SARS-CoV-2 uses CD4 to infect T helper lymphocytes
Figures
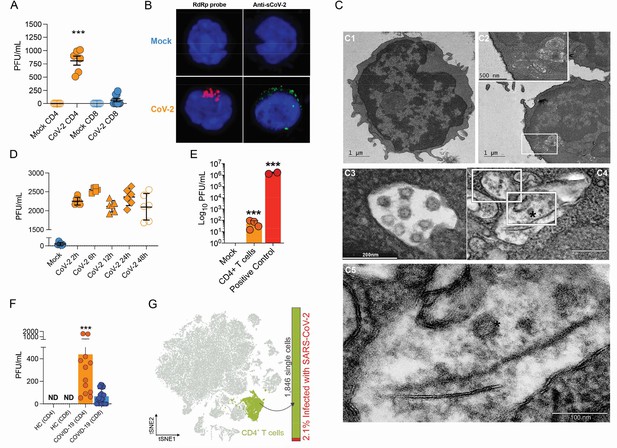
Severe acute respiratory syndrome coronavirus 2 (SARS-CoV-2) infects CD4+ T cells in vitro and in vivo.
Peripheral blood CD3+CD4+ and CD3+CD8+ were exposed to mock control or SARS-CoV-2 (CoV-2) (Multiplicity of Infection (MOI) = 1) for 1 hr under continuous agitation. (A) Viral load was assessed by RT-qPCR 24 hr after infection. (B) Cells were washed, cultured for 24 hr, fixed with 4% paraformaldehyde (PFA), and stained with the RdRp probe for in situ hybridization or anti-sCoV-2 for immunofluorescence. Cells were analyzed by confocal microscopy. (C, C1–C5) Representative transmission electron microscopy (EM) micrographs showing viral particles (asterisks) inside lymphocytes 2 hr after infection. (D) Viral load was determined by qPCR 2 hr, 6 hr, 12 hr, 24 hr, and 48 hr after infection. (E) Vero cells were incubated with the supernatant of mock control or CoV-2-infected CD4+T cells under continuous agitation for 1 hr. The viral load in Vero cells was measured after 72 hr using plaque assay. PFU, plaque-forming unit. (F) Viral load was measured by RT-qPCR in peripheral blood CD4+ and CD8+ T cells from healthy controls (HC) and COVID-19 patients. (G) CoV-2 RNA detection in CD4+ T cells from bronchoalveolar lavage fluid (BALF) single-cell RNA sequencing data revealing the presence of CoV-2 RNA. Data represents mean ± SEM of at least two independent experiments performed in triplicate or duplicate (f). ***p<0.001; ND, not detected.

Comprehensive analysis of ACE2, TMPRSS2, and CD4 expression in human tissues and cell types.
(A) Expression of ACE2, TMPRSS2, and CD4 in multiple tissues and cell types according to BioGPS database and The Human Protein Atlas. (B) NX expression denotes consensus mRNA expression between multiple databases. (C) Protein detection through immunohistochemistry data was plotted based on The Human Protein Atlas annotations (quantity classifications are subjective). Top 25 cells/tissues with the highest expression of CD4 are marked.

ACE2 expression in peripheral blood leukocytes.
(A, B) Flow cytometry analysis of ACE2 in peripheral blood cells (FMO: gray; anti-ACE2: orange): CD3+CD4+, CD3+CD8+, CD19+, CD123+, CD14+ and CD16+. CD14-.(C) mRNA expression of ACE2 and TMPRSS2 in T lymphocytes, A3.01, A2.01, and A2.04 cells.
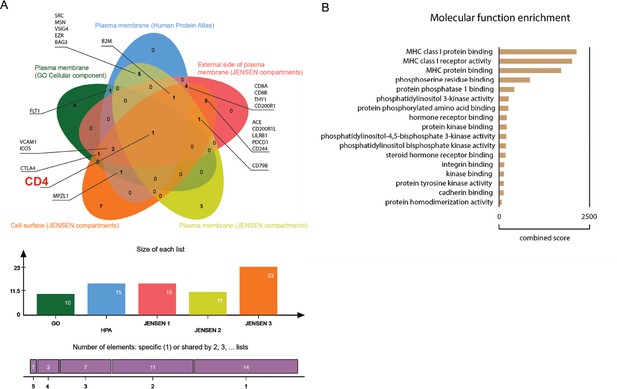
Proteomic in silico analysis unveils interactions of SARS-CoV-1 with human cellular membrane proteins.
