Automated cell annotation in multi-cell images using an improved CRF_ID algorithm
Figures
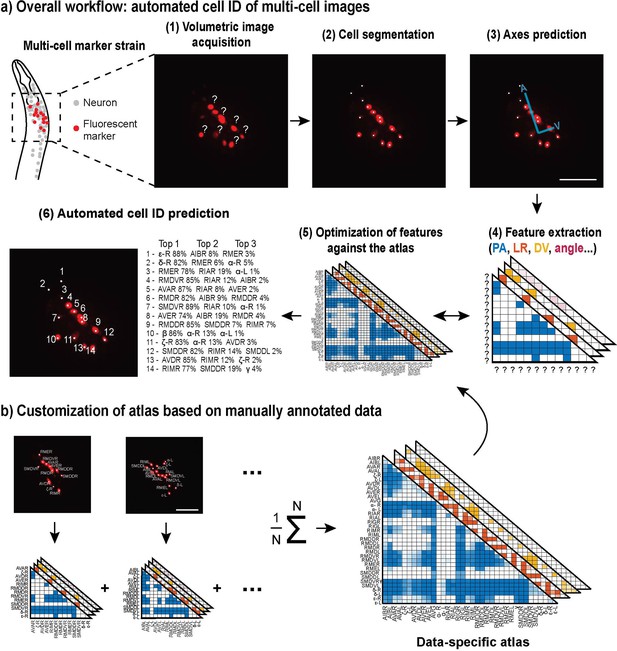
CRF_ID for multi-cell images.
(a) Computational workflow starting from image acquisition to final cell identity predictions. (1–3) Image preprocessing steps include automatic cell segmentation and coordinate axes prediction. (4) Feature variables that represent positional relationships of the cells are extracted (PA, posterior and anterior; LR, left and right; DV, dorsal and ventral). (5) The Conditional Random Fields (CRF) algorithm maximizes the similarities between the extracted features from the images and those from an atlas. (6) The final results are represented as a list of most likely neuron candidates for each cell with predicted probabilities. (b) The atlas can be customized to meet the specifications of the images, and this is easily done by compiling and averaging annotated data. The images are showing half volume (left or right side) of the specimen for illustration. Scale bar: 25 µm.
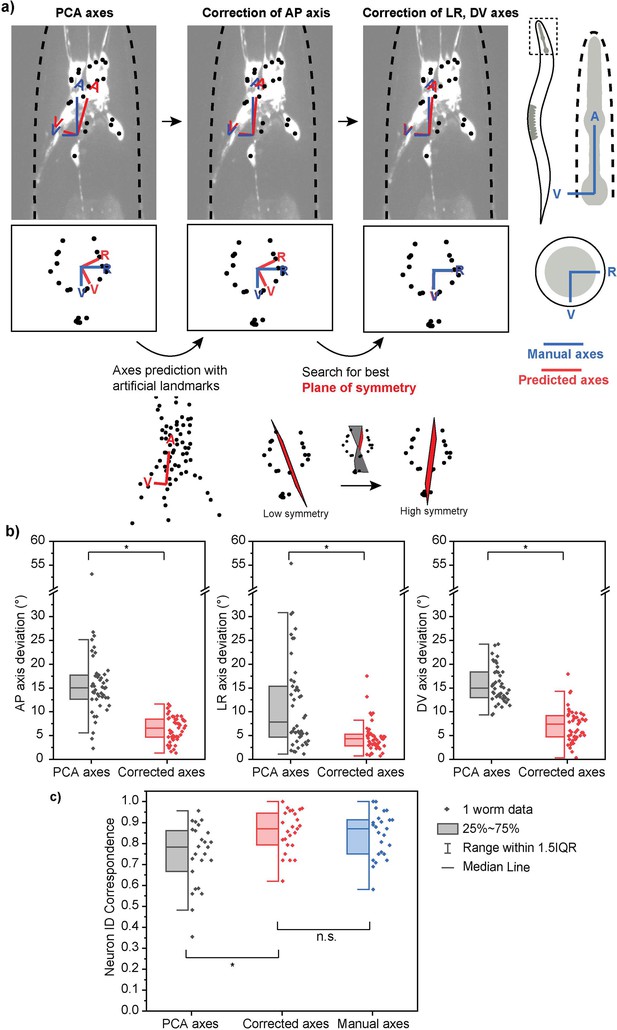
Improved method of assigning coordinate axes.
