Gene interaction perturbation network deciphers a high-resolution taxonomy in colorectal cancer
Figures
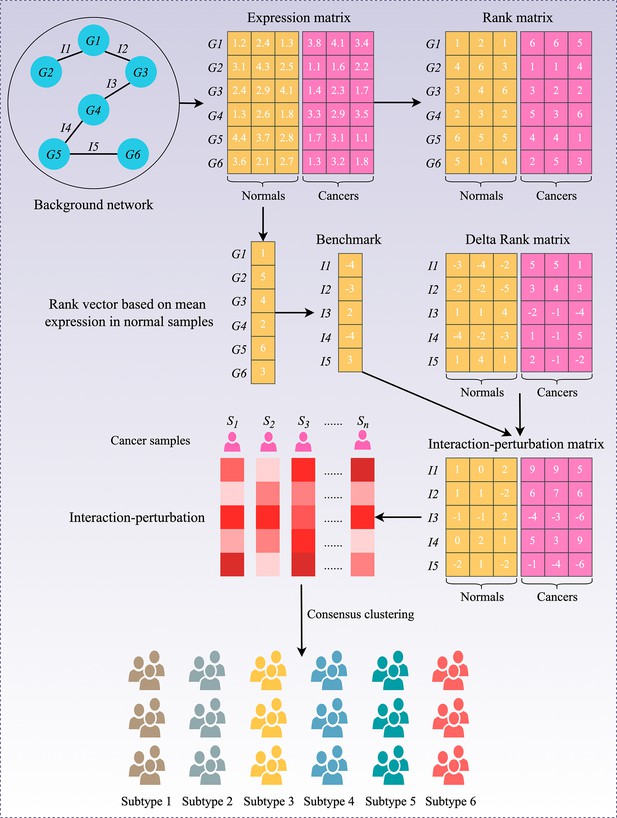
Flowchart of the interaction-perturbation-based program.
As an example, the background network consists of six genes and five interactions. There were three normal samples (yellow) and three cancer samples (pink). A rank matrix was obtained by ranking the genes according to the expression value of each sample. The rank matrix was converted to a delta rank matrix with five rows and six columns representing interactions and samples, respectively. The benchmark delta rank vector was calculated as the delta rank of the average expression value in all normal samples. The interaction-perturbation matrix was obtained by subtracting the benchmark delta rank vector from the delta rank matrix.
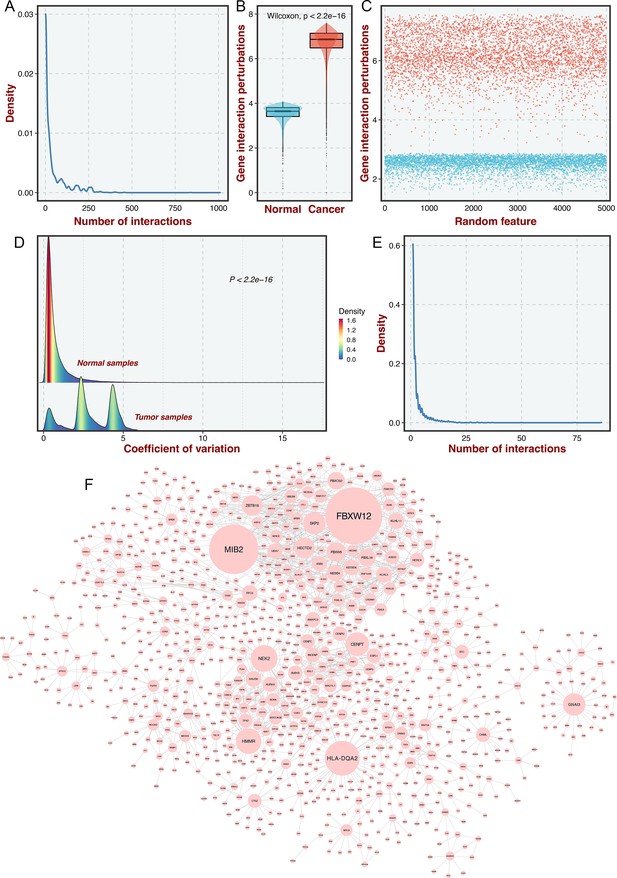
Construction of the gene interaction-perturbation network.
(A) As the number of interactions increased, the density decreased significantly, presenting a power distribution in the background networks. R was computed as the Pearson correlation between log10 (interaction number) and log10 (corresponding frequency), which was used to measure the fitting level of the power law curve. The better the curve fitting level is, the closer R is to 1. (B) The distribution of gene interaction perturbations between normal and tumor samples. (C) The scatterplot for the log2-transformed mean of the interaction perturbations in the 5,000 randomly selected edges in both normal (blue points) and CRC (red points) tissues. The interaction perturbations of normal samples were much denser and less than tumor samples. (D) 92.6% of all 148,942 gene pairs exhibited more dispersion in tumor samples than in normal samples by comparing the coefficient of variation (CV) of interaction perturbations. (E–F) This new network with 1,390 genes and 2,225 interactions also met the scale-free distribution (E) and was visualized (F), the node size represents connectivity.
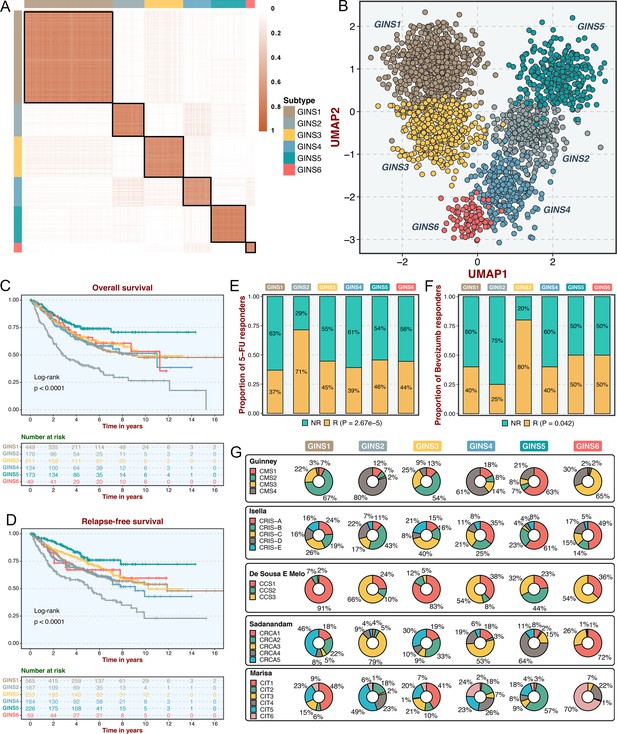
Six CRC subtypes were identified from the gene interaction-perturbation network.
