Statistical learning shapes pain perception and prediction independently of external cues
Figures
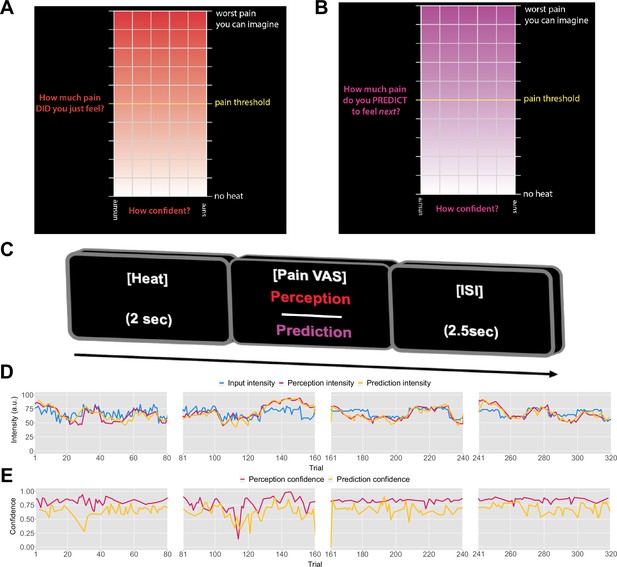
Task design.
On each trial, each participant received a thermal stimulus lasting 2s from a sequence of intensities. This was followed by a perception (A) or a prediction (B) input screen, where the y-axis indicates the level of perceived/predicted intensity (0–100) centred around participant’s pain threshold, and the x-axis indicates the level of confidence in one’s perception (0–1). The inter-stimulus interval (ISI; black screen) lasted 2.5s (trial example in C). (D) Example intensity sequences are plotted in green, participant’s perception and prediction responses are in red and black, respectively. (E) Participant’s confidence rating for perception (red) and prediction (black) trials.
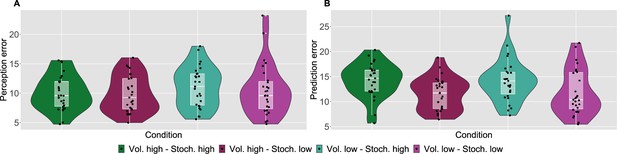
Participant’s model-naive performance in the task.
Violin plots of participant root mean square error (RMSE) for each condition for A: rating and B: prediction responses as compared with the input. Lower and upper hinges correspond to the first and third quartiles of partipants’ errors (the upper/lower whisker extends from the hinge to the largest/smallest value no further than 1.5 * ”Interquartile range” from the hinge); the line in the box corresponds to the median. Each condition has N=27 particpants.
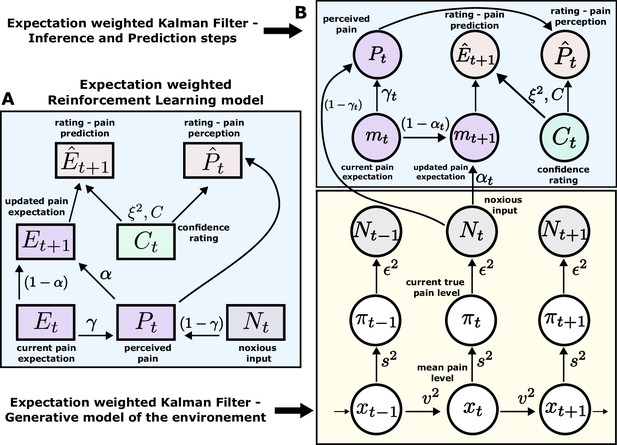
Expectation weighted models.
