TMS-evoked responses are driven by recurrent large-scale network dynamics
Figures
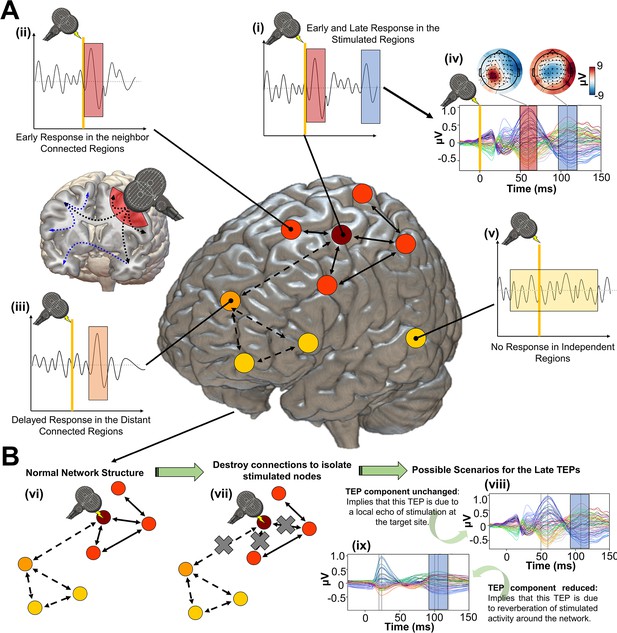
Studying the role of recurrent activity in stimulation-evoked neural responses with computational models.
Shown here is a schematic overview of the hypotheses, methodology, and general conceptual framework of the present work. (A) Single transcranial magnetic stimulation (TMS) pulse (i - diagram, iv - real data) applied to a target region (in this case left M1) generates an early response (TMS-EEG evoked potential (TEP) waveform component) at electroencephalography (EEG) channels sensitive to that region and its immediate neighbors (ii). This also appears in more distal connected regions such as the frontal lobe (iii) after a short delay due to axonal conduction and polysynaptic transmission. Subsequently, second and sometimes third late TEP components are frequently observed at the target site (i, iv), but not in non-connected regions (v). Our central question is whether these late responses represent evoked oscillatory ‘echoes’ of the initial stimulation that are entirely locally driven and independent of the rest of the network, or whether they rather reflect a chain of recurrent activations dispersing from and then propagating back to the initial target site via the connectome. (B) In order to investigate this, precisely timed communication interruptions or ‘virtual lesions’ (vii) are introduced into an accurately fitting individual subject computational model of TMS-EEG stimulation responses (vi), and the resulting changes in the propagation pattern (vii) are evaluated. No change in the TEP component of interest (e.g. blue shaded area) would support the ‘local echo’ scenario (viii), whereas suppressed TEPs (e.g. blue shaded area) would support the ‘recurrent activation’ scenario (ix).
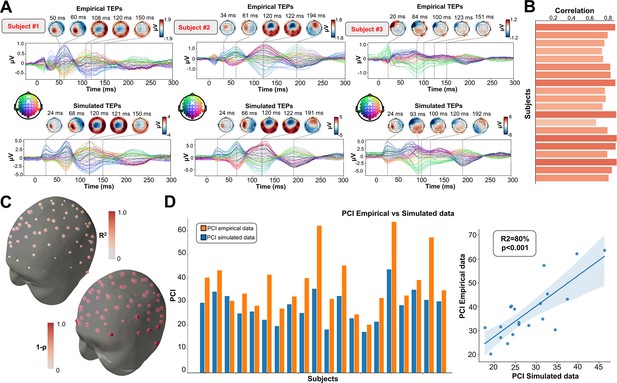
Comparison between simulated and empirical TMS-EEG data in channel space.
