Temporal context and latent state inference in the hippocampal splitter signal
Figures
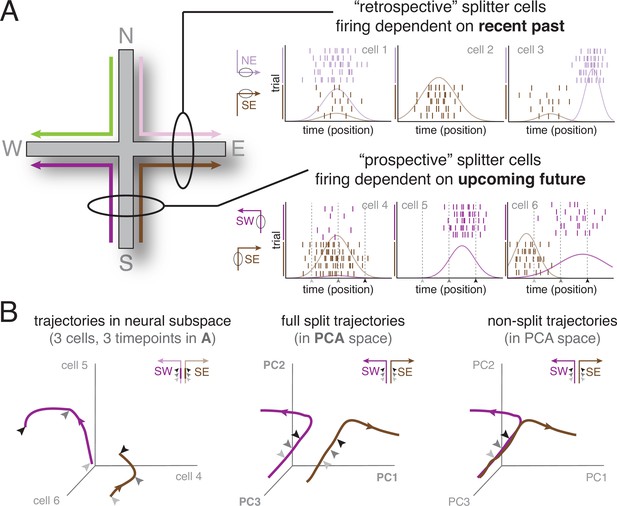
The splitter cell phenomenon at the single cell and ensemble levels.
(A) Schematic activity of six idealized splitter cells during performance of a plus maze task (left), in which four different spatial trajectories are possible (SW, SE, NW, NE). The firing of a true place cell, encoding current location only, does not depend on past or upcoming trajectory; however, splitter cells distinguish recent past (e.g. NE vs SE, top row; depicted cells fire in E) and/or upcoming future (e.g. SW vs SE, bottom row; depicted cells fire in S). Note that individual splitter cells may fire in the same place for both trajectories, but show a difference in firing rate (left column), fire for only one of the trajectories (middle column), or fire in different locations (right column) depending on trajectory. Rasterplots show spikes (tickmarks) for a number of trials of each trajectory (color-coded) as well as tuning curves averaged across trials. For simplicity, we assume subjects travel at constant speed so that time and position are equivalent. (B) Left: Schematic neural activity trajectories of multiple splitter cells in ensemble activity space for the same trajectories as cells shown in the bottom row of (A); SW, purple; SE, brown. Each of the three axes corresponds to the activity (firing rate) of one cell. Arrows are matched with corresponding arrows in (A) and indicate three different time points (locations) during each trial. Note that the SW and SE trajectories occupy clearly distinct areas of neural activity space, even though the subject is traversing the same area of space (same three locations, indicated by arrows, along the S arm), ‘splitting’ trajectories based on different upcoming futures (E vs W). Middle: Schematic neural activity trajectories for a population of neurons, obtained by projecting onto the first three principal components of ensemble activity. Full trajectories are now shown, enabling comparison of ensemble activity during the common segment of the trajectory (S arm indicated by arrows; distinct but relatively close) and the diverging segment (E vs W arm, separating further in ensemble space). Right: Hypothetical ensemble activity in the absence of a splitter signal, for comparison. Note how neural activity trajectories on the common segment (the S arm) overlap, indicating SE and SW trajectories are not distinguishable until the E vs W arms are actually entered.
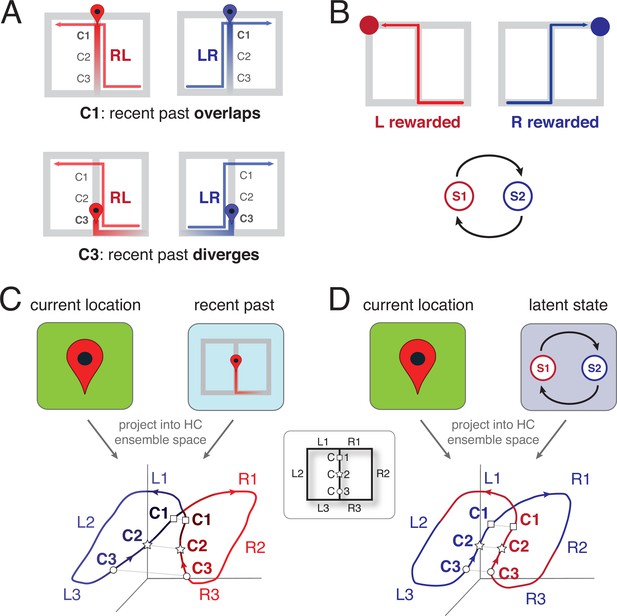
Schematic representation of the two leading theoretical accounts of splitter activity.