(A) The consensus score matrix of all samples when K achieved 6. A higher consensus score between two samples indicates they are more likely to be grouped into the same cluster in different iterations. (B) The UMAP analysis cast all samples in two-dimensional spatial coordinates, showing good discrimination. (C–D) Kaplan-Meier curves of overall survival and relapse-free survival with log-rank test for six GINS subtypes. Log-rank test. (E–F) Barplots showed the distribution of fluorouracil-based adjuvant chemotherapy (E) and bevacizumab (F) responders in six subtypes. Fisher’s exact test. (G) Pie charts showed the proportion of other CRC subtypes in the current GINS taxonomy.
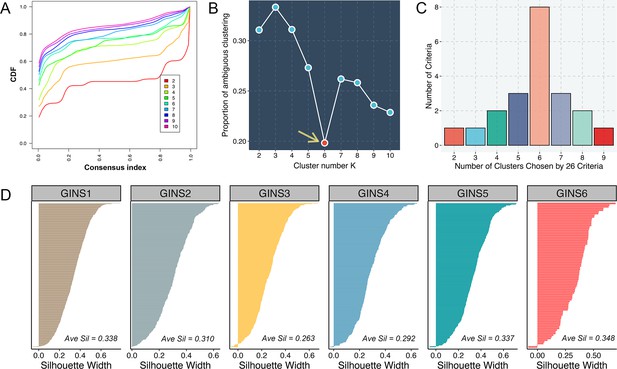
Six CRC subtypes were identified from the gene interaction-perturbation network.
(A) The CDF curves of consensus matrix for each K (indicated by colors). (B) The proportion of ambiguous clustering (PAC) score, a low value of PAC implies a flat middle segment, allowing conjecture of the optimal K (K=6) by the lowest PAC. (C) Recommended number of clusters using 26 criteria of Nbclust package. (D) Silhouette width of samples in each subtype.
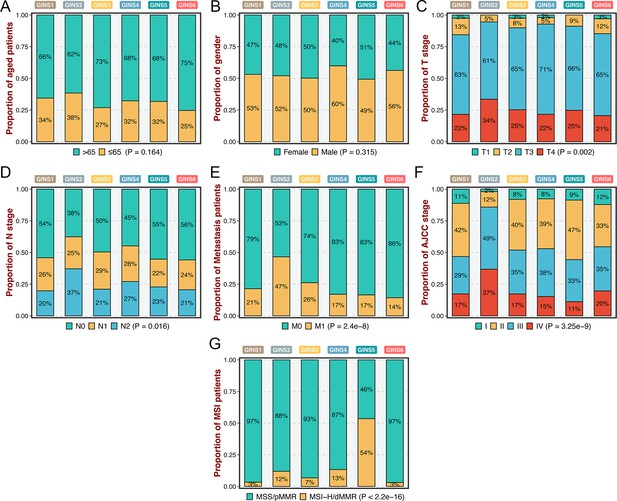
Clinical characteristics of six GINS subtypes.
(A-G) Barplots showed the distribution of age (A), gender (B), T stage (C), N stage (D), M stage (E), AJCC stage (F), and MSI status (G).
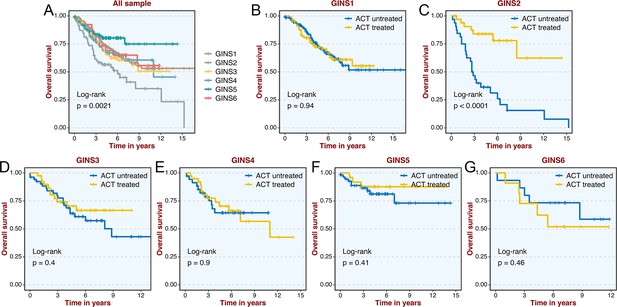
Association of GINS subtypes with ACT after surgery for 585 patients in GSE39582 dataset.
(A–G) Kaplan-Meier curves of overall survival for six GINS subtypes across all (A), GINS1 (B), GINS2 (C), GINS3 (D), GINS4 (E), GINS5 (F), GINS6 (G) samples, respectively.
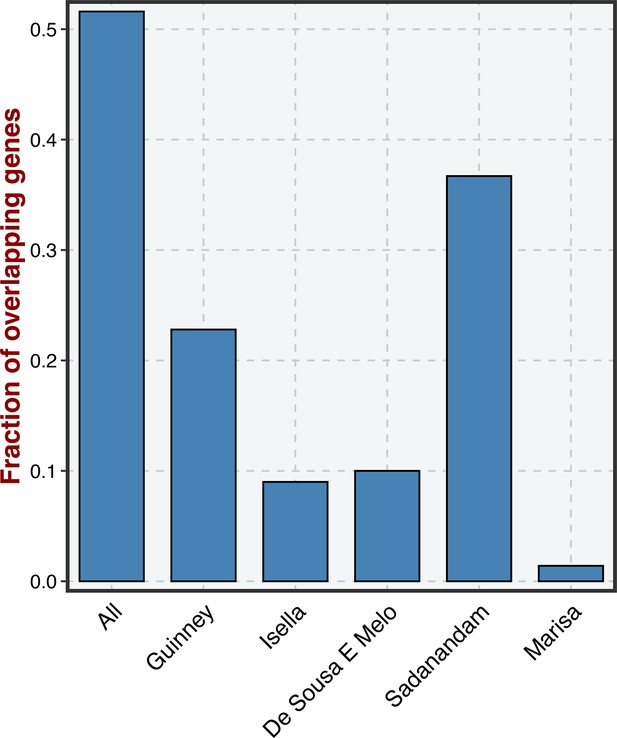
The overlapped genes between our classifier genes and the signature genes of all previous CRC classifications.
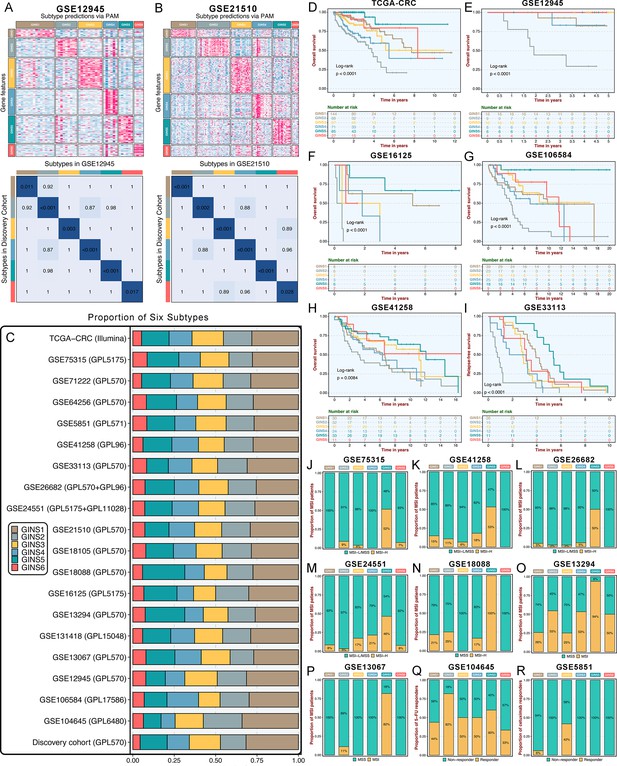
Six subtypes were reproductive and stable in external datasets.