(A) Empirical (upper row) and simulated (lower row) TMS-EEG butterfly plots with scalp topographies for three representative subjects, showing a robust recovery of individual empirical TMS-EEG evoked potential (TEP) patterns in model-generated activity electroencephalography (EEG) time series. (B) Pearson correlation coefficients between simulated and empirical TMS-EEG time series for each subject. (C) Time-wise permutation tests result showing the Pearson correlation coefficient (top) and the corresponding significant reversed p-values (bottom) for every electrode. (D) Perturbational complexity index (PCI) values extracted from the empirical (orange) and simulated (blue) TMS-EEG time series (left). A significant positive correlation (R2=80%, p<0.001) was found between the simulated and the empirical PCI (right), demonstrating high correspondence between empirical and simulated data.
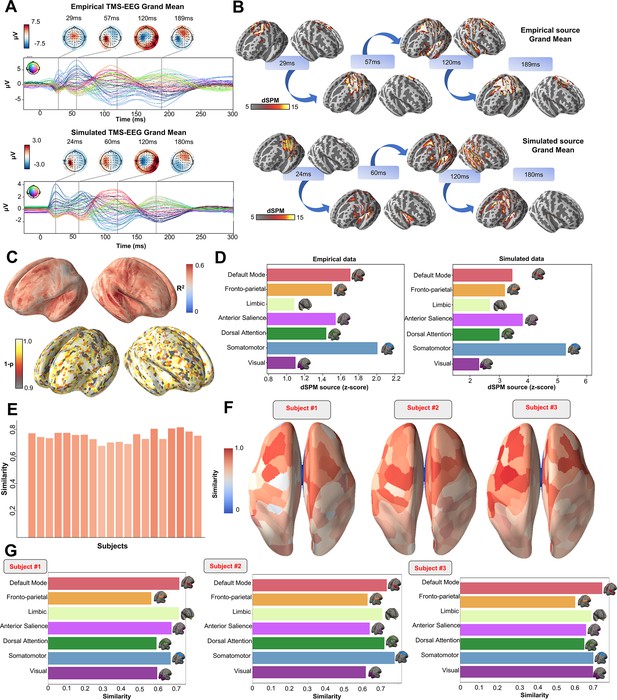
Comparison between simulated and empirical TMS-EEG data in source space.
(A) TMS-EEG time series showing a robust recovery of grand-mean empirical TMS-EEG evoked potential (TEP) patterns in model-generated electroencephalography (EEG) time series. (B) Source reconstructed TMS-evoked propagation pattern dynamics for empirical (top) and simulated (bottom) data. (C) Time-wise permutation test results showing the significant Pearson correlation coefficient (top) and the corresponding reversed p-values (bottom) for every vertex. (D) Network averaged dSPM values extracted for the grand mean empirical (left) and simulated (right) source-reconstructed time series. (E) Bar plot showing high vertex-wise cosine similarity between empirical and simulated sources for all the subjects. (F) Parcels-wise cosine similarities plotted on surface for three representative subjects, showing a robust recovery of empirical TMS-evoked patterns in model-generated activity EEG time series. (G) Network-based cosine similarity for three representative subjects.
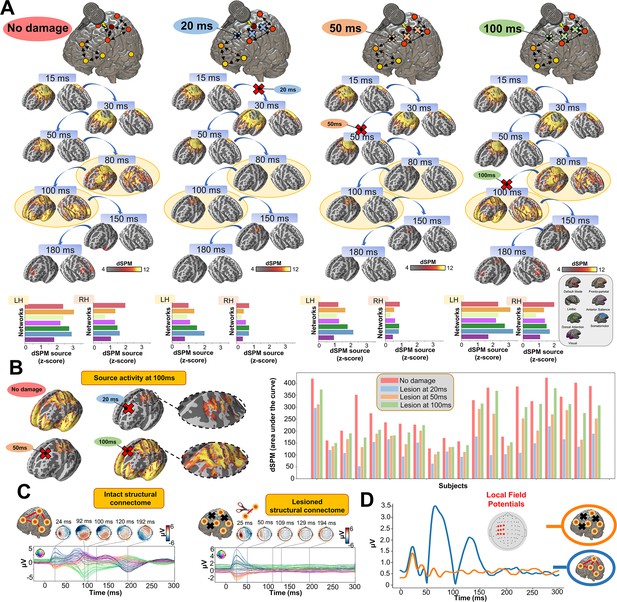
Removing recurrent connections from stimulated target nodes suppresses their late TMS-EEG evoked potential (TEP) activity.