(A) Venn diagram of human proteins that were predicted by P-HIPSTer to interact with sCoV-1 and considered as part of cellular membrane in at least one database. Proteins found in at least two databases are annotated. (B) Molecular function enrichment of 71 predicted human proteins that interact with sCoV-1 according to P-HIPSTer.
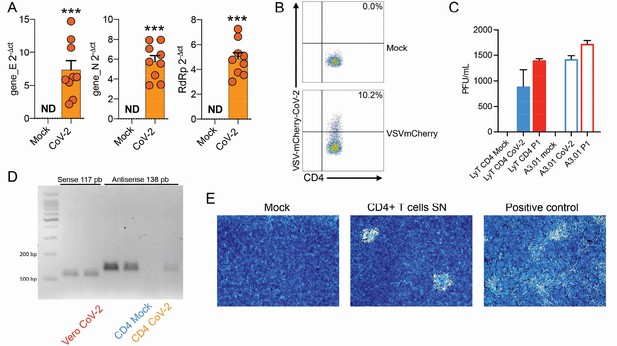
Evaluation of SARS-CoV-2 infection dynamics in CD4+ T cells.
(A) Relative expression of the viral envelope (E), nucleocapsid (N), and RNA-dependent RNA polymerase (RdRp) genes in peripheral blood CD4+ T cells infected with mock control or CoV-2. (B) Primary human CD4+ T cells were incubated with pseudotype (VSV-mCherry-CoV-2). (C) Peripheral blood CD3+CD4+ was infected with mock control or SARS-CoV-2 (CoV-2) lineages (B and P.1) (MOI 1) for 1 hr under continuous agitation. Viral load was assessed by RT-qPCR 24 hr after infection. (D) PCR for the antisense CoV-2 strand was performed in CD4+ T and Vero (positive control) cells infected with mock or CoV-2. (E) Vero cells were incubated with the supernatant of mock control or CoV-2-infected CD4+ T cells under continuous agitation for 1 hr. The viral load in Vero was measured after 72 hr using plate assay. PFU, plaque-forming units.
-
Figure 1—figure supplement 4—source data 1
Original agarose gel with PCR for the antisense CoV-2 strand in CD4+ T and Vero (positive control) cells infected with mock or CoV-2.
- https://cdn.elifesciences.org/articles/84790/elife-84790-fig1-figsupp4-data1-v1.zip
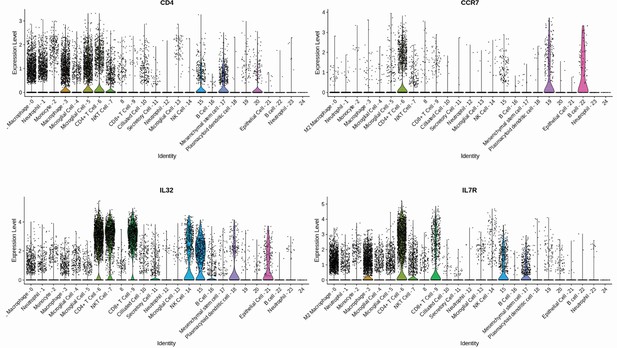
Gene signature of individual CD4+ T cells population.
CD4+ T cell subpopulations were annotated considering a high differential expression of CD4, CCR7, IL7R, and IL32 gene markers.
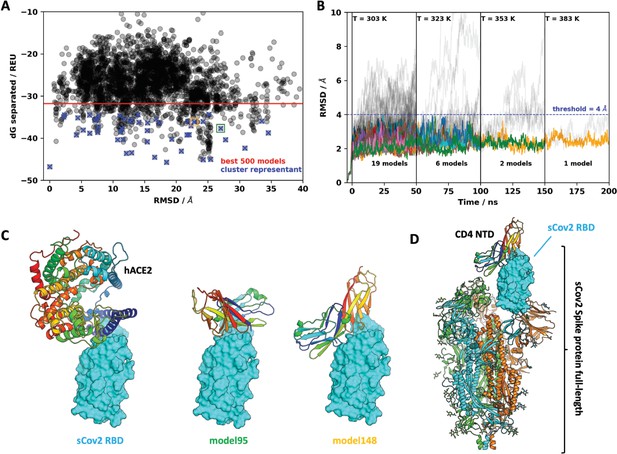
Molecular dynamics simulations of the sCoV-2 receptor binding domain (RBD) and CD4 N-terminal domain (NTD) interaction.
