Predicting the sequence-dependent backbone dynamics of intrinsically disordered proteins
Figures

Clock-like tree plot showing lack of homology among the 45 IDPs.
The level of homology between two sequences is measured by the distance from their convergence point to the center of the clock. The highest level of apparent identity is between A1-LCD and TDP-43, at 25%, but these two proteins differ in both secondary structure formation and characteristics. There is, however, a 20-residue overlap between the N-terminus of MBP-xα2 and the C-terminus of rmBG21.

Representative conformations of five IDPs.
(A–E) MKK4, α-synuclein, Mev-PNTD, Sev-NT, and CBP-ID4. Conformations were initially generated using TraDES (http://trades.blueprint.org; Feldman and Hogue, 2002), selected to have radius of gyration close to predicted by a scaling function (Å) (Bernadó and Blackledge, 2009). Conformations for residues predicted as helical by PsiPred plus filtering were replaced by an ideal helix. Finally residues are colored according to a scheme ranging from green for low predicted to red for high predicted .
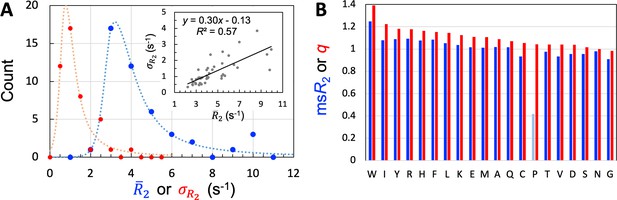
Properties of the 45 IDPs in the training set.
(A) Histograms of means and standard deviations, calculated for individual proteins. Curves are drawn to guide the eye. Inset: correlation between and . (B) Experimental mean scaled () and SeqDYN parameters, for the 20 types of amino acids. Note that Pro residues have low for the lack of backbone amide proton. Amino acids are in descending order of .
-
Figure 3—source data 1
Source data for Figure 3.
- https://cdn.elifesciences.org/articles/88958/elife-88958-fig3-data1-v1.xlsx
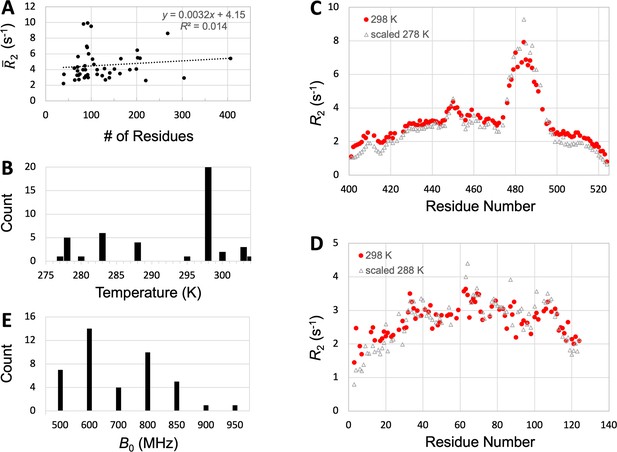
Possible effects of sequence length, temperature, and magnetic field on .
(A) Lack of dependence of on sequence length. (B) Counts of IDPs with measured at various temperatures. (C) Matching of profiles of Sev-NT measured at two temperatures after uniform scaling. (D) Matching of profiles of A1-LCD measured at two temperatures after uniform scaling. (E) Counts of IDPs with measured at various magnetic fields.
-
Figure 3—figure supplement 1—source data 1
Source data for Figure 3—figure supplement 1.
