A unified framework for measuring selection on cellular lineages and traits
Figures
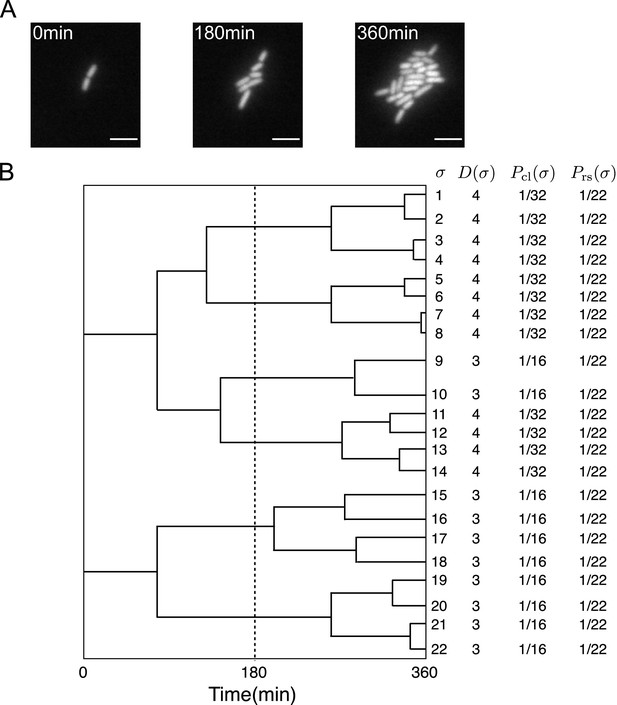
Representative single-cell lineage trees.
(A) Time-lapse images of a growing microcolony of Escherichia coli expressing green fluorescent protein (GFP) from plasmids. Scale bars, 5 μm. (B) Cellular lineage trees for the microcolony in A. Bifurcations in the trees represent cell divisions. denotes cell lineage labels. shows the number of cell divisions in each lineage. and are chronological and retrospective probabilities defined in the main text.
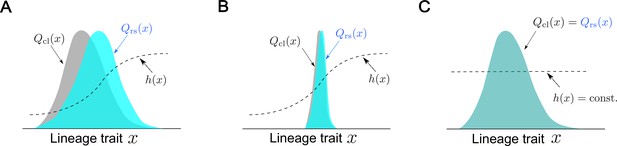
Conceptual illustration of the relationships between fitness landscapes, trait distributions, and selection strength.
(A) Non-uniform fitness landscape and broad trait distribution. The gray distribution represents a chronological distribution of lineage trait ; the cyan distribution represents a retrospective distribution of lineage trait ; and the black dashed line represents a fitness landscape. Due to the non-uniform fitness landscape and the broad chronological distribution, there is trait fitness heterogeneity for selection to act on. The retrospective distribution therefore shifts significantly from the chronological distribution, and the selection strength is large (). (B) Non-uniform fitness landscape and narrow trait distribution. Due to the lack of trait heterogeneity, there is little fitness heterogeneity for selection to act on. The retrospective distribution shifts only slightly from the chronological distribution, and the selection strength is small (). (C) Uniform fitness landscape. When the fitness landscape is constant () across the lineage trait state , there can be no trait fitness heterogeneity regardless of whether the trait distribution itself is narrow or broad. The selection strength is therefore zero ().
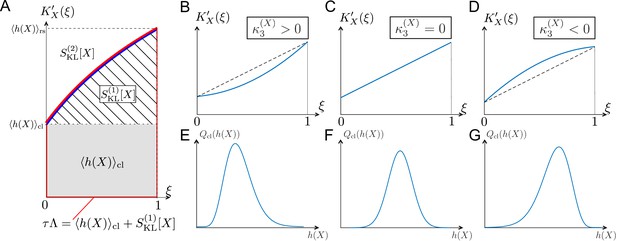
Relationships among chronological distributions’ shape and selection strength measures.