(a) Coordinate axes for multi-cell images generated by principal components analysis (PCA) alone are not accurate. A two-step correction process is implemented: correction of the AP axis by using natural landmarks and correction of LR, DV axes by searching for the best plane of symmetry. (b) The corrected axes are more accurate than the previous axes generated by PCA alone as they show decreased angle deviations from the ground truth axes for all three coordinate axes. (c) Corrected axes result in a higher and comparable neuron ID accuracy (correspondence to manual cell annotations) when compared with PCA predicted axes and ground truth axes, respectively. Best single prediction results are reported. Two-sample t-tests were performed for statistical analysis. The asterisk symbol denotes a significance level of p<0.05.
-
Figure 2—source data 1
Angle Deviations of PCA and Corrected Axes.
- https://cdn.elifesciences.org/articles/89050/elife-89050-fig2-data1-v1.xlsx
-
Figure 2—source data 2
Neuron ID Accuracy of PCA, Corrected, and Manual Axes.
- https://cdn.elifesciences.org/articles/89050/elife-89050-fig2-data2-v1.xlsx
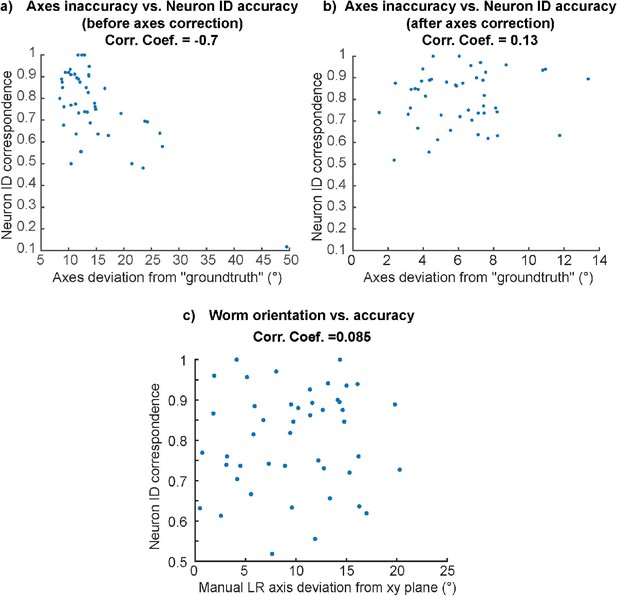
Neuron ID accuracy no longer depends on the axes inaccuracy after axes correction.
(a) High negative correlation between axes inaccuracy and neuron ID accuracy before axes correction. (b) No correlation between axes inaccuracy and neuron ID accuracy after axes correction. (c) No correlation between worm orientation and neuron ID accuracy.
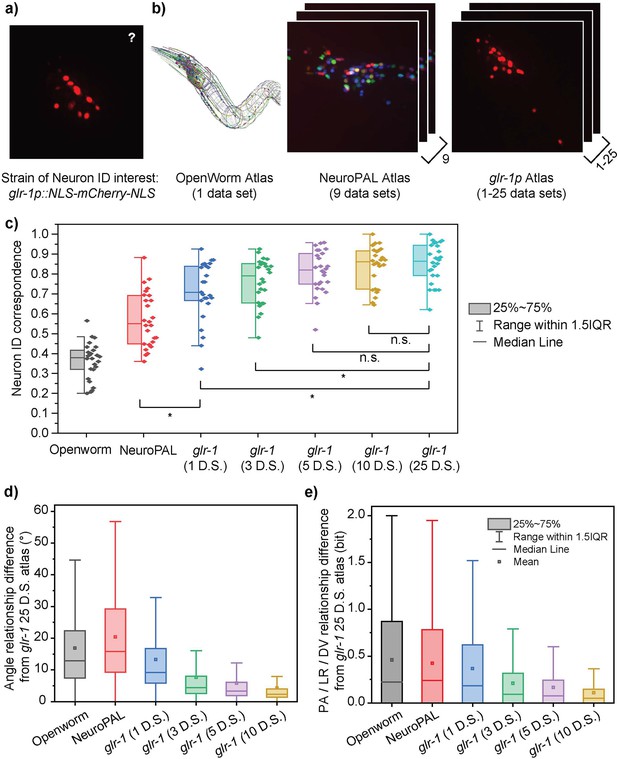
Characterizing the importance of data-specific atlases.
