Statistical and computational methods for integrating microbiome, host genomics, and metabolomics data
Figures
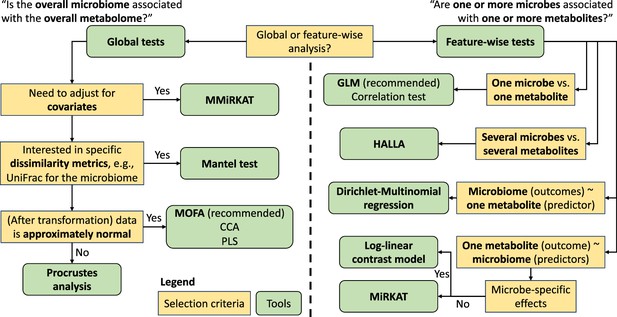
Flowchart of popular currently available methods and their best use cases.
Selection criteria are shown in yellow boxes and methods in green.
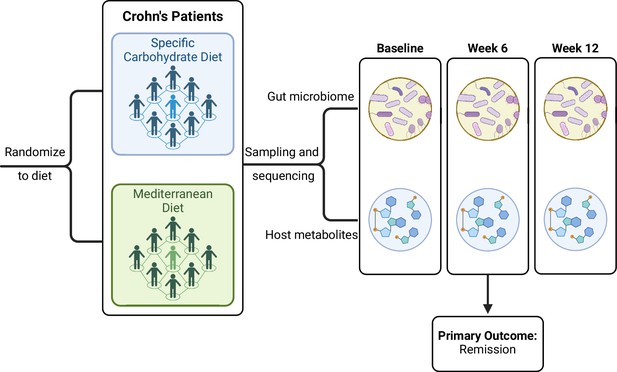
INducE remission in Crohn’s Disease (DINE-CD) study design diagram.
© 2024, BioRender Inc. Figure 2 was created using BioRender, and is published under a CC BY-NC-ND license. Further reproductions must adhere to the terms of this license.
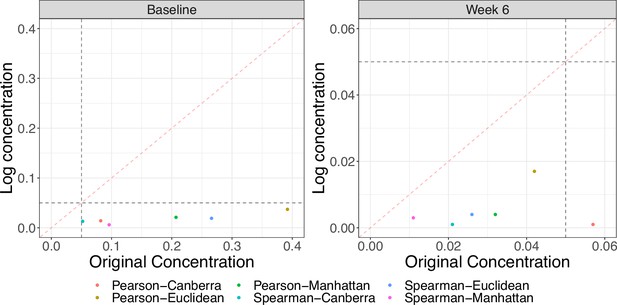
Comparison of Mantel test p-values using original and log-transformed metabolite concentrations at baseline and 6 weeks.
Gray dashed lines denote nominal significance at the 0.05 level and the red dashed line is the line. All tests are significant using the log-transformed data. There are large differences in p-values between the two scales, particularly at baseline.
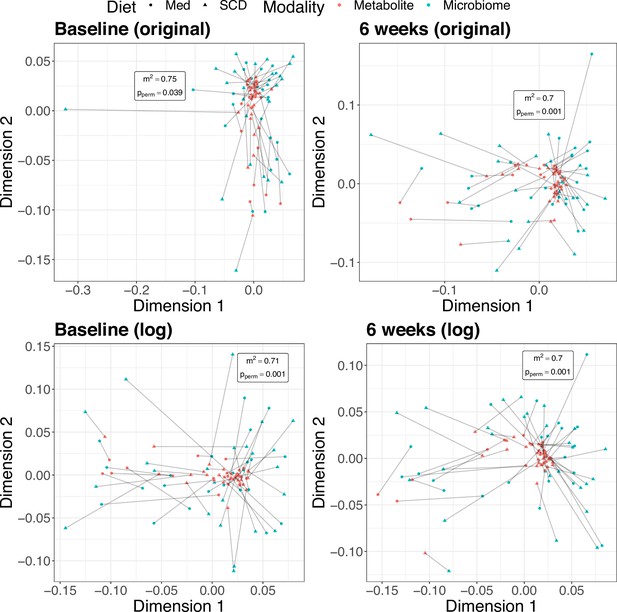
Multidimensional scaling (MDS) plots from Procrustes analysis.