(A-B) The GSE12945 (A) and GSE21510 (B) were assigned in six subtypes according to the classifier. The top and left bars indicated the subtypes. In the heatmap, rows indicated genes from the classifier and columns represent patients. The heatmap was color-coded on the basis of median-centered log2 gene expression levels (red, high expression; blue, low expression). SubMap plots, located in the bottom panel, assessed expressive similarity between corresponding subtypes from two different cohorts. (C) Barplots showed comparable fractions of patients being assigned to each subtype in the discovery cohort and 19 validation datasets. (D–H) Kaplan-Meier curves of overall survival for six GINS subtypes in TCGA-CRC (D), GSE12945 (E), GSE16125 (F), GSE106584 (G), and GSE41258 (H). Log-rank test. (I) Kaplan-Meier curves of relapse-free survival for six GINS subtypes in GSE33113. Log-rank test. (J–P) Barplots showed the distribution of MSI patients across six subtypes in GSE75315 (J), GSE41258 (K), GSE26682 (L), GSE24551 (M), GSE18088 (N), GSE13294 (O), and GSE13067 (P). Fisher’s exact test. (Q–R) Barplots showed the distribution of responders to six subtypes of fluorouracil-based adjuvant chemotherapy in GSE104645 (Q) and cetuximab in GSE5851 (R). Fisher’s exact test.
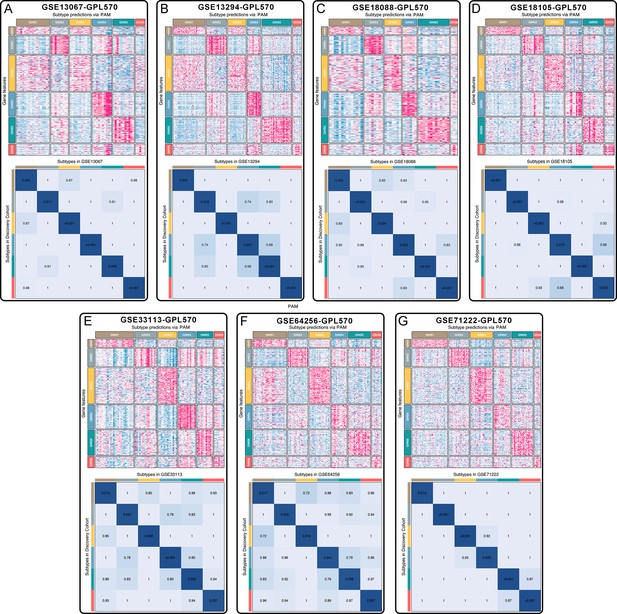
Subtype validation of seven datasets from the same platform (GPL570).
(A-G) Seven datasets, including GSE13067 (A), GSE13294 (B), GSE18088 (C), GSE18105 (D), GSE33113 (E), GSE64256 (F), and GSE71222 (G), were assigned in six subtypes according to the classifier. The top and left bars indicated the subtypes. In the heatmap, rows indicated genes from the classifier and columns represent patients. The heatmap was color-coded on the basis of median-centered log2 gene expression levels (red, high expression; blue, low expression). SubMap plots, located in the bottom panel, assessed expressive similarity between corresponding subtypes from two different cohorts.
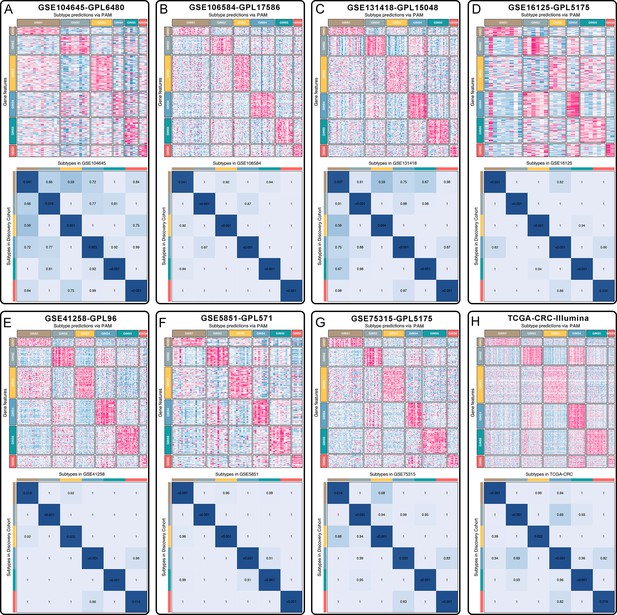
Subtype validation of seven microarrays from different platforms and a RNA-seq dataset.
(A-H) Eight datasets, including GSE104645 (A), GSE106584 (B), GSE131418 (C), GSE16125 (D), GSE41258 (E), GSE5851 (F), GSE75315 (G), and TCGA-CRC (H), were assigned in six subtypes according to the classifier. The top and left bars indicated the subtypes. In the heatmap, rows indicated genes from the classifier and columns represent patients. The heatmap was color-coded on the basis of median-centered log2 gene expression levels (red, high expression; blue, low expression). SubMap plots, located in the bottom panel, assessed expressive similarity between corresponding subtypes from two different cohorts.
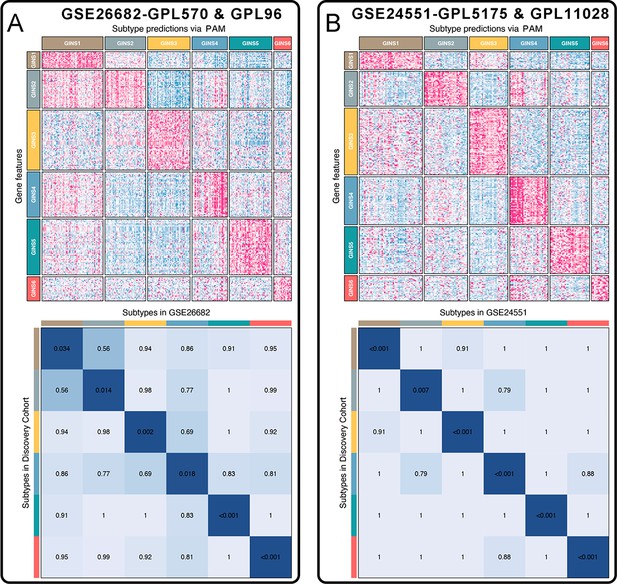
Subtype validation of two microarrays chip with two different platforms.
(A-B) Two datasets, GSE26682 and GSE24551, each chip from two different platforms (GPL570 & GPL96 for GSE26682 and GPL5175 & GPL11028 for GSE24551), were assigned in six subtypes according to the classifier. The top and left bars indicated the subtypes. In the heatmap, rows indicated genes from the classifier and columns represent patients. The heatmap was color-coded on the basis of median-centered log2 gene expression levels (red, high expression; blue, low expression). SubMap plots, located in the bottom panel, assessed expressive similarity between corresponding subtypes from two different cohorts.