(A) Graphical representation of various fitness and selection strength measures by -plot. Blue curve represents . The area between the horizontal axis and in the interval outlined in red corresponds to population growth . The gray and hatched regions correspond to and , respectively. The area between and corresponds to . (B-D) Representative shapes of depending on . Assuming that the contributions from fourth or higher-order cumulants are negligible, becomes convex downward when (B); a straight line when (C); and convex upward when (D). (E-G) Relationships between third-order fitness cumulant and skewness of chronological distribution .
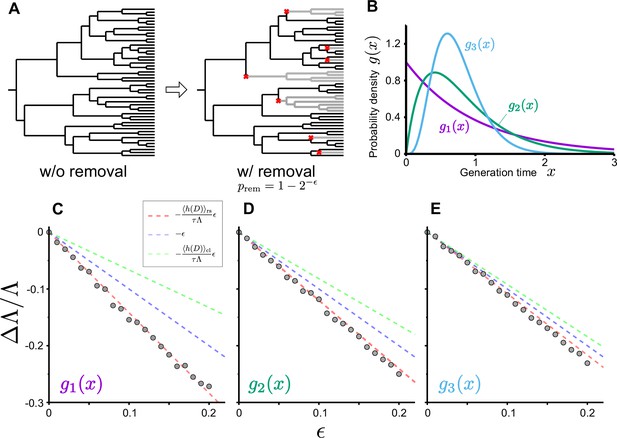
Population growth rate response to cell removal perturbation.
(A) Scheme of random cell removal. Here, we consider the situation where cells were removed probabilistically after each cell division. Red crosses represent cell removal positions in the tree. The lineages after cell removal points disappear from the tree. Consequently, the number of cells at the end time point decreases. (B) Generation time distributions used in the simulation. We assumed that cellular generation time follows gamma distributions in the simulation. We set the shape parameter to either 1 (), 2 (), or 5 (). (C-E) Population growth rate changes by cell removal perturbation. Gray points show the relative changes in population growth rate . Cell removal probability was set to in each condition of perturbation strength . Broken red lines represent the theoretical prediction . The lines of (blue) and (green) are shown for reference. The generation time distributions used in the simulation are for C, for D, and for E.

Response of population growth rate to cell removal perturbation with positive mother-daughter correlations of generation time.
We conducted the simulations of cell population growth with positive mother-daughter correlations (Gray points). We considered the generation time distribution that follows a gamma distribution with shape parameter 2 ( in Figure 4B). We generated correlated generation time of individual cells based on the mother cell’s value with the algorithm in Materials and methods. We randomly removed the individual cells from the population with the probability after each cell division. The broken red lines represent the theoretical prediction of the changes . The lines of (blue) and (green) were also shown for reference. (A) The result with no mother-daughter correlation of generation time (). (B) . (C) . (D) .
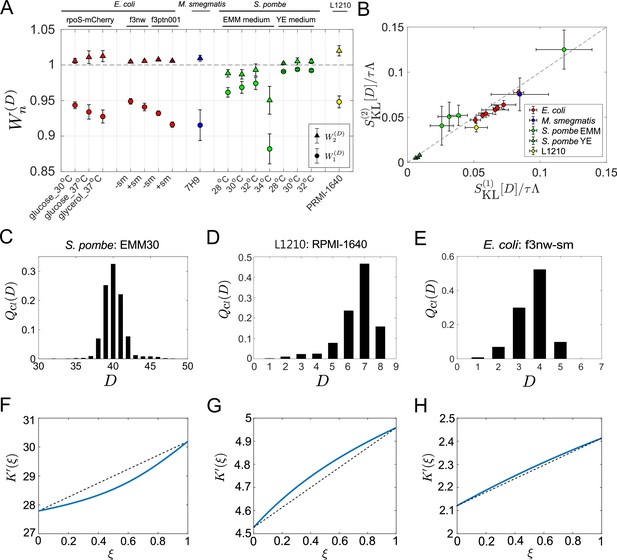
Application of cell lineage statistics to experimental data.
