Unsupervised discovery of family specific vocal usage in the Mongolian gerbil
Figures
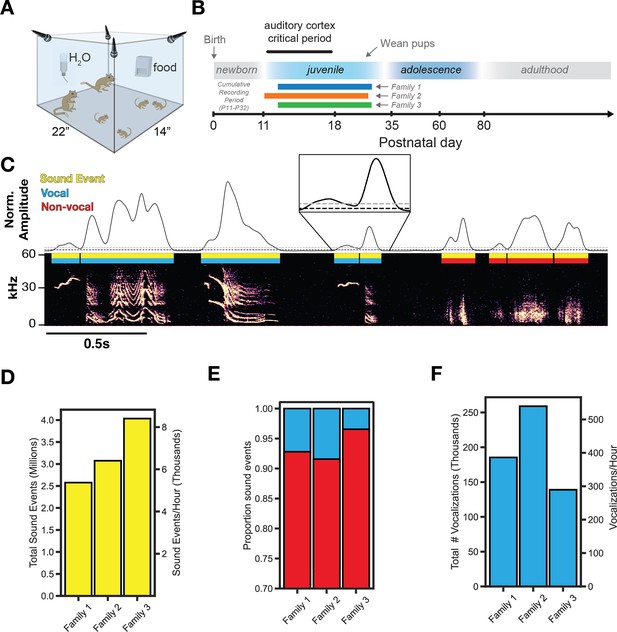
Longitudinal familial audio recording.
(A) Recording apparatus. Four ultrasonic microphones sampled at 125 kHz continuously recorded a family in an enlarged environment. (B) Experiment timeline. Three gerbil families with the same family composition (2 adults, 4 pups) were recorded continuously for 20 days. (C) Extraction of sound events from raw audio using sound amplitude thresholding (Gray threshold = ‘th_2’, black threshold = ‘th_1’ and ‘th_3’; see Methods). Vocalizations (n=583,237) are separated from non-vocal sounds (n=9,684,735) using a threshold on spectral flatness (Figure 1—figure supplement 1 see Methods). (D) Summary of total sound event emission and average emission per hour. (E) Proportion of all sound events that are vocal or non-vocal sounds. (F) Summary of total vocalization emission and average emission per hour.
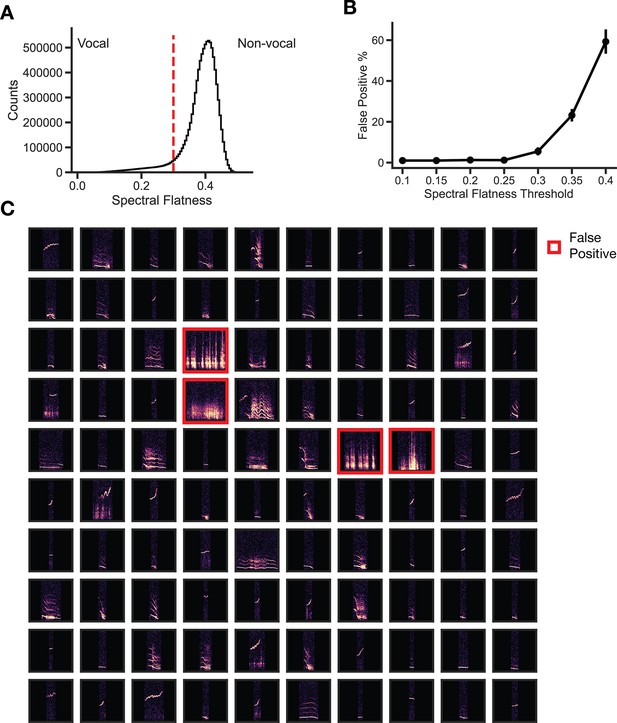
Vocalization extraction.
(A) Distribution of the spectral flatness of all sound events extracted. Vertical red line = 0.3. (B) False-positive percentage derived from human labeling of noise detected in randomly sampled 10x10 vocalization matrices. Random samples came from putative vocalizations with spectral flatness less than a moving threshold of 0.1, 0.15, 0.2, 0.25, 0.3, 0.35, 0.4 (n=10 random samples per group). (C) Example random sample matrix of vocalizations with spectral flatness <0.3. Four false positives observed in this grid.
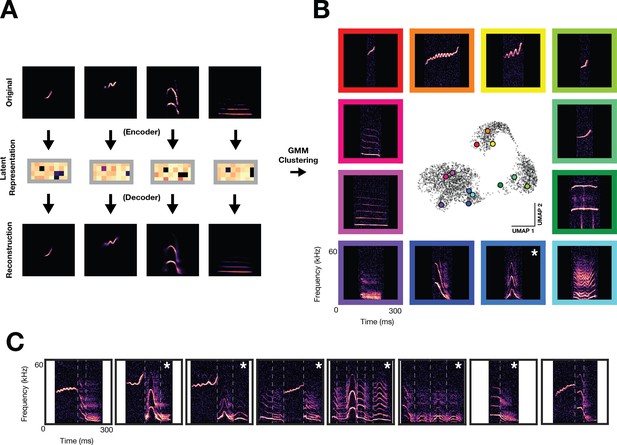
Unsupervised discovery of the Mongolian gerbil vocal repertoire.