- https://cdn.elifesciences.org/articles/88958/elife-88958-fig3-figsupp1-data1-v1.xlsx
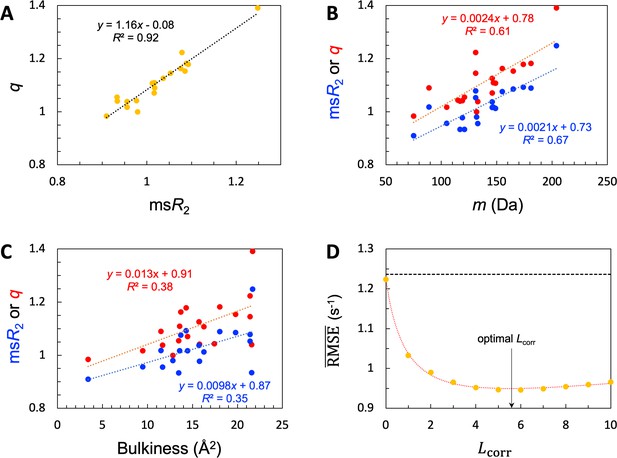
SeqDYN model parameters.
(A) Correlation between and . The values are also displayed as bars in Figure 3B. (B) Correlation of and with amino-acid molecular mass. (C) Correlation of and with bulkiness. (D) The optimal correlation length and deterioration of SeqDYN prediction as the correlation length is moved away from the optimal value.
-
Figure 4—source data 1
Source data for Figure 4.
- https://cdn.elifesciences.org/articles/88958/elife-88958-fig4-data1-v1.xlsx
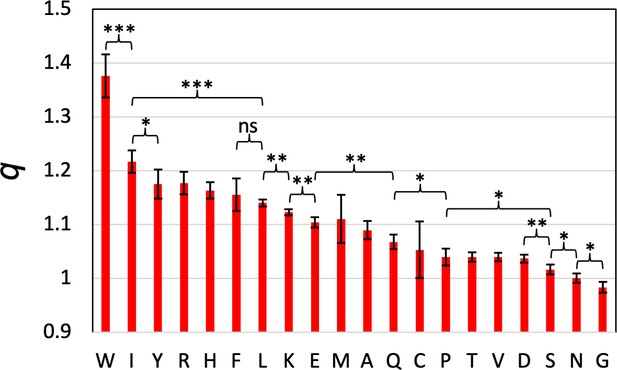
T-test of on the parameters for pairs of amino acids.
parameters were obtained from five-fold cross-validation training, resulting in five independent values for each parameter. Mean presented as red bars; standard deviation presented as error bar. *, p<0.05; **, p<0.01; ***, p<0.001; ns, not significant. parameters for all neighboring pairs not explicitly indicated are not significantly different.
-
Figure 4—figure supplement 1—source data 1
Source data for Figure 4—figure supplement 1.
- https://cdn.elifesciences.org/articles/88958/elife-88958-fig4-figsupp1-data1-v1.xlsx
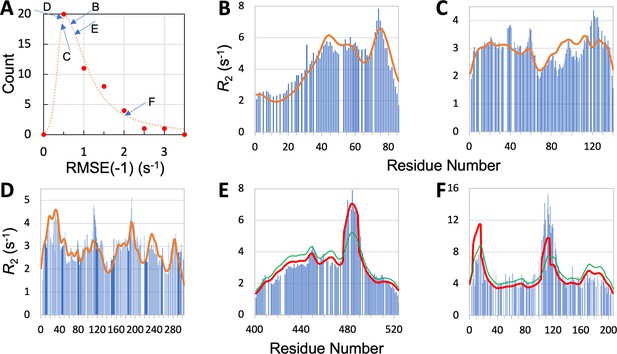
Quality of SeqDYN predictions.
(A) Histogram of RMSE(–1). Letters indicate RMSE(–1) values of the IDPs to be presented in panels (B–F). (B–F) Measured (bars) and predicted (curves) profiles for MKK4, α-synuclein, Mev-PNTD, Sev-NT, and CBP-ID4. In (E) and (F), green curves are SeqDYN predictions and red curves are obtained after a helix boost.
-
Figure 5—source data 1
Source data for Figure 5.
