Infrared molecular fingerprinting of blood-based liquid biopsies for the detection of cancer
Figures
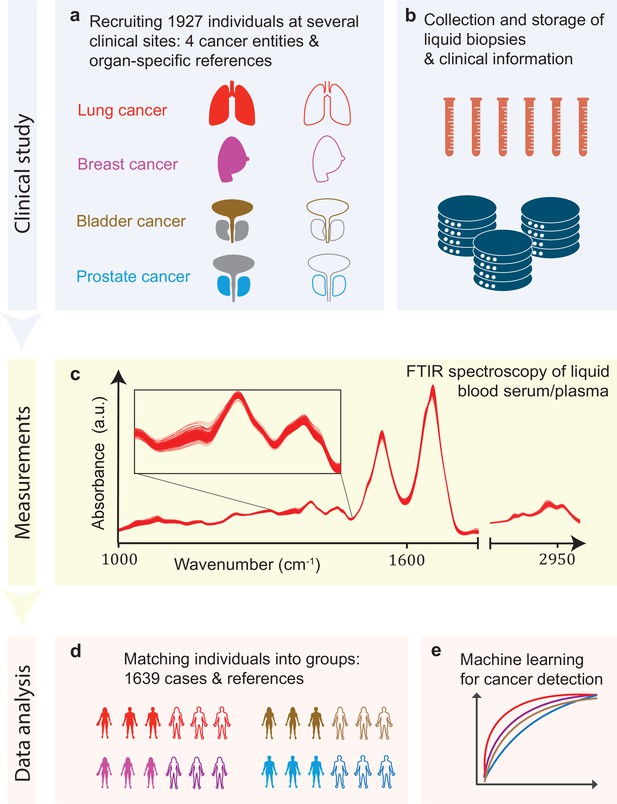
Infrared molecular fingerprinting workflow and clinical study design.
(a) Cohorts of therapy-naïve, lung, breast, prostate, and bladder cancer patients (cases), and organ-specific symptomatic references as well as non-symptomatic reference individuals were recruited at three different clinical sites – in total, 1927 individuals. (b) Blood samples from all individuals were drawn, and sera and plasma were prepared according to well-defined standard operating procedures. (c) Automated Fourier-transform infrared spectroscopy of liquid bulk sera and plasma were used to obtain IMFs. The displayed IMFs were pre-processed using water correction and normalization (see Methods). (d) For each clinical question studied, the characteristics of the case and the reference cohorts were matched for age, gender, and body mass index (BMI) to avoid patient selection bias. This resulted in total number of 1639 individuals upon matching. (e) Machine learning models were built on training datasets and evaluated on test datasets to separately evaluate the efficiency of classification for each of the four cancer entities.
-
Figure 1—source data 1
Breakdown of the overall participant pool used within the study.
All the following analyses were carried out on subsets of this participant pool; see also other source data files for further details. When selecting the sub-cohorts, special care was taken to match the case and reference cohorts separately, for each question – according to age, gender, and body mass index (BMI) – in order to avoid possible bias in patient selection.
- https://cdn.elifesciences.org/articles/68758/elife-68758-fig1-data1-v1.xlsx
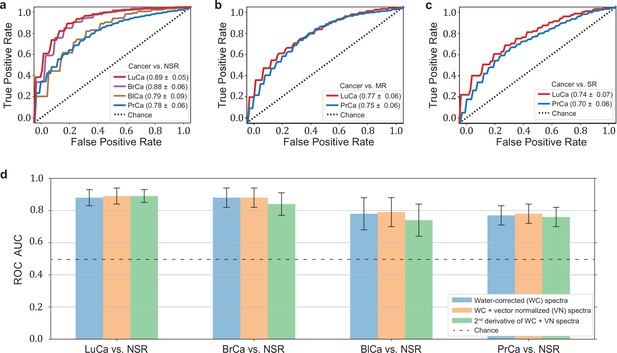
Diagnostic performance of lung, prostate, bladder, and breast cancer detection based on infrared molecular fingerprints (IMFs) of blood sera.