(a, b) Several example atlases (b) are compared on their performance in neuron ID prediction on the glr-1p::NLS-mCherry-NLS multi-cell images (a). (c) The neuron ID accuracy (correspondence to manual cell annotations) depends greatly on the atlas used. Each data point represents the cell cluster from one animal (n = 26). Best single prediction results are reported. Two-sample t-tests were performed for statistical analysis. The asterisk symbol denotes a significance level of p<0.05. (d, e) Difference of each atlas from the most accurate available atlas (glr-1 25 datasets) in terms of pairwise angle relationships (d) and PA/LR/DV positional relationships (e). All distributions in panels (d) and (e) had a p-value of <0.0001 for one-sample t-test against zero.
-
Figure 3—source data 1
Neuron ID Accuracy by Atlas.
- https://cdn.elifesciences.org/articles/89050/elife-89050-fig3-data1-v1.xlsx
-
Figure 3—source data 2
Diffrence in Angle Relationships by Atlas.
- https://cdn.elifesciences.org/articles/89050/elife-89050-fig3-data2-v1.xlsx
-
Figure 3—source data 3
Difference in PA/LR/DV Relationships by Atlas.
- https://cdn.elifesciences.org/articles/89050/elife-89050-fig3-data3-v1.xlsx
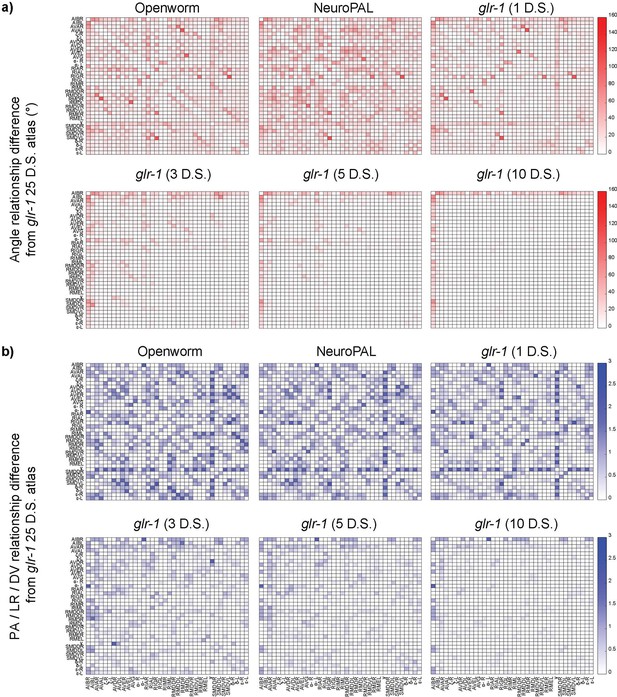
A more detailed visual representation of the difference of each atlas from the best available atlas (glr-1 from 25 datasets [D.S.]).
(a) Differences in angular relationships. The red color intensity indicates the angle differences of neuron pair vectors in the particular atlas and those in the best available atlas. (b) Differences in PA/LR/DV relationships. The blue color intensity (ranging from 0 to 3) indicates the summed absolute differences in the three pairwise relationships between the atlas and the best available atlas.
