panel), which served as a supplementary ROI in which to test predictions of the computational model (d–i). Middle (Model Simulations): Given participants’ observed attribute weights, we simulated expected neural response during normative vs. hedonistic choice, using the DDM, for all trials in Dataset 1 (d), as well as separately for each condition in Dataset 2 (e) and Dataset 3 (f). Bottom (dlPFC Results): Within the independently defined dlPFC ROI associated with self-control in dieters (green voxels in a–c), BOLD response during normative choice vs. hedonistic choice when attributes conflict, in (g) Dataset 1 (N = 50) for all trials, as well as in (h) Dataset 2 (N = 49) and (i) Dataset 3 (N = 113) as a function of regulatory goals. Regulatory goals were designed to decrease hedonic weights and increase normative weights on choice (Dataset 2: Ethics, Partner; Dataset 3: Health) or to put weights on hedonic attributes (Dataset 3: Taste). Only conflict trials are considered across datasets. As predicted, normative choices activate the dlPFC, but only when goals result in a greater weight on hedonistic than normative attributes. Contexts that increase normative attribute weights yield less dlPFC activity during normative choices (Dataset 2: Ethics, Partner; Dataset 3: Health). *p < 0.05 based on one-sample t-tests against 0, or paired-tests between conditions; **p < 0.01. Error bars show standard error of the mean.
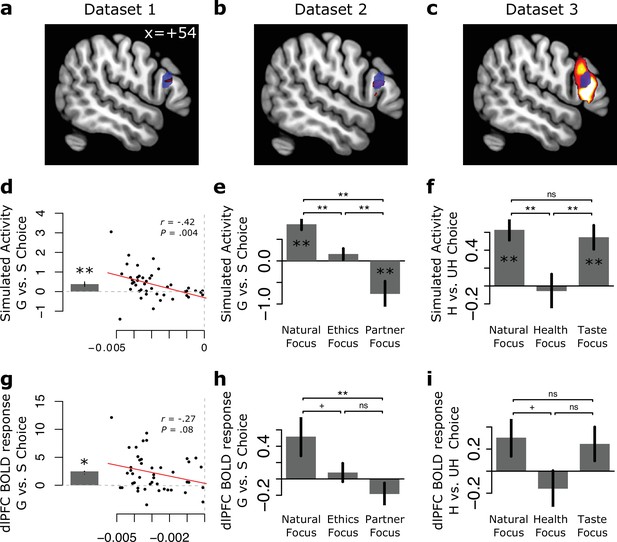
BOLD responses in the right dorsolateral prefrontal cortex (dlPFC) during self-control dilemmas.
Top (dlPFC): Trial-by-trial BOLD response in the right dlPFC correlates significantly with predicted activity of the drift diffusion model (DDM) across three separate datasets. This includes responses during altruistic choice (a, b) and dietary choice (c). Hot colors display DDM-related responses thresholded for display purposes at p < 0.001, uncorrected. The location of this activity is anatomically similar to an area of the left dlPFC, mirrored over the x-axis to the right hemisphere, and associated with inhibitory control in a meta-analysis Dockès et al., 2020 (blue ROI displayed in each panel), which served as a supplementary region of interest (ROI) in which to test predictions of the computational model (d–i). Middle (Model Simulations): Given participants’ observed attribute weights, we simulated expected neural response during normative vs. hedonistic choice, using the DDM, for all trials in Dataset 1 (d), as well as separately for each condition in Dataset 2 (e) and Dataset 3 (f). Bottom (dlPFC Results): Within the independently defined right dlPFC ROI (blue voxels in a–c), BOLD response during normative choice vs. hedonistic choice when attributes conflict, in (g) Dataset 1 (N = 50) for all trials, as well as in (h) Dataset 2 (N = 49) and (i) Dataset 3 (N = 113) as a function of regulatory goals. Regulatory goals were designed to decrease hedonic weights and increase normative weights on choice (Dataset 2: Ethics, Partner; Dataset 3: Health) or to put weights on hedonic attributes (Dataset 3: Taste). Only conflict trials are considered across datasets. As predicted, normative choices activate the dlPFC, but only when goals result in a greater weight on hedonistic than normative attributes. Contexts that increase normative attribute weights yield less dlPFC activity during normative choices (Dataset 2: Ethics, Partner; Dataset 3: Health). *p < 0.05 based on one-sample t-tests against 0, or paired-tests between conditions; **p < 0.01. Error bars show standard error of the mean.