Computational models used in the main analysis to capture participants’ pain perception () and prediction () ratings. Both types of ratings are affected by confidence rating () on each trial. (A) In the reinforcement learning model, participant’s pain perception () is taken to be weighted sum of the current noxious input () and their current pain expectation (). Following the noxious input, participant updates their pain expectation (). (B) In the Kalman filter model, a generative model of the environment is assumed (yellow background) - where the mean pain level () evolves according to a Gaussian random walk (volatility ). The true pain level on each trial () is then drawn from a Gaussian (stochasticity ). Lastly, the noxious input, , is assumed an imperfect indicator of the true pain level (subjective noise ). Inference and prediction steps are depicted in a blue box. Participant’s perceived pain is a weighted sum of expectation about the pain level () and current noxious input (). Following each observation, , participant updates their expectation about the pain level ().
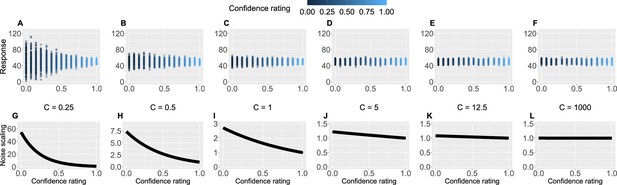
Confidence scaling factor demonstration.
(A–F) For a range of values of the confidence scaling factor , we simulated a set of typical responses a participant would make for various levels of confidence ratings. The belief about the mean of the sequence is set at 50, while the response noise at 10. The confidence scaling factor effectively scales the response noise, adding or reducing response uncertainty. (G–L) The effect of different levels of parameter on noise scaling. As increases the effect of confidence is diminished.
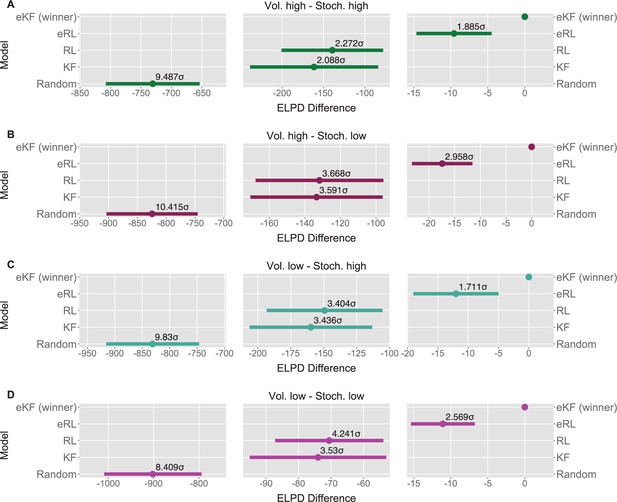
Model comparison for each sequence condition (A–D).
The dots indicate the expected log point-wise predictive density (ELPD) difference between the winning model (eKF - expectation weighted Kalman filter) and every other model. The line indicates the standard error (SE) of the difference. The non-winning models’ ELPD differences are annotated with the ratio between the ELPD difference and SE indicating the sigma effect, a significance heuristic.
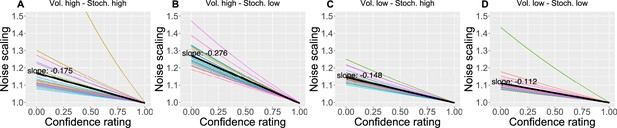
The effect of the confidence scaling factor on noise scaling for each condition.
(A–D) Each coloured line corresponds to one participant, with the black line indicating the mean across all participants. The mean slope for each condition is annotated.
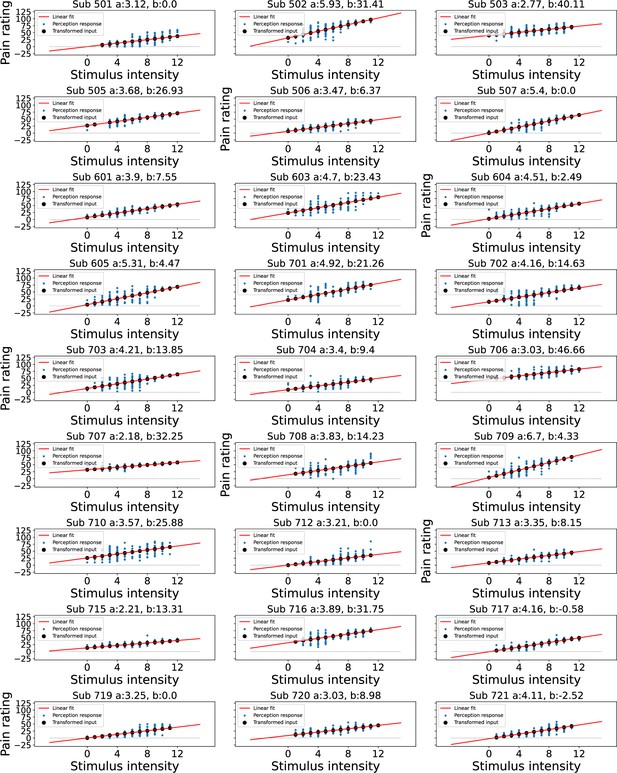
Linear transformation of the input at perception trials.