(A) Contributions of the cumulants of a fitness landscape to population growth. and were evaluated for the experimental cell lineage data from E. coli (red), M. smegmatis (blue), S. pombe (green), and L1210 mouse leukemia cells (yellow). The E. coli rpoS-mcherry data were newly obtained in this study (see Materials and methods). The other data were taken from literature: E. coli f3nw and f3ptn001 from Nozoe et al., 2017; M. smegmatis from Wakamoto et al., 2013; S. pombe from Nakaoka and Wakamoto, 2017; and L1210 from Seita et al., 2021. Circles and triangles represent and , respectively. Error bars represent the two standard deviation ranges estimated by resampling the cellular lineages (see Materials and methods). (B) Relationship between and . Colors correspond to the cellular species as in A. The S. pombe data were further categorized into two groups: Circles for the EMM conditions; and triangles for the YE conditions. (C-E) Representative chronological distributions of division count, . (F-H) Graphical representation of . F for S. pombe EMM30; G for L1210 RMPI-1640; and H for E. coli f3nw-sm.

Chronological distributions of division count, .
(A-G) E. coli. (H). M. smegmatis. (I-O) S. pombe. P. L1210 mouse leukemia cells.

Graphical representation of .
A-G. E. coli. H. M. smegmatis. I-O. S. pombe. P. L1210 mouse leukemia cells.
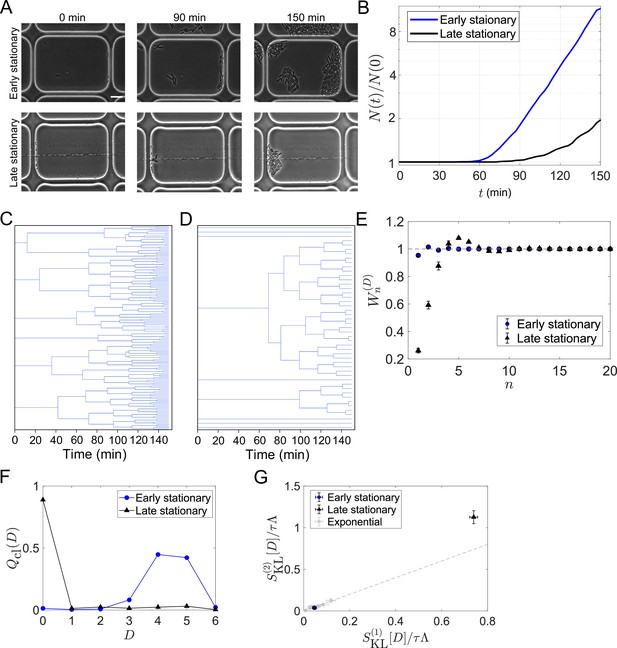
Strong selection in the E.coli population regrowing from a late stationary phase.
(A) Time-lapse images. Cellular regrowing dynamics from early and late stationary phases were observed by time-lapse microscopy. Cells were enclosed in the microchambers etched on coverslips. The top three images show representative images of the cells from an early stationary phase. The bottom three images show the cells from a late stationary phase. Scale bar, 5 μm. (B) Population dynamics. The number of cells at each time point normalized by the initial cell number () was plotted against time was 307 for the early stationary sample and 295 for the late stationary sample. (C, D) Representative cellular lineage trees in the regrowing kineics from the early stationary phase (C) and the late stationary phase (D). The trees correspond to the time-lapse images in A. (E) Cumulative contributions of the cumulants of the fitness landscape to population growth. Error bars represent the two standard deviation ranges estimated by resampling the cellular lineages (see Materials and methods). (F) Chronological distributions of division count . (G) Relationships between and . The blue and black points show the results for the early stationary phase sample and the late stationary phase sample, respectively. Gray points represent the results for the cell populations growing at approximately constant growth rates shown in Figure 5B.