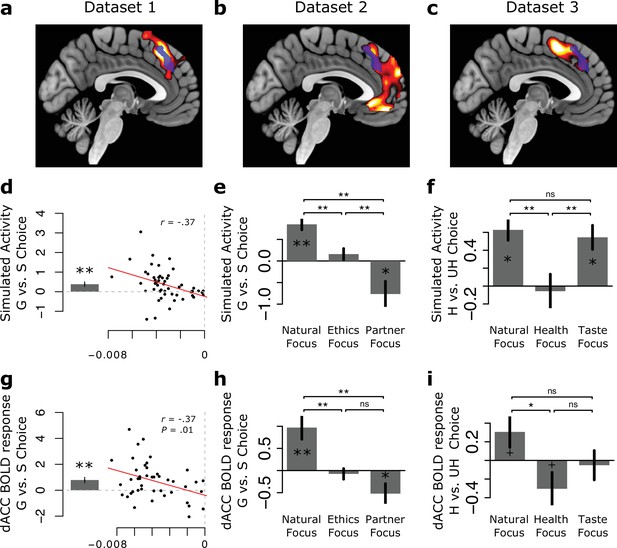
BOLD responses in the dorsal anterior cingulate cortex (dACC) during self-control dilemmas.
Top (dACC): Trial-by-trial BOLD response in the dACC correlates significantly with predicted activity of the drift diffusion model (DDM) across three separate datasets (hot colors display DDM-related responses thresholded at p < 0.001, uncorrected for display purposes). This includes responses during altruistic choice (a, b) and dietary choice (c). Voxels of overlap across all three datasets are indicated in blue, and served as a region of interest (ROI) to extract BOLD responses during normative and hedonistic choices in each dataset (g–i). Here, we show for illustrative purposes only that activity in this same ROI shows similar patterns of response on normative vs. hedonistic choices as the left dlPFC and resembles model predictions (d–f). Middle (Model Simulations): Given participants’ observed attribute weights, we simulated expected neural response during normative vs. hedonistic choice, using the DDM, for all trials in Dataset 1 (d), as well as separately for each condition in Dataset 2 (e) and Dataset 3 (f). Bottom (dACC Results): Within the dACC ROI associated with the DDM (blue voxels in a–c), BOLD response differed as predicted during normative choice vs. hedonistic choice when attributes conflict, in (g) Dataset 1 (N = 50) for all trials, as well as in (h) Dataset 2 (N = 49) and (i) Dataset 3 (N = 113) as a function of regulatory goals. Regulatory goals were designed to decrease hedonic weights and increase normative weights on choice (Dataset 2: Ethics, Partner; Dataset 3: Health) or to put weights on hedonic attributes (Dataset 3: Taste). Only conflict trials are considered across datasets. As predicted, normative choices activate the dACC, but only when goals result in a greater weight on hedonistic than normative attributes. Contexts that increase normative attribute weights yield less dACC activity during normative choices (Dataset 2: Ethics, Partner; Dataset 3: Health). +p < 0.05, one-tailed; *p < 0.05, two-tailed; **p < 0.01, two-tailed, based on one-sample t-tests against 0, or paired-tests between conditions. Error bars show standard error of the mean
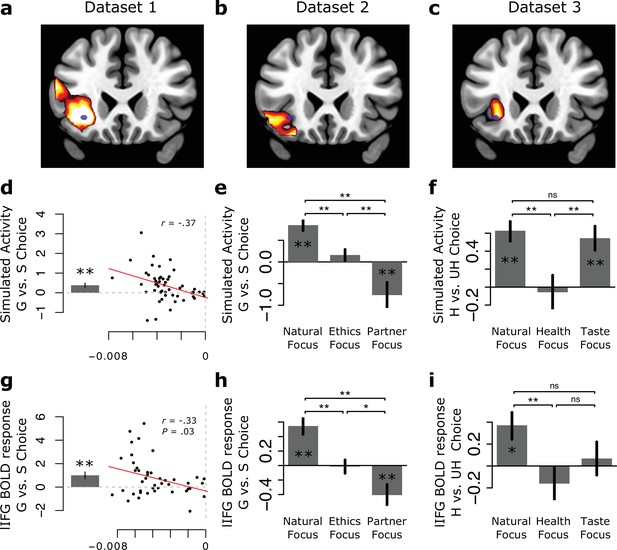
BOLD responses in the inferior frontal gyrus/anterior insula (IFG/aIns) during self-control dilemmas.