Blue dots indicate participant’s perception responses for a given level of stimulus intensity, black dots indicate transformed intensity values, a linear least squares regression was performed to achieve the best fitting line through participant’s responses as shown in red, the intercept was constrained>0.
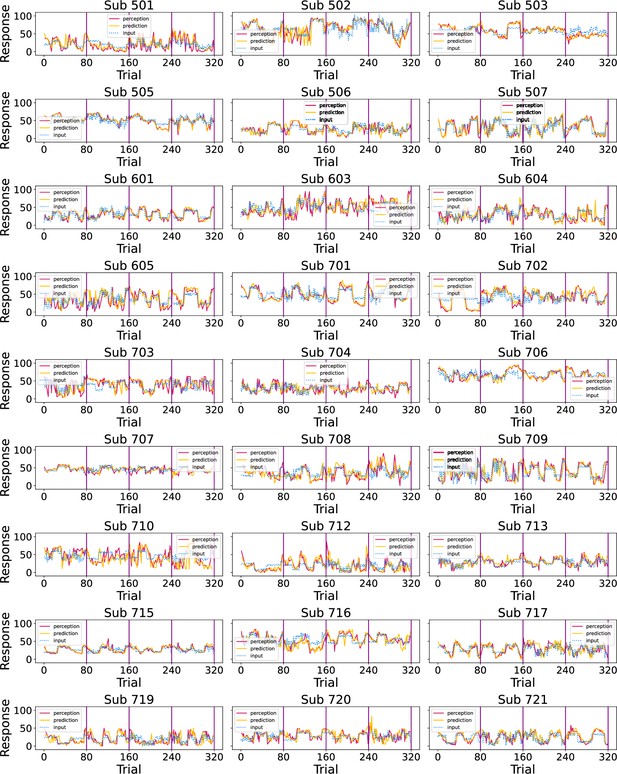
Participants’ responses (red - perception; green - prediction) to the noxious input (dotted line) sequences.
Vertical purple lines mark the end of each condition.
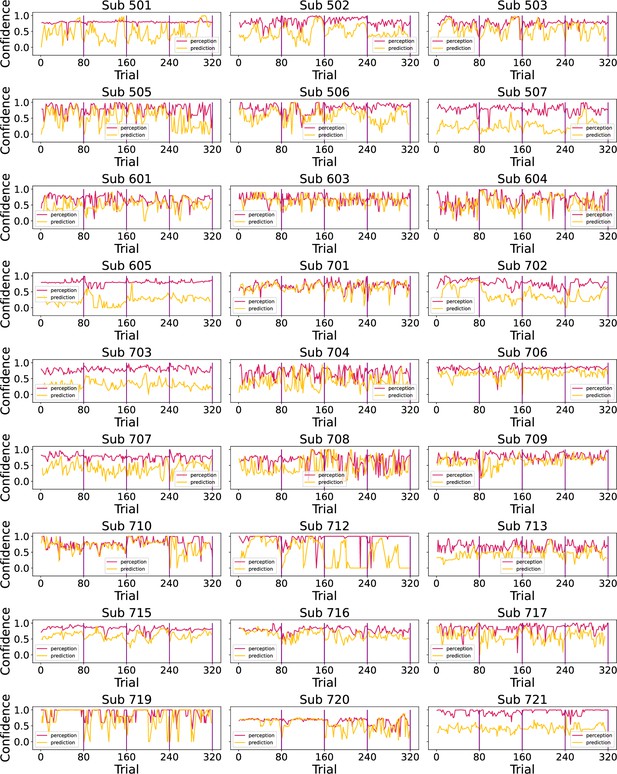
Participants’ confidence ratings (red - perception; green - prediction) during the task.