Variational autoencoder and clustering. (A) Vocalization spectrograms (top) are input to a variational autoencoder (VAE) which encodes the spectrogram as a 32-D set of latent features (middle). The VAE learns latent features by minimizing the difference between original spectrograms and spectrograms reconstructed from the latent features by the VAE decoder (bottom). A gaussian mixture model (GMM) was trained on the latent features to cluster vocalizations into discrete categories. (B) Representative vocalizations from 12 distinct GMM clusters featuring monosyllabic vocalizations are shown surrounding a UMAP embedding of the latent features. Asterisk denotes vocal type not previously characterized. (C) Examples of multisyllabic vocalizations. White vertical lines indicate boundaries of monosyllabic elements. Asterisks denote multisyllabic vocal types not previously characterized.
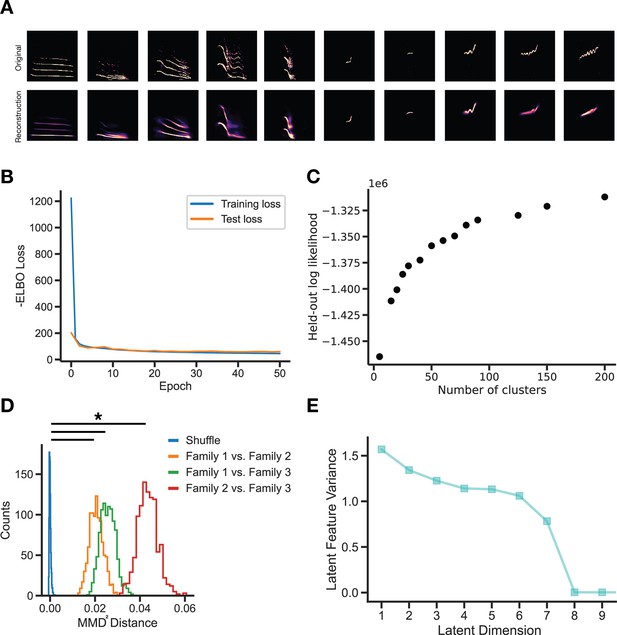
VAE training and GMM clustering.
(A) VAE reconstruction examples for different vocalization types. (B) VAE test and training loss show plateau in performance after a few epochs (model used in this study is epoch 50). (C) GMM held-out log likelihood as a function of the number of clusters used during model training. Seventy clusters were used in this study. (D) MMD2 permutation comparisons. All family comparisons occur greater than expected by chance (p<0.01, independent t-test). (E) Number of latent features used by VAE.
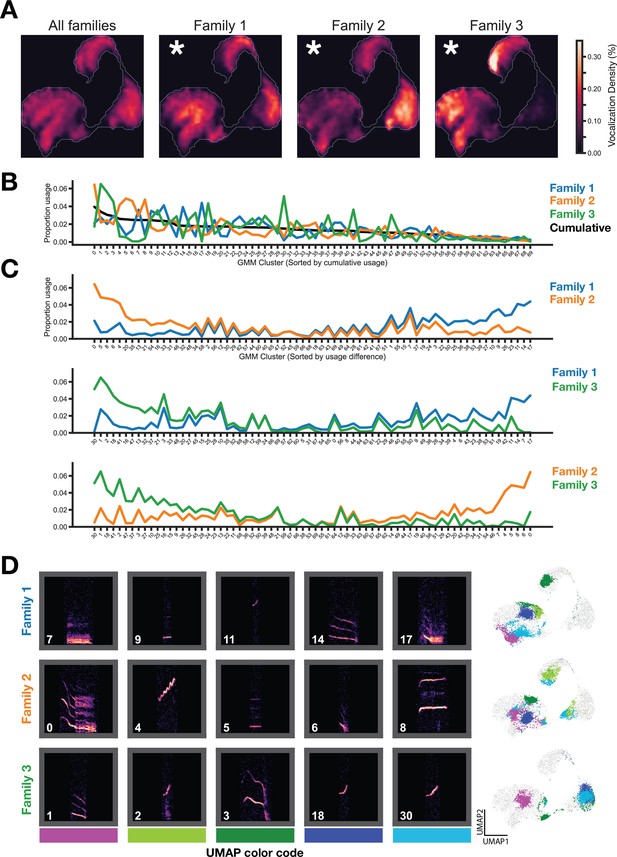
Family specific vocal usage.