Receiver operating characteristic (ROC) curves for the binary classification of the test set with support vector machine (SVM) models trained on water-corrected and vector-normalized IMFs. The different cancer entities were tested against (a) non-symptomatic references, (b) mixed references that also include organ-specific symptomatic references, and (c) organ-specific symptomatic references only. Detailed cohort characteristics can be found in Figure 2—source data 1. (d) Area under the receiver operating characteristic curve (AUC) for the test sets according to different spectral pre-processing of the IMFs. The error bars show the standard deviation of the individual results of the cross-validation (LuCa: lung cancer; PrCa: prostate cancer; BrCa: breast cancer; BlCa: bladder cancer; NSR: non-symptomatic references; MR: mixed references; SR: symptomatic references).
-
Figure 2—source data 1
Characteristics of the matched groups of individuals utilized for the analysis as presented in Table 1, Figures 2 and 3a-c.
- https://cdn.elifesciences.org/articles/68758/elife-68758-fig2-data1-v1.xlsx
-
Figure 2—source data 2
Zipped folder with trained machine learning models and application instructions.
- https://cdn.elifesciences.org/articles/68758/elife-68758-fig2-data2-v1.zip
-
Figure 2—source data 3
Potential impact of clinical site to classification performance.
- https://cdn.elifesciences.org/articles/68758/elife-68758-fig2-data3-v1.xlsx
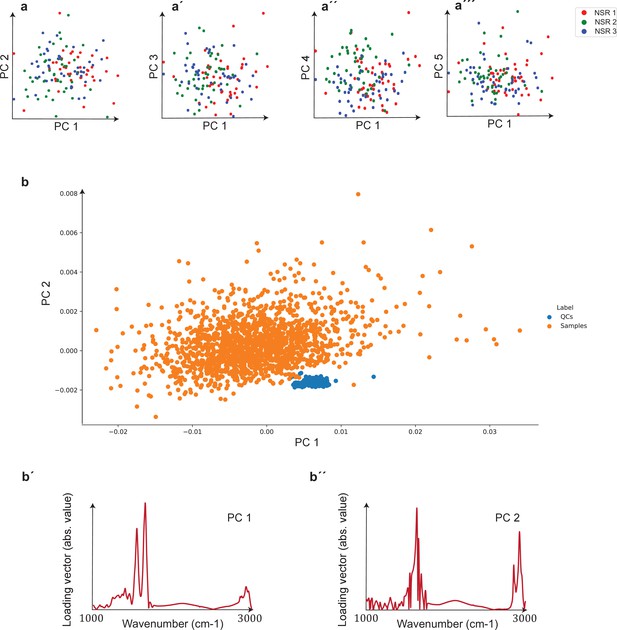
Unsupervised comparison between data from the three clinical sites as well as quality control (QC) analysis of measurements.
(a–a′′′) Principal component analysis (PCA) of samples of non-symptomatic healthy individuals collected from three different clinical sites. Plots depict the first five principal components, which correspond to 95% of the explained variance. The three groups are statistically matched in terms of age, gender, and body mass index (BMI). Cohort characteristics are given in Figure 2—figure supplement 1—source data 1. (b) PCA plot of biological samples and QCs. The two first principal components included in the plot correspond to 93% of the explained variance. (b′, b′′) Loading vectors for the two principal components shown in (b).
-
Figure 2—figure supplement 1—source data 1
Characteristics of the matched groups utilized for the analysis presented in Figure 2—figure supplement 1.
