Multiple timescales of sensory-evidence accumulation across the dorsal cortex
Figures
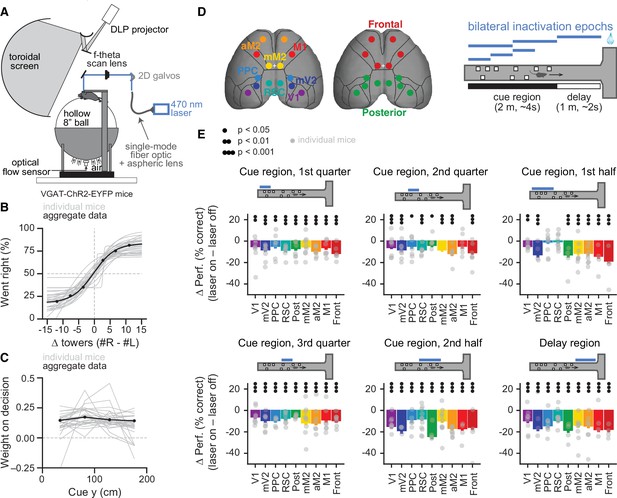
Temporally specific inactivation of multiple dorsal cortical regions during performance of a virtual reality-based evidence-accumulation task.
(A) Schematics of the experimental setup. (B) Psychometric functions for control trials, showing the probability of right-side choice as a function of the strength of right sensory evidence, ∆ towers (#R – #L). Thin gray lines: best fitting psychometric functions for each individual mouse (n=28). Black circles: aggregate data (n=108,940 trials), black line: fit to aggregate data, error bars: binomial confidence intervals. (C) Logistic regression curves for the weight of sensory evidence from four equally spaced bins on the final decision, from control trials. Thin gray lines: individual animals, thick black line: aggregate data, error bars: ± SD from 200 bootstrapping iterations. (D) Experimental design. We bilaterally inactivated seven dorsal cortical areas, alone or in combination, yielding a total of nine area sets, while mice performed the accumulating towers task. Bilateral inactivation happened during one of six regions in the maze spanning different parts of the cue region or delay. We thus tested a total of 54 area-epoch combinations. (E) Effects of sub-trial inactivation on overall performance during all 54 area-epoch combinations. Each panel shows inactivation-induced change in overall % correct performance for each inactivation epoch, for data pooled across mice. Error bars: SD across 10,000 bootstrapping iterations. Black circles indicate significance according to the captions on the leftmost panel. Light gray circles indicate data from individual mice (n=4–11, see Figure 1—source data 1 for details about n per condition).
-
Figure 1—source data 1
Numbers of mice, sessions, and trials for each of the 54 experimental conditions.
Last line shows the number of unique mice and trials across all experiments, as conditions were partially overlapping for a given mouse and behavioral session.
- https://cdn.elifesciences.org/articles/70263/elife-70263-fig1-data1-v1.docx
-
Figure 1—source data 2
Source data for plots in Figure 1.
- https://cdn.elifesciences.org/articles/70263/elife-70263-fig1-data2-v1.zip
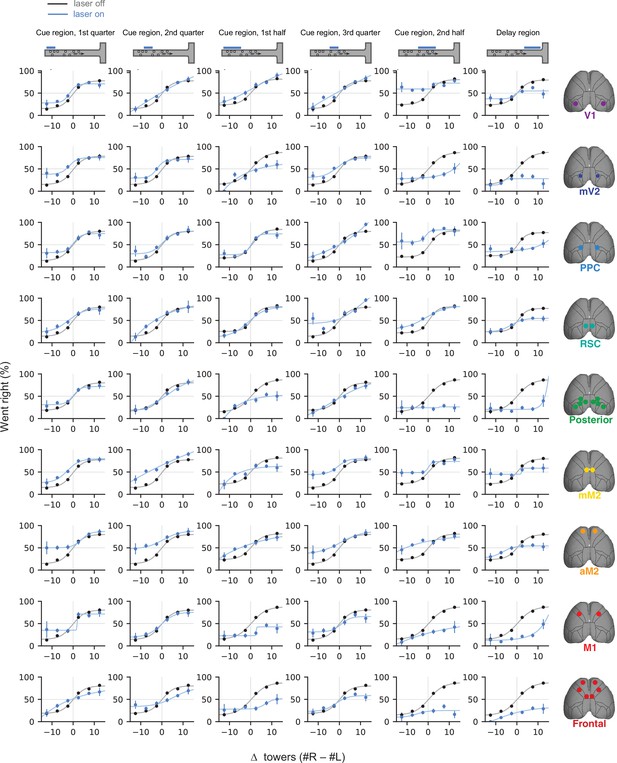
Effects of sub-trial inactivation on psychometric functions during all 54 area-epoch combinations.