(A) Interaction energy (dG separated) versus root-mean-square deviation (RMSD) plot shows a high diversity of binding modes with similar energies. Among the 500 best models, 50 cluster best-ranked representatives (shown as blue crosses) were selected for further evaluation with molecular dynamics simulations. (B) RMSD to the initial docked complex as a function of molecular dynamics simulation time over 200 ns and four steps of temperature. At each temperature step, well-behaved models are depicted as colored curves, while divergent candidates are shown as gray curves. Kinetically stable models making reasonable interactions remain close to their initial docked conformation. Only two models remain stable after the third step of 50 ns at 353 K. For both models, resilient contacts throughout simulation are shown in Fig. S5. (C) Interaction models of ACE2 and sCoV-2 RBD. The two best candidates according to molecular dynamics simulation, model 95 and model 148, present distinct binding modes. For the first case, interaction occurs mainly in the N-terminal portion of CD4 NTD, while the latter have important contributions to the central part of this domain. (D) Full-length model of sCoV-2 and CD4 NTD interaction obtained by alignment of sCoV-2 RBD from model 148 to the sCoV-2 RBD open state from PDB 6vyb EM structure.
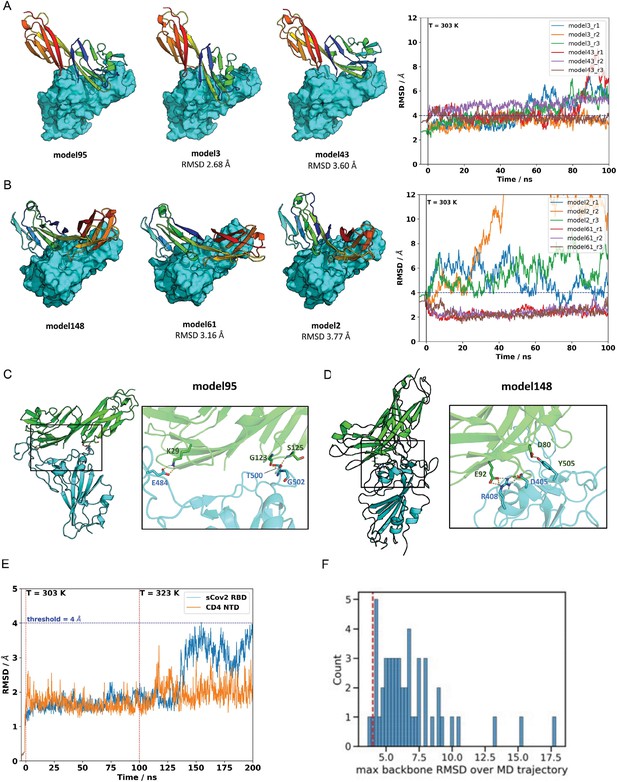
Molecular dynamics simulations of the sCoV-2 receptor binding domain (RBD) and CD4 N-terminal domain (NTD) interaction.
The two closest cluster members to model 95 (A) and model 148 (B) were subjected to 100 ns molecular dynamics (MD) simulations at 303 K in triplicate. Neighboring models 3 and 43, in the root-mean-square deviation (RMSD) range of ~2.6–3.8 A to the reference model 95, represent closely related binding modes. Similarly, models 2 and 61 are closely related to cluster-representative model 148. The right panels show the RMSD relative to the reference model as a function of trajectory time. Model 61, initially at 3.16 A from its cluster representative (model 148), converged to the structure of model 148 for all runs. This result provides additional evidence that model 148 is a likely candidate for CD4-RBD binding. (C) For model 95, three polar contacts are well defined in the interface region: CD4 lysine 29 is hydrogenbonded to sCov2 glutamic acid 484; glycine carbonyl oxygen and amide hydrogen of serine 125 from CD4 make h-bonds to amide hydrogen of glycine 502 and threonine 500 of sCov2, respectively. (D) For model 148, two polar contacts are persistent: one hydrogen bond between CD4 aspartic acid 80 and sCov2 tyrosine 505 and a second hydrogen bond between CD4 glutamic acid 92 and sCov2 arginine 408 whose side-chain orientation is also supported by a third residue, sCov2 aspartic acid 405, which completes a three-residue hydrogen bond network. (E) MD simulations on both the sCov2 RBD and CD4 monomers at 303 K and 323 K. (F) Histogram of maximum backbone RMSD observed over MD trajectories evaluated in the article. Dashed red line shows where the 4 Å is in the whole distribution. RDD, receptor binding domain.