(A) Decaying trace of the recent past during performance of a continuous T-maze alternation (figure-of-eight) task. In this account, the recent past overlaps at location C1 for LR and RL trajectories because of their shared recent past (the central stem; top row). In contrast, at the base of the central stem (C3) the recent past for the RL and LR trajectories diverges. Note that the trace of the past decays gradually, to be stronger for the recent past compared to the distant past. (B) Latent task states. In this account, the hippocampus encodes the different states of the task, which for T-maze alternation are two: ‘left rewarded’ and ‘right rewarded’. These states are discrete, and serve to enable the association of state-specific action policies; in this case, the ‘left rewarded’ state specifies that the correct action at the choice point is to turn left. (C) Hypothesized neural activity trajectories in principal component space based on the decaying-trace hypothesis in A. Neural activity follows the general figure-of-eight structure of the task itself. Additionally, ensemble similarity between the LR (blue) and RL (red) activity trajectories on the central stem depends on location: at the base of the stem (C3, small circles), activity is more different than at the top (C1, squares) because of the difference in recent past. (D) Hypothesized neural activity trajectories for the latent-state hypothesis in B. Unlike the account in C, there is no difference in neural activity similarity along the central stem, because task state switches after information about reward is received (on the side arms). HC: Hippocampus.
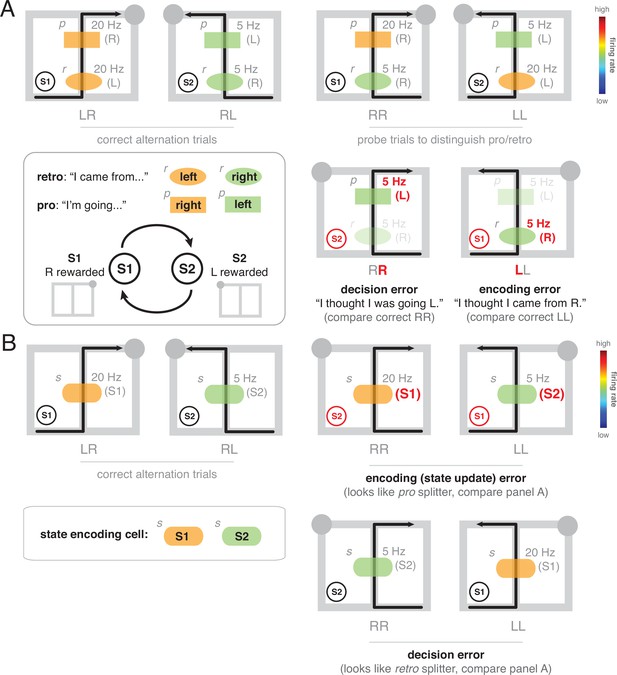
Illustration of sources of error in prospective, retrospective, and state-encoding splitter cells on a continuous alternation task, which has two states, ‘S1’ (right arm rewarded, circle indicates currently rewarded location) and ‘S2’ (left arm rewarded).
(A) Prospective and retrospective cells do not encode these states directly, but simply track where the subject is going (pro) and where it came from (retro). Thus, a retrospective cell (r, oval-shaped firing field) will fire differentially on the central stem for LR and RL trajectories (correct alternation trials, top left; orange indicates high firing rate, green indicates low firing rate) because of the L vs R difference in where the animal came from. Similarly, a prospective cell (p, square-shaped firing field) will fire differentially because of a difference in where the animal is going, and so these two types cannot be distinguished on correct alternation trials; note that the p and r cells have the same activity (colors) for the LR and RL trials. Retrospective cells are revealed by comparing LR and RR (or RL and LL) trajectories (probe trials, top right), and prospective cells are revealed by comparing LR and LL (or RL and RR) trajectories; note that the firing rates (colors) are now different for prospective and retrospective cells. In this scheme, two sources of error are possible. First, the cells may correctly encode what the animal should do, but the decision taken does not match this encoding (decision error, note that the prospective cell correctly indicates an upcoming left turn — which is rewarded in S2 - but the animal incorrectly goes R). Second, the cells may incorrectly encode where the animal came from (encoding error, note that the retrospective cell fires as if the animal came from R, but actually came from L). (B) Splitter cell that encodes S1 (R rewarded) and S2 (L rewarded) directly. Again, two sources of error are possible. First, this cell may incorrectly encode the current state (e.g. fail to update after getting reward), such that when the task is actually in S2, the cell’s activity indicates S1, and vice versa (encoding error, top right panels). Alternatively, the cell correctly signals the current task state, but this is ignored in the decision taken (decision error, bottom right panels). Surprisingly, for state-encoding cells, encoding errors lead to a pattern of activity that looks like a prospective splitter cell (compare p cell in top row of A), whereas decision errors lead to a pattern of activity that looks like a retrospective splitter cell (compare r cell in top row of A). This observation illustrates how the apparently prospective or retrospective character of state-encoding cells is actually an epiphenomenon, resulting from the interaction between task encoding with the task and with the animal’s behavior.