(A) UMAP probability density plots (axes same as Figure 2B) show significant differences between family repertoires (p<0.01, MMD permutation test on latent space; see Methods). (B) GMM vocal cluster usage by family. Clusters sorted by cumulative usage across all families. Families show distinct usage patterns of different vocal clusters. (C) Clusters are resorted by the usage difference between families. (D) Spectrogram examples from top differentially used clusters (left) and location of clusters in embedding space (right).
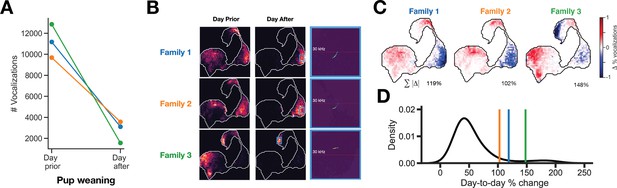
Pup removal biases vocal repertoire usage.
(A) Pup weaning causes a consistent reduction in vocal emission across families. (B) UMAP probability densities of the vocal repertoire pre and post pup weaning. Example vocalization from high-density post-weaning regions. (C). Difference in probability densities and total percent-change in repertoire pre-post pup weaning. (D) Quantification of day-to-day percent-change throughout the experiment shows that the percent-change magnitude observed in C is rare.
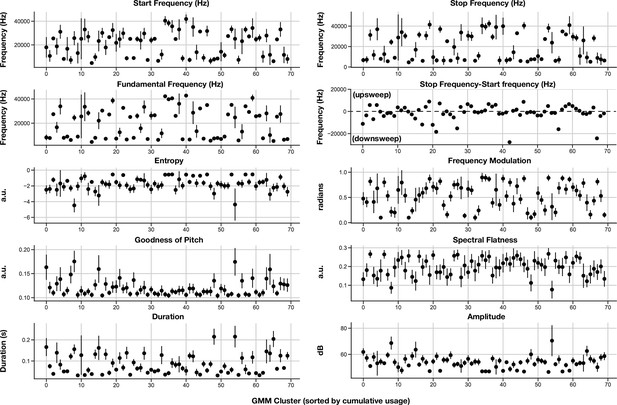
Acoustic features for GMM clusters.
Acoustic features computed on the top 100 most probable vocalizations from each GMM cluster. Mean values ± standard deviation shown. Details on acoustic feature calculation are described in the Methods section.
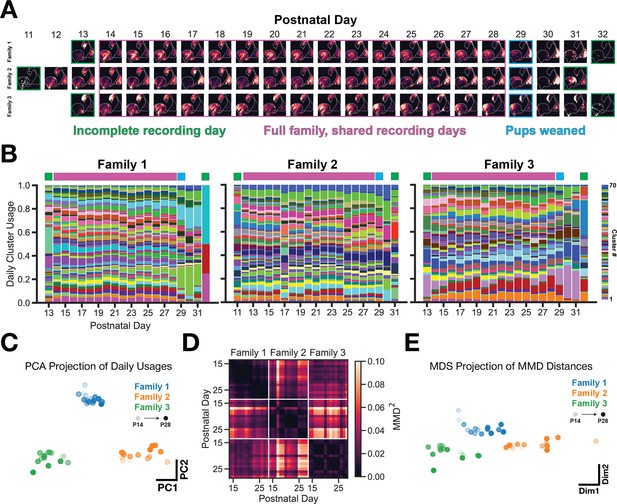
Vocal usage differences remain stable across days of development.
(A) UMAP probability density plots for each day of the recording, across families. Purple box indicated recording days that are shared across families. These days are used for subsequent analyses in C-E. (B) GMM vocal cluster usage per day. Usages are normalized on a per-day basis. A unique color is used for each cluster type. (C) PCA projection of daily usages within the purple (shared recording days) period showing that families use a unique subset of clusters stably across days. (D) Maximum Mean Discrepancy (MMD) distance between VAE latent distributions of vocalizations between days and across families. (E) Multidimensional scaling projection of MMD matrix from (D). Family vocal repertoires are distinct and remain so across days.
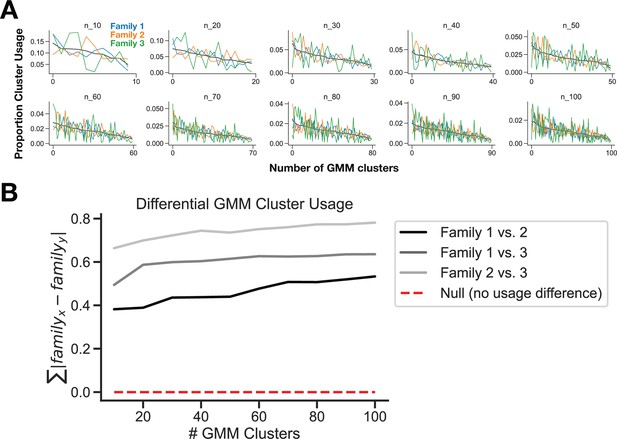
Family specific cluster usages do not depend on GMM cluster size.