- https://cdn.elifesciences.org/articles/68758/elife-68758-fig2-figsupp1-data1-v1.xlsx
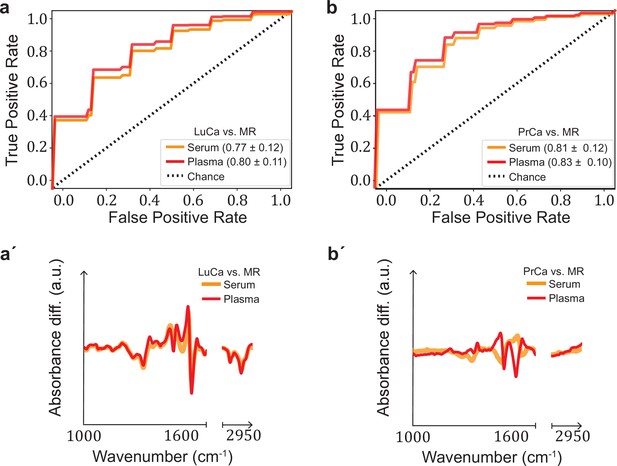
Performance comparison of serum- and plasma-based fingerprints for cancer detection.
Receiver operating characteristic (ROC) curves for (a) lung cancer (LuCa) and (b) prostate cancer (PrCa) vs. mixed references (MR). Differential fingerprints (a′, b′) for the same comparisons as above. The characteristics of the cohort used for this analysis are given in Figure 2—figure supplement 2—source data 1.
-
Figure 2—figure supplement 2—source data 1
Characteristics of the matched groups utilized for the analysis presented in Figure 2—figure supplement 2.
- https://cdn.elifesciences.org/articles/68758/elife-68758-fig2-figsupp2-data1-v1.xlsx
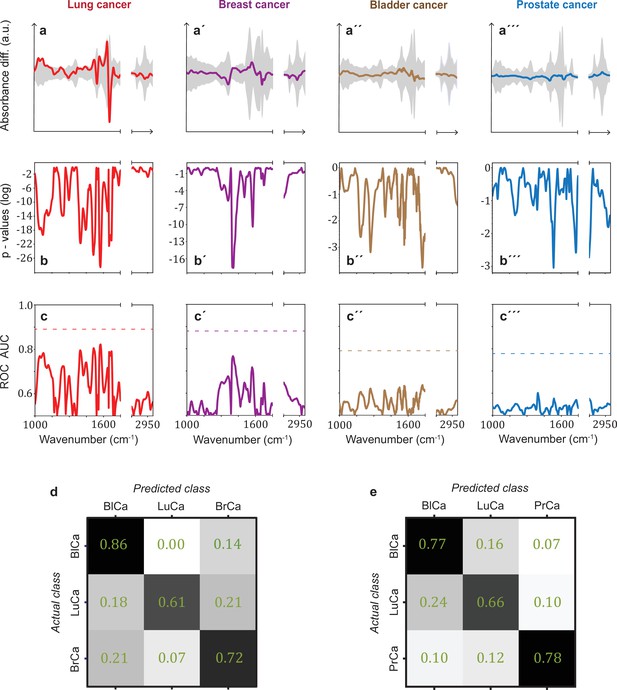
Infrared spectral signatures of lung, prostate, bladder, and breast cancer.
(a-a''') Differential fingerprints (standard deviations of the reference cohorts are displayed as grey areas), (b-b''') two-tailed p-value of Student’s t-test, and (c-c''') area under the receiver operating characteristic curve (AUC) per wavenumber (extracted by application of Mann–Whitney U test) compared to the AUC of the combined model (dashed horizontal lines). Confusion matrix summarizing the per-class accuracies of multiclass classification of (d) lung, bladder, and breast cancer (matched female cohort) with overall model accuracy of 0.73 ± 0.11, and (e) lung, bladder, and prostate cancer (matched male cohort) with overall model accuracy of 0.74 ± 0.13. Detailed cohort characteristics can be found in Figure 3—source data 1. Chance level for the three-class classification corresponds to 0.33 (LuCa: lung cancer; PrCa: prostate cancer; BrCa: breast cancer; BlCa: bladder cancer).
-
Figure 3—source data 1
Characteristics of the matched groups utilized for the analysis presented in Figure 3d and e.