Black lines show control trials and blue lines show inactivation trials, for data pooled across mice. Error bars, binomial confidence intervals. Lines are best fitting psychometric functions.
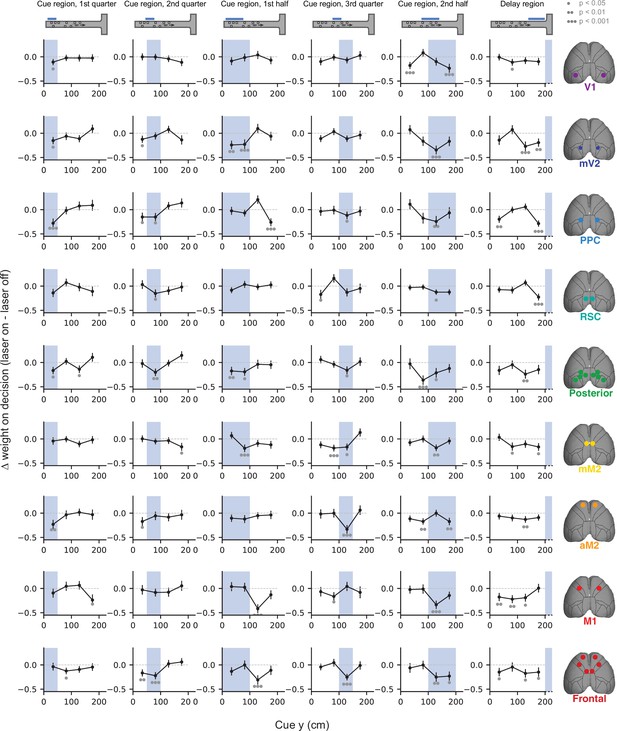
Effects of sub-trial inactivation on evidence-weighting functions during all 54 area-epoch combinations.
Black lines show the inactivation-induced change in evidence weights (laser on – laser off), and shaded areas indicate inactivation epoch. Data were pooled across mice. Error bars, SD across 10,000 bootstrapping iterations. Gray circles indicate statistical significance according to the caption on top.
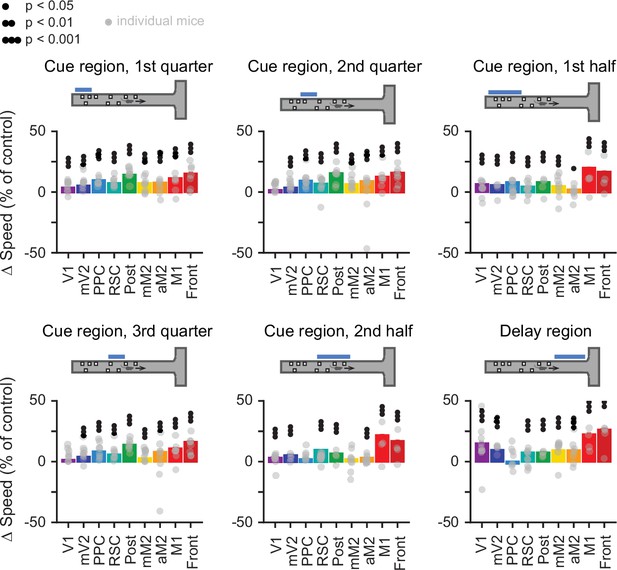
Inactivation of cortical areas moderately increases running speeds in some inactivation conditions.
Effects of sub-trial inactivation on running speed during all 54 area-epoch combinations. Each panel shows inactivation-induced change in speed during the laser-on epoch or equivalent maze regions in control trials. Error bars: SD across 10,000 bootstrapping iterations, for data pooled across mice. Black circles indicate statistical significance according to the caption on top. Gray circles: individual mice.