- https://cdn.elifesciences.org/articles/88958/elife-88958-fig5-data1-v1.xlsx
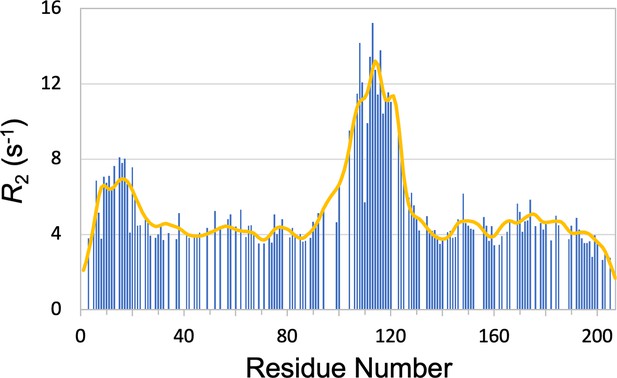
Close reproduction (curve) of the measured profile (bars) of CBP-ID4 when that set of data alone was used to parameterize SeqDYN.
The resulting model has no value for predicting for other proteins.
-
Figure 5—figure supplement 1—source data 1
Source data for Figure 5—figure supplement 1.
- https://cdn.elifesciences.org/articles/88958/elife-88958-fig5-figsupp1-data1-v1.xlsx
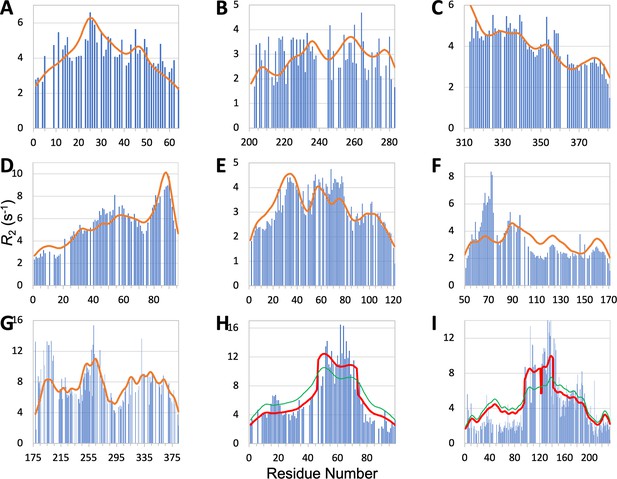
Measured (bars) and predicted (curves) profiles for ChiZ N-terminal region, TIA1 prion-like domain, Pdx1 C-terminal region, synaptobrevin-2, α-endosulfine, YAP, AMOTL1, FtsQ, and CAHS-8.
In (C), does not fall off at the N-terminus because the sequence is preceded by an expression tag MGSSHHHHHHHHHHHHS. In (H) and (I), green curves are SeqDYN predictions and red curves are obtained after a helix boost.
-
Figure 6—source data 1
Source data for Figure 6.
- https://cdn.elifesciences.org/articles/88958/elife-88958-fig6-data1-v1.xlsx
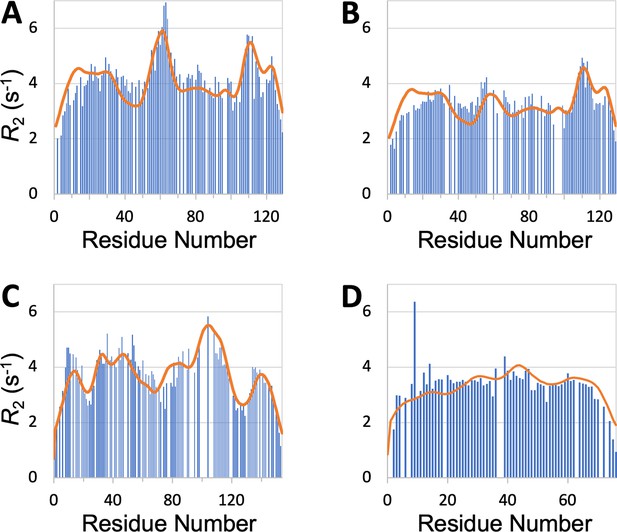
profiles predicted (curves) by SeqDYN show close agreement with those measured (bars) on structured proteins in the unfolded state.
