Evidence for a deep, distributed and dynamic code for animacy in human ventral anterior temporal cortex
Figures
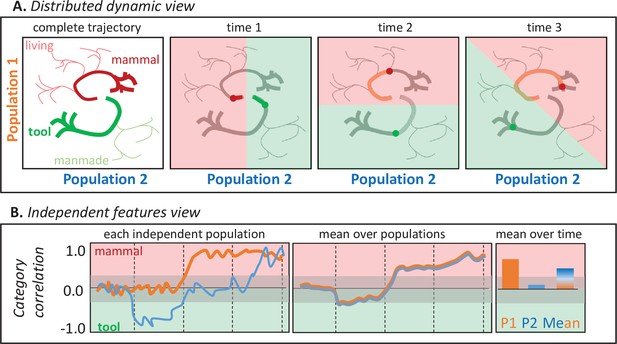
Two views of neural representation.
A. Hypothetical joint activations of two neural populations to living and manmade items (left), and the classification plane that would best discriminate tools from mammals at different timepoints. Jointly the two populations always discriminate the categories, but the contribution of each population to classification changes over time so that the classification plane rotates. B. Independent correlations between each population’s activity and a binary category label (tool/mammal) for the same trajectories plotted above, shown across time for each population (left), averaged across the two populations (middle), and averaged over time for each population independently or for both populations (right). Independent correlations suggest conclusions about when and how semantic information is represented that are incorrect under the distributed and dynamic view.
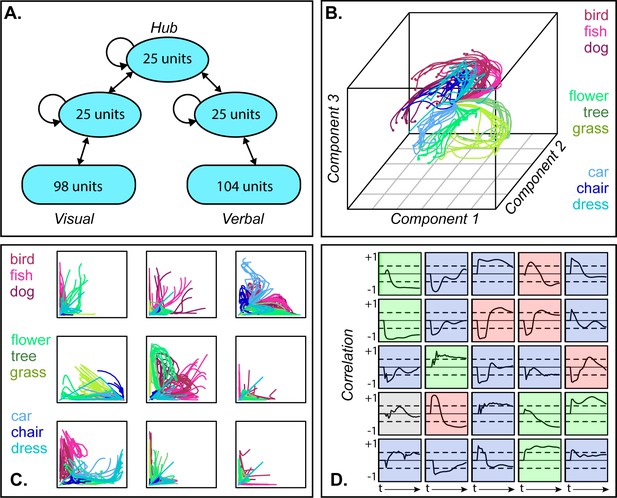
Dynamic representation in a neural network model of semantic processing.
A. Model architecture. B. 3D MDS of hub activation patterns learned in one model run—each line shows the trajectory of a single item over time in the compressed space. C. The same trajectories shown in uncompressed unit activations for nine randomly sampled unit pairs, horizontal and vertical axes each showing activation of one unit. D. Feature-based analysis of each hub unit in one network run. Each square shows one unit. Lines trace, across time, the correlation between unit activation and category labels across items with dashed lines showing significance thresholds. Color indicates different patterns of responding (see text).
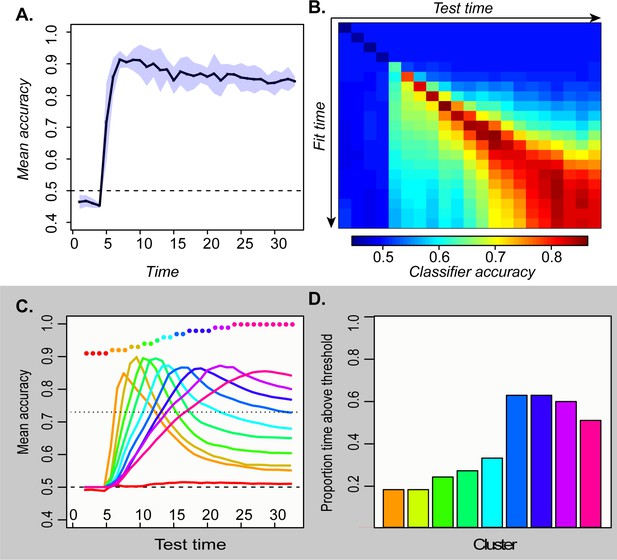
Temporal generalization profiles for deep network.
A. Mean and 95 % confidence interval of the hold-out accuracy for classifiers trained at each tick of time in the model. B. Accuracy for each classifier (rows) tested at each point in time (columns). C. Mean accuracy for each cluster of classifiers at every point in time. Colored dots show the timepoints grouped together in each cluster. D. Proportion of the full time-window for which mean classifier accuracy in each cluster was reliably above chance.