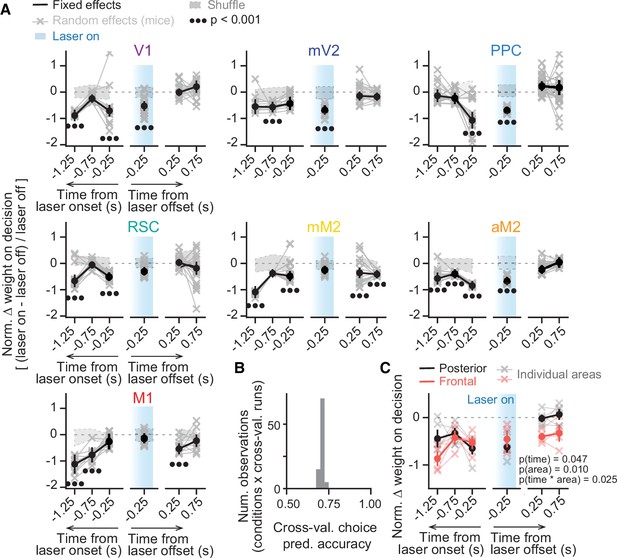
Inactivating different cortical areas leads to evidence-accumulation deficits on distinct timescales.
(A) Results from mixed-effects logistic regression models fit to inactivation data from different areas, combined across mice and inactivation epochs, with 10-fold cross-validation. For each area, we plot normalized evidence weights for inactivation trials, such that 0 means no difference from control, and –1 indicates complete lack of evidence weight on decision. Net evidence (#R – #L towers) was binned in time (0.5 s time bins) and aligned to either laser onset or offset. Coefficients were extracted from the model with highest cross-validated accuracy. Thin gray lines and crosses, mouse random effects (primary visual cortex [V1]: n=15, medial secondary visual cortex [mV2]: n=14, posterior parietal cortex [PPC]: n=20, retrosplenial cortex [RSC]: n=21, posteromedial portion of the premotor cortex [mM2]: n=15, anterior portion of the premotor cortex [aM2]: n=15, primary motor cortex [M1]: n=11; note that we omitted 10/666 random effect outliers outside the 1st–99th percentile range for clarity, but they were still considered in the analysis). The zero-mean random effects were added to the fixed effects for display only. Error bars, ± SEM on coefficient estimates. Black circles below the data points indicate statistical significance from t-tests, with false discovery rate correction. We also imposed an additional significance constraint that the coefficient ± SEM does not overlap with ± SD intervals for coefficients for models fit to shuffled data (gray shaded areas, see Materials and methods for details). (B) Distribution of model prediction accuracy, defined as the proportion of correctly predicted choices in 10% of the data not used to fit the model (n=9 areas × 10 cross-validation runs). (C) Comparison of the inactivation effects between areas in the posterior (V1, mV2, PPC, RSC) and frontal cortex (mM2, aM2, M1). Thin lines with crosses, individual areas. Thick lines and error bars, mean ± SEM across areas. p-values are from a two-way ANOVA with repeated measures, with time bins and area group as factors.
-
Figure 2—source data 1
Source data for plots in Figure 2.
- https://cdn.elifesciences.org/articles/70263/elife-70263-fig2-data1-v1.zip
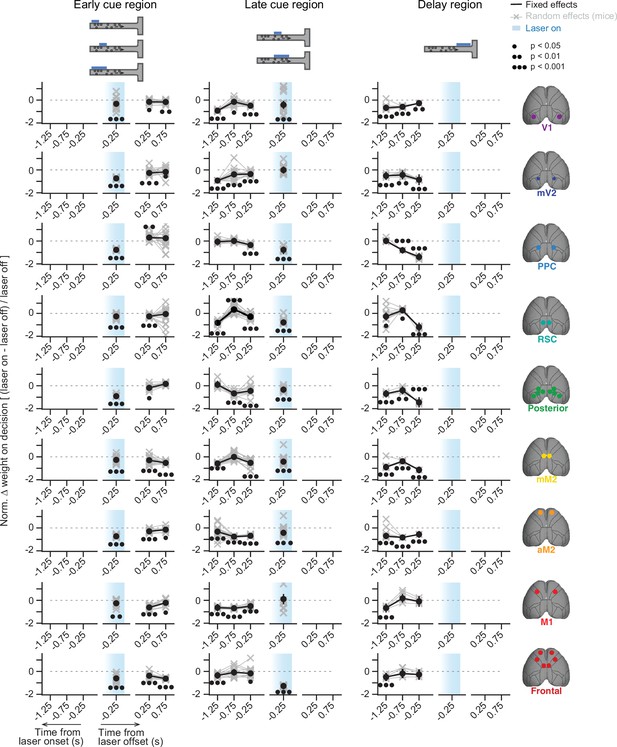
Effects of inactivation on sensory-evidence weights across trial time appear to depend on when the inactivation occurred, for a subset of areas.