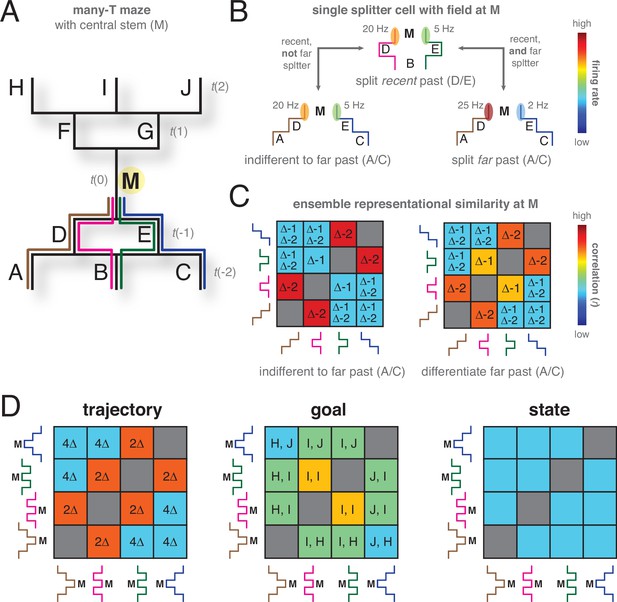
Hypothetical task to illustrate the possible role of representational similarity analyses (RSA) in distinguishing different hypotheses about the content of the splitter signal.
(A) Task design. A maze (left) that has a single central stem ( M) which can be reached from three possible starting points (segments A-C) through four possible trajectories (brown, magenta, green, blue). Similarly, there are three possible goal locations (segments H-J) with four possible trajectories. Analyses focus on the M segment (t=0) so that points A-E are in the past, and F-J in the future. (B) On this task, a hypothetical splitter cell at M could distinguish the recent past (D vs E, t = –1) while being independent of the far past (same activity as for D vs E, even though A vs C at t = –2 are now also different, bottom left panel). Alternatively, its activity could depend on both the recent and the far past (different activity compared to D vs E, bottom right panel). (C) Different timescales of encoding traces of the past result in different representational similarity matrices comparing ensemble neural activity at M across different trajectories. Δ–1 indicates comparisons with a difference at t-1, Δ–2 indicates comparisons with a difference at t-2. If only the recent past is encoded in ensemble hippocampal activity (left matrix), trajectories that share the recent segment (no difference at t = –1, tiles with no Δ–1 in the matrix) are correlated (red matrix elements), while trajectories that differentiate the recent segment (tiles with Δ–1) are uncorrelated. In contrast, an ensemble that differentiates both recent and far past in a graded manner (right matrix) will show some positive correlation when the far past is shared (no Δ–2, yellow matrix elements). (D) Similarly, different possible hypotheses about what is encoded by the splitter cell signal (full trajectory, goal, task state) result in different RSA matrices. For the full trajectory RSA matrix (left panel), correlations are driven by the number of overlapping trajectory segments (high correlation if only 2 segments are different, 2Δ; low correlation if all four segments of the trajectory are different, 4Δ). If ensemble similarity is based on goal (center panel), correlations are driven only by how close in space the goal is (highest for the same goal, I, I; intermediate for adjacent goals such as H, I and J, I; low for far goals). Finally, if neural activity encodes task state (i.e. what trajectory needs to be executed in order to get reward; right panel), every trajectory is equally uncorrelated with every other trajectory, because no spatial information is required to distinguish states. Underlying these RSA predictions is the idea that computations based on state have different information content compared to those based on trajectory: state-based computations, minimally, only require the states to be different. In contrast, trajectories are spatial, and have similarity structure based on factors like distance and amount of overlap.
Tables
Summary of model predictions and open questions.
Temporal context model | Hidden state inference | Data | |
---|---|---|---|
Temporal gradedness | Y | N | Y |
Dissociations between prospective and retrospective splitting | Y: result from different processes (buffer vs. learned from experience) | Depends: both occur as a result of the same process, but interacts with task and behavior | Some indications for Y, but not tested much |
Increase in retrospective splitting with time | N: no reason why buffer isn’t operational from trial 1 | Y: state representations need to be learned from experience | Y |
More retrospective than prospective splitters even if task fully learned | ? | Depends: on task and type of errors | Y |
Dependence on task-relevance | N: at least not for retrospective splitters: temporal context is always there | Y: bias for representing states that have predictive value | Mixed evidence, needs better tests |
Dependence on training procedure | N | Y | Generally Y, but rarely tested systematically |
Discrete state transitions | N | Y | Untested |
Splitters generalize across remapped tasks with identical structure | N | Y | Mixed: N in Bahar and Shapiro, 2012 & Hallock and Griffin, 2013, Y in Takahashi, 2013 & Sun et al., 2020 |