(A) We found that TMS-evoked propagation dynamics in the model change significantly depending on the specific time that a virtual lesion is applied (highlighted orange circle). Specifically, early significant reductions in the TMS-evoked activity (50 ms-100 ms time window) were found when important connections were removed at 20 ms (blue) and 50 ms (orange) after the transcranial magnetic stimulation (TMS) pulse, as compared to both a later virtual lesion (100 ms green) and no damage (red) conditions. These results are demonstrated also for network-based dynamic Statistical Parametric Mapping (dSPM) values (bottom row) extracted for all four conditions. (B) Zoom of the source localized electroencephalography (EEG) activities for the four different conditions showing how early lesions (e.g. 20 ms and 50 ms) compromised the propagation of the TMS-induced signal compared to control (no damage) and late lesion (100 ms) conditions (left). For all the subjects, we reported a reduced TMS-evoked activity (dSPM area under the curve) when the lesion was applied at 20 ms and 50 ms compared to the others conditions (right bar plot). (C) Demonstration of the network recurrence-based theory for one representative subject. Simulation of TMS-EEG dynamics run using the intact (left) and lesioned (right) anatomical connectome. In the latter case, the connection was removed 50 ms after the TMS pulse and external perturbation generate a local response that reverberates locally and terminates after ~50 ms. This demonstrates again how later TEPs are driven by recurrent network dynamics. (D) Local Mean Field Power (LMFP) at the stimulation site for intact (blue line) and lesioned (orange line) anatomical connectome. Red dots inside the scalp map correspond to the electrodes from where the LMFP was extracted.
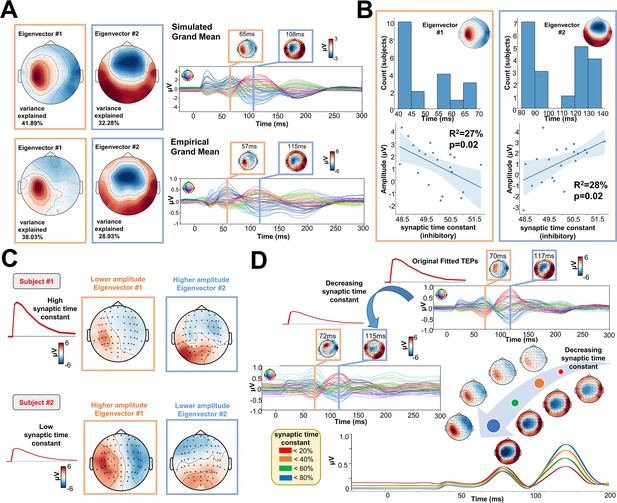
Synaptic time constants of inhibitory neural populations affect early and late TMS-EEG evoked potential (TEP) amplitudes.
(A) Grand mean Singular value decomposition (SVD) topoplots for simulated (top) and empirical (bottom) TMS-EEG data. Results revealed that the first (orange outline) and the second (blue outline) SVD eigenmodes were located ~65 ms and ~110 ms after the transcranial magnetic stimulation (TMS) pulse, respectively. (B) First and second SVD temporal eigenmode latencies and amplitudes were extracted for every subject, and the distribution plots (top row) show the time window where the highest cosine similarity with the SVD spatial eigenvectors was found. Scatter plots (bottom row) show a significant negative (left) and positive (right) correlation between the synaptic time constant of the inhibitory population and the amplitude of the first and second eigenvectors. (C) Model-generated first and second SVD eigenmodes for two representative subjects with high (top) and low (bottom) estimated values for the synaptic time constant of the inhibitory population. The topoplots show that the magnitude of the synaptic time constant is closely coupled to the amplitude of the individual first and second SVD modes. (D) Model-generated TMS-EEG data were run using the optimal (top right) or 85% decreased (central left) value for the synaptic time constant of the inhibitory population. The bottom right panel shows absolute values for different magnitudes of this parameter. Results show an increase in the amplitude of the first, early, and local TEP components; and a decrease of the second, late, and global TEP components, as a function of the inhibitory synaptic time constant.