Vertical purple lines mark the end of each condition.
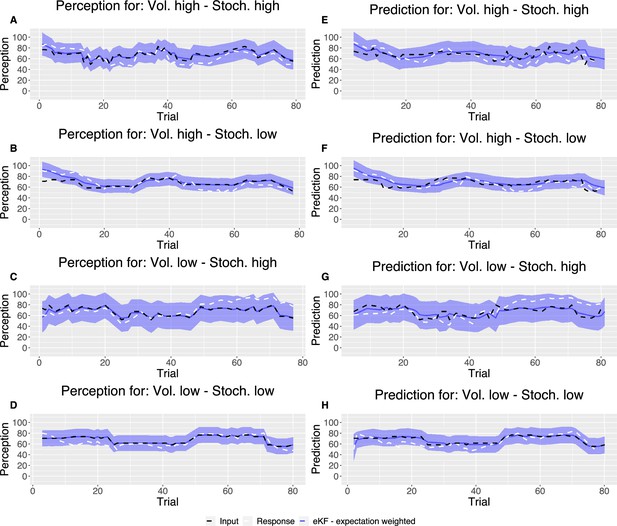
Example plot of the input sequences (black) for each condition, one participant’s responses (white) and the winning, expectation weighted Kalman filter (eKF), model predictions (blue) including 95% confidence intervals (shaded blue) for (A–D) perception and (E–H) prediction.
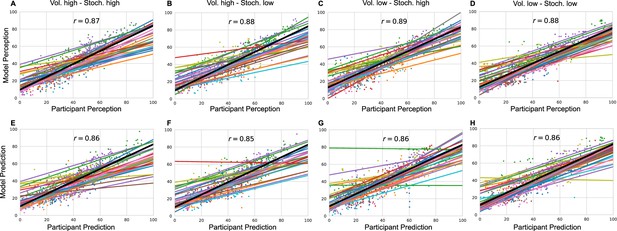
Model responses against participants’ responses for each condition and each response type (A–D) perception and (E–H) prediction.
The annotated value is the grand mean correlation across subjects for each condition and response type.

Parameter recovery average SD for: (A) eRL; (B) RL; (C) eKF; (D) KF; (E) Random model.
The average SD is plotted as a function of simulation number averaged across 500 permutations of ≈100 simulations. The coloured shading corresponds to 1 SD around the average error.

Parameter recovery scatter plot for expectation weighted reinforcement learning (eRL) model from ≈100 simulations for: (A) ɑ; (B) ɣ; (C) ξ; (D) E0; (E) C parameter.

Parameter recovery scatter plot for reinforcement learning (RL) model from ≈100 simulations for: (A) ɑ; (B) ξ; (C) E0; (D) C parameter.

Parameter recovery scatter plot for expectation weighted Kalman filter (eKF) model from ≈100 simulations for: (A) ε; (B) s; (C) v; (D) ξ; (E) E0; (F) w0; (G) C parameter.

Parameter recovery scatter plot for Kalman filter (KF) model from ≈100 simulations for: (A) s; (B) v; (C) ξ; (D) E0; (E) w0; (F) C parameter.
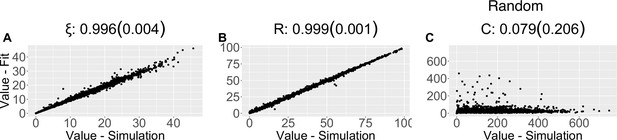
Parameter recovery scatter plot for random model from ≈100 simulations for: (A) ξ; (B) R; (C) C parameter.