(A) Wild-type lysozyme (8 M urea; pH 2; cysteine-methylated). (B) Lysozyme with Trp62 to Gly mutation (pH 2). Methylated cysteines were treated as Ala in the SeqDYN predictions. (C) Apomyoglogin (8 M urea; pH 2.3). (D) Ubiquitin (8 M urea; pH 2).
-
Figure 7—source data 1
Source data for Figure 7.
- https://cdn.elifesciences.org/articles/88958/elife-88958-fig7-data1-v1.xlsx
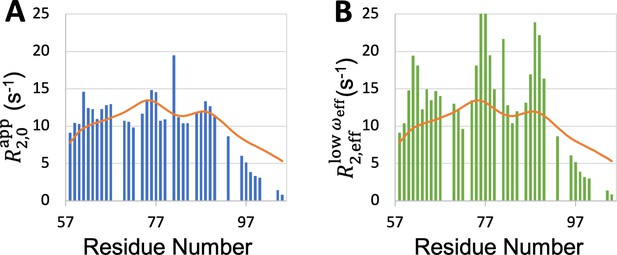
Comparison between SeqDYN prediction (curves) and effective transverse relaxation rate (bars) from 1H dispersion relaxation experiment.
(A) in the high- limit. (B) at low .
-
Figure 8—source data 1
Source data for Figure 8.
- https://cdn.elifesciences.org/articles/88958/elife-88958-fig8-data1-v1.xlsx
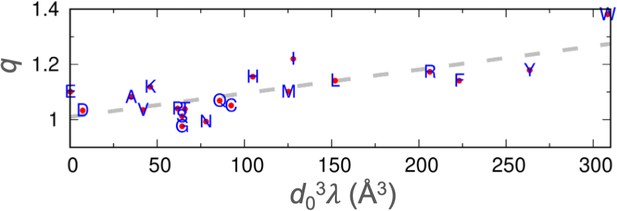
Correlation between the stickiness parameters (λ) and the NMR relaxation parameters (q).
The regression line is shown as dashes.
-
Figure 9—source data 1
Source data for Figure 9.
- https://cdn.elifesciences.org/articles/88958/elife-88958-fig9-data1-v1.xlsx
Tables
Experimental conditions, mean and standard deviation of measured , and SeqDYN prediction RMSE.
Protein name | # of res | Temp(K) | B0 (MHz) | (s–1) | (s–1) | RMSE(s–1) | PMID; ref |
---|---|---|---|---|---|---|---|
Training set (45 IDPs) * | |||||||
A1-LCD | 131 | 298 | 800 | 2.68 | 0.46 | 0.60 | 32029630; Martin et al., 2020 |
Aβ40 | 40 | 278 | 600 | 3.40 | 0.92 | 0.38 | 31181936; Rezaei-Ghaleh et al., 2019 |
Ash1 | 83 | 278 | 800 | 9.80 | 1.40 | 1.41 | 27807972; Martin et al., 2016 |
Beclin1 | 165 | 288 | 800 | 5.37 | 1.03 | 1.14 | 27288992; Yao et al., 2016 |
CAPRIN1 | 103 | 303 | 600 | 5.34 | 0.88 | 0.72 | 31898464; Wong et al., 2020 |
CBP-ID4 | 207 | 283 | 700 | 5.45 | 2.55 | 2.01;1.90† | 29790640; Murrali et al., 2018 |
GbnD4-DHD | 91 | 280 | 700 | 6.81 | 1.55 | 1.28 | 29309054; Jenner et al., 2018 |
ERD14 | 185 | 288 | 600 | 3.96 | 0.87 | 0.54 | 21336827; Szalainé Ágoston et al., 2011 |
ExsE | 88 | 298 | 600 | 3.18 | 0.88 | 0.76 | 22138394; Zheng et al., 2012 |
FCP1 | 85 | 298 | 500 | 2.94 | 0.54 | 0.43 | 26286791; Lawrence and Showalter, 2012 |
FUS | 163 | 298 | 850 | 3.48 | 0.51 | 0.54 | 26455390; Burke et al., 2015 |
GAb1 | 82 | 298 | 500 | 3.99 | 0.88 | 0.89 | 34929201; Gruber et al., 2022 |