Top (lIFG/anterior insula [aIns]): Trial-by-trial BOLD response in the lIFG/aIns correlates significantly with predicted activity of the drift diffusion model (DDM) across three separate datasets (hot colors display DDM-related responses thresholded at Pp < 0.001, uncorrected for display purposes). This includes responses during altruistic choice (a, b) and dietary choice (c). Voxels of overlap across all three datasets are indicated in blue, and served as an region of interest (ROI) to extract BOLD responses during normative and hedonistic choices in each dataset (g–i). Here, we show for illustrative purposes only that activity in this same ROI shows similar patterns of response on normative vs. hedonistic choices as the left dlPFC and resembles model predictions (d–f). Middle (Model Simulations): Given participants’ observed attribute weights, we simulated expected neural response during normative vs. hedonistic choice, using the DDM, for all trials in Dataset 1 (d), as well as separately for each condition in Dataset 2 (e) and Dataset 3 (f). Bottom (lIFG/aIns Results): Within the lIFG/aIns ROI associated with the DDM (blue voxels in a–c), BOLD response differed as predicted during normative choice vs. hedonistic choice when attributes conflict, in (g) Dataset 1 (N = 50) for all trials, as well as in (h) Dataset 2 (N = 49) and (i) Dataset 3 (N = 113) as a function of regulatory goals. Regulatory goals were designed to decrease hedonic weights and increase normative weights on choice (Dataset 2: Ethics, Partner; Dataset 3: Health) or to put weights on hedonic attributes (Dataset 3: Taste). Only conflict trials are considered across datasets. As predicted, normative choices activate the dACC, but only when goals result in a greater weight on hedonistic than normative attributes. Contexts that increase normative attribute weights yield less lIFG/aIns activity during normative choices (Dataset 2: Ethics, Partner; Dataset 3: Health). * Pp < 0.05, two-tailed; ** Pp < 0.01, two-tailed, based on one-sample t-tests against 0, or paired-tests between conditions. Error bars show standard error of the mean.
Tables
Model fits as assessed by AIC and BIC.
Model | Recurrence | Lateral Inhibition | RelativeValues | NaturalAIC/BIC | TasteAIC/BIC | HealthAIC/BIC |
---|---|---|---|---|---|---|
1 | x | x | x | 1401/1418 | 1397/1415 | 1418/1435 |
2 | x | x | 1403/1418 | 1395/1410 | 1414/1430 | |
3 | x | x | 1396/1411 | 1394/1409 | 1392/1407 | |
4 | x | 1395/1407 | 1388/1400 | 1409/1421 | ||
5_ | x | x | 1436/1453 | 1409/1427 | 1436/1453 | |
6 | x | 1428/1443 | 1420/1435 | 1445/1460 | ||
7 | x | 1417/1432 | 1403/1418 | 1436/1451 | ||
8 | 1429/1442 | 1419/1432 | 1445/1457 | |||
9 | Canonical DDM | 1397/1409 | 1393/1406 | 1412/1425 |
Additional files
-
Supplementary file 1
Table S1.
Estimated Model Parameters: All Datasets. Parameter values were estimated using a differential-evolution Markov chain Monte Carlo method developed by Holmes and Trueblood, 2018. Parameters beginning with w indicate weighting parameters applied to different attributes. Datasets 1 and 2: proposed payoff to self vs. the default, proposed payoff to other vs. the default, fairness [|$Self − $Other|], and a constant bias toward the proposal; Dataset 3: tastiness and healthiness vs. the default as well as a constant bias toward the displayed food. B: choice-defining threshold. ndt: non-decision time. A priori constraints on the parameters, determined based on previous work and on theoretical limits, restricted them to the range indicated. In Datasets 2 and 3, columns indicated by different subscripts (a–c) differ significantly from each other at p<0.05, corrected for multiple comparisons. * Average parameter values collapsing over three independently collected samples of participants (see Supplementary file 2 for details of each sample separately).
- https://cdn.elifesciences.org/articles/65661/elife-65661-supp1-v1.docx
-
Supplementary file 2
Table S2.
Estimated Model Parameters: Dataset 3, separately by Samples 3a, 3b, and 3c. Parameter values were estimated using a differential-evolution Markov chain Monte Carlo method developed by Holmes and Trueblood, 2018. Parameters beginning with w indicate weighting parameters applied to tastiness and healthiness vs. the default as well as a constant bias toward the displayed food. B: choice-defining threshold. ndt: non-decision time. A priori constraints on the parameters, determined based on previous work and on theoretical limits, restricted them to the range indicated. Columns indicated by different subscripts (a–c) differ significantly from each other at p < 0.05, corrected for multiple comparisons.
- https://cdn.elifesciences.org/articles/65661/elife-65661-supp2-v1.docx
-
Supplementary file 3
Table S3.
Neural correlates of the drift diffusion model (DDM) across datasets. Regions are reported at a voxel level of p < 0.001, uncorrected and a whole-brain FWE cluster-corrected level of p < 0.05, unless otherwise noted. * Distinct peak within larger cluster. † Significant at p < 0.005, uncorrected, reported for completeness.
- https://cdn.elifesciences.org/articles/65661/elife-65661-supp3-v1.docx
-
Supplementary file 4
Table S4.
Neural correlates of normative vs. hedonistic choice across datasets. Regions are reported at a voxel-level threshold of p < 0.001, uncorrected, and a minimum volume of k = 10 voxels, unless otherwise noted. * Significant at p < 0.005, uncorrected and minimum volume of k=20 voxels, reported for completeness. ** Distinct peak within larger cluster, reported for completeness.
- https://cdn.elifesciences.org/articles/65661/elife-65661-supp4-v1.docx
-
Transparent reporting form
- https://cdn.elifesciences.org/articles/65661/elife-65661-transrepform1-v1.pdf