(A) GMM cluster usages for each family over a range of GMM cluster sizes. (B) Quantification of pairwise cluster usage differences showing stability of family differences over all cluster sizes.
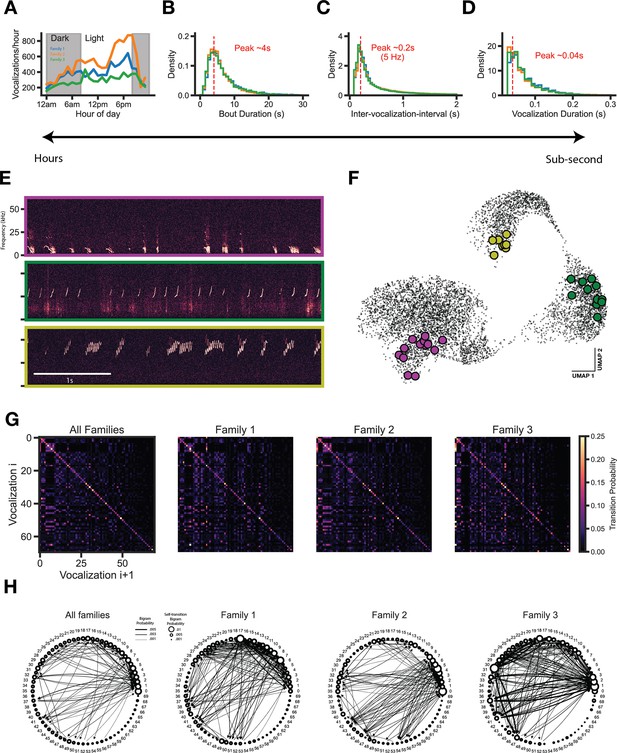
Transition structure, but not emission structure, shows family specific differences.
(A) Vocalizations are emitted in a diurnal cycle. (B) Vocalizations consistently occur in seconds-long bouts across families. (C) Vocalization intervals (onset-to-onset) are consistent across families. (D) Vocalization durations are consistent across families. (E) Raw data examples of bouts. (F) Bouts typically occupy a similar area of vocal space. (G) Vocal cluster transition matrix. Vocalizations strongly favor self-transition. (H) Bigram probability graph. Self and other vocalization transition tendencies show family specific transitions (edges > 0.001 usage shown).
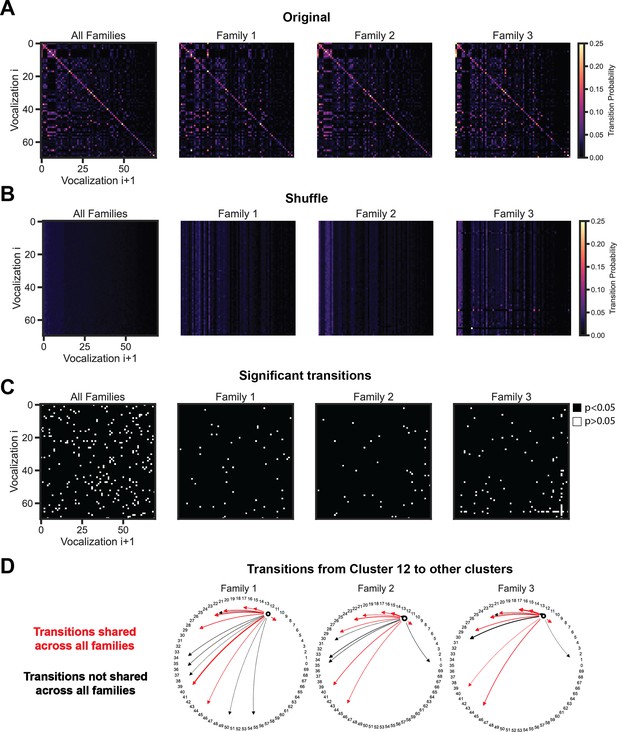
Vocalization transitions are non-random and family specific.
(A) Vocal cluster transition matrix (same as Figure 5G). (B) Random transition matrix, computed after shuffling vocal cluster label sequence. (C) Transitions that occur greater than expected by chance (1000-iteration random shuffle with one-sample t-test and post hoc Benjamini-Hochberg multiple comparisons correction; see Methods). (D) Most common transitions (>0.04% usage) from cluster 12 (roughly equally used across all families) to other clusters. Red lines indicate transitions that are shared across families, black lines indicate unique family specific transitions.