hACTR | 69 | 304 | 600 | 3.26 | 0.47 | 0.49 | 18177052; Ebert et al., 2008 |
Hahellin | 92 | 298 | 800 | 9.94 | 2.69 | 2.85 | 24671380; Patel et al., 2014 |
hCSD1 | 141 | 298 | 500 | 3.56 | 0.93 | 0.99 | 18537264; Kiss et al., 2008 |
HOX-DFD | 90 | 298 | 600 | 6.98 | 3.15 | 1.99 | 30802457; Maiti et al., 2019 |
hZIP4-ICL2 | 100 | 283 | 800 | 9.54 | 2.37 | 1.58 | 30793391; Bafaro et al., 2019 |
Jaburetox | 94 | 298 | 800 | 6.01 | 2.30 | 2.27 | 25605001; Lopes et al., 2015 |
KRS-NT | 72 | 303 | 600 | 3.26 | 0.93 | 0.83 | 24983501; Cho et al., 2014 |
MBP-xα2 | 70 | 295 | 600 | 3.83 | 0.60 | 0.54 | 25343306; De Avila et al., 2014 |
MKK4 | 86 | 278 | 850 | 4.49 | 1.42 | 0.63 | 29276882; Delaforge et al., 2018 |
N-Cby | 63 | 298 | 4.19 | 1.20 | 1.25 | 21182262; Mokhtarzada et al., 2011 | |
Niv-PNTD | 406 | 288 | 700 | 5.41 | 1.82 | 1.66 | 33177626; Schiavina et al., 2020 |
NS5A-D2D3 | 268 | 278 | 800 | 8.62 | 3.85 | 2.14 | 26445449; Sólyom et al., 2015 |
NUPR1 | 93 | 298 | 600 | 2.98 | 0.82 | 0.76 | 31325636; Neira et al., 2019 |
OPN | 220 | 310 | 800 | 2.59 | 0.82 | 0.54 | 31794728; Mateos et al., 2020 |
p53TAD | 73 | 298 | 850 | 2.72 | 0.66 | 0.33 | 30240067; Xie et al., 2018 |
PDEγ | 87 | 298 | 3.96 | 1.05 | 0.71 | 18230733; Song et al., 2008 | |
PKIα | 75 | 300 | 900 | 3.41 | 0.87 | 0.52 | 32338601; Olivieri et al., 2020 |
Mev-PNTD | 304 | 298 | 950 | 2.92 | 0.59 | 0.48 | 30140745; Milles et al., 2018 |
ProTα | 113 | 283 | 800 | 3.40 | 0.56 | 0.43 | 29466338; Borgia et al., 2018 |
Pup | 64 | 298 | 850 | 2.66 | 0.51 | 0.43 | 30240067; Xie et al., 2018 |
rmBG21 | 199 | 300 | 600 | 4.06 | 0.90 | 0.63 | 17676872; Ahmed et al., 2007 |
RPB1 | 201 | 277 | 850 | 6.48 | 1.74 | 1.33 | 28945358; Janke et al., 2018 |
securin | 202 | 283 | 500 | 5.49 | 1.13 | 1.08 | 19053469; Csizmok et al., 2008 |
Sev-NT | 124 | 298 | 600 | 3.20 | 1.42 | 0.76;0.38† | 27112095; Abyzov et al., 2016 |
Sic1 | 92 | 278 | 500 | 3.34 | 0.59 | 0.48 | 20399186; Mittag et al., 2010 |
SKIPN | 71 | 298 | 5.64 | 1.05 | 1.46 | 20007319; Wang et al., 2010 | |
SLBP-NT | 113 | 298 | 600 | 3.96 | 1.40 | 1.61 | 15260482; Thapar et al., 2004 |
α-synuclein | 140 | 298 | 600 | 2.96 | 0.53 | 0.44 | 30184304; Rezaei-Ghaleh et al., 2018 |
SOCS5-JIR | 70 | 303 | 800 | 4.32 | 2.36 | 1.91 | 26173083; Chandrashekaran et al., 2015 |