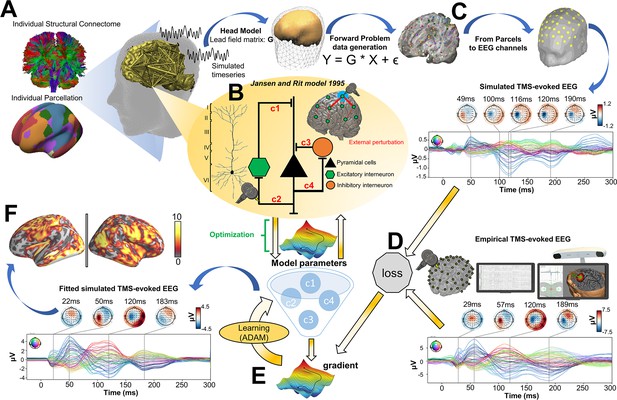
Methodological workflow for subject-specific connectome-based neurophysiological modelling of TMS-EEG TEPs.
(A) Diffusion-weighted MRI (DW-MRI) tractography was computed from a sample of healthy young individuals from the Human Connectome Project (HCP) Dataset (Van Essen et al., 2012), and then averaged to give a grand-mean anatomical connectome. The 200-parcel Schaefer atlas (Schaefer et al., 2018) was used, which usefully aggregates its 200 brain regions into seven canonical functional networks (Visual network: VISN, Somatomotor network: SMN, Dorsal attention network: DAN, Anterior salience network: ASN, Limbic network: LIMN, Fronto-parietal network: FPN, Default mode network: DMN). These parcels were mapped to the individual’s FreeSurfer parcellation using spherical registration (Fischl et al., 1999). Once this brain parcellation covering cortical structures was extrapolated, it was then used to extract individual anatomical connectomes. (B) The Jansen-Rit model (Jansen and Rit, 1995), a neural mass model comprising pyramidal, excitatory interneuron, and inhibitory interneuron populations was embedded in every parcel for simulating and fitting neural activity time series. The TMS-induced depolarization of the resting membrane potential was modeled by a perturbing voltage offset to the mean membrane potential of the excitatory interneuron population. (C) A lead field matrix was then used for moving the parcels’ time series into channel space and generating simulated electroencephalography (EEG) measurements. (D) The goodness-of-fit (loss) was calculated as the cosine similarity between simulated and empirical TMS-EEG time series. (E) Utilizing the autodiff-computed gradient (Rall, 1981) between the objective function and model parameters, model parameters were optimized using the ADAM algorithm (Kingma and Ba, 2017). (F) Finally, the optimized model parameters were used to generate the fitted, simulated TMS-EEG activity, for which we report comparisons with the empirical data at both the channel and source level using conventional statistical techniques.
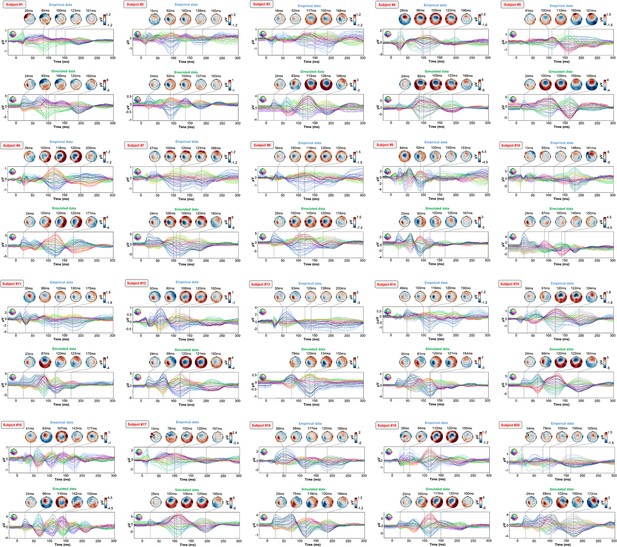
Optimized TMS-EEG evoked potential (TEP) models for all subjects.