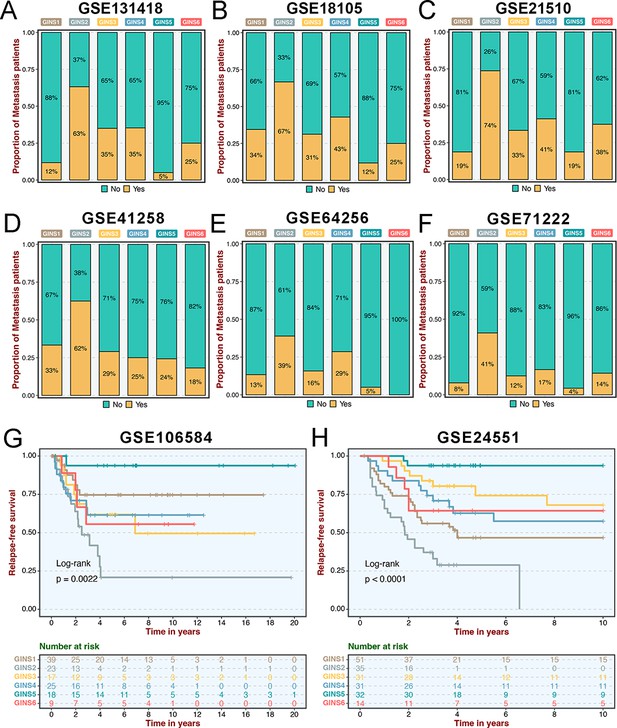
Clinical characteristics of six GINS subtypes in validation datasets.
(A-F) Barplots showed the distribution of metastasis patients to six subtypes in GSE131418 (A), GSE18105 (B), GSE21510 (C), GSE41258 (D), GSE64256 (E), and GSE71222 (F). (G–H) Kaplan-Meier curves of overall survival for six GINS subtypes in GSE106584 (G) and GSE24551 (H).
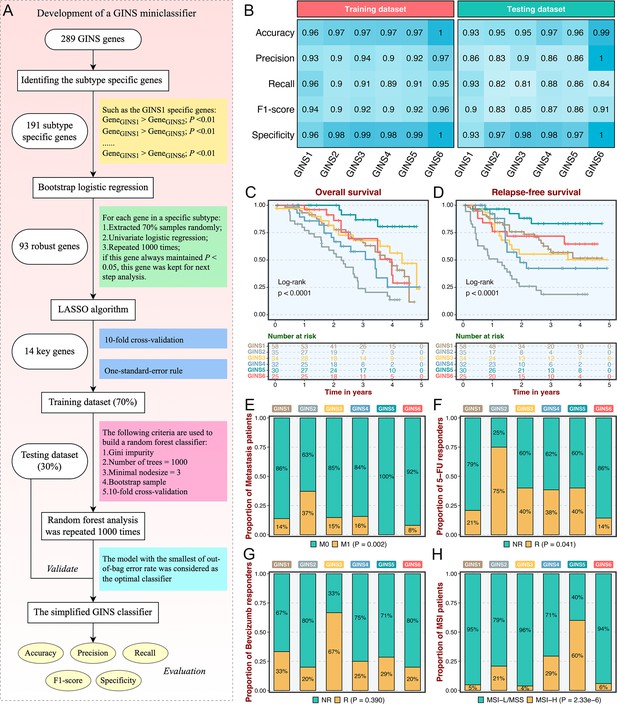
Subtype validation in an in-house clinical cohort.
(A) Overview of the miniclassifier development procedures. (B) Performance of the miniclassifier in the training and testing datasets. (C–D) Kaplan-Meier curves of overall survival and relapse-free survival with log-rank test for six GINS subtypes. Log-rank test. (E–H) Barplots showed the distribution of metastasis patients (E), fluorouracil-based adjuvant chemotherapy responders (F), bevacizumab responders (G), and MSI patients (H) in six subtypes. Fisher’s exact test.
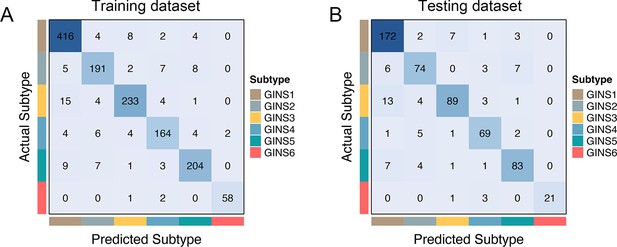
Performance of the miniclassifier.
(A-B) Confusion matrix displayed the general tendency of classification effect in the training (A) and testing (B) datasets, respectively.
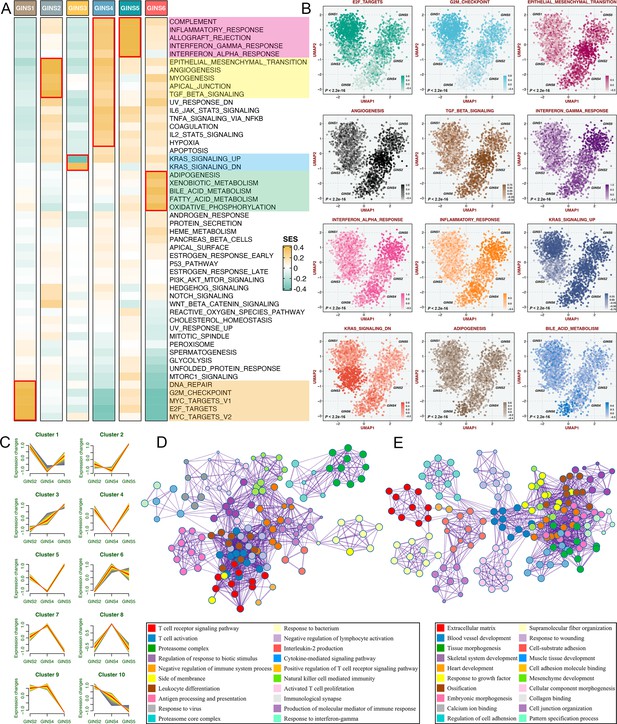
Biological peculiarities of six subtypes.
(A) SSEA-based analysis delineated the biological attributes inherent to GINS subtypes. (B) GSVA further estimated differences in pathway activity across six subtypes. Anova test. (C) Ten gene clusters were obtained via the soft clustering method (Mfuzz) in GINS2/4/5. (D–E) Enrichment analysis of gene cluster 3 (D) and 10 (E).