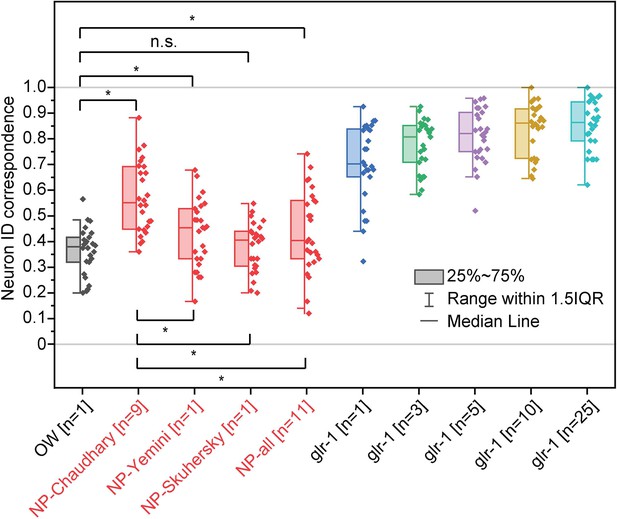
Comparison of neuron ID correspondences resulting from additional atlases—atlases driven from NeuroPAL neuron positional data from multiple sources (Chaudhary et al., 2021Chaudhary et al., 2021; Skuhersky et al., 2022; Yemini et al., 2021) in red compared to other atlases in Figure 3.
Two-sample t-tests were performed for statistical analysis (n=26). The asterisk symbol denotes a significance level of p<0.05, and n.s. denotes no significance. OW: atlas driven by data from OpenWorm project, NP-source: NeuroPAL atlas driven by data from the source. NP-Chaudhary atlas corresponds to NeuroPAL atlas in Figure 3.
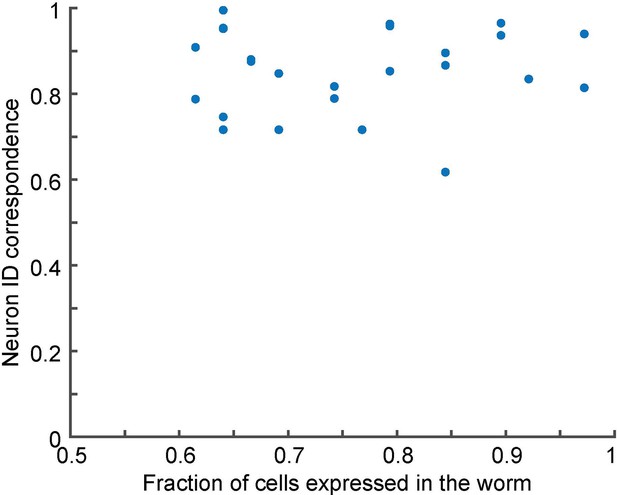
No correlation between the degree of mosaicism (fraction of cells expressed in the worm) and neuron ID correspondence.
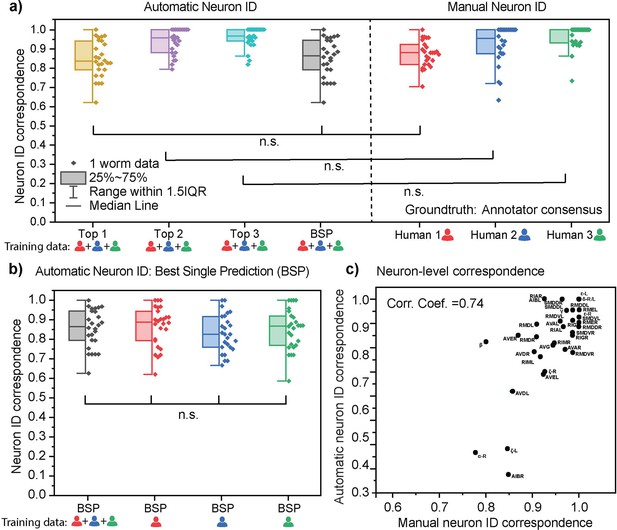
Characterization of neuron identification accuracy using CRF_ID 2.0.
(a) Side-by-side comparison of automated neuron ID accuracy and manual neuron ID accuracy. Each datapoint represents the cell cluster from an animal. For automated neuron ID, top 1, 2, 3 results are from an iterative operation of the CRF_ID algorithm, and the best single prediction (BSP) results are from a single run. The atlas is a compiled data from three different annotators. The ground truth labels are defined by the consensus of the three annotators. Two-sample t-tests were performed for statistical analysis (n=26). (b) No significant differences in best single prediction accuracies are found when using atlases derived from data annotated by different annotators. One-way ANOVA was performed for statistical analysis. (c) There is a positive correlation between the automatic and manual neuron ID accuracy of each neuron.