For every pair of rows, empirical (upper) and simulated (lower) TMS-EEG responses are shown for every study subject, extending main text Figure 2 where a selected subset of subjects’ data are shown. These data reiterate and reinforce the demonstrations in Figure 2 that the model-generated electroencephalography (EEG) activity time series achieve robust recovery of individual subjects’ empirical TEP propagation patterns.
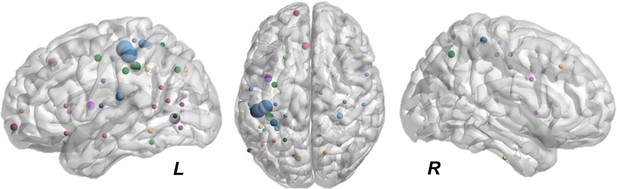
Representation of the maximally activated nodes.
Sizes indicate for how many subjects that node was identified as maximally activated by the external perturbation. A clear pattern is evident, where the more important nodes are the ones more affected by the stimulation (e.g. motor, parietal, and frontal cortex). Once those nodes were identified, their incoming and the outgoing connection weights were set to zero for the duration of the simulation.
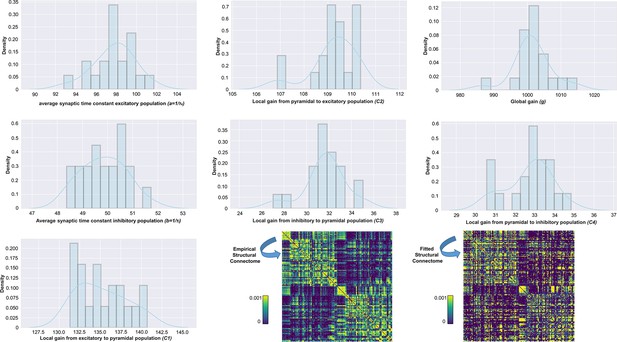
Distributions of physiological parameter estimates over subjects.
Histograms and kernel density estimates of the estimated values for the Jansen-Rit model physiological parameters over all subjects. Also shown are prior and posterior parameter values for anatomical connectome weights for a single example subject (bottom right). Parameter estimation was performed using our novel automatic differentiation and gradient-based approach inspired by current techniques in deep learning (Griffiths et al., 2022).
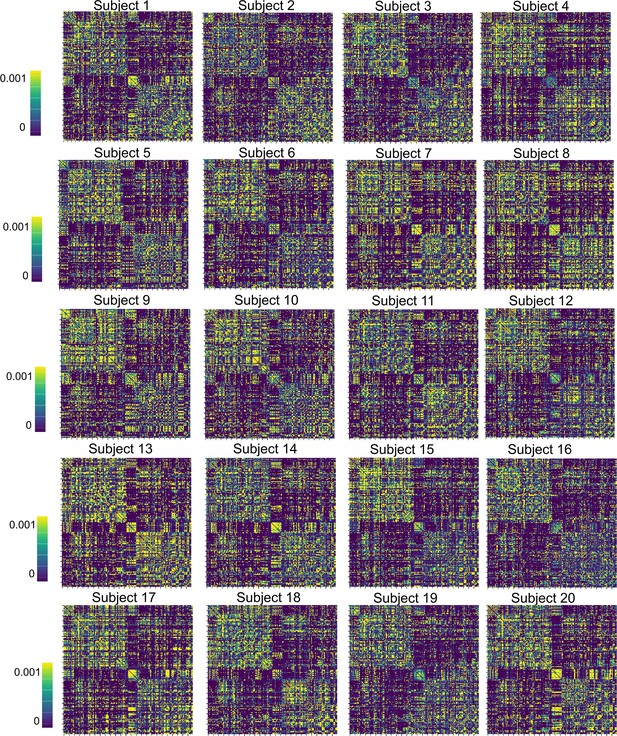
Matrices of the posterior mean structural connectivity weights for all subjects.