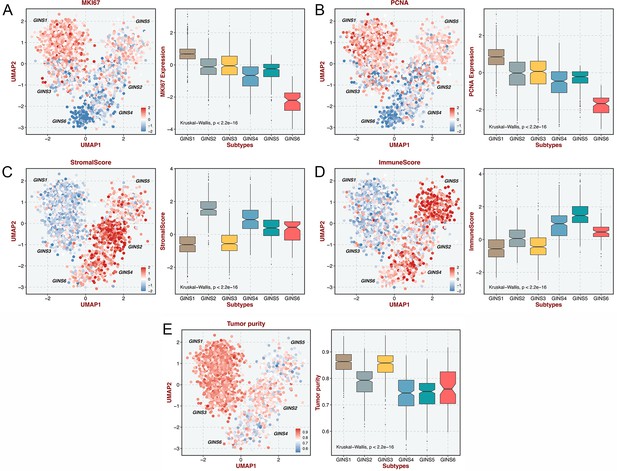
The distribution of MKI67 (A), PCNA (B), stromal score (C), immune score (D), and tumor purity (E) in six subtypes.
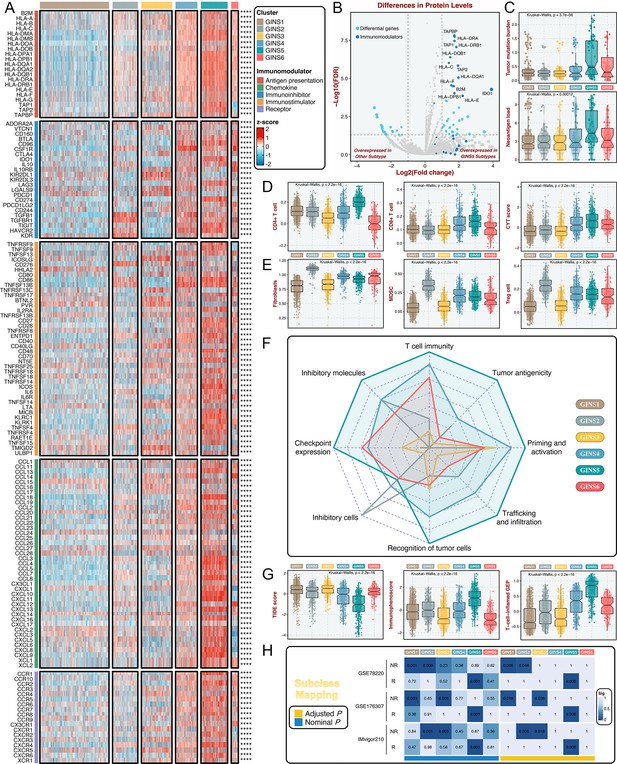
Immune landscape and immunotherapeutic potential of six subtypes.
(A) The expression distribution of 145 immunomodulators among six subtypes. ****p<0.0001. (B) Differential analysis was performed between GINS5 and other subtypes, and 13/26 of immunomodulators were up-regulated in GINS5. (C) The distribution of TMB and NAL score among six subtypes. Kruskal-Wallis test. (D) The distribution of CD4 +T cells, CD8 +T cells, and CYT score among six subtypes. Kruskal-Wallis test. (E) The distribution of fibroblasts, MDSC, and Treg cells among six subtypes. Kruskal-Wallis test. (F) Radar plots showed the immunogram patterns of the six subtypes. Kruskal-Wallis test. (G) The distribution of TIDE score, immunophenoscore, and T-cell-inflamed gene expression profiles (GEP) among six subtypes. Kruskal-Wallis test. (H) SubMap analysis delineated the similar expression pattern between GISN5 tumors and immunotherapeutic responders from three cohorts with treatment annotations, and GINS1/2/3 shared the transcriptional modes with non-responders from 2/3 of immunotherapeutic cohorts. ‘R’ represents responder, whereas ‘NR’ represents non-responder.
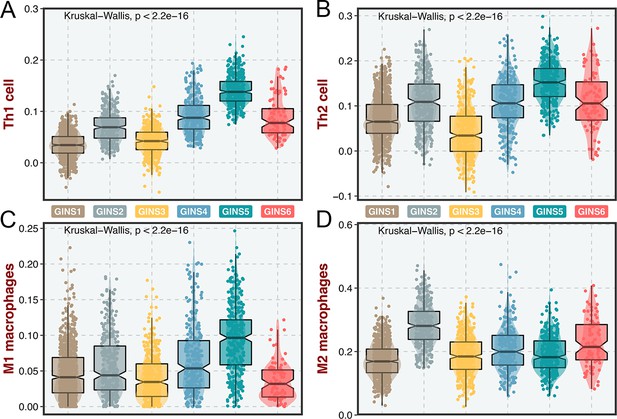
Immune cell infiltrations of six subtypes.
(A-D) The infiltration distribution of Th1 (A), Th2 (B), M1 (C), and M2 (D) cells.
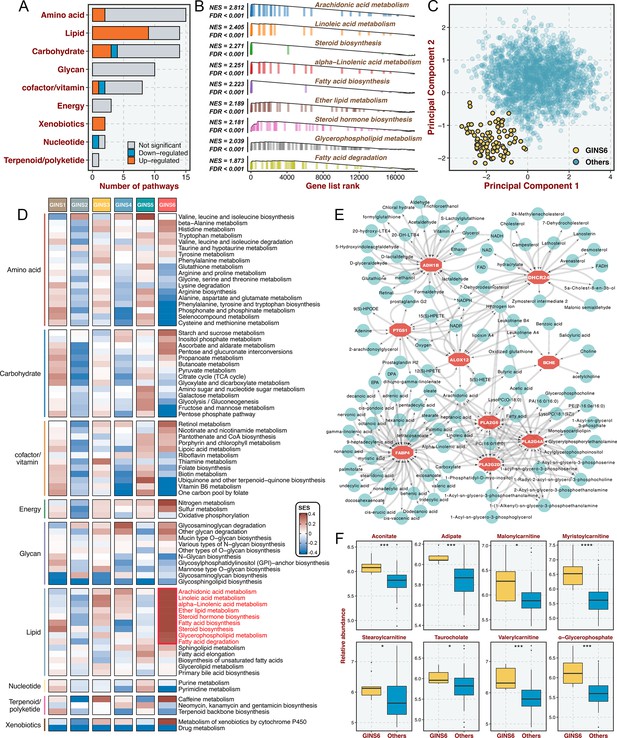
GINS6 tumors conveyed rich lipid metabolisms.
(A) The number of metabolic pathways that was significantly upregulated or downregulated (FDR <0.05) in GINS6 versus the other subtypes among each of nine metabolic categories. (B) GSEA plots of nine lipid metabolism pathways (FDR <0.001). (C) Principal component analysis of all samples in the discovery cohort for the mRNA expression of lipid metabolic genes. (D) SSEA-based framework further confirmed that GINS6 predominantly enriched high-ranking samples with stronger lipid signature scores. (E) Metabolite-protein interaction network (MPIN) was established via nine GINS6-specific genes with broad and tight connections with lipid metabolites. (F) Metabolomics results further demonstrated that GINS6 featured by abundant fatty acids metabolites. Wilcoxon test. *p<0.05, ***p<0.001, ****p<0.0001.