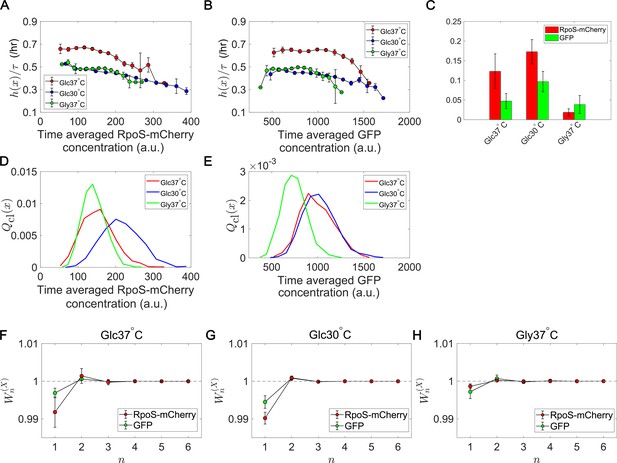
Fitness landscapes and selection strength for RpoS expression levels.
(A) Fitness landscapes for the time-averaged concentration (mean fluorescent intensity) for RpoS-mCherry. The time-averaged mean fluorescent intensity of RpoS-mCherry was adoped as a lineage trait and changes in fitness were plotted against the trait values . Fitness landscapes were scaled by the lineage length (observation duration) . Error bars represent the two standard deviation ranges estimated by resampling the cellular lineages. (B) Fitness landscapes for the time-averaged concentration for GFP. The time-averaged mean fluorescent intensity of GFP was adoped as a lineage trait and changes in fitness were plotted against the trait values . (C) Relative selection strength for the time-averaged concentrations of RpoS-mCherry (red) and GFP (green). (D, E) Chronological distributions for the time-averaged concentrations of RpoS-mCherry (D) and GFP (E). (F-H) Cumulative contributions of fitness cumulants to population growth, , assuming that is either time-averaged concentration of RpoS-mCherry (red) or time-averaged concentration of GFP (green). Error bars represent the two standard deviation ranges estimated by resampling the cellular lineages. Panel F is for the Glucose-37°C condition; Panel G for the Glucose-30°C condition; and Panel H for the Glycerol-37°C condition.

The relative selection strength values for time-averaged RpoS-mCherry and GFP fluorescence intensity compared with the randomized data.
The blue histograms show the distributions of the relative selection strength values calculated from the lineage data in which the correspondences between division counts and trait values are shuffled. The vertical lines indicate the relative selection strength values for the experimental data. A. GFP under the Glucose-37°C condition. B. RpoS-mCherry under the Glucose-37°C condition. C. GFP under the Glucose-30°C condition. D. RpoS-mCherry under the Glucose-30°C condition. E. GFP under the Glycerol-37°C condition. F. RpoS-mCherry under the Glycerol-37°C condition.
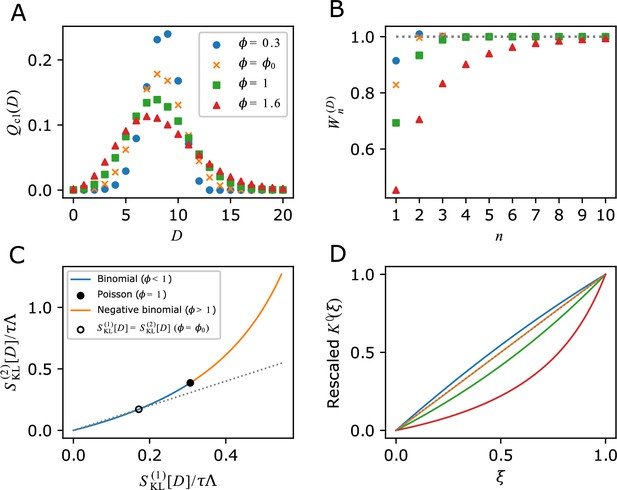
Analytical calculations of and related relations given specific form of division count distributions.