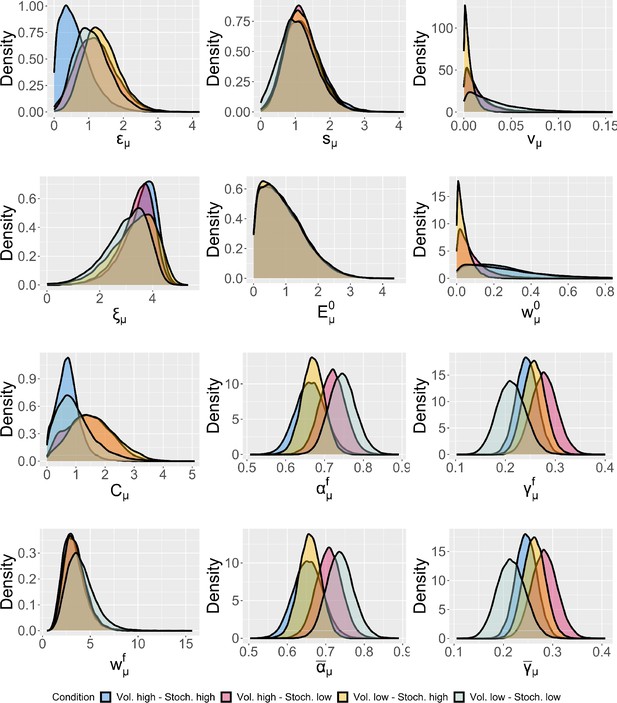
Group-level distributions for parameters for each condition for the expectation weighted Kalman filter (eKF) model.
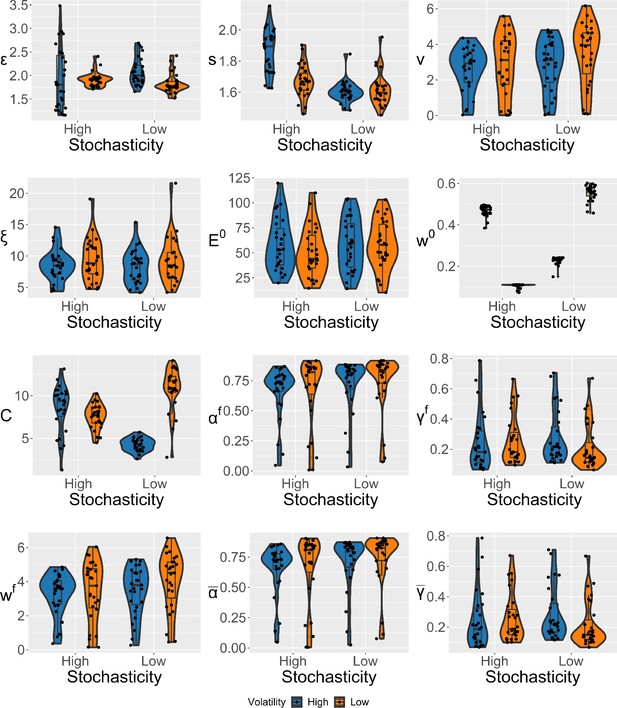
Violin plots (and box-plots) of individual-level parameters for each condition in the winning expectation weighted Kalman filter (eKF) model.
Lower and upper hinges correspond to the first and third quartiles of partipants’ errors (the upper/lower whisker extends from the hinge to the largest/smallest value no further than 1.5 * ”Interquartile range” from the hinge); the line in the box corresponds to the median. Each condition has N=27 particpants.
Tables
Within-subjects effects from repeated measures ANOVA of participant’s RMSE scores with stochasticity, volatility, and response type factors.