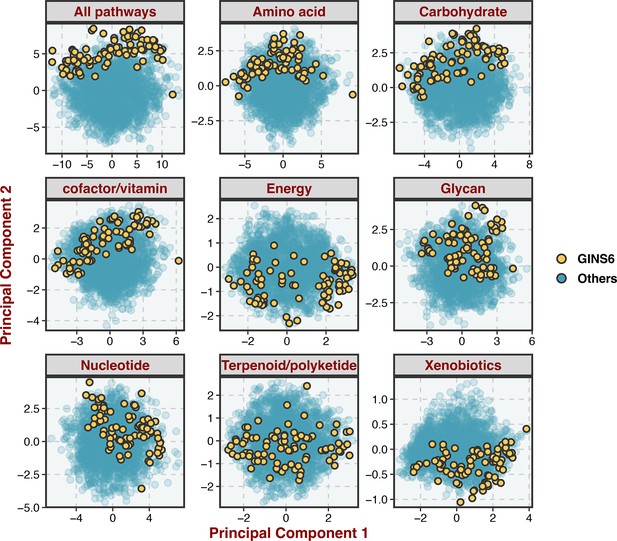
Principal component analysis of all samples in the discovery cohort for the mRNA expression of metabolic genes.
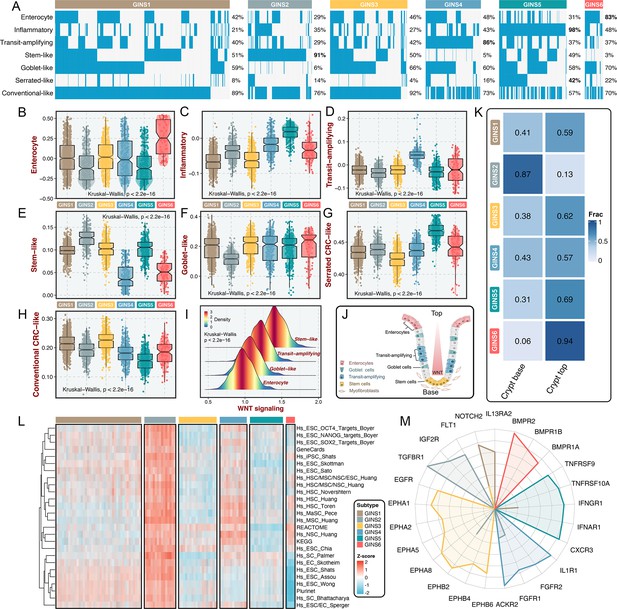
GINS subtypes were associated with cellular phenotypes and autocrine loops.
(A) Supervised approach identified phenotype origins peculiar to individual GINS classes. (B–H) Unsupervised-based GSVA showed the distribution of enterocyte (B), inflammatory (C), transit-amplifying (D), stem-like (E), goblet-like (F), serrated CRC-like (G), conventional CRC-like (H) scores among six subtypes. Kruskal-Wallis test. (I) The distribution of WNT signaling score in different cell-like tumors. Kruskal-Wallis test. (J) CRC cellular phenotypes correlated with colon-crypt location and WNT signaling. (K) Fractions of the crypt base and top phenotypes among six subtypes. Nearest template prediction (NTP) algorithm based on published signatures assigned each sample into the crypt base and top phenotypes in the discovery cohort. (L) GSVA analysis revealed that GINS2 displayed superior stemness abundance relative to other subtypes. (M) Radar plot showed autocrine stimulation loops in GINS subtypes.
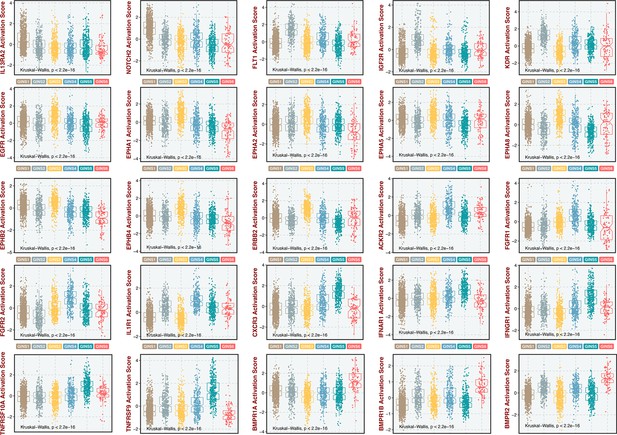
GSVA further estimated differences in Receptor-Ligand activities across six subtypes.
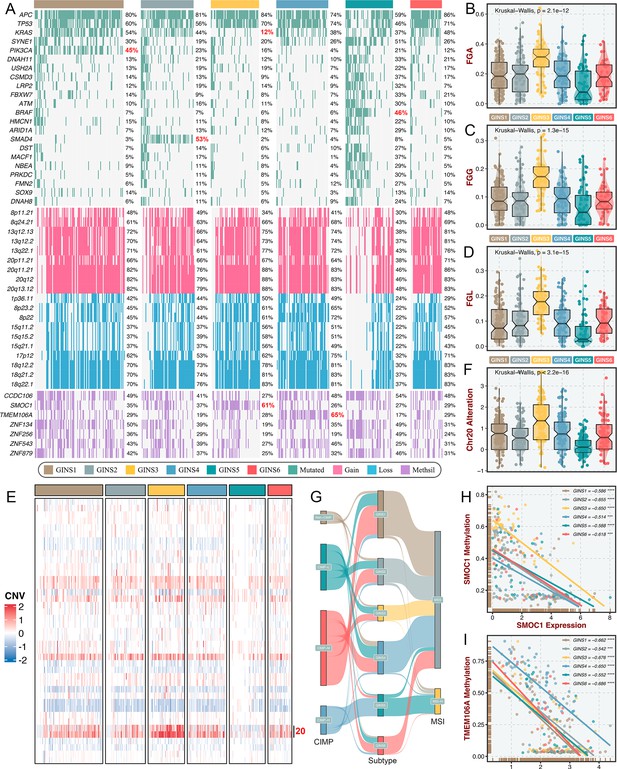
Multi-omics alteration characteristics of six subtypes.
(A) Genomic alteration landscape according to GINS taxonomy. The mutational genes were selected based on mutation frequency >10% and MutSigCV q-value <0.05. The focal gain and loss regions were selected based on CNV frequency >40% and GISTIC q-value <0.05. The methylation silencing (Methsil) genes were identified based on our pipeline. (B–D) The distribution of fraction of genome alteration (FGA), fraction of genome gained (FGG), and fraction of genome lost (FGL) among six subtypes. Kruskal-Wallis test. (E) Heatmap showed the distribution of broad copy number changes across six subtypes in the TCGA-CRC dataset. (F) The distribution of Ch20 alterations in six subtypes. Kruskal-Wallis test. (G) Sankey plot showed connections among GINS subtypes, CIMP phenotypes, and MSI phenotypes. (H–I) The expression levels of SMOC1 and TMEM106A were significantly inversely correlated with their methylation levels. ***p<0.001, ****p<0.0001.