tau K18 | 129 | 283 | 700 | 4.12 | 0.95 | 0.83 | 23740819; Barré and Eliezer, 2013 |
TC1 | 106 | 298 | 600 | 4.65 | 1.61 | 1.24 | 23189168; Cino et al., 2012 |
TDP-43 | 151 | 283 | 500 | 4.07 | 1.51 | 0.96 | 27545621; Conicella et al., 2016 |
γ-tubulin-CT | 39 | 288 | 500 | 2.23 | 0.35 | 0.27 | 29127738; Harris et al., 2018 |
Test set (9 IDPs) | |||||||
AMOTL1 | 207 | 283 | 800 | 8.45 | 2.55 | 2.04 | 35481651; Vogel et al., 2022 |
CAHS-8 | 233 | 303 | 850 | 4.43 | 3.25 | 2.36;1.92† | 34750927; Malki et al., 2022 |
ChiZ | 64 | 298 | 800 | 4.33 | 0.89 | 0.74 | 32585849; Hicks et al., 2020 |
α-endosulfine | 121 | 298 | 800 | 3.21 | 0.81 | 0.48 | 34346186; Thapa et al., 2022 |
FtsQ | 99 | 305 | 800 | 6.44 | 3.78 | 2.32;1.71† | 36959324; Smrt et al., 2023 |
Pdx1 | 83 | 298 | 500 | 2.98 | 0.70 | 0.76 | 30525611; Cook et al., 2019 |
synaptobrevin-2 | 96 | 278 | 600 | 5.54 | 1.80 | 0.72 | 30975750; Lakomek et al., 2019 |
TIA-1 | 91 | 310 | 800 | 4.01 | 0.89 | 0.55 | 36112647; Sekiyama et al., 2022 |
YAP | 122 | 298 | 800 | 3.19 | 1.44 | 1.23 | 35378854; Feichtinger et al., 2022 |
-
*
For training set, RMSE is calculated for prediction based on leave-one-out training (using 44 IDPs).
-
†
First number is for SeqDYN prediction; second number is after applying a helix boost.
RMSEs (s–1) of predictions by SeqDYN and MD for 10 IDPs.
IDP name | SeqDYN | MD |
---|---|---|
A1-LCD | 0.60* | 0.59 §, ¶ |
Aβ40 | 0.38* | 0.38 § |
HOX-DFD | 1.99* | 1.40 § |
α-synuclein | 0.44* | 0.50 § |
p53TAD | 0.33* | 1.04 ** |
Pup | 0.43* | 1.00** |
Sev-NT | 0.38*,† | 1.10 §,†† |
tau K18 | 0.83* | 0.80 § |
ChiZ | 0.74 ‡ | 1.40 ‡ ‡ |
FtsQ | 1.71 §,† | 1.70 § § |
-
*
Based on leave-one-out training (using 44 IDPs).
-
†
Helix boost applied.
-
‡
Based on training by the full training set (45 IDPs).
-
§
From Dey et al., 2022.
-
¶
RMSE is scaled down by a factor of 2.39, to correct for the effect of temperature (MD at 288 K; see Figure 3—figure supplement 1C).
-
**
-
††
RMSE is scaled down by a factor of 2.99, to correct for the effects of temperature and magnetic field (MD at 274 K and 850 MHz; see Figure 3—figure supplement 1B).
-
‡ ‡
Originally calculated in Hicks et al., 2020 with correction in Hicks et al., 2021.
-
§ §
From Smrt et al., 2023.