OpenApePose, a database of annotated ape photographs for pose estimation
Figures
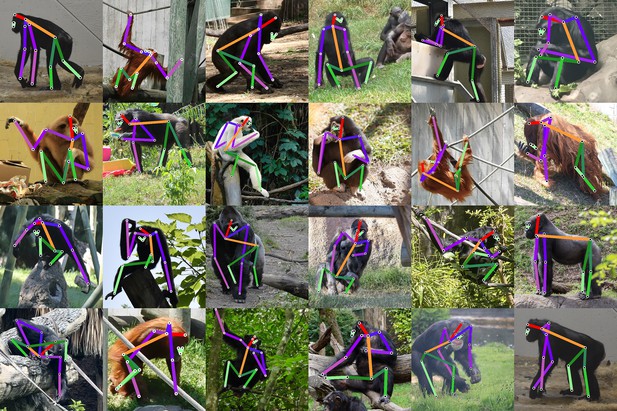
Sampling of annotated images in the OpenApePose dataset.
Thirty-two photographs chosen to illustrate the range of photographs available in our larger set, illustrating the variety in species, pose, and background. Each annotated photograph contains an annotation for sixteen different body landmarks (shown here with connecting lines).
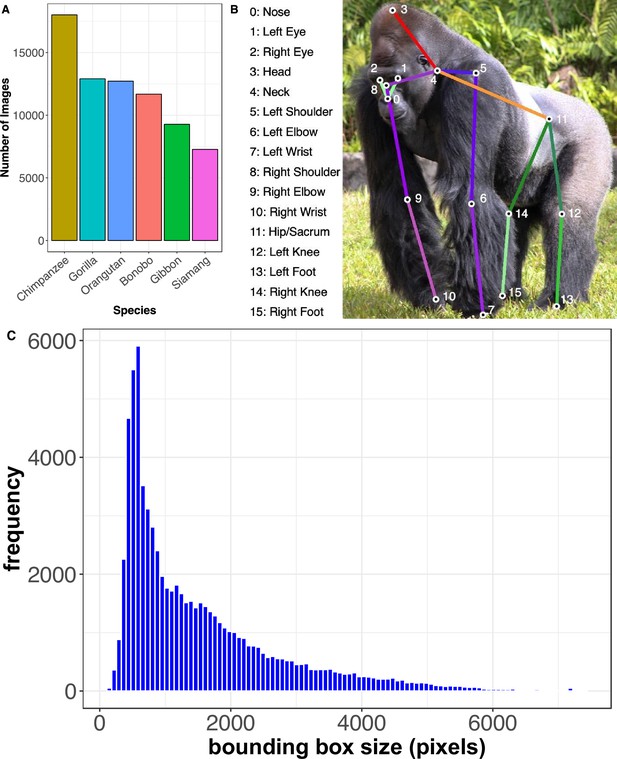
Properties of the OpenApePose database.
(A) Number of annotated images per different species in the OpenApePose dataset. (B) Illustration of our annotations. All 16 annotated points are indicated and labeled on a gorilla image drawn from the database. (C) Histogram of bounding box sizes in the database as defined as length of the bounding box diagonal in pixels.
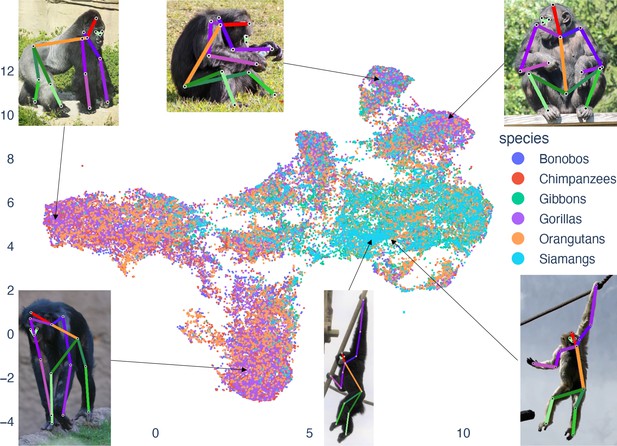
Uniform Manifold Approximation and Projection (UMAP) visualization of the distribution of poses with the species IDs labeled.
X- and Y-dimensions indicate positions in a UMAP space. Each dot indicates a single photograph/pose. Dot colors indicate species (see inscribed legend, right). We include, as insets, example poses, with an arrow pointing to their position in the UMAP plot.
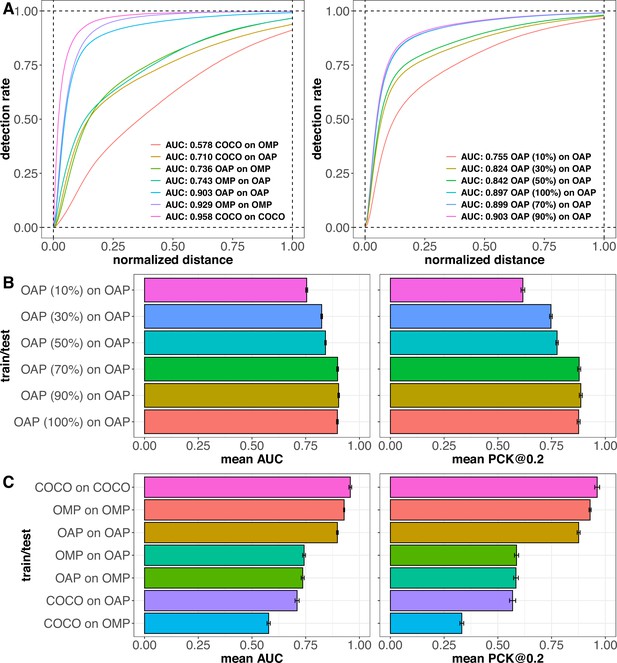
Keypoint detection performance of HRNet-W48 models on different datasets.