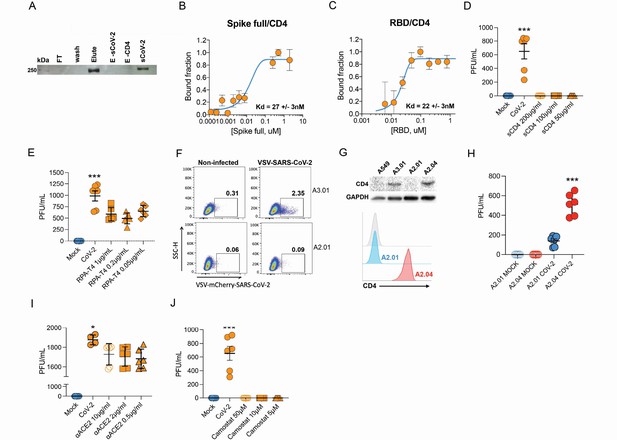
Infection of CD4+ T cells by SARS-CoV-2 is dependent on CD4 and ACE2 molecules.
(A) Recombinant sCoV-2 (with twin-strep-tag) and CD4 were co-incubated and immunoprecipitated with anti-CD4. Complex formation was determined by Affinity blotting using streptavidin-HRP. (B, C) Fluorescence anisotropy curves of Spike full length and Spike receptor binding domain (RBD) binding to CD4 labelled protein, presenting dissociation constants (Kd) of 27 ± 3 nM and 22 ± 3 nM, respectively. The CD4 was labeled with FITC by incubation with fluorescein isothiocyanate probe, at molar ratio 3FITC:1protein, 4°C for 3 hr. The probe excess was removed by a desalting column (HiTrap 5 ml, GE) in a buffer containing 137 mM NaCl, 10 mM Na phosphate, 2.7 mM KCl, pH of 7.4. To evaluate binding affinities, serial dilutions of Spike (RBD or full length) were performed, from 4.5 μM to 100 nM, over 20 nM of labeled CD4. The measurements were taken using ClarioStar plate reader (BMG, using polarization filters of 520 nm for emission and of 495 nm for excitation) and data analysis were performed using OriginPro 8.6 software. The Kds were obtained from data fitted to binding curves through the Hill model. (D) Cells were exposed to mock control or SARS-CoV-2 (CoV-2) in the presence of vehicle or soluble CD4 (sCD4) in different concentrations (200, 100, or 50 μg/ml) for 1 hr under continuous agitation. Viral load was assessed by RT-qPCR 24 hr after infection. (E) Primary human CD4+ T cells were incubated with IgG control, or monoclonal anti-CD4 (RPA- T4) antibody 18 hr prior to exposure with mock control or CoV-2. Viral load was determined by RT-qPCR 24 hr after infection. (F) A2.01 and A3.01 lineages were cultivated with pseudotype virus (VSV-mCherry-CoV-2). Percentage of infected cells and flow cytometry analysis. (G) CD4 abundance by western blotting (upper panel) and flow cytometry (lower panel). (H) Viral load of CoV-2 in A2.01 and A2.04. (I) Peripheral blood CD4+ T cells were incubated with IgG control or anti-ACE2 (αACE2) polyclonal antibody 18 hr prior to exposure with mock control or CoV-2 for 1 hr. Viral load was determined 24 hr after infection. (J) CD4+ T cells were incubated with vehicle or camostat mesylate for 18 hr before the exposure with mock control or CoV-2 for 1 hr. Viral load was analyzed by RT-qPCR. Data represents mean ± SEM of at least two independent experiments performed in triplicate. *p<0.05, **p<0.01, ***p<0.0001.
-
Figure 3—source data 1
Original co-immunoprecipitation blot.
Recombinant sCoV-2 and CD4 were co-incubated and immunoprecipitated with anti-CD4.
- https://cdn.elifesciences.org/articles/84790/elife-84790-fig3-data1-v1.zip
-
Figure 3—source data 2
Original ACE2 abundance by western blotting in T cell lines.