Results from mixed-effects logistic regression models fit to inactivation data from different areas (rows), combined across mice and subsets of inactivation epochs (columns, captions on top), with 10-fold cross-validation. For each area, we plot normalized evidence weights for inactivation trials, such that 0 means no difference from control, and –1 indicates complete lack of evidence weight on decision. Net evidence (#R – #L towers) was binned in time (0.5 s time bins) and aligned to either laser onset or offset. Coefficients were extracted from the model run with highest cross-validated accuracy. Thin gray lines and crosses, mouse random effects (note that we omitted random effect outliers outside the 1st – 99th percentile range for clarity, but they were still considered in the analysis). Error bars, ± SEM on coefficient estimates. Black circles below the data points indicate statistical significance from t-tests, with false discovery rate correction. V1, primary visual cortex; mV2, medial secondary visual cortex; PPC, posterior parietal cortex; RSC, retrosplenial cortex; mM2, posteromedial portion of the premotor cortex; aM2, anterior portion of the premotor cortex; M1, primary motor cortex.
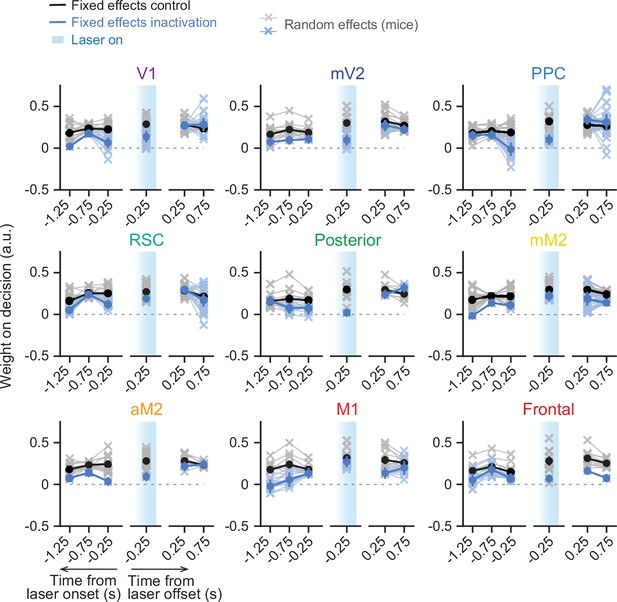
Sensory-evidence weights across trial time plotted separately for control and laser trials.
Results from mixed-effects logistic regression models fit to inactivation data from different areas, combined across mice and inactivation epochs, with 10-fold cross-validation. For each area, we plot evidence weights for control (black) and inactivation trials (blue). Net evidence (#R – #L towers) was binned in time (0.5 s time bins) and aligned to either laser onset or offset. Coefficients were extracted from the model run with highest cross-validated accuracy. Thin gray lines and crosses, mouse random effects (primary visual cortex [V1]: n=15, medial secondary visual cortex [mV2]: n=14, posterior parietal cortex [PPC]: n=20, retrosplenial cortex [RSC]: n=21, posterior, n=11, posteromedial portion of the premotor cortex [mM2]: n=15, anterior portion of the premotor cortex [aM2]: n=15, primary motor cortex [M1]: n=11, frontal, n=11). Error bars, ± SEM on coefficient estimates.
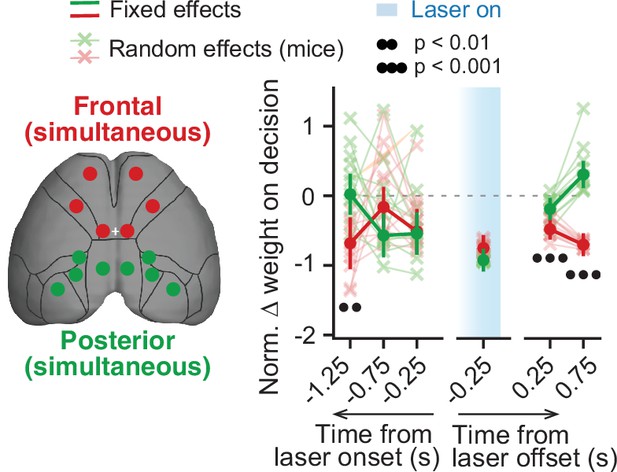
Simultaneous inactivation of frontal or posterior cortical areas confirms distinct contributions to sensory-evidence-based decisions.