SS - sum of squares, MS - mean square, RMSE - root mean square error
Effect | SS | df | MS | F | p | ||
---|---|---|---|---|---|---|---|
Volatility | 10.714 | 1 | 10.714 | 0.960 | 0.336 | 0.007 | 0.036 |
Residuals | 290.166 | 26 | 11.160 | ||||
Stochasticity | 113.964 | 1 | 113.964 | 19.939 | <0.001* | 0.074 | 0.434 |
Residuals | 148.603 | 26 | 5.715 | ||||
Type | 365.000 | 1 | 365.000 | 85.109 | <0.001* | 0.237 | 0.766 |
Residuals | 111.503 | 26 | 4.289 | ||||
Volatility × stochasticity | 0.006 | 1 | 0.006 | 5.688e-4 | 0.981 | 3.723e-6 | 2.188e-5 |
Residuals | 261.912 | 26 | 10.074 | ||||
Volatility × type | 7.313 | 1 | 7.313 | 3.196 | 0.085 | 0.005 | 0.109 |
Residuals | 59.487 | 26 | 2.288 | ||||
Stochasticity × type | 63.662 | 1 | 63.662 | 29.842 | <0.001* | 0.041 | 0.534 |
Residuals | 55.466 | 26 | 2.133 | ||||
Volatility × stochasticity × type | 1.356 | 1 | 1.356 | 0.704 | 0.409 | 8.807e-4 | 0.026 |
Residuals | 50.060 | 26 | 1.925 |
-
* indicates statistical significance at 0.05 level.
Post hoc comparisons for the repeated measures ANOVA’s interaction effect of stochasticity × type.
95%CI for mean diff. | |||||||
---|---|---|---|---|---|---|---|
Mean diff. | Lower | Upper | SE | t | pbonf | ||
High, perception | Low, perception | 0.367 | −0.687 | 1.421 | 0.381 | 0.963 | 1.000 |
High, prediction | −3.686 | −4.636 | −2.735 | 0.345 | −10.688 | <0.001* | |
Low, prediction | −1.147 | −2.329 | 0.034 | 0.430 | −2.665 | 0.062 | |
Low, perception | High, prediction | −4.053 | −5.234 | −2.871 | 0.430 | −9.415 | <0.001* |
Low, prediction | −1.514 | −2.464 | −0.564 | 0.345 | −4.390 | <0.001* | |
High, prediction | Low, prediction | 2.539 | 1.484 | 3.593 | 0.381 | 6.658 | <0.001* |
-
* indicates statistical significance at 0.05 level.
Pearson correlation coefficient (SD) from the parameter recovery analysis for each model.
eRL | |||||||
---|---|---|---|---|---|---|---|
(SD) | 0.685 (0.113) | 0.92 (0.049) | 0.993 (0.005) | 0.723 (0.093) | 0.481 (0.131) | ||
RL | |||||||
(SD) | 0.842 (0.081) | 0.993 (0.004) | 0.625 (0.107) | 0.455 (0.133) | |||
eKF | |||||||
(SD) | 0.742 (0.1) | 0.531 (0.13) | 0.745 (0.09) | 0.986 (0.075) | 0.849 (0.118) | 0.309 (0.179) | 0.472 (0.123) |
KF | |||||||
(SD) | 0.605 (0.129) | 0.589 (0.117) | 0.993 (0.005) | 0.585 (0.157) | 0.298 (0.18) | 0.442 (0.146) | |
Random model | |||||||
(SD) | 0.996 (0.004) | 0.999 (0.001) | 0.079 (0.206) |
Confusion matrix from the model recovery analysis based on ≈100 simulations.
The y-axis indicates which model simulated the dataset, while the x-axis indicates which model fit the data based on leave-one-out information criterion (LOOIC).
eRL | RL | eKF | KF | Random | ||
---|---|---|---|---|---|---|
Simulated | eRL | 0.327 | 0.173 | 0.404 | 0.096 | 0.000 |
RL | 0.223 | 0.234 | 0.223 | 0.319 | 0.000 | |
eKF | 0.382 | 0.067 | 0.427 | 0.124 | 0.000 | |
KF | 0.229 | 0.281 | 0.281 | 0.208 | 0.000 | |
Random | 0.292 | 0.000 | 0.358 | 0.000 | 0.349 | |
Fit |
Model comparison results for each condition.