- https://cdn.elifesciences.org/articles/84790/elife-84790-fig3-data2-v1.zip
-
Figure 3—source data 3
Original CD4 abundance by western blotting in T cell lines.
- https://cdn.elifesciences.org/articles/84790/elife-84790-fig3-data3-v1.zip
-
Figure 3—source data 4
Original GAPDH abundance by western blotting in T cell lines.
- https://cdn.elifesciences.org/articles/84790/elife-84790-fig3-data4-v1.zip
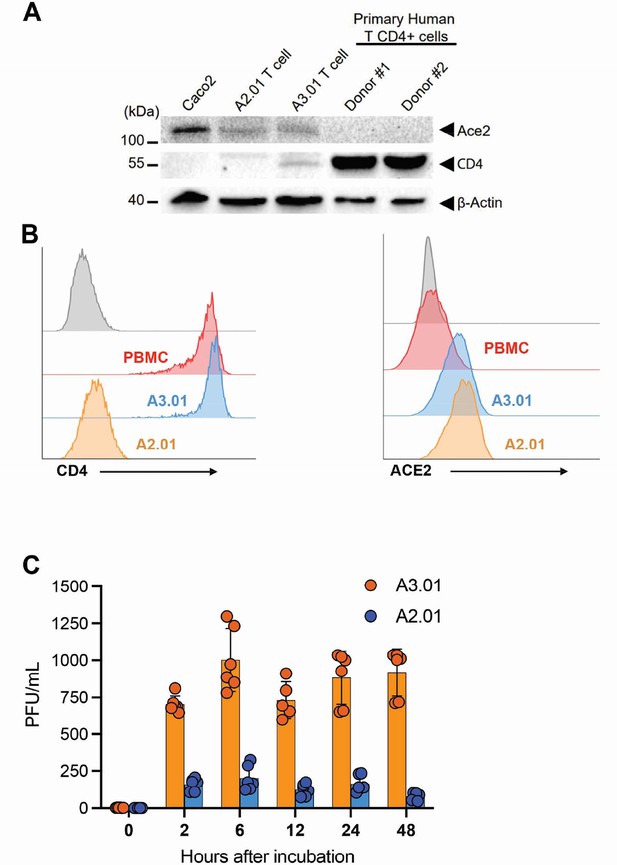
Implication of ACE2 and CD4 expression profile for SARS-CoV-2 infection dynamics.
(A) Western blotting of ACE2 and CD4 in Caco-2, A2.01, A3.01 cells, and CD4+ T lymphocytes. (B) Flow cytometry analysis of CD4 (left) and ACE2 (right) expression primary CD4+ T cells (peripheral blood mononuclear cell [PBMC]), A2.01 and A3.01. (C) Temporal viral load of SARS-CoV-2 in A2.01 and A3.01 lineages cells.
-
Figure 3—figure supplement 1—source data 1
Western blotting of ACE2 in Caco-2, A2.01, A3.01, and CD4+ T lymphocytes.
- https://cdn.elifesciences.org/articles/84790/elife-84790-fig3-figsupp1-data1-v1.zip
-
Figure 3—figure supplement 1—source data 2
Western blotting of CD4 in Caco-2, A2.01, A3.01, and CD4+ T lymphocytes.
- https://cdn.elifesciences.org/articles/84790/elife-84790-fig3-figsupp1-data2-v1.zip
-
Figure 3—figure supplement 1—source data 3
Western blotting of B-actin in Caco-2, A2.01, A3.01, and CD4+ T lymphocytes.
- https://cdn.elifesciences.org/articles/84790/elife-84790-fig3-figsupp1-data3-v1.zip

Infection of CD4+ T cells by SARS-CoV-2 alters cell function and triggers IL-10 production.
(A) Heatmap of differentially expressed proteins and their associated biological processes. (B) Network of differentially expressed proteins and their associated biochemical pathways. (C) Relative expression of IFNγ, IL-17A, TGFβ, and IL-10 genes in peripheral blood CD3+ CD4+ cells from COVID-19 patients (moderate or severe) and healthy control (HC). Data represents mean ± SEM. Each dot representes a patient sample. (D) Relative expression of IL-10 gene in primary CD4+ T cells infected with SARS-CoV-2. (E) Representative immunoblotting of phosphorylated CREB-1Ser133 (pCREB) and total or phosphorylated STAT3 in peripheral blood CD3+ CD4+ exposed to mock control or SARS-CoV-2. Phosphorylated ATF-1 is detected by the same antibody used to detect pCREB. ***p<0.001, **p<0.01, *p<0.05.