Comparison of the effect of simultaneous posterior or frontal cortical inactivation on the use of sensory evidence as recovered from the mixed-effects logistic regression models combined across mice and inactivation epochs, with 10-fold cross-validation. For each area, we plot normalized evidence weights for inactivation trials as in Figure 2. Thin gray lines and crosses, mouse random effects (posterior: n=11, frontal: n=11). Error bars, ± SEM on coefficient estimates. Black circles below the data points indicate p-values from z-tests on the coefficients, with false discovery rate correction (captions on top, see Materials and methods for details).
-
Figure 3—source data 1
Source data for plots in Figure 3.
- https://cdn.elifesciences.org/articles/70263/elife-70263-fig3-data1-v1.zip
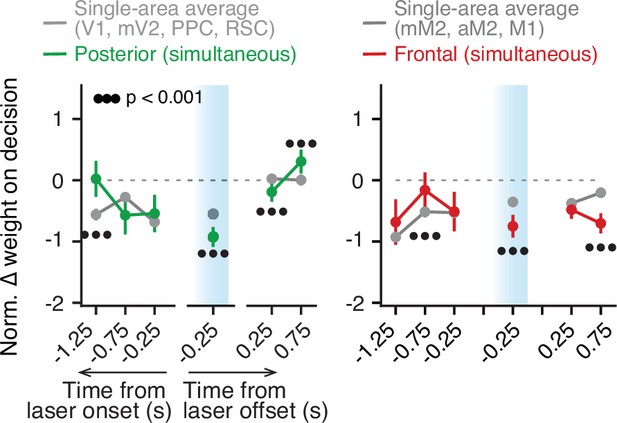
Sensory-evidence information from different areas is combined unevenly.
Comparison between the effects of simultaneous inactivation of either posterior (left) or frontal (right) areas and the average of when the areas were individually inactivated. Error bars, ± SEM for model coefficient estimates. For the data averaged across areas, error bars correspond to the pooled SEM across conditions. Black circles below data points indicate p-values from z-tests on the coefficients, with false discovery rate correction. V1, primary visual cortex; mV2, medial secondary visual cortex; PPC, posterior parietal cortex; RSC, retrosplenial cortex; mM2, posteromedial portion of the premotor cortex; aM2, anterior portion of the premotor cortex; M1, primary motor cortex.
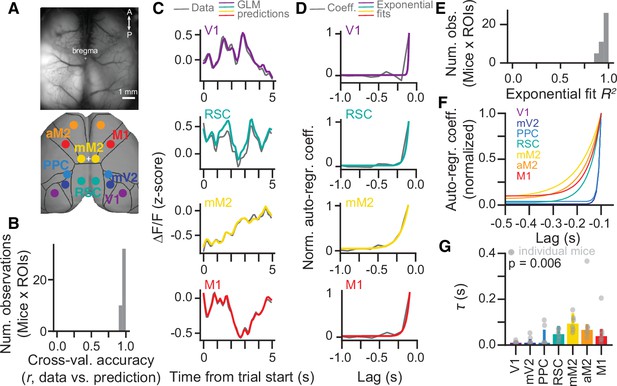
A hierarchy of activity timescales during evidence accumulation.
(A) Top: Example widefield imaging field of view showing GCaMP6f fluorescence across the dorsal cortex. Bottom: Approximate correspondence between the field of view and regions of interest (ROIs) defined from the Allen Brain Atlas, ccv3. (B) Distribution of cross-validated accuracies across mice (n=6, sessions for each mouse are averaged) and ROIs (n=7, averaged across hemispheres). (C) Example of actual ∆F/F (gray) and model predictions (colored lines) for the first 5 s of the same held-out single trial, and four simultaneously imaged ROIs. Traces are convolved with a 1-SD Gaussian kernel for display only. (D) Auto-regressive model coefficients as a function of time lags for an example imaging session and four example ROIs. Gray, coefficient values. Colored lines, best fitting exponential decay functions. (E) Distribution of R2 values for the exponential fits across mice (n=6, sessions for each mouse are averaged) and ROIs (n=7, averaged across hemispheres). (F) Exponential decay functions for all seven cortical areas, fitted to the average across mice (n=6). (G) Median time constants extracted from the exponential decay fits, for each area. Error bars, interquartile range across mice (n=6). p-value is from a one-way ANOVA with repeated measures with ROIs as factors. Light gray circles indicate data from individual mice.
-
Figure 4—source data 1
Source data for plots in Figure 4.