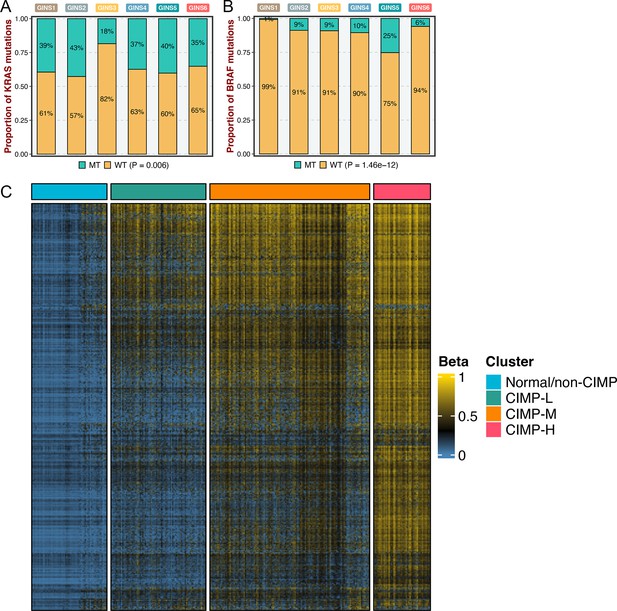
Genomic alterations of six subtypes.
(A-B) Barplots showed the distribution of KRAS (A) and BRAF (B) mutations to six subtypes in the discovery cohort. (C) Four CpG island methylator phenotype (CIMP) were identified from the TCGA-CRC cohort using the beta value of 5000 CpG island promoters with the most variation.
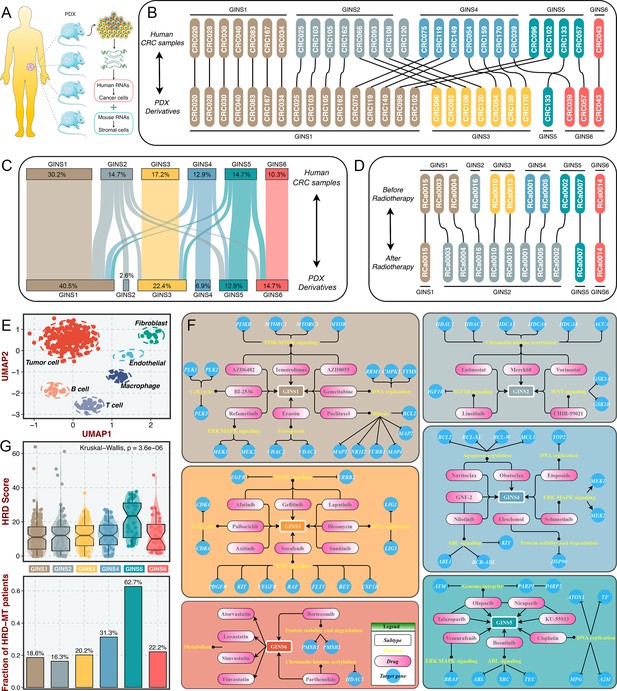
Stromal contributions and potential therapeutic agents.
(A) Schematic diagram showed the PDX transcriptome is a mixture of human RNAs (deriving from cancer cells) and mouse RNAs (deriving from stromal cells). (B–C) Transcriptional classification of paired human CRC samples and PDX derivatives in Uronis cohort (B) and Isella cohort (C). (D) Transcriptional classification of paired samples before and after radiotherapy. (E) UMAP projected all cells annotated by reference component analysis (RCA) in spatial distribution. (F) Identification of potential therapeutic agents for six subtypes. (G) The distribution of HRD score and HRD pathway mutations among six subtypes. Kruskal-Wallis test.
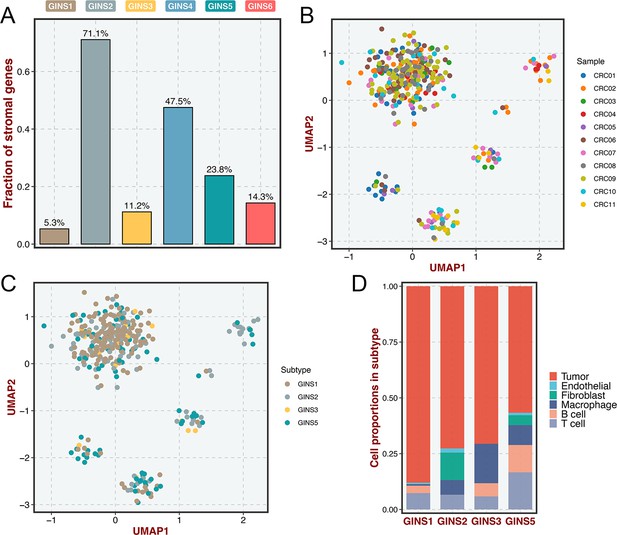
Stromal contribution to the subtype transitions.
(A) Fractions of subtype-discriminant genes of the PAM classifier belonged to stromal genes. (B–C) The cell distribution of 11 patients (B) and GINS subtypes (C) according to the two-dimension UMAP analysis. (D) The composition of cells in each subtype.
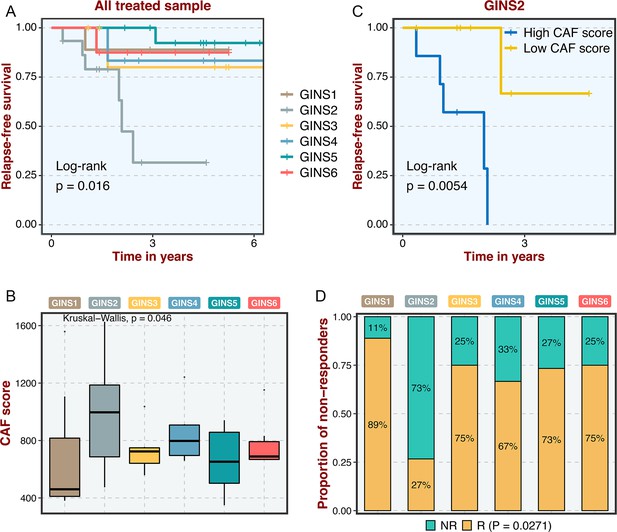
Clinical significance of six subtypes in GSE56699.
(A) Kaplan-Meier curves of overall survival for six GINS subtypes across all treated samples. (B) The distribution of CAF score in six subtypes. (C) Kaplan-Meier curves of overall survival for high and low CAF groups across GINS2 samples. (D) The distribution of radiotherapeutic non-responders in six subtypes.