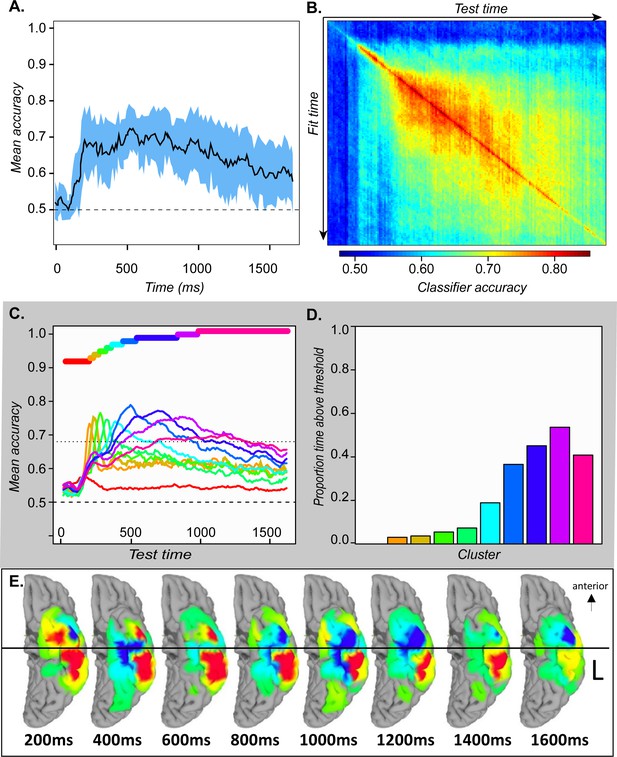
ECoG analyses.
A. Mean and 95 % confidence interval of the hold-out accuracy for classifiers trained at each 50 ms time window of ECoG data. B. Mean accuracy across participants for each classifier (rows) tested at each timepoint (columns) in the ECoG data. C. Mean accuracy for each cluster of classifiers at every point in time. Colored bars show the timepoints grouped together in each cluster. D. Proportion of the full time-window for which mean classifier accuracy in each cluster was reliably above chance. E. Mean classifier coefficients across participants plotted on a cortical surface at regular intervals over the 1640 ms window. Warm vs cool colors indicate positive versus negative mean coefficients, respectively. In A and C, vertical line indicates mean onset of naming.
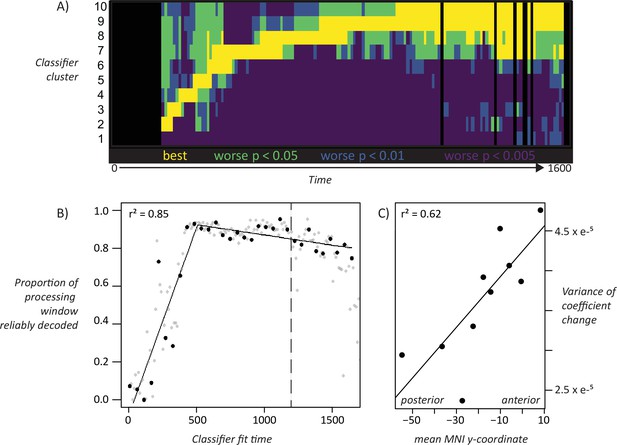
Statistical assessment of key patterns.
A. Statistical assessment of ‘overlapping waves’ pattern. Each row corresponds to one cluster of decoding models as shown in Figure 4C. Black vertical lines indicate timepoints where decoding is not reliable across subjects. Yellow shows the best-performing model cluster and other clusters that statistically perform as well. Green, blue, and purple indicate clusters that perform reliably worse than the best-performing cluster at increasingly strict statistical thresholds controlling for a false-discovery rate of 0.05. B. Broadening window of generalization. For classifiers fit at each time window, breadth of classifier generalization (as proportion of full processing window) is plotted againt the time at which the classifier was fit. The line shows a piecewise-linear model fit to 32 non-overlapping time windows (black dots). The most likely model had a single inflection point at 473 ms post stimulus-onset, with breadth of generalization increasing linearly over this span, then hittng ceiling through most of the remaining processing window. The dashed line shows mean response latency. C. Fluctuating codes in more anterior regions. Correlation between mean variance of coefficient change (see text) and anterior/posterior electrode location for electrodes grouped by decile along the anterior/posterior axis.