-
Figure 4—source data 1
Neuron ID Accuracy of CRF_ID and Manual Annotations.
- https://cdn.elifesciences.org/articles/89050/elife-89050-fig4-data1-v1.xlsx
-
Figure 4—source data 2
CRF_ID Accuracy of Atlases Constructed by Different Annotators.
- https://cdn.elifesciences.org/articles/89050/elife-89050-fig4-data2-v1.xlsx
-
Figure 4—source data 3
Correlation between Automatic and Manual Neuron ID Accuracy.
- https://cdn.elifesciences.org/articles/89050/elife-89050-fig4-data3-v1.xlsx
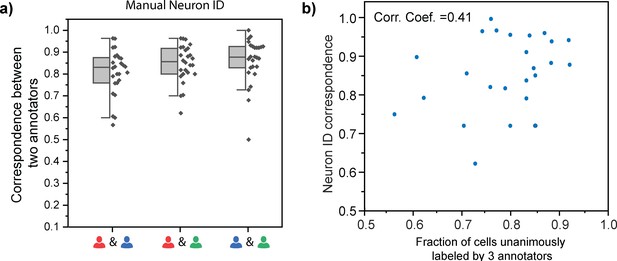
Characterization of manual annotations.
(a) Correspondence between any two among the three annotators. (b) Slight correlation between the fraction of cells unanimously labeled by three annotators and neuron ID correspondence.
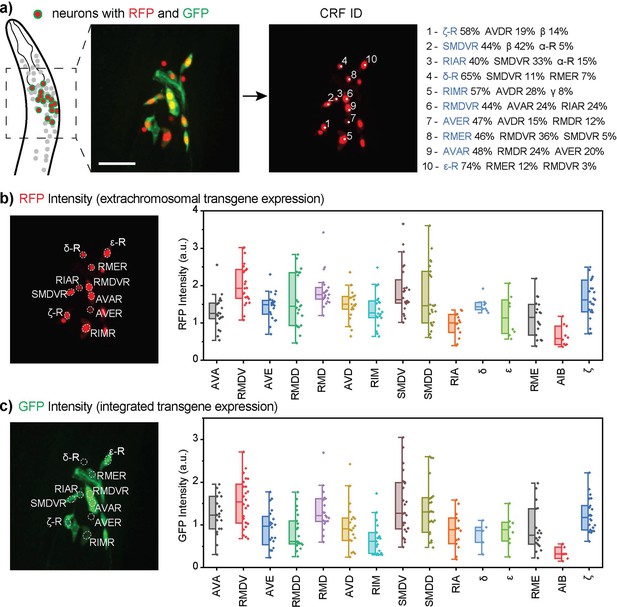
Multi-cell neuron identification in in vivo gene expression analysis.
(a) CRF_ID 2.0 facilitates multi-cell annotation by providing top 3 most likely neuron labels for each cell, from which the user makes the final decision. (b, c) The example strain contained extrachromosomal (b) and integrated reporter transgenes for glr-1 (c). Plotted are the neuron-specific gene expression levels displayed as the normalized fluorescence intensities of selected neurons. The neurons labels on the x-axis are listed in the descending order of single-cell RNA sequencing expression levels reported by CeNGEN (Taylor et al., 2021). Box plots indicate median, quartiles and whiskers indicate 1.5 IQR. Data points indicate signals from individual worms (n=30). The images are showing half volume (right side) of the specimen for illustration. a.u.: arbitrary unit.
-
Figure 5—source data 1
Neuron-Specific Extrachromosomal Transgene Expression.
- https://cdn.elifesciences.org/articles/89050/elife-89050-fig5-data1-v1.xlsx
-
Figure 5—source data 2
Neuron-Specific Integrated Transgene Expression.