Color indicates omics modality. Shape indicates diet, Mediterranean (Med) or specific carbohydrate (SCD). Lines connect samples from the same subject in the metabolomics and microbial sequencing data sets. The sum of these distances squared is smaller than expected by chance for both time points thus indicating a concordance.
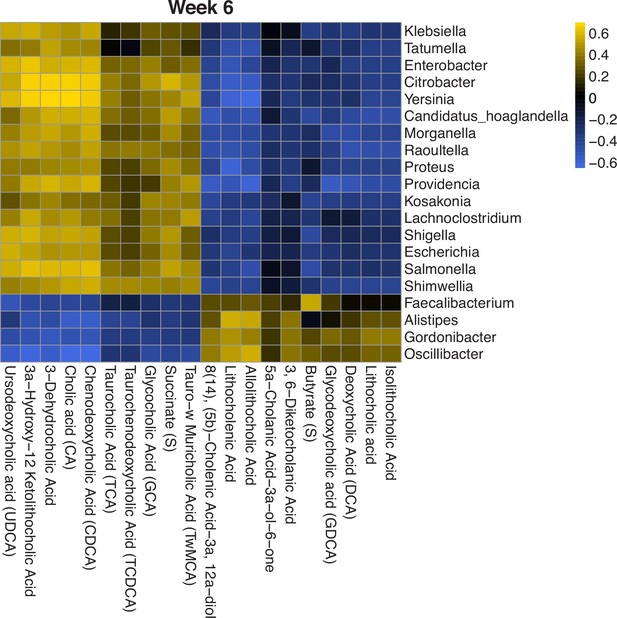
Heatmap of Spearman’s correlation between top metabolites and microbes contributing to the first latent factor identified by multiomics factor analysis (MOFA).
Correlations are calculated using log/clr-transformed data and clusters are identified from hierarchical clustering. There are four distinct microbe-metabolite clusters, two with positive correlations and two with negative correlations. clr, centered log-ratio.
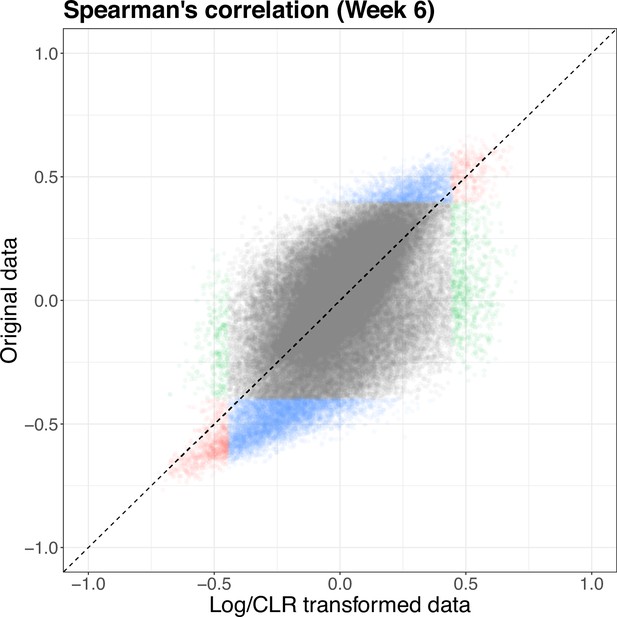
Scatterplot of Spearman’s correlation estimated on the original (y-axis) and log/clr-transformed (x-axis) data at 6 weeks.
Color denotes significance in log-transformed data (green), original data (blue), red = both (red). Identified significant pairs vary based on which data scale is used. clr, centered log-ratio.
Tables
Examples of available multiomics integration methods, along with main advantages and disadvantages, split by analysis type: global, feature-wise, network, longitudinal, mediation.