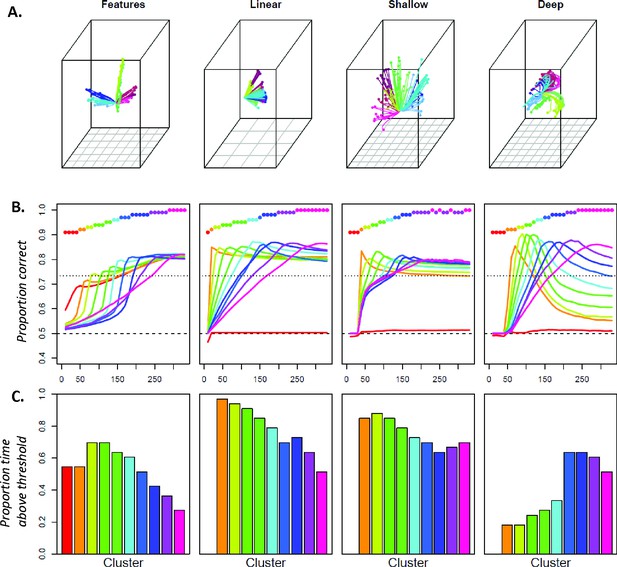
Comparison of simulation results for a feature-based model, a distributed linear model, a shallow recurrent network, and the deep, distributed and dynamic model.
A. Multi-dimensional scaling showing the trajectory of each item through representation space under four different models. Only the deep model shows radically nonlinear change. B. Mean accuracy for clusters of classifiers under each model type. Only the deep model shows the overlapping-waves pattern. C. Proportion of time-window where classifiers in each cluster show reliably above-chance responding. Only the deep model shows a generalization window that widens over time.
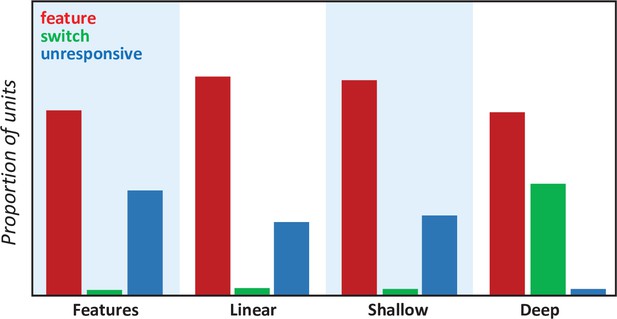
Types of units in each model.
For each model type, the proportion of units that behave like feature-detectors (red), detectors that switch their category preference over time (green), and units that seem unresponsive to the semantic category (blue). Only the deep, distributed, dynamic model has units whose responses switch their category preference over time.
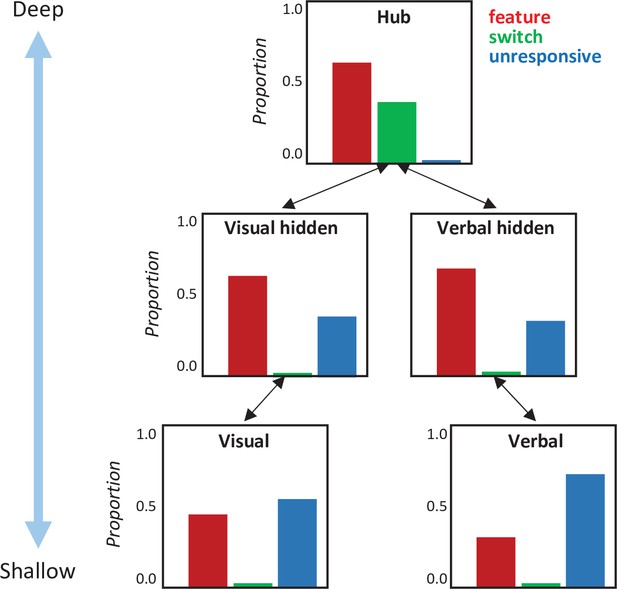
Types of unit in each layer of deep model.
For each layer in the deep, distributed and dynamic model, the proportion of units that behave like feature-detectors (red), detectors that switch their category preference over time (green), and units that seem unresponsive to the semantic category (blue) when the model processes visual inputs. Only the hub layer of the network—the model analog to the ventral anterior temporal cortex—contained units whose responses switch their category preference over time.