- https://cdn.elifesciences.org/articles/89050/elife-89050-fig5-data2-v1.xlsx
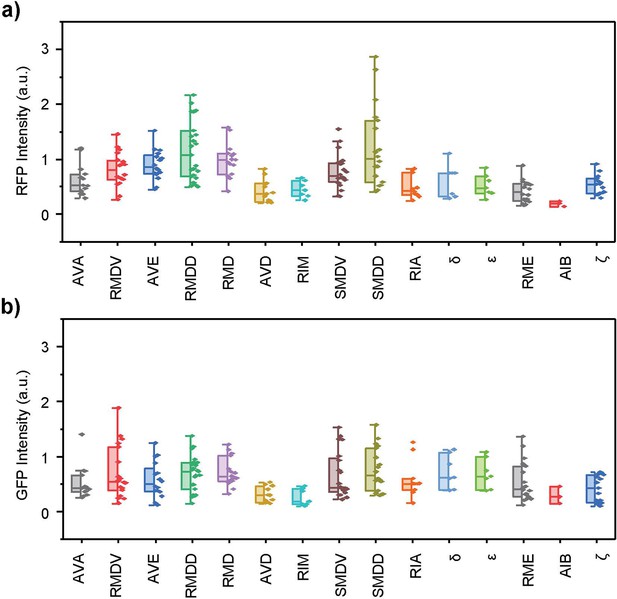
Neuron-specific expressions of the glr-1 gene for neurons on the ‘dim’ side of the specimen.
These left/right paired neurons are on the side of the C. elegans farther away from the objective. (a) Extrachromosomal transgene expression. (b) Integrated transgene expression.
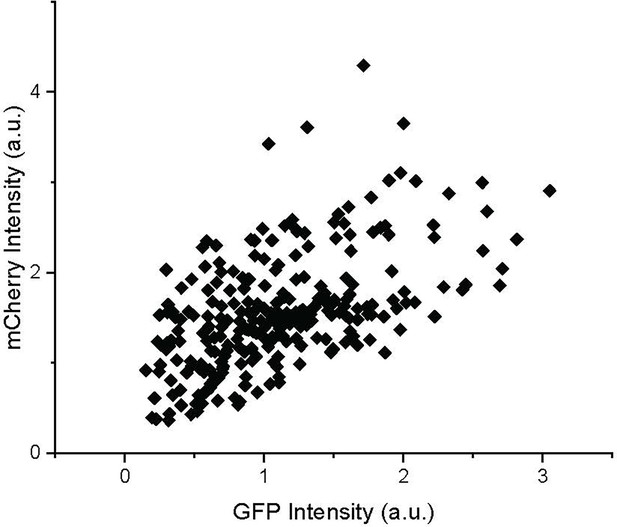
High correlation between extrachromosomal (mCherry) and integrated (GFP) transgene expressions.
Each data point indicates the GFP and mCherry (RFP) intensities of a single neuron.

Figure3- figure supplement 2.
Comparison of neuron ID correspondences resulng from addional atlases- atlases driven from NeuroPAL neuron posional data from mulple sources (Chaudhary et al., Yemini et al., and Skuhersky et al.) in red compared to other atlases in Figure 3. Two sample t-tests were performed for stascal analysis. The asterisk symbol denotes a significance level of p<0.05, and n.s. denotes no significance. OW: atlas driven by data from OpenWorm project, NP-source: NeuroPAL atlas driven by data from the source. NP-Chaudhary atlas corresponds to NeuroPAL atlas in Figure 3.
Tables
Reagent type (species) or resource | Designation | Source or reference | Identifiers | Additional information |
---|---|---|---|---|
Strain, strain background (Caenorhabditis elegans) | ZC3292 | This work | yxEx1701[glr-1p::GCaMP6s, glr-1p::NLS-mCherry-NLS] | Available upon request from Yun Zhang |
Strain, strain background (C. elegans) | ZC3612 | This work | lin-15B&lin-15A(n765) kyIs30[glr-1::GFP, lin-15(+)] X; yxEx1933[glr-1p::NLS-mCherry-NLS] | Available upon request from Yun Zhang |