- https://cdn.elifesciences.org/articles/70263/elife-70263-fig4-data1-v1.zip
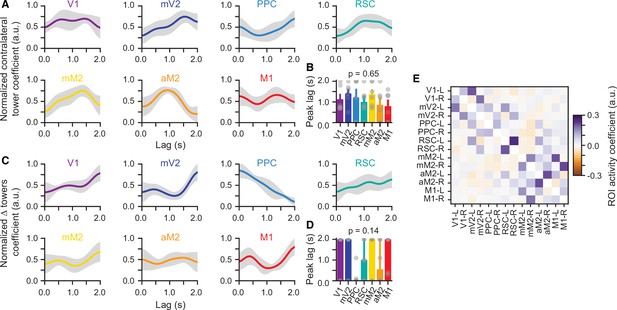
Time course of sensory-evidence predictors in the encoding model of widefield Ca2+ dynamics does not significantly differ between cortical areas.
(A) Average normalized encoding model coefficients for contralateral-tower responses as a function of time lag for each region of interest (ROI). Shaded areas, ± SEM across mice (n=6). (B) Time of peak for contralateral tower coefficients. Gray circles: individual mice, bars: median, error bars: interquartile range. p-value is from a one-way ANOVA with repeated measures. (C–D). Same as (A) and (B), for coefficients for cumulative ∆ towers (#R – #L), taken from left hemisphere ROIs. (E) Coupling coefficients for ROI activity predictors. For each ROI (rows), shown are the average coefficients (n=6 mice) for the predictors consisting of zero-lag activity of the other simultaneously imaged ROIs. Note that the diagonal elements are not defined since zero-lag self-activity predictors were not in the model. Data from the somatosensory cortex ROI has been omitted for symmetry with the inactivation data. L and R indicate left and right hemispheres, respectively.
Tables
Reagent type (species) or resource | Designation | Source or reference | Identifiers | Additional information |
---|---|---|---|---|
Mouse line (Mus musculus) | (B6.Cg-Tg(Slc32a1-COP4*H134R/EYFP)8Gfng/J) | The Jackson Laboratory | JAX: 014548 | A.k.a VGAT-ChR2-EYFP |
Mouse line (Mus musculus) | IgS6tm93.1(tetO-GCaMP6f)Hze Tg(Camk2a-tTA)1Mmay/J | The Jackson Laboratory | JAX: 024108 | A.k.a. Ai93-D;CaMKIIα-tTA |
Mouse line (Mus musculus) | B6.129S2-Emx1tm1(cre)Krj/J | The Jackson Laboratory | JAX: 005628 | A.k.a. Emx1-IRES-Cre |
Software, algorithm | Matlab 2015b, 2016b, 2017b | Mathworks | https://www.mathworks.com/products/matlab.html | |
Software, algorithm | ViRMEn | Aronov and Tank, 2014 | https://pni.princeton.edu/pni-software-tools/virmen | |
Software, algorithm | NI DAQmx 9.5.1 | National Instruments | https://www.ni.com/en-us/support/downloads/drivers/download.ni-daqmx.html | |
Software, algorithm | HCImage | Hamamatsu | https://hcimage.com | |
Software, algorithm | Scanning laser control software | Pinto et al., 2019; Pinto, 2019 | https://github.com/BrainCOGS/laserGalvoControl | |
Software, algorithm | Widefield data analysis software | Pinto et al., 2019; Pinto, 2020 | https://github.com/BrainCOGS/widefieldImaging | |
Software, algorithm | Inactivation analysis | This paper, Pinto, 2022 | https://github.com/BrainCOGS/PintoEtAl2020_sub-trial_inact | |
Software, algorithm | Python 3.8 | Python | https://www.python.org | |
Software, algorithm | Numpy 1.18.1 | van der Walt et al., 2011 | https://www.numpy.org | |
Software, algorithm | Scipy 1.4.1 | Virtanen et al., 2020 | https://www.scipy.org | |
Software, algorithm | Deepdish 0.3.4 | University of Chicago, Larsson, 2022 | https://github.com/uchicago-cs/deepdish | |
Software, algorithm | Statsmodels 0.11.0 | Skipper, 2010 | https://www.statsmodels.org | |
Software, algorithm | Matplotlib 3.1.3 | Hunter, 2007 | https://www.matplotlib.org | |
Software, algorithm | Pandas 1.0.1 | McKinney, 2010 | https://www.pandas.pydata.org | |
Software, algorithm | Pingouin 0.3.8 | Vallat, 2018 | https://pingouin-stats.org | |
Software, algorithm | Mat7.3 | Simon Kern, Kern, 2022 | https://github.com/skjerns/mat7.3 | |
Software, algorithm | Pymer4 | Jolly, 2018 |