(A) Keypoint detection performance of HRNet-W48 models measured using percent correct keypoints (PCK) values at different thresholds. Left: models trained on the full training sets of COCO, OpenApePose (OAP), and OpenMonkeyPose (OMP), and tested on the same dataset, as well as across datasets. Right: models trained on different sizes of the full OAP training set, and tested on the OAP testing set. (B) Barplots showing the keypoint detection performance of state-of-the-art (HRNet-W48) models as measured using percent keypoints correct at 0.2 (PCK@0.2) and area under the curve (AUC) of the PCK curves at thresholds ranging from 0.01 to 1. Error bars: standard deviation of the performance metrics. Models are trained on different sizes of the full training set of OAP and tested on held-out OAP test sets. (C) Same as (B) but models are trained on full training sets of COCO, OAP, and OMP, and tested on the same dataset, as well as across datasets.
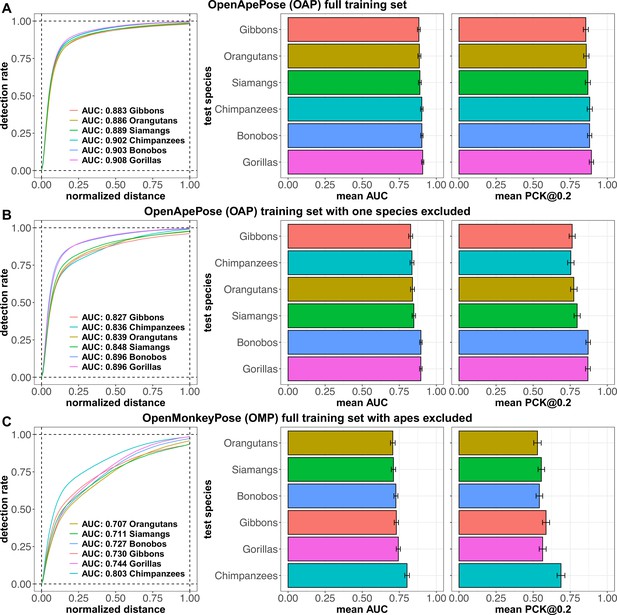
Keypoint detection performance of HRNet-W48 models tested on each species from the OpenApePose (OAP) test set and trained on (A) the full OAP training set, (B) the OAP training set with the corresponding species excluded, and (C) the full OpenMonkeyPose (OMP) dataset with apes excluded.
Left panel includes the probability of correct keypoint (PCK) values at different thresholds ranging from 0 to 1. Middle panel indicates the mean area under the PCK curve for each species. Right panel indicates the mean PCK values at a threshold of 0.2 for each species.
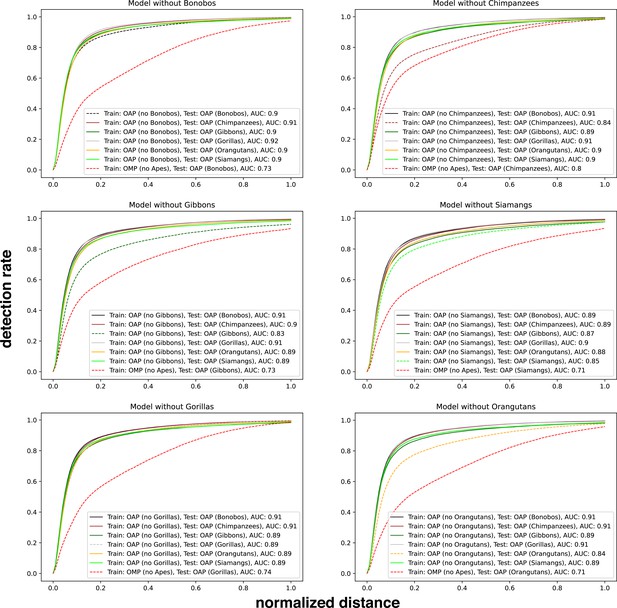
The probability of correct keypoint (PCK) values (y-axis) at different thresholds ranging from 0 to 1 (x-axis) of HRNet-W48 models tested on each species from the OpenApePose (OAP) test set and trained on the OAP training set with the corresponding species excluded.
Dotted lines indicate the performance on the species excluded from training in the case of OAP and the performance of the OpenMonkeyPose model trained on monkeys on the excluded species.
Videos
Demonstration of the OpenApePose model capabilities on inferences on videos.
The video clip is analyzed using the mmpose and mmdetection libraries—mmdetection infers a bounding box around the ape and mmpose uses the OpenApePose model to infer the pose in each frame.
Additional files
-
Supplementary file 1
Datasheet for the OpenApePose dataset.
- https://cdn.elifesciences.org/articles/86873/elife-86873-supp1-v1.docx
-
Supplementary file 2
Tables S1 and S2.
(a) Pairwise t-tests comparing the AUC values for different training and testing set combinations. AUCs were obtained across 100 random samples of 500 images from the test sets. p-Values adjusted for multiple comparisons using Bonferroni correction. ** not significant. (b) Pairwise t-tests comparing the PCK@0.2 values for different training and testing set combinations. PCK@0.2 values were obtained across 100 random samples of 500 images from the test sets. p-Values adjusted for multiple comparisons using Bonferroni correction. ** not significant
- https://cdn.elifesciences.org/articles/86873/elife-86873-supp2-v1.docx
-
Supplementary file 3
List of instructions sent to Hive annotators.
- https://cdn.elifesciences.org/articles/86873/elife-86873-supp3-v1.pdf
-
MDAR checklist
- https://cdn.elifesciences.org/articles/86873/elife-86873-mdarchecklist1-v1.docx