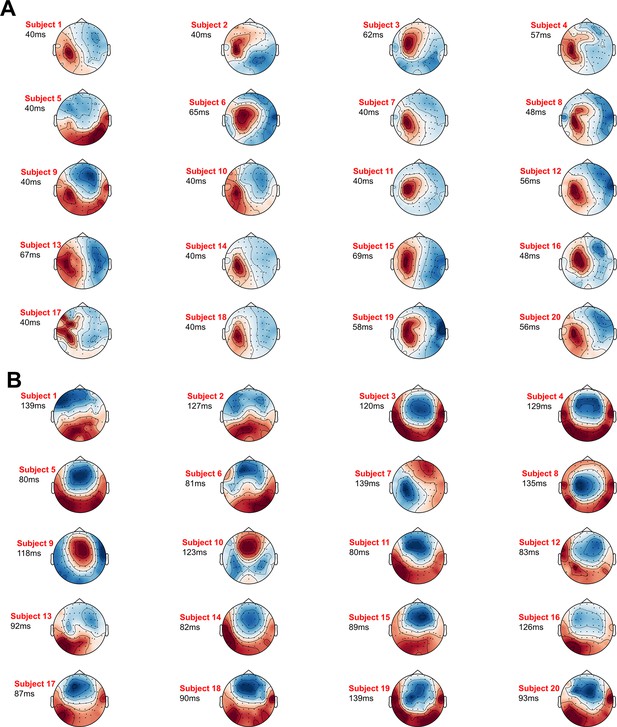
Timing and topographies of the prototypical TMS-EEG evoked potential (TEP) response pattern in each subject.
These figures extend the single-subject examples from TEP channel data singular value decompositions (SVD) decompositions in Figure 5. (A) First right singular vectors from TEP SVDs for all subjects, with corresponding time location indicating the time point of maximum expression for the corresponding left singular vector (temporal eigenmode). (B) Second right singular vectors and corresponding time points of maximum expression.
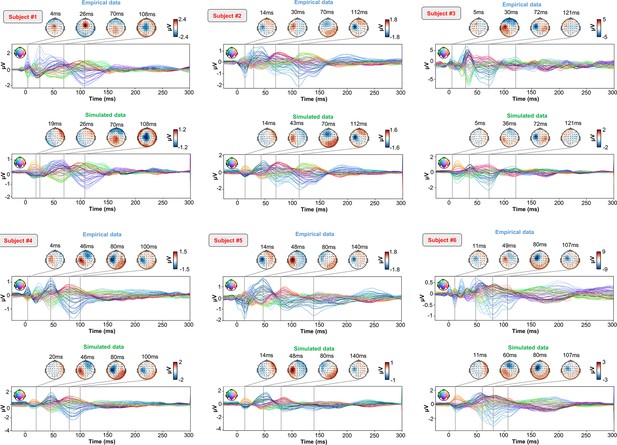
Optimized TMS-EEG evoked potential (TEP) models for all subjects of the Fecchio, Pigorini et al., high voltage motor evoked potential dataset.
For every pair of rows, empirical (upper) and simulated (lower) TMS-EEG responses are shown for every study subject, validating the results using the Rogasch’s data. These data reiterate and reinforce the demonstrations of the main paper that the model-generated electroencephalography (EEG) activity time series achieve robust recovery of individual subjects’ empirical TEP propagation patterns.