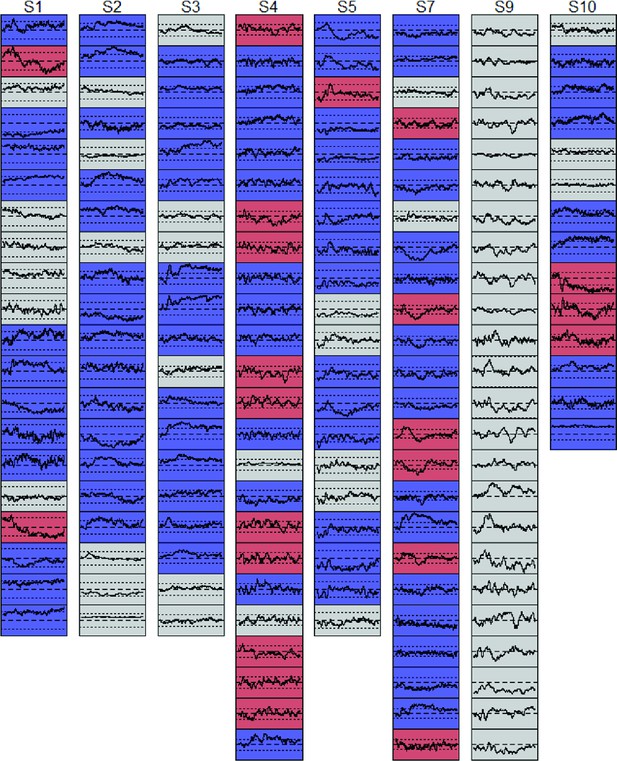
Independent correlations for each electrode.
Each panel shows, for each electrode in each participant, the correlation over time between the measured VP for each item and the category label. Dotted lines show statistical significance threholds for this correlations. Gray panels never exceed the threshold in either direction. Blue panels exceed it in one direction only. Red panels exceed it in both directions at different points in time.
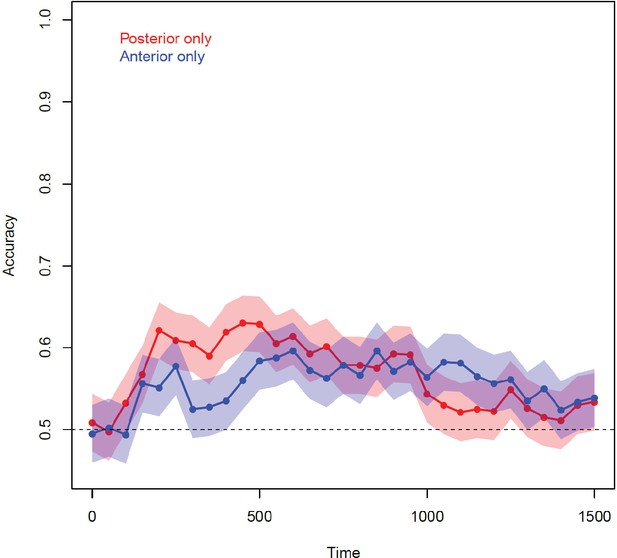
Accuracy for classifiers trained on anterior or posterior electrodes only.
Curves show expected probability of correct classification and 95 % confidence intervals (from binomial distribution) across participants at each window for classifiers trained only on the anterior (blue) or posterior (red) half of the electrodes. Decoding accuracy exceeds chance for both subsets and does not reliably differ between these.
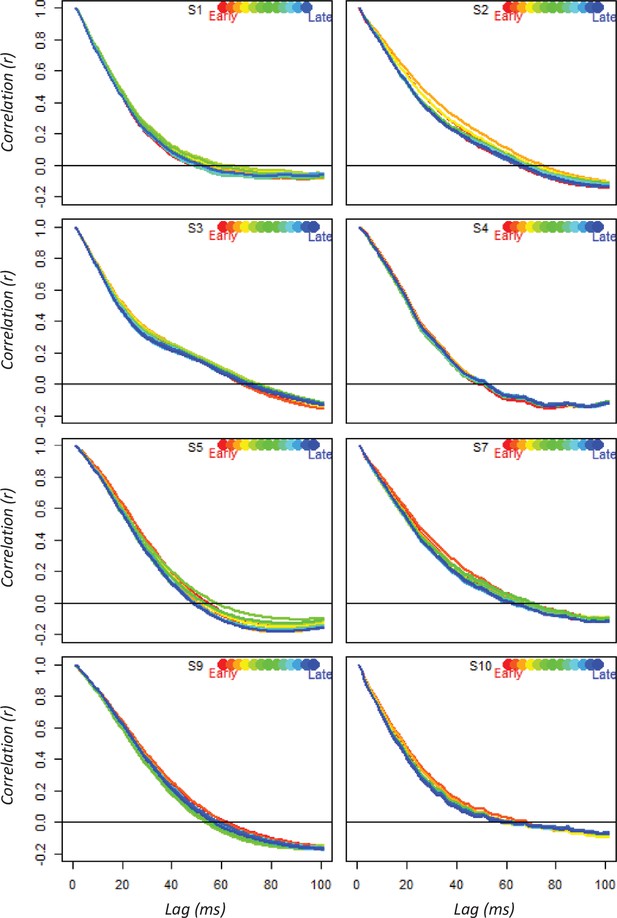
Temporal autocorrelation.