- https://cdn.elifesciences.org/articles/68758/elife-68758-fig3-data1-v1.xlsx
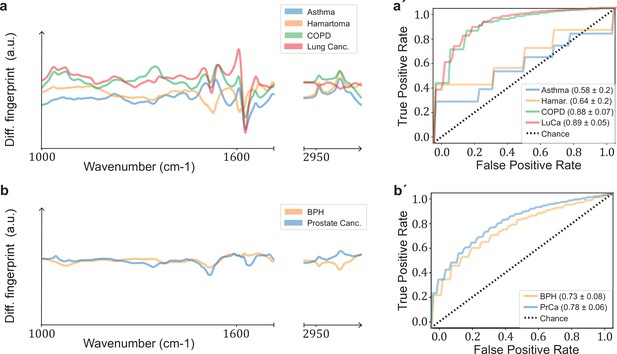
Comparison of signatures from different organ-specific pathologies.
Differential fingerprints for (a) lung-related conditions (asthma, lung hamartoma, chronic obstructive pulmonary disease [COPD], lung cancer) and (b) prostate-related pathologies (benign prostate hyperplasia [BPH], prostate cancer). Receiver operating characteristic (ROC) curves for (a′) lung and (b′) prostate pathologies. All comparisons are against non-symptomatic references. The characteristics of the cohort used for this analysis are given in Figure 3—figure supplement 1—source data 1.
-
Figure 3—figure supplement 1—source data 1
Characteristics of the matched groups utilized for the analysis presented in Figure 3—figure supplement 1.
- https://cdn.elifesciences.org/articles/68758/elife-68758-fig3-figsupp1-data1-v1.xlsx
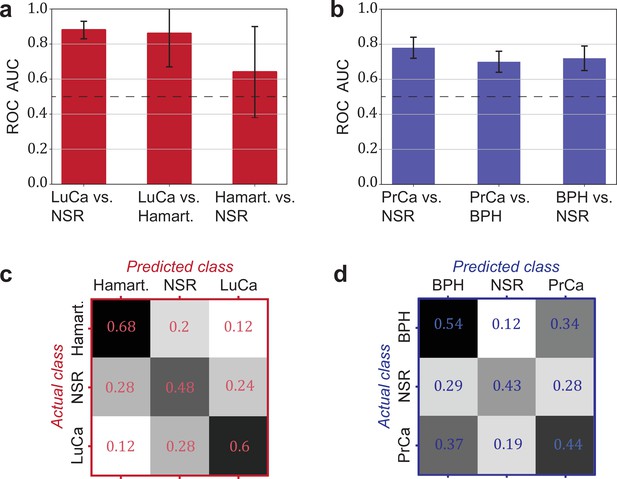
Detection efficiency of benign conditions and multiclass classification.
(a) Pairwise classification performance results between lung cancer (LuCa), hamartoma (Hamart.) and non-symptomatic reference group (NSR) with overall model accuracy of 0.46 ± 0.18, and (b) pairwise classification performance between prostate cancer (PrCa), benign prostate hyperplasia (BPH), and NSR with overall model accuracy of 0.43 ± 0.06. The error bars show the standard deviation of the individual results of the cross-validation. Confusion matrix summarizing the per-class accuracies of multiclass classification in (c) the LuCa cohort and (d) the PrCa cohort. The characteristics of the cohort used for this analysis are given in Figure 4—source data 1. Chance level for the three-class classification corresponds to 0.33.
-
Figure 4—source data 1
Characteristics of the matched groups utilized for the analysis presented in Figure 4.
- https://cdn.elifesciences.org/articles/68758/elife-68758-fig4-data1-v1.xlsx
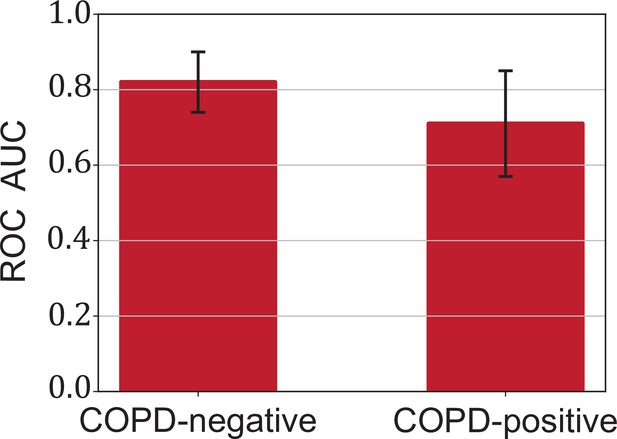
Influence of chronic obstructive pulmonary disease (COPD) in lung cancer (LuCa) detection.