Condition | Model name | ELPD difference | SE difference | Sigma effect | LOOIC |
---|---|---|---|---|---|
Vol. high Stoch. high | eKF - expectation weighted | 0.000 | 0.000 | 15748.389 | |
eRL - expectation weighted | –9.560 | 5.071 | 1.885 | 15767.509 | |
RL | –139.407 | 61.362 | 2.272 | 16027.202 | |
KF | –161.444 | 77.335 | 2.088 | 16071.277 | |
Random response | –730.600 | 77.009 | 9.487 | 17209.588 | |
Vol. high Stoch. low | eKF - expectation weighted | 0.000 | 0.000 | 15682.115 | |
eRL - expectation weighted | –17.439 | 5.896 | 2.958 | 15716.993 | |
RL | –131.817 | 35.936 | 3.668 | 15945.749 | |
KF | –133.464 | 37.171 | 3.591 | 15949.042 | |
Random response | –824.346 | 79.148 | 10.415 | 17330.807 | |
Vol. low Stoch. high | eKF - expectation weighted | 0.000 | 0.000 | 15990.114 | |
eRL - expectation weighted | –12.027 | 7.029 | 1.711 | 16014.169 | |
RL | –149.338 | 43.874 | 3.404 | 16288.789 | |
KF | –159.738 | 46.485 | 3.436 | 16309.590 | |
Random response | –831.096 | 84.549 | 9.830 | 17652.306 | |
Vol. low Stoch. low | eKF - expectation weighted | 0.000 | 0.000 | 15904.936 | |
eRL - expectation weighted | –11.068 | 4.309 | 2.569 | 15927.072 | |
RL | –70.588 | 16.643 | 4.241 | 16046.111 | |
KF | –74.031 | 20.972 | 3.530 | 16052.997 | |
Random response | –901.792 | 107.244 | 8.409 | 17708.519 |
Bulk and tail effective sample size (ESS) values for vol. high - stoch. high.
Model | Param. | ESS (bulk) | ESS (tail) |
---|---|---|---|
eRL | 58.166 | 47.491 | |
90.5 | 79.142 | ||
54.655 | 137.729 | ||
31.233 | 47.726 | ||
39.509 | 49.335 | ||
RL | 56.22 | 36.057 | |
99.642 | 52.599 | ||
126.757 | 467.373 | ||
31.322 | 36.92 | ||
eKF | 89.281 | 83.274 | |
37.723 | 103.977 | ||
94.203 | 429.332 | ||
53.099 | 41.511 | ||
1665.566 | 4593.161 | ||
616458.467 | 467603.626 | ||
31.322 | 47.1 | ||
KF | 101.584 | 55.345 | |
122.76 | 512.134 | ||
114.644 | 54.015 | ||
438.028 | 730.579 | ||
904.643 | 6759.804 | ||
31.457 | 36.763 | ||
Random | 27.939 | 33.982 | |
397.862 | 259.967 | ||
32.334 | 41.271 |
Bulk and tail effective sample size (ESS) values for vol. high - stoch. low.
Model | Param. | ESS (bulk) | ESS (tail) |
---|---|---|---|
eRL | 86.32 | 60.849 | |
235.396 | 373.736 | ||
43.489 | 109.903 | ||
30.471 | 36.664 | ||
42.125 | 55.178 | ||
RL | 49.221 | 40.877 | |
328.761 | 455.542 | ||
63.341 | 111.689 | ||
30.304 | 38.063 | ||
eKF | 227.813 | 363.944 | |
33.393 | 104.395 | ||
376.691 | 1218.299 | ||
45.861 | 37.486 | ||
99526.69 | 148393.383 | ||
567627.288 | 634817.458 | ||
30.438 | 36.66 | ||
KF | 328.005 | 448.632 | |
57.467 | 124.471 | ||
293.426 | 480.255 | ||
164.454 | 598.211 | ||
412979.973 | 354163.251 | ||
30.16 | 38.105 | ||
Random | 28.397 | 32.922 | |
1794.614 | 1170.459 | ||
30.204 | 34.896 |
Bulk and tail effective sample size (ESS) values for vol. low - stoch. high.