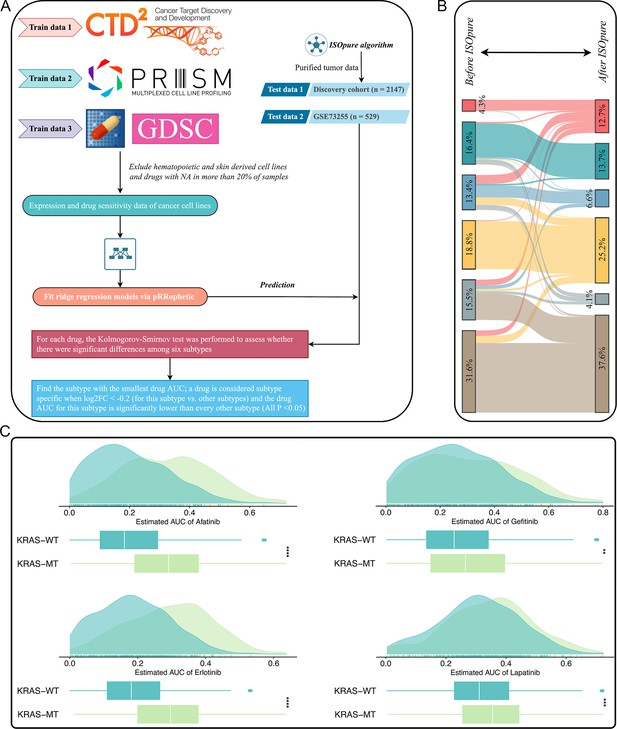
Identification of potential therapeutic agents for six subtypes.
(A) Overview of the analysis procedures. (B) Transcriptional classification of paired samples before and after purification. (C) Comparison of estimated drug sensitivity (logAUC) between KRAS mutant and wild type group. **p<0.01, ***p<0.001, ****p<0.0001.
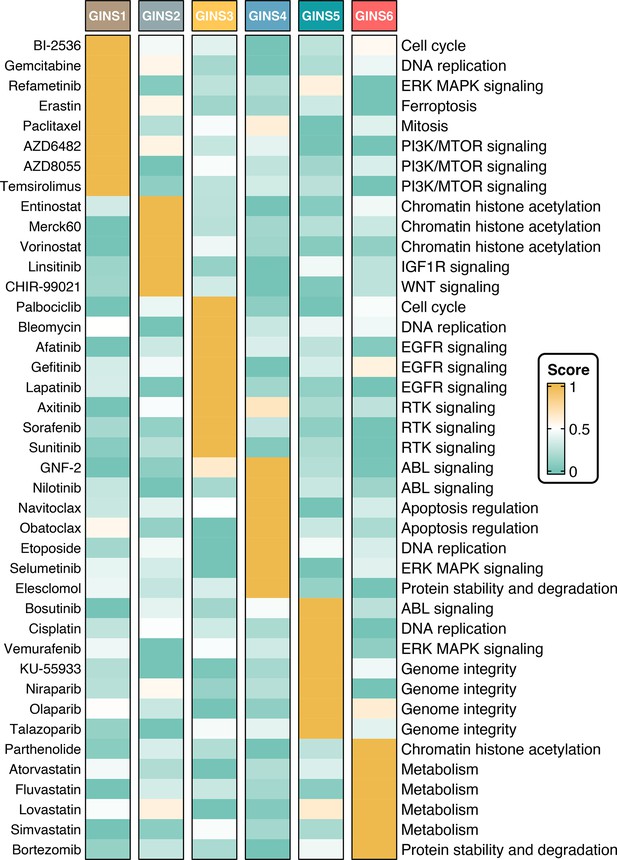
Candidate agents of six subtypes.
Score represents the normalized drug sensitivity (logAUC).
Additional files
-
Supplementary file 1
The PAM-centroid distance classifier included 289 subtype-discriminant genes.
- https://cdn.elifesciences.org/articles/81114/elife-81114-supp1-v1.xlsx
-
Supplementary file 2
Clinical characteristics of six subtypes in validation datasets.
- https://cdn.elifesciences.org/articles/81114/elife-81114-supp2-v1.xlsx
-
Supplementary file 3
Details of baseline information in our in-house dataset.
- https://cdn.elifesciences.org/articles/81114/elife-81114-supp3-v1.xlsx
-
Supplementary file 4
The forward and reverse primers for qRT-PCR.
- https://cdn.elifesciences.org/articles/81114/elife-81114-supp4-v1.xlsx
-
Supplementary file 5
Clinical characteristics of six subtypes in our in-house dataset.
- https://cdn.elifesciences.org/articles/81114/elife-81114-supp5-v1.xlsx
-
Supplementary file 6
Publicly available gene signatures used in this study.
- https://cdn.elifesciences.org/articles/81114/elife-81114-supp6-v1.xlsx
-
Supplementary file 7
Sample set enrichment analysis delineated the biological attributes inherent to GINS subtypes.
*P<0.05, **P<0.01, ***P<0.001.
- https://cdn.elifesciences.org/articles/81114/elife-81114-supp7-v1.xlsx
-
Supplementary file 8
Gene cluster 3 and 10 were identified by Mfuzz analysis.
- https://cdn.elifesciences.org/articles/81114/elife-81114-supp8-v1.xlsx
-
Supplementary file 9
The expression differences of 145 immunomodulators among six subtypes.
- https://cdn.elifesciences.org/articles/81114/elife-81114-supp9-v1.xlsx
-
Supplementary file 10
The proteome (Reverse Phase Protein Array) data available from the TCGA portal included only 26 immunomodulators.
- https://cdn.elifesciences.org/articles/81114/elife-81114-supp10-v1.xlsx
-
Supplementary file 11
GSEA results of 69 metabolic pathways from the Kyoto Encyclopedia of Genes and Genomes (KEGG) database.
- https://cdn.elifesciences.org/articles/81114/elife-81114-supp11-v1.xlsx
-
Supplementary file 12
Metabolite-protein interaction network (MPIN) was established via nine GINS6-specific genes with broad and tight connections with lipid metabolites.
- https://cdn.elifesciences.org/articles/81114/elife-81114-supp12-v1.xlsx
-
Supplementary file 13
Sample set enrichment analysis of ligand/receptor pairs expression in GINS subtypes.
NES and FDR are reported. NE, not enriched.
- https://cdn.elifesciences.org/articles/81114/elife-81114-supp13-v1.xlsx
-
Supplementary file 14
The targeted pahtways and molecules of candidate durgs for six subtypes.
- https://cdn.elifesciences.org/articles/81114/elife-81114-supp14-v1.xlsx
-
Supplementary file 15
Details of data sources.
- https://cdn.elifesciences.org/articles/81114/elife-81114-supp15-v1.xlsx
-
Supplementary file 16
Detailed methods for this article.
- https://cdn.elifesciences.org/articles/81114/elife-81114-supp16-v1.docx
-
MDAR checklist
- https://cdn.elifesciences.org/articles/81114/elife-81114-mdarchecklist1-v1.docx