-
Figure 4—source data 1
Original immunoblotting of phosphorylated CREB-1Ser133 (pCREB) and phoshorylated ATF-1.
- https://cdn.elifesciences.org/articles/84790/elife-84790-fig4-data1-v1.zip
-
Figure 4—source data 2
Original immunoblotting of phosphorylated STAT3.
- https://cdn.elifesciences.org/articles/84790/elife-84790-fig4-data2-v1.zip
-
Figure 4—source data 3
Original immunoblotting of total STAT3.
- https://cdn.elifesciences.org/articles/84790/elife-84790-fig4-data3-v1.zip
-
Figure 4—source data 4
Original immunoblotting of Vinculin.
- https://cdn.elifesciences.org/articles/84790/elife-84790-fig4-data4-v1.zip

Analysis of differentially expressed proteins and their impact on biological processes.
(A) Foam Tree according to the biological processes affected by the differentially expressed proteins. (B) Network of enriched terms colored by cluster ID; nodes that share the same cluster ID are typically close to each other. Bar graph of enriched terms across input gene lists, colored by p-values.

Functional protein network and enrichment analysis in infected CD4+ T cells.
(A) Functional interaction network of proteins (identified by their gene name). (B) Protein interaction (identified by their gene name) of significantly altered proteins in infected CD4+ T cells with 0.7 confidence score and functional enrichment for top 5 Gene Ontology terms. Node size is related to p-value, and node colors show the direction of expression.
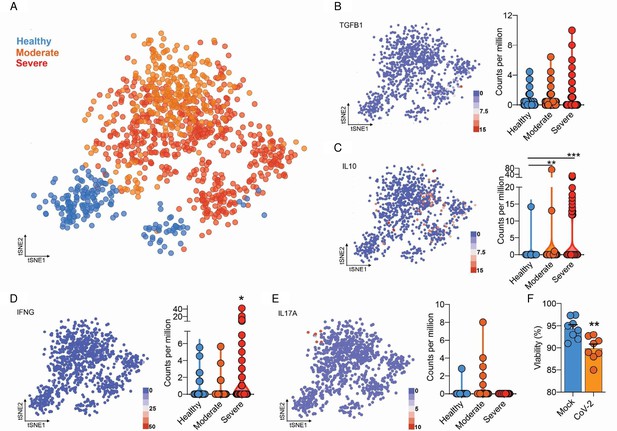
Integrated analysis of BAL CD4+ T cells shows immune dysregulation in COVID-19 patients.
(A) Flowchart of the of t-SNE-based analysis of human BAL (subclustered of CD4+ T cells) of healthy, moderate, and severe COVID-19 patients. (B) Gene expression of TGFB1 in counts per million. (C) Gene expression of IL10 in counts per million. (D) Gene expression of IFNG in counts per million. (E) Gene expression of IL17A in counts per million. Each dot represents a cell. (F) Viability of CD4+ T cells 24 hr after incubation with mock (blue) or CoV-2 (orange). ***p<0.001, **p<0.01, *p<0.05.
Additional files
-
Supplementary file 1
Clinical data about cohorts.
Gender, age, and admittance oxygen saturation were evaluated.
- https://cdn.elifesciences.org/articles/84790/elife-84790-supp1-v1.docx
-
Supplementary file 2
Detail results of mass spectrometry-based shotgun proteomics performed in CD4+ T cells exposed to SARS-CoV-2 ex vivo.
- https://cdn.elifesciences.org/articles/84790/elife-84790-supp2-v1.xls
-
Supplementary file 3
Information about antibodies used in the article.
- https://cdn.elifesciences.org/articles/84790/elife-84790-supp3-v1.xlsx
-
Supplementary file 4
Cycle thresholds (Cts) obtained during qPCR performances in CD4 + and CD8 + T lymphocytes infection (Figure 1A) and CD4+ T cell temporal infection (Figure 1D).
- https://cdn.elifesciences.org/articles/84790/elife-84790-supp4-v1.xlsx
-
MDAR checklist
- https://cdn.elifesciences.org/articles/84790/elife-84790-mdarchecklist1-v1.pdf