Analysis type | Methods | Advantages | Disadvantages |
---|---|---|---|
Global | Mantel test, multivariate MiRKAT, (sparse) CCA, (sparse) PLS, Procrustes analysis | Attributes variation in single analytes into other molecular modalities; extracts strongest signals for covariation between molecular profiles | Covariation signals can lack interpretability; microbiome-specific properties must be properly adjusted for with advanced methods |
Feature-wise | Pairwise correlation (Pearson, Spearman’s, and Kendall’s tau), MiRKAT, HAllA, log-linear contrast regression, Dirichlet-multinomial regression | Assumes ‘guilt-by-association’; individual tests easily implemented; appropriate for initial hypothesis generation | Potential correlation structure in molecular profiles must be adjusted for to control false discoveries |
Network | SPIEC-EASI (transkingdom), MIMOSA2, AMON, DIABLO, MiMeNet | Decipher complex interaction patterns between microbes and other molecular features; identify stable ‘hubs’ in community structures | Complex networks require regularization; differential network analysis to contrast between host conditions difficult to perform |
Longitudinal | Linear mixed effects models, GLMMlasso, dynamic Bayesian networks | Detects patterns in microbes and other analytes over time or space; facilitates understanding of causal relationship | Requires knowledge of directionality; regularization or FDR control is needed; large sample sizes needed |
Mediation | Linear structural equation models, compositional mediation analysis | Quantifies direct and indirect effects; incorporates demographic and/or clinical information | Prior knowledge of confounding and causal relationships are needed |
Correlations from Mantel tests at baseline and 6 weeks (W6) using three different distances for the original metabolite concentrations.
Microbial distance was measured using the Bray-Curtis distance. Both Pearson and Spearman’s correlations were assessed. Correlation and significance, denoted by *, estimates vary based upon choice of distance and correlation type.
Metabolite distance | Correlation | Baseline | W6 |
---|---|---|---|
Euclidean | Pearson | 0.022 | 0.222* |
Manhattan | Pearson | 0.083 | 0.239* |
Canberra | Pearson | 0.148 | 0.141 |
Euclidean | Spearman | 0.061 | 0.244* |
Manhattan | Spearman | 0.146 | 0.266* |
Canberra | Spearman | 0.153 | 0.171* |
MMiRKAT p-values across several different coefficient of variation quantile cutoffs to select the most variable metabolites using the original and log-transformed metabolite concentrations.
Bray-Curtis distance is used for microbiome data.
Quantile (q) | Baseline | Week 6 | ||
---|---|---|---|---|
Original | Log | Original | Log | |
q = 0.5 | 0.163 | 0.170 | 0.064 | 0.250 |
q = 0.6 | 0.158 | 0.092 | 0.016 | 0.174 |
q = 0.7 | 0.109 | 0.166 | 0.003 | 0.101 |
q = 0.8 | 0.501 | 0.182 | 0.001 | 0.011 |
q = 0.9 | 0.834 | 0.030 | <0.001 | 0.004 |
MMiRKAT p-values across several different pseudocounts for the log-transformation of metabolite concentrations.
Coefficient of variation quantile cutoff is held constant at 0.9. Bray-Curtis distance is used for microbiome data. Choice of pseudocount influences p-value and significance.
Pseudocount | Baseline | Week 6 |
---|---|---|
1×10-6 | 0.220 | 0.018 |
1×10-5 | 0.005 | 0.003 |
1×10-4 | 0.020 | 0.008 |
1×10-3 | 0.030 | 0.004 |
1×10-2 | 0.156 | 0.010 |
Additional files
-
Supplementary file 1
Pairwise correlations.
Table of estimated Pearson and Spearman’s correlations for all microbe and metabolite pairs. Correlations are calculated at baseline and 6 weeks using the original and log-transformed data.
- https://cdn.elifesciences.org/articles/88956/elife-88956-supp1-v1.xlsx
-
Supplementary file 2
Hierarchical all-against-all (HAllA) clusters.
Table of significant HAllA clusters and their associated adjusted p-value.
- https://cdn.elifesciences.org/articles/88956/elife-88956-supp2-v1.xlsx
-
Supplementary file 3
Linear regression coefficients.
Table of regression coefficients, standard errors, z-scores, and p-values from the model regressing each metabolite on each microbe and prior surgery status. Adjusted p-values, after false discovery rate (FDR) correction at the 0.05 level, for the microbe coefficient are reported as well. Models are fit at both baseline and week 6.
- https://cdn.elifesciences.org/articles/88956/elife-88956-supp3-v1.xlsx