Each panel shows the mean temporal autocorrelation curves for a 300 ms moving window, averaged over all electrodes for each subject. If VPs auto-correlate over an increasingly wide temporal window, then later curves would show a broader envelope than earlier curves. Instead the different windows sit on top of one another, showing temporal autocorrelation that decays to zero after about 60 ms.
Videos
Animation showing direction of classifier coefficients across all participants, projected and smoothed along the cortical surface, across successive time-windows over the course of stimulus processing.
Colored regions indicate areas receiving non-zero coefficients, with cool colors indicating negative mean coefficients, green indicating means near zero, and warm colors indicating positive mean coefficients. Coefficients anterior to the dashed line fluctuate more relative to those posterior to the line, which are more consistent over time.
Tables
Patient characteristics.
CPS: complex partial seizure; GTCS: generalized tonic clonic seizure; ECoG: electrocorticogram; ERS: epigastric rising sensation; a/pMTG: anterior/posterior part of the middle temporal gyrus; a/pMTG: anterior/posterior part of the middle temporal gyrus; FCD: focal cortical dysplasia; * dual pathology ** diagnosed by clinical findings.
Patient 1 | Patient 2 | Patient 3 | Patient 4 | |
---|---|---|---|---|
Age, gender, handedness | 22 M R | 29 M R&L | 17 F R | 38 F R |
WAIS-R (VIQ,PIQ,TIQ) | 70, 78, 69 | 72, 78, 72 | 67, 76, 69 | 84,97,89 |
WMS-R(Verb, Vis, Gen,Attn, Del recall) | 99, 64, 87, 91, 82 | 99, 92, 97, 87, 83 | 51, < 50, < 50, 81, 56 | 75,111,83,62,53 |
WAB | 95.6 | 96 | 97.2 | 98.5 |
WADA test (Language) | Left | Bilateral | Left | Left |
Age of seizure onset | 16 | 10 | 12 | 29 |
Seizure type | non-specific aura→ CPS, GTCS | aura (metamorphosia, ERS) → CPS | discomfort in throat→ CPS | ERS →CPS |
Ictal ECoG onset | aMTG | PHG | PHG | PHG |
MRI | L basal frontal cortical dysplasiaL anterior temporal arachnoid cyst | L posterior temporal cortical atrophy | L temporal tip arachnoid cyst | L hippocampal atrophy/sclerosis |
Pathology | FCD type IA | FCD type IAHippocampal sclerosis* | FCD type IB | Hippocampal sclerosis** |
Patient 5 | Patient 7 | Patient 9 | Patient 10 | |
Age, gender, handedness | 55 M R | 41 F R | 51 M R | 38 F R |
WAIS-R (VIQ,PIQ,TIQ) | 105,99,103 | 72, 83, 75 | 73, 97, 83 | 109, 115,112 |
WMS-R(Verb, Vis, Gen,Attn, Del recall) | 71,117,84,109,72 | 83,111,89,94,82 | 80,101,85,1919,91 | 71,79,70,90,58 |
WAB | 98 | 97.3 | 89.6 | 96.9 |
WADA test (Language) | Left | Right | Left | Left |
Age of seizure onset | 55 | 19 | 43 | 28 |
Seizure type | CPS (once) | aura (nausea,feeling pale) → CPS | CPS | non-specific aura→ CPS |
Ictal ECoG onset | none | PHG | mITG | SMG |
MRI | Low-grade gliomaL medial temporal lobe | L hippocampal atrophy/screrosisL parieto-occipital perinatal infarction | Left temporal cavernoma | L parietal opercurum tumor |
Pathology | Diffuse astrocytoma | FCD IAHippocampal sclerosis* | arteriovenous malformation | Oligoastrocytoma |