(A) Chronological division count distributions. and are binomial, is Poisson and is negative binomial. is fixed. (B) Cumulative contributions of fitness cumulants. Parameter values are given in panel A legend. (C) The relation between two selection strength measures. Binomial (blue curve), Poisson (closed black circle) and negative binomial (orange curve) are indicated on the single curve plotted using Equations 37 and 38 within the range of . The point where () is indicated by the open black circle. The grey dotted line corresponds to . (D) Convexity of . Y-axis shows a rescaling of according to . The same values of as in A are used; (blue), (orange), (green) and (red). The grey dotted line indicates the case that is a linear function of
Tables
Relationships between and quantities in cellular lineage statistics.
Quantities in lineage statistics | Symbol | Correspondence to | |
---|---|---|---|
Fitness | Population growth | ||
Chronological mean fitness | |||
Retrospective mean fitness | |||
Chronological fitness variance | |||
Retrospective fitness variance | |||
Selection strength | Jeffreys divergence bet. and | ||
KL divergence of from | |||
KL divergence of from | |||
Growth rate gain/loss | Growth rate gain | ||
Additional growth rate loss upon perturbation |
Summary of cellular species, culture conditions, and observation setup used in the experiments in Figure 5.
Species | Label | Strain | Medium | Temperature (°C) | Device | |
---|---|---|---|---|---|---|
E. coli | rpoS-mcherry glucose_30°C | MG1655 F3 rpoS-mcherry /pUA66-PrpsL-gfp | M9 minimal medium +0.2%(w/v) glucose +1/2 MEM amino acids solution (Sigma) | 30 | Microchamber array | This study |
E. coli | rpoS-mcherry glucose_37°C | MG1655 F3 rpoS-mcherry /pUA66-PrpsL-gfp | M9 minimal medium +0.2%(w/v) glucose +1/2 MEM amino acids solution (Sigma) | 37 | Microchamber array | This study |
E. coli | rpoS-mcherry glycerol_37°C | MG1655 F3 rpoS-mcherry /pUA66-PrpsL-gfp | M9 minimal medium +0.1%(v/v) glycerol +1/2 MEM amino acids solution (Sigma) | 37 | Microchamber array | This study |
E. coli | f3nw -sm | F3NW | M9 minimal medium +0.2%(w/v) glucose +1/2 MEM amino acids solution (Sigma)+0.1mM Isopropyl β-D-1 thiogalactopyranoside (IPTG) | 37 | Agar pad | Nozoe et al., 2017 |
E. coli | f3nw +sm | F3NW | M9 minimal medium +0.2%(w/v) glucose +1/2 MEM amino acids solution (Sigma)+0.1 mM Isopropylβ-D-1 thiogalactopyranoside (IPTG)+100 μg/ml streptomycin | 37 | Agar pad | Nozoe et al., 2017 |
E. coli | f3ptn001 -sm | F3/pTN001 | M9 minimal medium +0.2%(w/v) glucose +1/2 MEM amino acids solution (Sigma)+0.1 mM Isopropylβ-D-1 thiogalactopyranoside (IPTG) | 37 | Agar pad | Nozoe et al., 2017 |
E. coli | f3ptn001+sm | F3/pTN001 | M9 minimal medium +0.2%(w/v) glucose +1/2 MEM amino acids solution (Sigma)+0.1 mM Isopropylβ-D-1 thiogalactopyranoside (IPTG)+200 μg/ml streptomycin | 37 | Agar pad | Nozoe et al., 2017 |