Classification performance of LuCa vs. mixed references as a function of the COPD status. The characteristics of the cohort used for this analysis are given in Figure 4—figure supplement 1—source data 1.
-
Figure 4—figure supplement 1—source data 1
Characteristics of the matched groups utilized for the analysis presented in Figure 4—figure supplement 1.
- https://cdn.elifesciences.org/articles/68758/elife-68758-fig4-figsupp1-data1-v1.xlsx
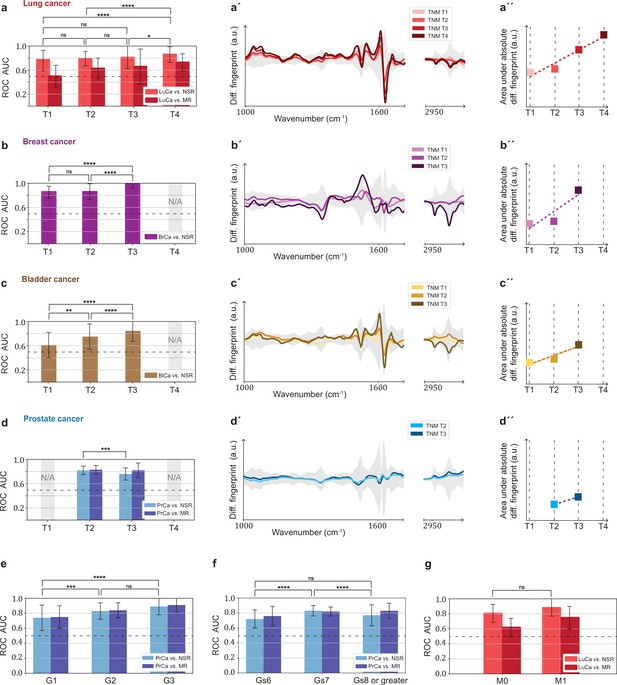
Efficiency of binary classification and infrared spectral changes in dependence of tumour progression.
(a–d) Binary classification performance of lung, breast, bladder, and prostate cancer against references as a function of T-classification (of TNM-staging). (a′–d′) Differential fingerprints in relation with the tumour size (TNM class T) for all four cancer entities. (a′′–d′′) Area under the absolute differential fingerprints in relation with the tumour size for all dour cancer entities. The y-axes of the diagrams in the panels (a'–d') and (a''–d'') each have the same linear scaling, thus directly comparable. (e) Classification performance of prostate cancer versus references as a function of tumour grade score. (f) Classification performance of prostate cancer as a function of the Gleason score (Gs). (g) Classification performance of lung cancer versus references as a function of the metastasis status. The detailed cohort breakdown and classification results are given as Figure 5—source data 1, Figure 5—source data 2, Figure 5—source data 3, Figure 5—source data 4. Some cohorts did not include sufficient number of participants so that a reliable machine learning model could not be built and were therefore not evaluated. LuCa: lung cancer; PrCa: prostate cancer; BrCa: breast cancer; BlCa: bladder cancer; NSR: non-symptomatic references; MR: mixed references; n.s.: not significant; *p<10–2; **p<10–3; ***p<10–4; ****p<10–5; The error bars show the standard deviation of the individual results of the cross-validation.
-
Figure 5—source data 1
Characteristics of the matched groups utilized for the analysis presented in Figure 5a-d, a'-d' and a"-d".
- https://cdn.elifesciences.org/articles/68758/elife-68758-fig5-data1-v1.xlsx
-
Figure 5—source data 2
Characteristics of the matched groups utilized for the analysis presented in Figure 5e.