Model | Param. | ESS (bulk) | ESS (tail) |
---|---|---|---|
eRL | 43.312 | 40.66 | |
248.885 | 434.44 | ||
49.006 | 85.409 | ||
29.68 | 34.909 | ||
45.37 | 52.755 | ||
RL | 39.911 | 35.351 | |
433.949 | 435.575 | ||
181.442 | 618.317 | ||
29.527 | 36.192 | ||
eKF | 248.848 | 418.003 | |
35.363 | 51.728 | ||
1272.838 | 2427.211 | ||
41.144 | 40.915 | ||
2399.657 | 6854.212 | ||
612283.163 | 531588.25 | ||
29.699 | 34.762 | ||
KF | 423.339 | 417.747 | |
88.749 | 302.863 | ||
58.795 | 47.015 | ||
206.969 | 672.666 | ||
499152.469 | 573964.793 | ||
29.511 | 36.341 | ||
Random | 27.892 | 32.919 | |
269.239 | 106.139 | ||
29.69 | 44.38 |
Bulk and tail effective sample size (ESS) values for vol. low - stoch. low.
Model | Param. | ESS (bulk) | ESS (tail) |
---|---|---|---|
eRL | 57.116 | 40.932 | |
162.472 | 129.413 | ||
43.707 | 117.295 | ||
29.632 | 34.486 | ||
65.497 | 151.548 | ||
RL | 45.892 | 37.244 | |
158.681 | 98.898 | ||
80.406 | 441.719 | ||
29.558 | 35.077 | ||
eKF | 149.16 | 126.209 | |
38.88 | 73.732 | ||
653.635 | 1473.554 | ||
48.883 | 43.445 | ||
2263.547 | 9318.066 | ||
635517.969 | 313426.188 | ||
29.699 | 34.721 | ||
KF | 158.729 | 105.929 | |
71.438 | 457.431 | ||
91.988 | 69.957 | ||
287.835 | 895.249 | ||
527620.655 | 587092.529 | ||
29.527 | 35.147 | ||
Random | 28.474 | 38.123 | |
2426.581 | 1279.66 | ||
29.532 | 34.731 |
Model diagnostics for each condition - estimated Bayesian fraction of missing information (E-BFMI), number of divergent transition E-BFMI values per chain.
Condition | Model | # chains low E-BFMI | # div. transitions | E-BFMI values |
---|---|---|---|---|
HVHS | eRL | 0 | 0 | 0.696 0.713 0.695 0.691 |
RL | 0 | 0 | 0.76 0.748 0.771 0.806 | |
eKF | 0 | 0 | 0.755 0.767 0.771 0.759 | |
KF | 0 | 0 | 0.633 0.596 0.547 0.563 | |
Random | 0 | 0 | 0.842 0.851 0.843 0.835 | |
HVLS | eRL | 0 | 0 | 0.689 0.76 0.69 0.689 |
RL | 0 | 0 | 0.624 0.688 0.688 0.685 | |
eKF | 0 | 0 | 0.741 0.764 0.753 0.779 | |
KF | 0 | 0 | 0.654 0.734 0.689 0.674 | |
Random | 0 | 0 | 0.883 0.779 0.836 0.833 | |
LVHS | eRL | 0 | 0 | 0.73 0.732 0.728 0.702 |
RL | 0 | 0 | 0.719 0.714 0.742 0.7 | |
eKF | 0 | 0 | 0.753 0.755 0.792 0.766 | |
KF | 0 | 0 | 0.75 0.768 0.729 0.754 | |
Random | 0 | 0 | 0.864 0.849 0.883 0.845 | |
LVLS | eRL | 0 | 0 | 0.764 0.762 0.75 0.764 |
RL | 0 | 0 | 0.714 0.764 0.719 0.697 | |
eKF | 0 | 0 | 0.783 0.751 0.772 0.77 | |
KF | 0 | 0 | 0.705 0.695 0.702 0.726 | |
Random | 0 | 0 | 0.835 0.829 0.852 0.847 |