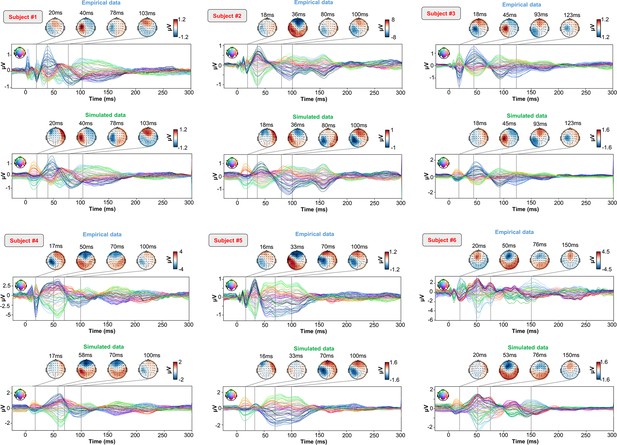
Optimized TMS-EEG evoked potential (TEP) models for all subjects of the Fecchio, Pigorini et al., low voltage motor evoked potential dataset.
For every pair of rows, empirical (upper) and simulated (lower) TMS-EEG responses are shown for every study subject, validating the results using the Rogasch’s data. These data reiterate and reinforce the demonstrations of the main paper that the model-generated electroencephalography (EEG) activity time series achieve robust recovery of individual subjects’ empirical TEP propagation patterns.
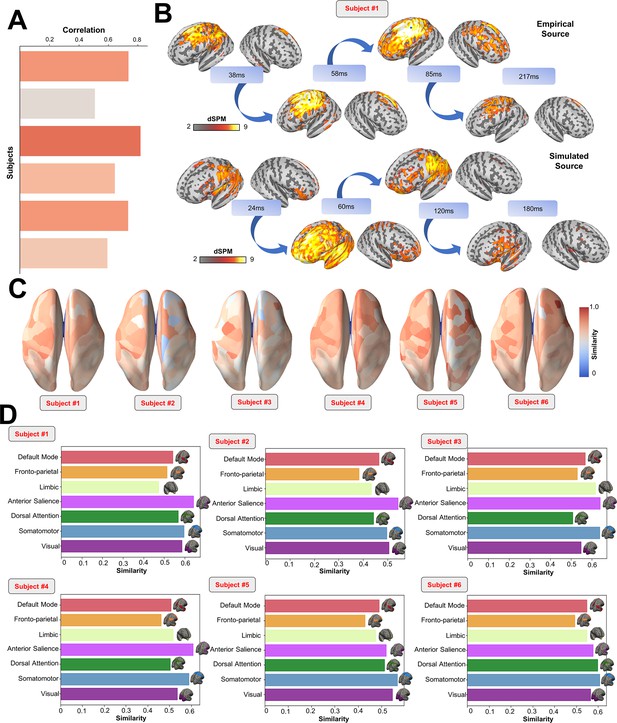
Comparison between simulated and empirical TMS-EEG data in source space for the Fecchio, Pigorini et al., dataset.
(A) Bar plot showing high vertex-wise cosine similarity between empirical and simulated sources for all the subjects. (B) Source reconstructed TMS-evoked propagation pattern dynamics for empirical (top) and simulated (bottom) data for one representative subject. (C) Parcels-wise cosine similarities plotted on the surface for the entire sample of the Fecchio, Pigorini et al., dataset (Fecchio et al., 2017), showing a robust recovery of empirical TMS-evoked patterns in model-generated activity electroencephalography (EEG) time series. (D) Network-based cosine similarity for all the subjects. This results validate the findings using the Rogasch’s dataset in a completely independent dataset.