- https://cdn.elifesciences.org/articles/68758/elife-68758-fig5-data2-v1.xlsx
-
Figure 5—source data 3
Characteristics of the matched groups utilized for the analysis presented in Figure 5f.
- https://cdn.elifesciences.org/articles/68758/elife-68758-fig5-data3-v1.xlsx
-
Figure 5—source data 4
Characteristics of the matched groups utilized for the analysis presented in Figure 5e.
- https://cdn.elifesciences.org/articles/68758/elife-68758-fig5-data4-v1.xlsx
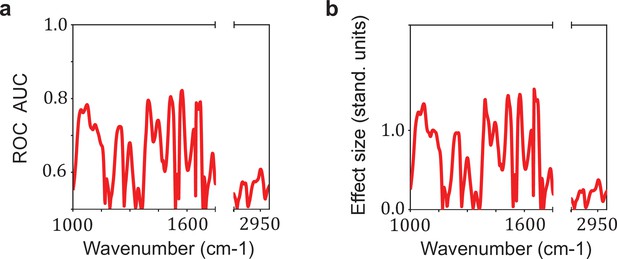
Relation between the effect size and the area under the receiver operating characteristic curve (AUC) per wavenumber.
Comparison between (a) the AUC per wavenumber and (b) the effect size per wavenumber. The effect size is defined as the standardized difference between the sample means of cases and references, also known as Cohen’s d. The AUC per wavenumber is calculated using the U statistic of Mann–Whitney U test by the relation AUC = U/(n1 * n2). This example was performed for the comparison lung cancer (LuCa) vs. non-symptomatic references (NSR).
Tables
Detection efficiency for different binary classifications.
Different cancer types were compared to each other, as well as the impact of using different reference groups was analysed. Detailed cohort characteristics can be found in Figure 2—source data 1 (NSR: non-symptomatic references; MR: mixed references; SR: symptomatic references; AUC: area under the receiver operating characteristic curve; *sensitivity and specificity values are obtained by minimizing the distance of the receiver operating characteristic [ROC] curve to the upper-left corner).
Clinical question for binary classification | # of Individuals | AUC | Sensitivity/specificity* | sensitivity at95% specificity |
---|---|---|---|---|
Lung cancer vs. NSR | 214/193 | 0.89 ± 0.05 | 0.86/0.79 | 0.45 |
Lung cancer vs. MR | 214/208 | 0.77 ± 0.06 | 0.72/0.67 | 0.36 |
Lung cancer vs. SR | 214/143 | 0.74 ± 0.07 | 0.67/0.71 | 0.24 |
Prostate cancer vs. NSR | 278/278 | 0.78 ± 0.06 | 0.71/0.71 | 0.36 |
Prostate cancer vs. MR | 278/278 | 0.75 ± 0.06 | 0.71/0.68 | 0.23 |
Prostate cancer vs. SR | 278/278 | 0.70 ± 0.06 | 0.65/0.68 | 0.20 |
Breast cancer vs. NSR | 161/161 | 0.88 ± 0.06 | 0.82/0.81 | 0.35 |
Bladder cancer vs. NSR | 118/118 | 0.79 ± 0.09 | 0.72/0.73 | 0.23 |
LuCa – lung cancer, BrCa – breast cancer, BlCa – bladder cancer, PrCa – prostate cancer.
LuCa vs. | BrCa vs. | BlCa vs. | PrCa vs. | |
---|---|---|---|---|
MR (Study Site 1) | 0.96 ± 0.02 | 0.71 ± 0.07 | 0.98 ± 0.01 | 0.77 ± 0.12 |
MR (Study Site 2) | 0.72 ± 0.05 | 0.88 ± 0.08 | 0.84 ± 0.09 | 0.62 ± 0.20 |
MR (Study Site 3) | 0.92 ± 0.03 | 0.86 ± 0.04 | 0.77 ± 0.06 | 0.74 ± 0.04 |