M. smegmatis | mc2155 7H9 | mc2155 | Middlebrook 7H9 medium +0.5% albumin +0.2% glucose +0.085% NaCl+0.5% glycerol +0.05% Tween-80 | 37 | Membrane cover | Wakamoto et al., 2013 |
S. pombe | EMM28 | HN0025 | Edinburgh minimal medium +2% (w/v) glucose | 28 | Mother machine | Nakaoka and Wakamoto, 2017 |
S. pombe | EMM30 | HN0025 | Edinburgh minimal medium +2%(w/v) glucose | 30 | Mother machine | Nakaoka and Wakamoto, 2017 |
S. pombe | EMM32 | HN0025 | Edinburgh minimal medium +2%(w/v) glucose | 32 | Mother machine | Nakaoka and Wakamoto, 2017 |
S. pombe | EMM34 | HN0025 | Edinburgh minimal medium +2%(w/v) glucose | 34 | Mother machine | Nakaoka and Wakamoto, 2017 |
S. pombe | YE28 | HN0025 | Yeast extract medium +3%(w/v) glucose | 28 | Mother machine | Nakaoka and Wakamoto, 2017 |
S. pombe | YE30 | HN0025 | Yeast extract medium +3%(w/v) glucose | 30 | Mother machine | Nakaoka and Wakamoto, 2017 |
S. pombe | YE34 | HN0025 | Yeast extract medium +3%(w/v) glucose | 34 | Mother machine | Nakaoka and Wakamoto, 2017 |
L1210 mouse leukemia cell | L1210 RPMI-1640 | L1210 (ATCC CCL-219) | RPMI-1640 medium (Wako)+10% fetal bovine serum (Biosera) under 5% CO2 atmosphere | 37 | Mother machine | Seita et al., 2021 |
Summary of the data used in the analysis in Figure 5.
tstart and tend are the start and end times for the analysis time window .
Species | label | (hr) | (hr) | (hr) | ||
---|---|---|---|---|---|---|
E. coli | rpoS-mcherry glucose_37°C | 5 | 0.95 | 5.95 | 163 | 3989 |
E. coli | rpoS-mcherry glucose_30°C | 8 | 0.95 | 8.95 | 197 | 6173 |
E. coli | rpoS-mcherry glycerol_37°C | 6.5 | 0.95 | 7.45 | 253 | 5825 |
E. coli | f3nw-sm | 5 | 0 | 5 | 305 | 4343 |
E. coli | f3nw +sm | 5 | 0 | 5 | 291 | 3164 |
E. coli | f3ptn001-sm | 5 | 0 | 5 | 984 | 9229 |
E. coli | f3ptn001+sm | 5 | 0 | 5 | 977 | 7429 |
M. smegmatis | mc2155 7H9 | 10 | 1.75 | 11.75 | 39 | 311 |
S. pombe | EMM28 | 167 | 0 | 167 | 1148 | - |
S. pombe | EMM30 | 131 | 0 | 131 | 963 | - |
S. pombe | EMM32 | 123.5 | 0 | 123.5 | 883 | - |
S. pombe | EMM34 | 152 | 0 | 152 | 1078 | - |
S. pombe | YE28 | 108 | 0 | 108 | 1177 | - |
S. pombe | YE30 | 90 | 0 | 90 | 866 | - |
S. pombe | YE34 | 78 | 0 | 78 | 863 | - |
L1210 mouse leukemia cell | L1210 RPMI-1640 | 60 | 0 | 60 | 474 | - |
Reagent type (species) or resource | Designation | Source or reference | Identifiers | Additional information |
---|---|---|---|---|
Recombinant DNA reagent | pUA66-PrpsL-gfp (plasmid) | Zaslaver et al., 2006 | ||
Strain, strain background (Escherichia coli) | MG1655 F3 | Wakamoto lab | MG1655ΔfliCΔfimAΔflu | |
Strain, strain background (Escherichia coli) | MG1655 F3 rpoS- mcherry /pUA66-P rplS-gfp | Wakamoto lab | MG1655ΔfliCΔfimAΔflu rpoS-mcherry /pUA66-PrplS-gfp |