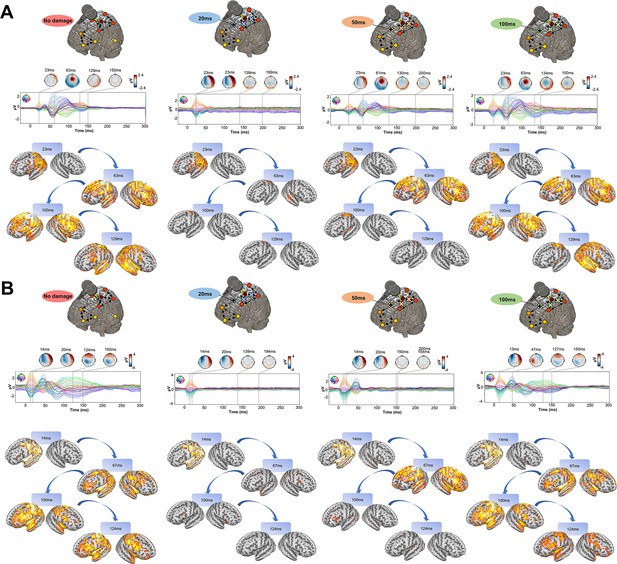
Removing recurrent connections from stimulated target nodes suppresses their late TMS-EEG evoked potential (TEP) activity using the dataset of Fecchio, Pigorini et al.
TMS-evoked propagation dynamics in the model change significantly depending on the specific time that a virtual lesion is applied. Specifically, early significant reductions in the TMS-evoked activity (50 ms-100 ms time window) were found when important connections were removed at 20 ms (blue) and 50 ms (orange) after the TMS pulse, as compared to both a later virtual lesion (100 ms green) and no damage (red) conditions. This findings was found using both high (Panel A) and low (Panel B) voltage data and replicate the results reported in the main paper and in Figure 4 using the Rogasch’s dataset.
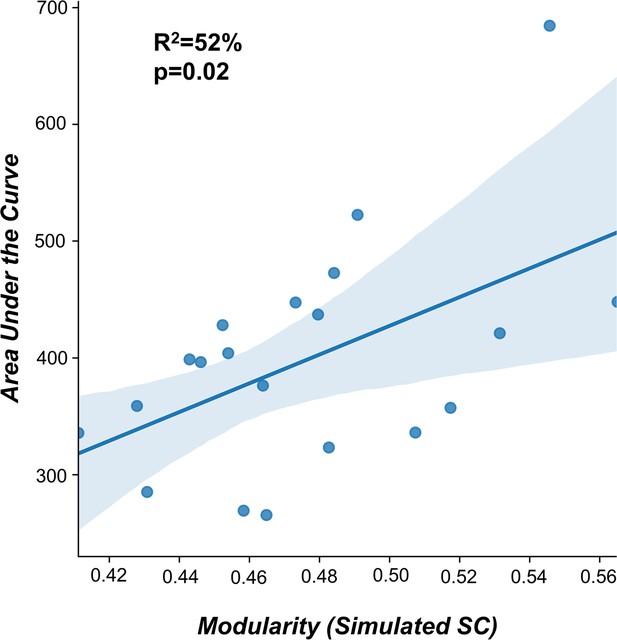
Structural connectivity predictor of TMS-EEG propagation.
A significant positive correlation (R2=52%, p=0.02) was found between the modularity of the fitted structural connectomes and the area under the curve (AUC) extracted for significant post-TMS time points. This findings replicate the results reported in Momi et al., 2021b.
Tables
a | b | C1 | C2 | C3 | C4 | g | |
---|---|---|---|---|---|---|---|
Our paper | 97.78305 | 49.85237 | 1352205 | 109.2454 | 31.65449 | 32.72027 | 1001.478 |
Stefanovski 2019 | 100 | 50 | 135 | 108 | 33.75 | 33.75 | 0<g>600 |
Jansen and Rit 1995 | 100 | 50 | 135 | 108 | 33.75 | 33.75 | n/a |
Ableidinger et al., 2017 | 100 | 50 | 135 | 108 | 33.75 | 33.75 | 1000 |
Ahmadizadeh et al., 2018 | 100 | 50 | 135 | 108 | 33.75 | 33.75 | 1000 |
Additional files
-
Supplementary file 1
Used fitted parameters for each Jansen-Rit element in the large-scale brain network.
- https://cdn.elifesciences.org/articles/83232/elife-83232-supp1-v1.csv
-
MDAR checklist
- https://cdn.elifesciences.org/articles/83232/elife-83232-mdarchecklist1-v1.pdf